In this course, you will see how to use advanced machine-learning techniques to build more sophisticated recommender systems. Machine Learning is able to provide recommendations and make better predictions, by taking advantage of historical opinions from users and building up the model automatically, without the need for you to think about all the details of the model.

Schenken Sie Ihrer Karriere Coursera Plus mit einem Rabatt von $160 , der jährlich abgerechnet wird. Sparen Sie heute.
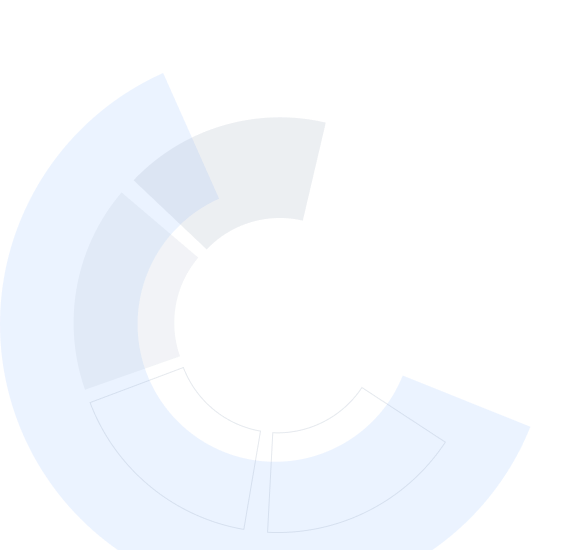

(23 Bewertungen)
Empfohlene Erfahrung
Was Sie lernen werden
You will be able to use some machine learning techniques in order to build more sophisticated recommender systems.
