This course introduces basic time series analysis and forecasting methods. Topics include stationary processes, ARMA models, modeling and forecasting using ARMA models, nonstationary and seasonal time series models, state-space models, and forecasting techniques.

Schenken Sie Ihrer Karriere Coursera Plus mit einem Rabatt von $160 , der jährlich abgerechnet wird. Sparen Sie heute.
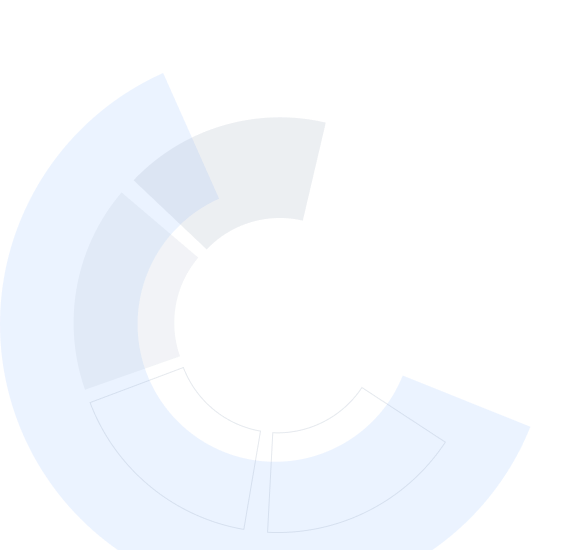
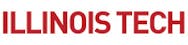
Empfohlene Erfahrung
Kompetenzen, die Sie erwerben
