An introduction to the field of deep learning, including neural networks, convolutional neural networks, recurrent neural networks, transformers, generative models, neural network compression and transfer learning. This course will benefit students’ careers as a machine learning engineer or data scientist.

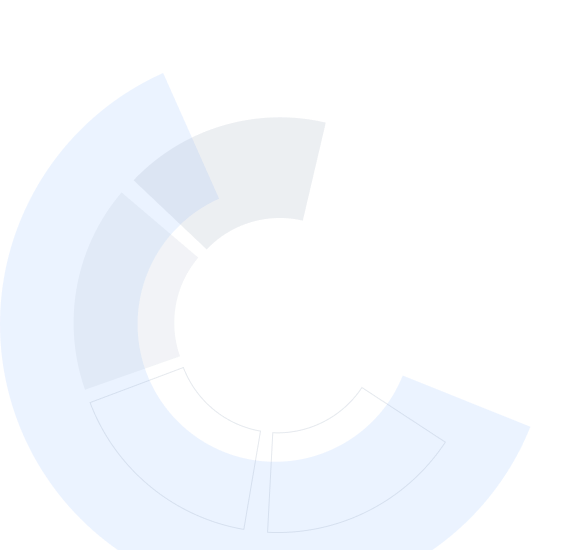
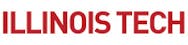
(10 Bewertungen)
Empfohlene Erfahrung