You will learn how to combine different basic approaches into a hybrid recommender system, in order to improve the quality of recommendations.
You will know how to integrate different kinds of side information (about content or context) in a recommender system.
You'll learn how to use factorization machines and represent the input data, mixing together different kinds of filtering techniques.
Wichtige Details

Zu Ihrem LinkedIn-Profil hinzufügen
4 Aufgaben
Erfahren Sie, wie Mitarbeiter führender Unternehmen gefragte Kompetenzen erwerben.
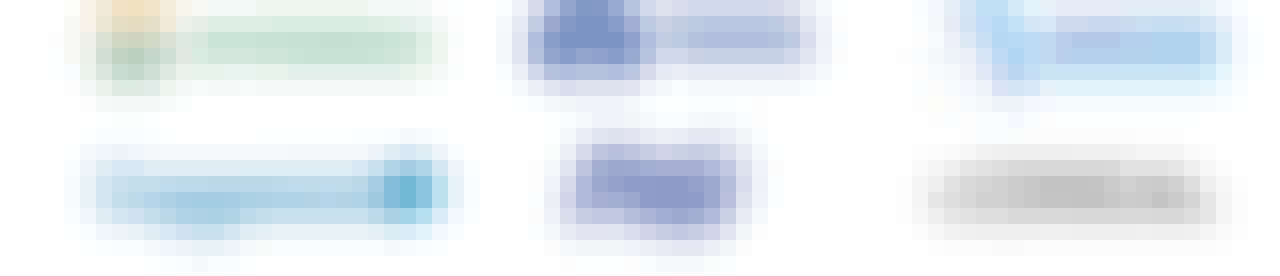
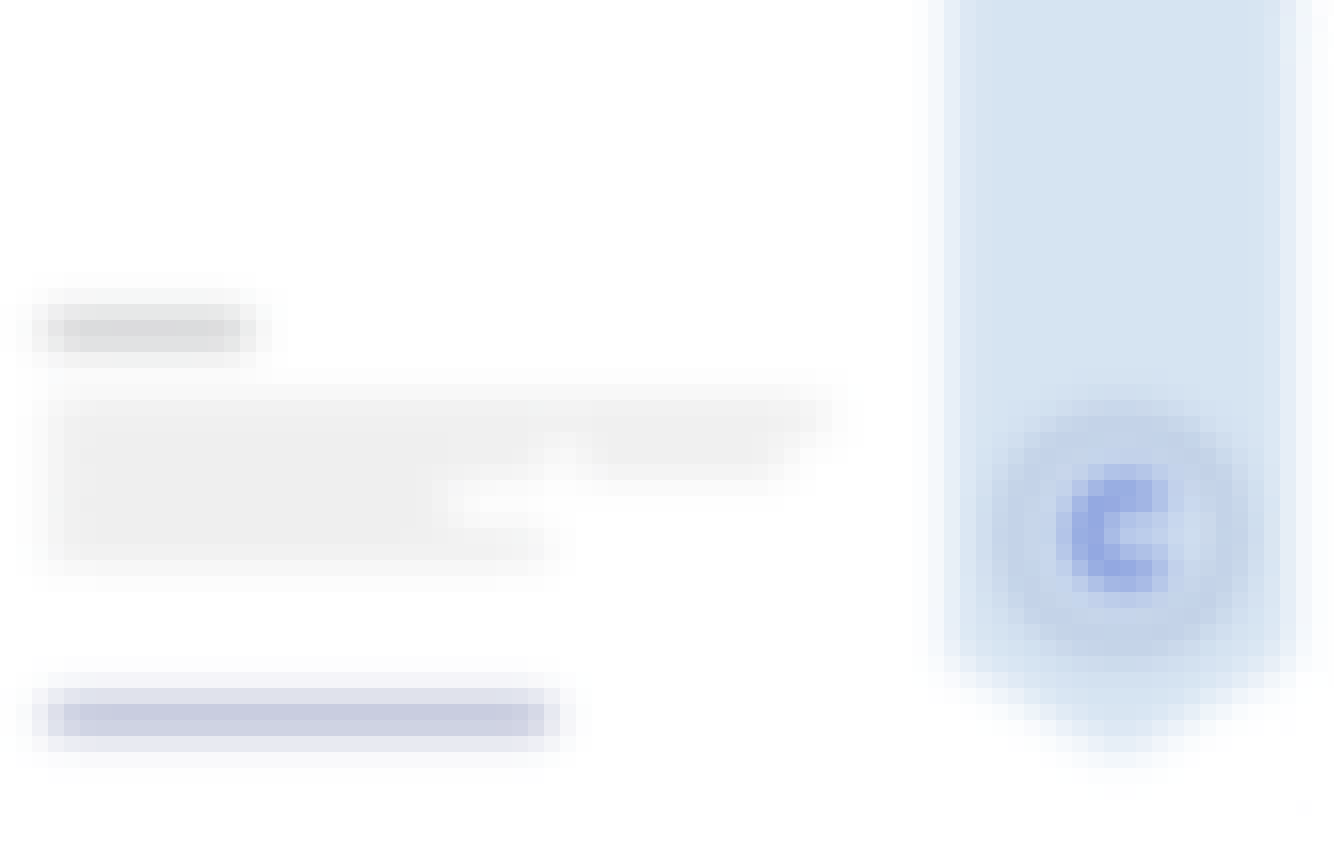
Erwerben Sie ein Karrierezertifikat.
Fügen Sie diese Qualifikation zur Ihrem LinkedIn-Profil oder Ihrem Lebenslauf hinzu.
Teilen Sie es in den sozialen Medien und in Ihrer Leistungsbeurteilung.