- Kategorie: Statistical Analysis
- Kategorie: Data Analysis in R
- Kategorie: Time Series Analysis And Forecasting
Wichtige Details

Zu Ihrem LinkedIn-Profil hinzufügen
27 Aufgaben
Erfahren Sie, wie Mitarbeiter führender Unternehmen gefragte Kompetenzen erwerben.
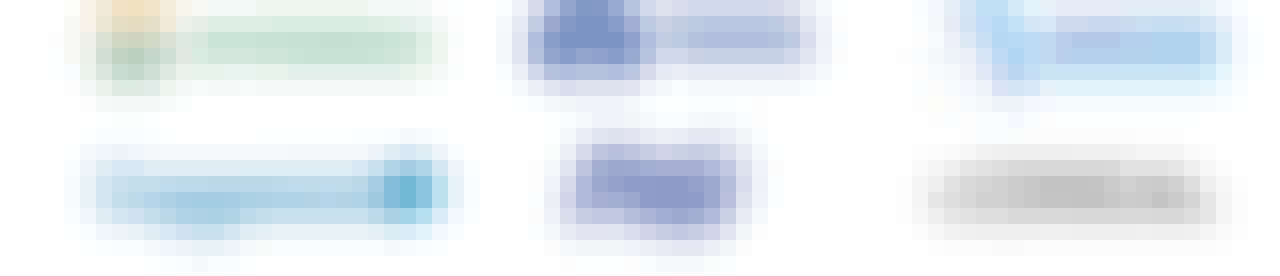
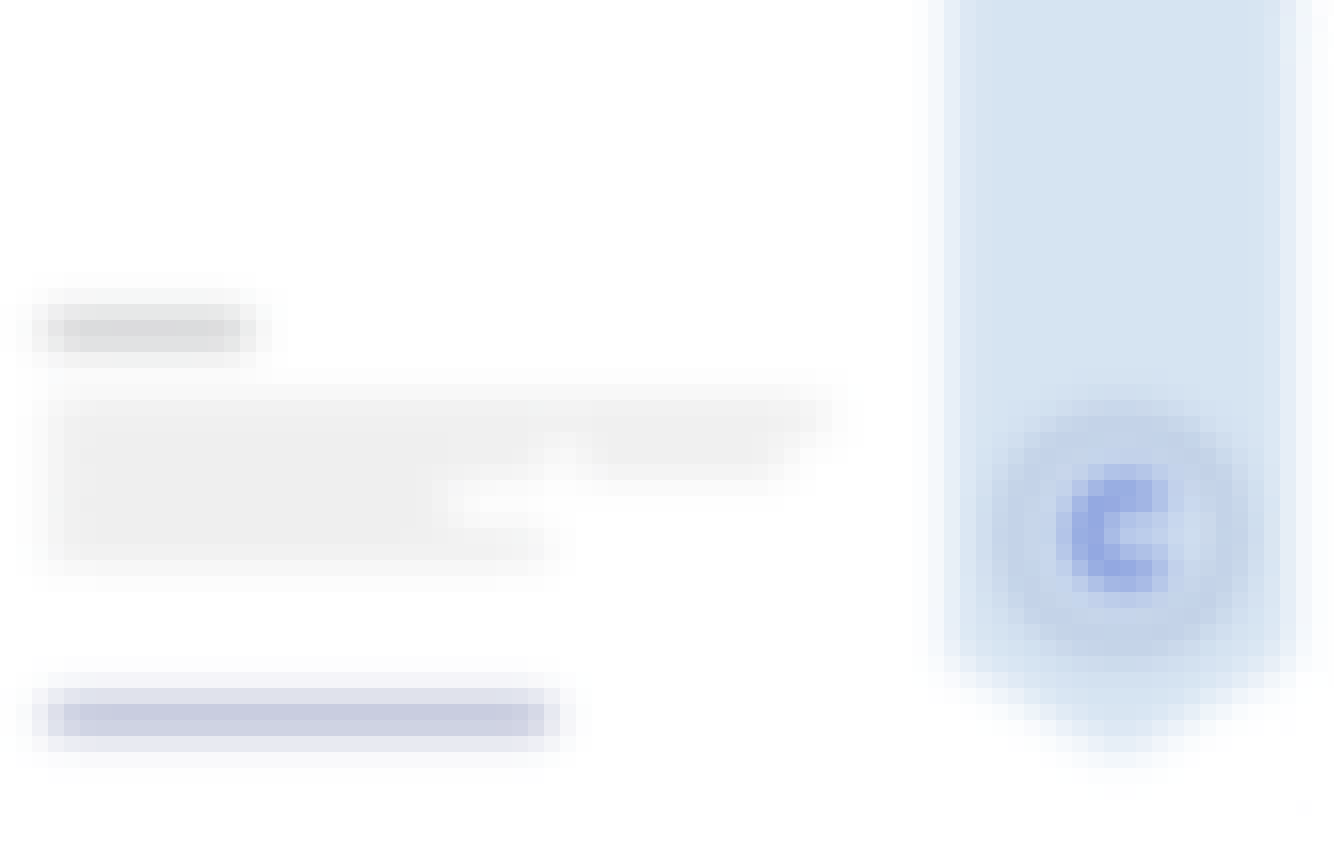
Erwerben Sie ein Karrierezertifikat.
Fügen Sie diese Qualifikation zur Ihrem LinkedIn-Profil oder Ihrem Lebenslauf hinzu.
Teilen Sie es in den sozialen Medien und in Ihrer Leistungsbeurteilung.