Wichtige Details

Zu Ihrem LinkedIn-Profil hinzufügen
32 Aufgaben
Erfahren Sie, wie Mitarbeiter führender Unternehmen gefragte Kompetenzen erwerben.
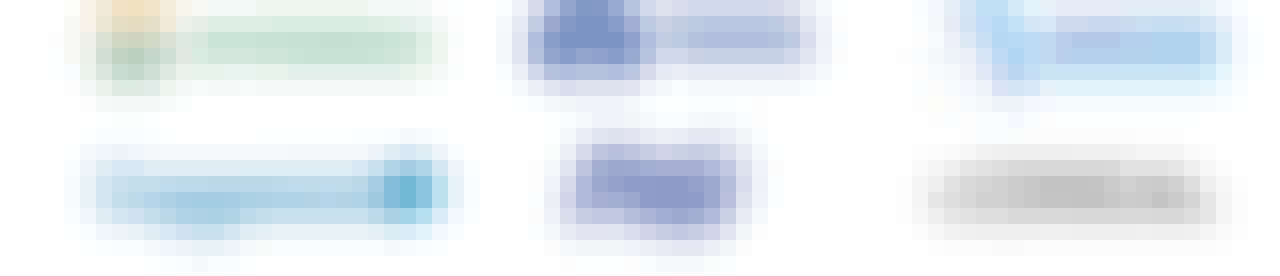
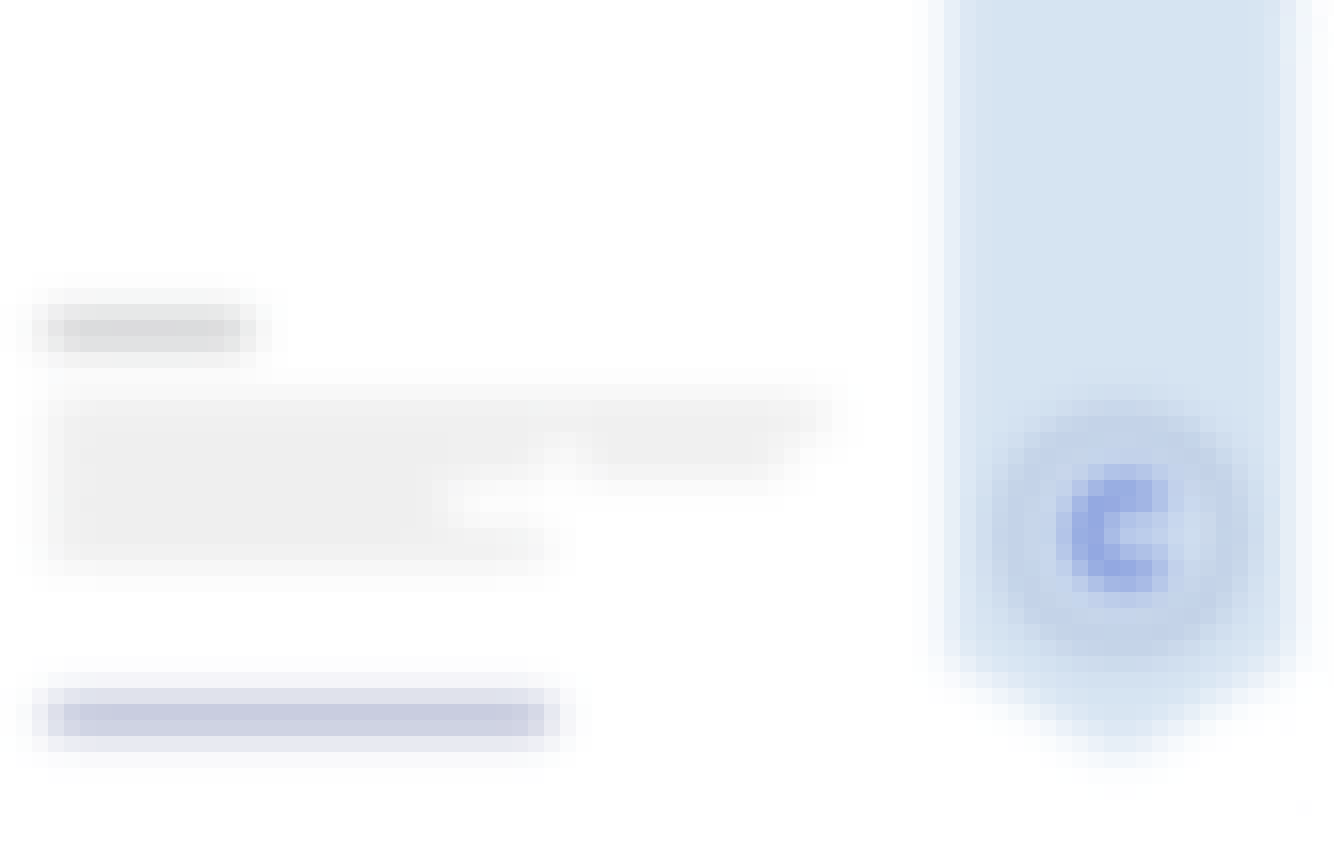
Erwerben Sie ein Karrierezertifikat.
Fügen Sie diese Qualifikation zur Ihrem LinkedIn-Profil oder Ihrem Lebenslauf hinzu.
Teilen Sie es in den sozialen Medien und in Ihrer Leistungsbeurteilung.