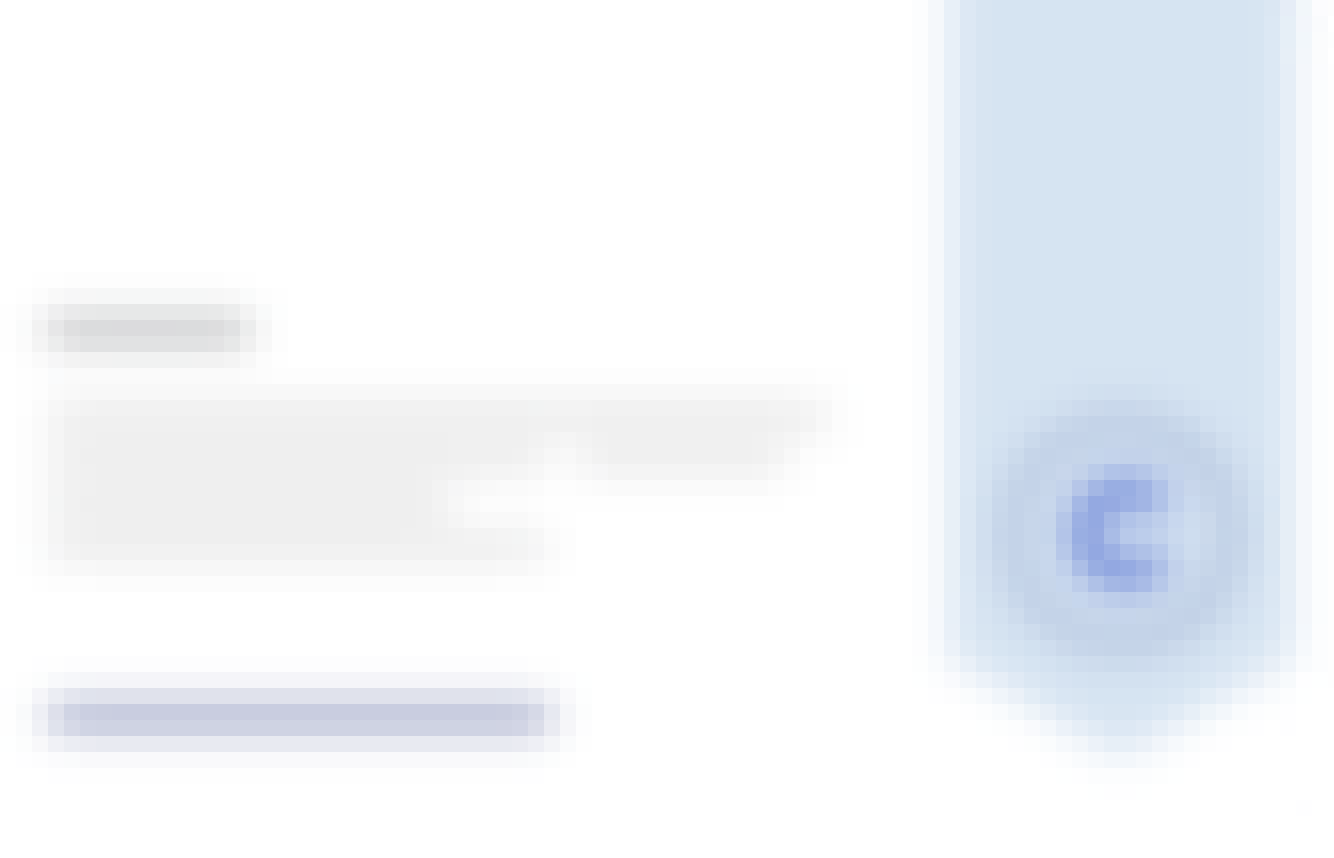
In diesem Kurs gibt es 5 Module
In this first module, we will see how to apply machine learning to collaborative filtering techniques. We will learn how to write an item-based collaborative algorithm which is able to automatically learn the best similarities between items, in order to provide improved recommendations that better match the user opinions predicted by the model with the true user opinions. We will also understand how to train collaborative filtering algorithms that minimize this gap. We will finally define a new error metric based on ranking comparisons, useful to design learning-to-rank algorithms.
Das ist alles enthalten
7 Videos2 Lektüren1 Aufgabe2 peer reviews
In this second module, we will study a new family of collaborative filtering techniques based on dimensionality reduction and matrix factorization approaches, all inspired by SVD (Singular Value Decomposition). We will see the difference between memory-based and model-based recommender systems, discussing their limitations and advantages. In particular, we will learn how to turn basic matrix factorization algorithms from memory-based into model-based approaches. We will also analyse a new important parameter, the number of latent features. We will learn how to choose the correct number of latent features in order to provide personalised recommendations and to reduce the risk of overfitting historical data.