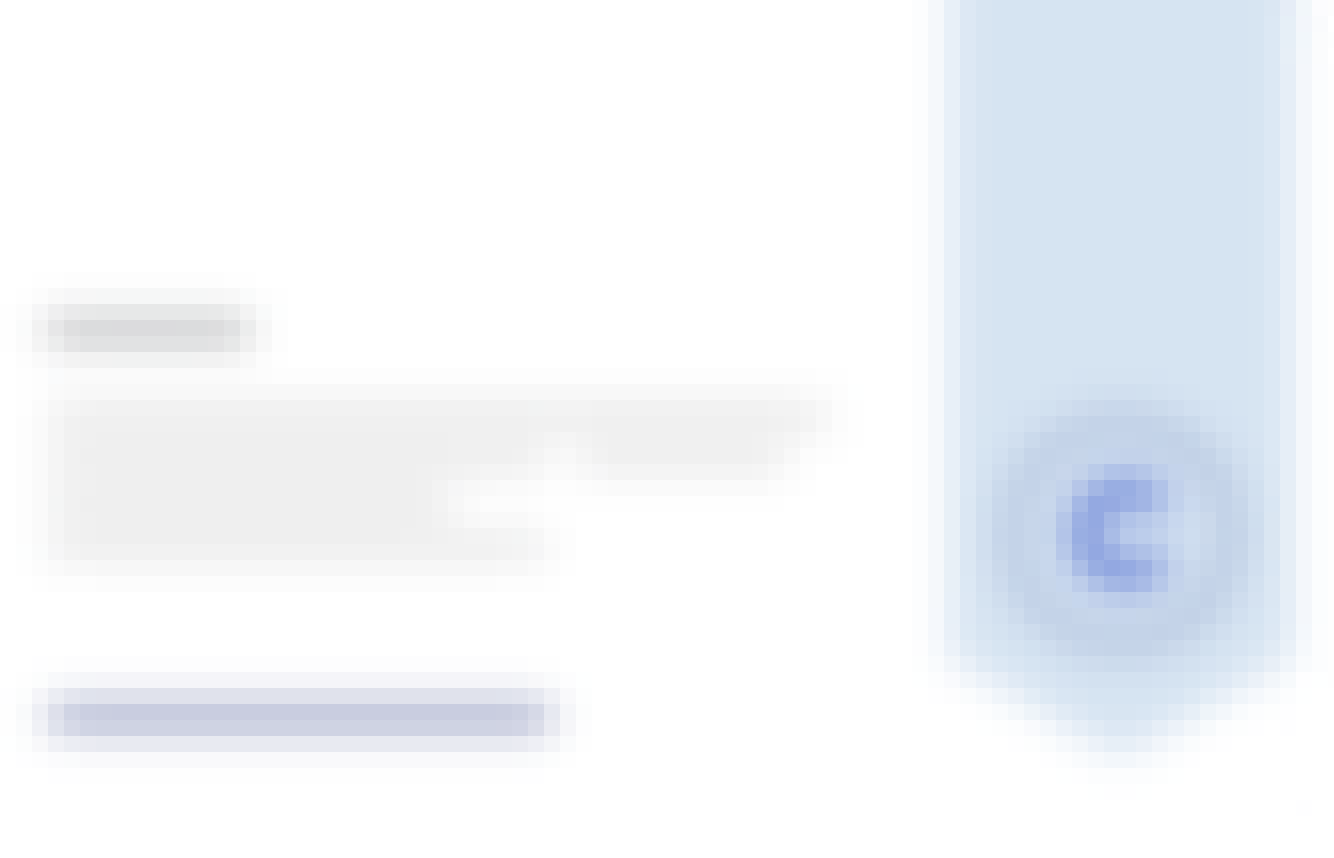
In diesem Kurs gibt es 9 Module
Welcome to Introduction to Time Series! In this module we'll define time series and time series models, and we'll develop some intuition for the fundamental concept of stationarity, and why it's useful.
Das ist alles enthalten
8 Videos6 Lektüren4 Aufgaben1 Diskussionsthema
In this module, we'll discuss stationarity in more detail. We'll learn the technical definitions of weak and strong stationarity, and explain why the weaker version is more practical to use. We'll discuss the autocovariance and autocorrelation functions for stationary processes---concepts that will be with us for the rest of the course. And finally, we'll see some examples of ARMA processes, which we'll treat more deeply in the coming modules.
Das ist alles enthalten
9 Videos3 Lektüren3 Aufgaben
In this module, we'll focus on ARMA processes, and what is arguably their most important feature, namely their autocorrelation structure. We'll see how to compute these "from scratch" (with a little help from R for the computations), and look at plots of the autocorrelation function (ACF) to get some intuition for how the ACF of an ARMA process behaves and what it can tell us.
Das ist alles enthalten
10 Videos4 Lektüren3 Aufgaben
In this module, we begin by discussing the ACF's of more complicated ARMA processes. Our main focus, though, is on one-step-ahead forecasts. We learn about the best linear predictor: both how it is defined and how to use it. Finally, we use what we have learned in order to define the Partial Autocorrelation Function (PACF), which is another fundamental tool in the study of stationary processes.