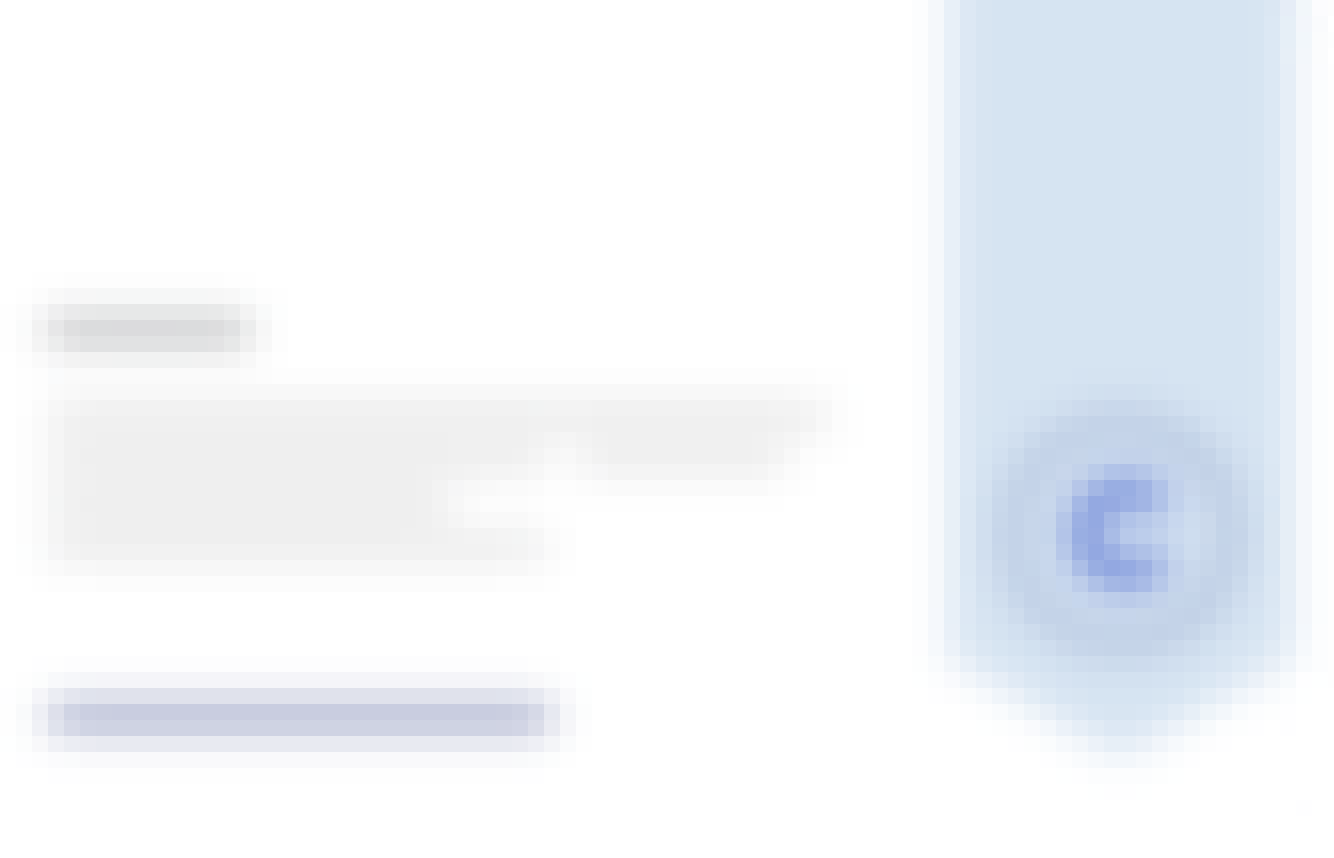
In diesem Kurs gibt es 9 Module
Welcome to Deep Learning! In module 1, we will give an introduction to deep learning. Deep learning is a branch of machine learning which is based on artificial neural networks. It is capable of learning complex patterns and relationships within data. Particularly, we will discuss feed-forward deep neural network. We will also discuss backpropagation – the way to optimize deep neural networks.
Das ist alles enthalten
9 Videos7 Lektüren4 Aufgaben1 Diskussionsthema
In module 2, we will discuss Convolutional Neural Networks (CNNs). A CNN, also known as ConvNet, is a specialized type of deep learning algorithm mainly designed for tasks that necessitate object recognition, including image classification, detection, and segmentation. Particularly, we will discuss the important layers in CNNs, such as convolution, pooling. We will also show different CNN applications.
Das ist alles enthalten
6 Videos5 Lektüren4 Aufgaben
In module 3, we will provide important practical deep learning tips including activation function chosen, adaptive gradient descent learning methods, regularization and dropout.
Das ist alles enthalten
7 Videos7 Lektüren5 Aufgaben
In module 4, we will discuss Recurrent Neural Networks (RNNs) which are used for sequential data. RNN is a type of Neural Network where the output from the previous step is fed as input to the current step. Particularly we will discuss Vanila version RNNs and Long Short-term Memory (LSTM). We will also discuss the learning problems on RNNs.