Das ist alles enthalten
8 Videos1 Aufgabe1 peer review1 Diskussionsthema
In this third module, we will see how to combine two or more basic algorithms, such as collaborative filtering and content-based techniques, into a hybrid recommender system, in order improve the quality recommendations. We will study different hybridization approaches, from the simplest heuristic-based, to the more sophisticated machine learning-based. Thanks to hybrid techniques, we will be able to enrich the input of a collaborative recommender system with either content or contextual information.
Das ist alles enthalten
10 Videos1 Aufgabe1 peer review2 Diskussionsthemen
In this fourth and last module, we will introduce a new advanced technique of collaborative filtering with side information, which is called Factorization Machine (FM), and we’ll see how the input data should be represented when using this technique. With only one mathematical model, based on how you build the input table, we will be able to create a simple matrix factorization algorithm or a sophisticated collaborative filtering algorithm with side information (context, attributes on items or attributes on users). We will also discuss benefits and critical issues of algorithms based on FMs. At the end of the module you will know how to use FMs to mix together different kinds of filtering techniques and how to balance different kinds of input information, playing with coefficients and weights, in order to make better and more sophisticated predictions.
Das ist alles enthalten
7 Videos1 Aufgabe1 peer review1 Diskussionsthema
The RecSys Challenge is the best way to train your competences: it's a practical exercise which provides a "hands-on" opportunity to put to good use and improve what you've been learning during this course (learning by doing). The application domain is an online store, the dataset we provide contains 4 months of transactions collected from an online supermarket. The main goal of the competition is to discover which item a user will interact with. The RecSys Challenge is optional and it is not required to pass the course. If you complete it, you will receive an Honors designation on your Course certificate.
Das ist alles enthalten
1 Lektüre1 Programmieraufgabe
Dozent

Empfohlen, wenn Sie sich für Machine Learning interessieren
University of Minnesota
Indian School of Business
University of Colorado Boulder
University of Leeds
Warum entscheiden sich Menschen für Coursera für ihre Karriere?




Bewertungen von Lernenden
Zeigt 3 von 23
23 Bewertungen
- 5 stars
56,52 %
- 4 stars
13,04 %
- 3 stars
0 %
- 2 stars
13,04 %
- 1 star
17,39 %
Geprüft am 24. Juni 2021

Neue Karrieremöglichkeiten mit Coursera Plus
Unbegrenzter Zugang zu über 7.000 erstklassigen Kursen, praktischen Projekten und Zertifikatsprogrammen, die Sie auf den Beruf vorbereiten – alles in Ihrem Abonnement enthalten
Bringen Sie Ihre Karriere mit einem Online-Abschluss voran.
Erwerben Sie einen Abschluss von erstklassigen Universitäten – 100 % online
Schließen Sie sich mehr als 3.400 Unternehmen in aller Welt an, die sich für Coursera for Business entschieden haben.
Schulen Sie Ihre Mitarbeiter*innen, um sich in der digitalen Wirtschaft zu behaupten.
Häufig gestellte Fragen
Access to lectures and assignments depends on your type of enrollment. If you take a course in audit mode, you will be able to see most course materials for free. To access graded assignments and to earn a Certificate, you will need to purchase the Certificate experience, during or after your audit. If you don't see the audit option:
The course may not offer an audit option. You can try a Free Trial instead, or apply for Financial Aid.
The course may offer 'Full Course, No Certificate' instead. This option lets you see all course materials, submit required assessments, and get a final grade. This also means that you will not be able to purchase a Certificate experience.
When you enroll in the course, you get access to all of the courses in the Specialization, and you earn a certificate when you complete the work. Your electronic Certificate will be added to your Accomplishments page - from there, you can print your Certificate or add it to your LinkedIn profile. If you only want to read and view the course content, you can audit the course for free.
If you subscribed, you get a 7-day free trial during which you can cancel at no penalty. After that, we don’t give refunds, but you can cancel your subscription at any time. See our full refund policy.