Das ist alles enthalten
9 Videos3 Lektüren3 Aufgaben
In this module, we learn about fitting a stationary time series model to data. The fitting process involves determining what values of the parameters to use. We discuss preliminary estimation and maximum likelihood estimation of these parameters.
Das ist alles enthalten
9 Videos4 Lektüren4 Aufgaben
In this module, we discuss model diagnostics and order selection. Given an ARMA order, we've already seen how to best fit the parameters of the associated model. Given several different fitted models, the tools we develop in this module will allow us to make an intelligent choice about which one to use.
Das ist alles enthalten
7 Videos3 Lektüren3 Aufgaben
This module introduces students to ARIMA and SARIMA modeling techniques, essential for analyzing non-stationary and seasonal time series data. In the first lesson, students will learn to define ARIMA processes, use the Dickey-Fuller test to determine the need for differencing, and fit ARIMA models using R. The second lesson extends these skills to SARIMA models, focusing on identifying seasonality and fitting these models to capture seasonal patterns in data.
Das ist alles enthalten
9 Videos3 Lektüren3 Aufgaben
This module equips students with more sophisticated forecasting techniques beyond one-step-ahead predictions. We treat both (S)ARIMA models and exponential smoothing models and show how to handle forecasts in R. For the simplest of these models, we look inside the "black box" a little bit and demonstrate how these forecasts are generated.
Das ist alles enthalten
9 Videos3 Lektüren3 Aufgaben
This module contains the summative course assessment that has been designed to evaluate your understanding of the course material and assess your ability to apply the knowledge you have acquired throughout the course.
Das ist alles enthalten
1 Aufgabe
Dozent

Empfohlen, wenn Sie sich für Data Analysis interessieren
Politecnico di Milano
Duke University
University of London
Queen Mary University of London
Auf einen Abschluss hinarbeiten
Dieses Kurs ist Teil des/der folgenden Studiengangs/Studiengänge, die von Illinois Techangeboten werden. Wenn Sie zugelassen werden und sich immatrikulieren, können Ihre abgeschlossenen Kurse auf Ihren Studienabschluss angerechnet werden und Ihre Fortschritte können mit Ihnen übertragen werden.¹
Warum entscheiden sich Menschen für Coursera für ihre Karriere?





Neue Karrieremöglichkeiten mit Coursera Plus
Unbegrenzter Zugang zu über 7.000 erstklassigen Kursen, praktischen Projekten und Zertifikatsprogrammen, die Sie auf den Beruf vorbereiten – alles in Ihrem Abonnement enthalten
Bringen Sie Ihre Karriere mit einem Online-Abschluss voran.
Erwerben Sie einen Abschluss von erstklassigen Universitäten – 100 % online
Schließen Sie sich mehr als 3.400 Unternehmen in aller Welt an, die sich für Coursera for Business entschieden haben.
Schulen Sie Ihre Mitarbeiter*innen, um sich in der digitalen Wirtschaft zu behaupten.
Häufig gestellte Fragen
Access to lectures and assignments depends on your type of enrollment. If you take a course in audit mode, you will be able to see most course materials for free. To access graded assignments and to earn a Certificate, you will need to purchase the Certificate experience, during or after your audit. If you don't see the audit option:
The course may not offer an audit option. You can try a Free Trial instead, or apply for Financial Aid.
The course may offer 'Full Course, No Certificate' instead. This option lets you see all course materials, submit required assessments, and get a final grade. This also means that you will not be able to purchase a Certificate experience.
When you purchase a Certificate you get access to all course materials, including graded assignments. Upon completing the course, your electronic Certificate will be added to your Accomplishments page - from there, you can print your Certificate or add it to your LinkedIn profile. If you only want to read and view the course content, you can audit the course for free.
You will be eligible for a full refund until two weeks after your payment date, or (for courses that have just launched) until two weeks after the first session of the course begins, whichever is later. You cannot receive a refund once you’ve earned a Course Certificate, even if you complete the course within the two-week refund period. See our full refund policy.