Das ist alles enthalten
8 Videos5 Lektüren4 Aufgaben
In module 5, we will discuss the generative models. Particularly, Generative Adversarial Networks (GANs) and Diffusion Models (DMs). GANs are a way of training a generative model by framing the problem as a supervised learning problem with two sub-models: the generator model that we train to generate new examples, and the discriminator model that tries to classify examples as either real or fake. DMs are Markov chain of diffusion steps to slowly add random noise to data and then learn to reverse the diffusion process to construct desired data samples from the noise.
Das ist alles enthalten
7 Videos4 Lektüren3 Aufgaben
In module 6, we will discuss a powerful deep learning model - transformer. The transformer is a neural network component that can be used to learn useful representations of sequences or sets of data-points. The transformer has driven recent advances in natural language processing, computer vision, and spatio-temporal modelling.
Das ist alles enthalten
8 Videos4 Lektüren3 Aufgaben
In module 7, we will discuss neural network compression. Model compression reduces the size of a neural network without compromising accuracy. This size reduction is important because bigger neural networks are difficult to deploy on resource-constrained devices.
Das ist alles enthalten
7 Videos5 Lektüren4 Aufgaben
In module 8, we will discuss transfer learning. Transfer learning is a machine learning technique that reuses a completed model that was developed for one task as the starting point for a new model to accomplish a new task. Particularly, we will discuss fine-tuning, multitask learning, domain adverbial training and zero-shot learning.
Das ist alles enthalten
6 Videos5 Lektüren4 Aufgaben
This module contains the summative course assessment that has been designed to evaluate your understanding of the course material and assess your ability to apply the knowledge you have acquired throughout the course.
Das ist alles enthalten
1 Aufgabe
Dozenten


Empfohlen, wenn Sie sich für Algorithms interessieren
Johns Hopkins University
DeepLearning.AI
Auf einen Abschluss hinarbeiten
Dieses Kurs ist Teil des/der folgenden Studiengangs/Studiengänge, die von Illinois Techangeboten werden. Wenn Sie zugelassen werden und sich immatrikulieren, können Ihre abgeschlossenen Kurse auf Ihren Studienabschluss angerechnet werden und Ihre Fortschritte können mit Ihnen übertragen werden.¹
Warum entscheiden sich Menschen für Coursera für ihre Karriere?





Neue Karrieremöglichkeiten mit Coursera Plus
Unbegrenzter Zugang zu 10,000+ Weltklasse-Kursen, praktischen Projekten und berufsqualifizierenden Zertifikatsprogrammen - alles in Ihrem Abonnement enthalten
Bringen Sie Ihre Karriere mit einem Online-Abschluss voran.
Erwerben Sie einen Abschluss von erstklassigen Universitäten – 100 % online
Schließen Sie sich mehr als 3.400 Unternehmen in aller Welt an, die sich für Coursera for Business entschieden haben.
Schulen Sie Ihre Mitarbeiter*innen, um sich in der digitalen Wirtschaft zu behaupten.
Häufig gestellte Fragen
Access to lectures and assignments depends on your type of enrollment. If you take a course in audit mode, you will be able to see most course materials for free. To access graded assignments and to earn a Certificate, you will need to purchase the Certificate experience, during or after your audit. If you don't see the audit option:
The course may not offer an audit option. You can try a Free Trial instead, or apply for Financial Aid.
The course may offer 'Full Course, No Certificate' instead. This option lets you see all course materials, submit required assessments, and get a final grade. This also means that you will not be able to purchase a Certificate experience.
When you purchase a Certificate you get access to all course materials, including graded assignments. Upon completing the course, your electronic Certificate will be added to your Accomplishments page - from there, you can print your Certificate or add it to your LinkedIn profile. If you only want to read and view the course content, you can audit the course for free.
You will be eligible for a full refund until two weeks after your payment date, or (for courses that have just launched) until two weeks after the first session of the course begins, whichever is later. You cannot receive a refund once you’ve earned a Course Certificate, even if you complete the course within the two-week refund period. See our full refund policy.