This course, Machine Learning for Accounting with Python, introduces machine learning algorithms (models) and their applications in accounting problems. It covers classification, regression, clustering, text analysis, time series analysis. It also discusses model evaluation and model optimization. This course provides an entry point for students to be able to apply proper machine learning models on business related datasets with Python to solve various problems.

Schenken Sie Ihrer Karriere Coursera Plus mit einem Rabatt von $160 , der jährlich abgerechnet wird. Sparen Sie heute.
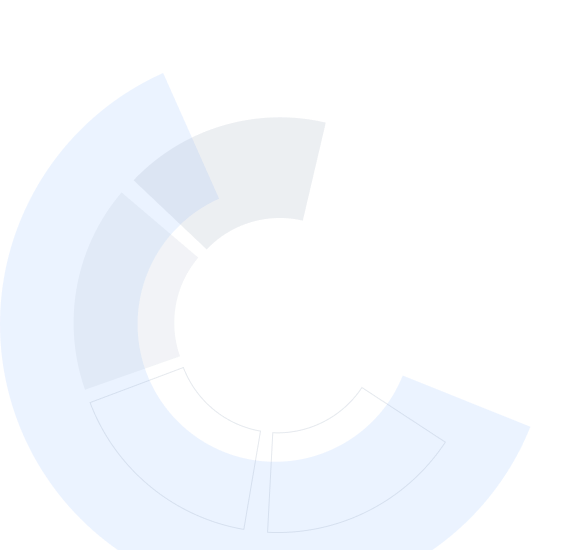
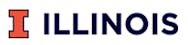
Machine Learning for Accounting with Python
Dieser Kurs ist Teil von Spezialisierung Accounting Data Analytics

Dozent: Linden Lu
9.365 bereits angemeldet
Bei enthalten
(43 Bewertungen)
Was Sie lernen werden
The concept of various machine learning algorithms.
How to apply machine learning models on datasets with Python in Jupyter Notebook.
How to evaluate machine learning models.
How to optimize machine learning models.
Kompetenzen, die Sie erwerben
- Kategorie: Python Programming
- Kategorie: Machine Learning Model Evaluation and Optimization
- Kategorie: Basic Time Series Analysis
- Kategorie: Machine Learning Modeling
- Kategorie: Text Analysis
Wichtige Details

Zu Ihrem LinkedIn-Profil hinzufügen
8 Quizzes
Erfahren Sie, wie Mitarbeiter führender Unternehmen gefragte Kompetenzen erwerben.
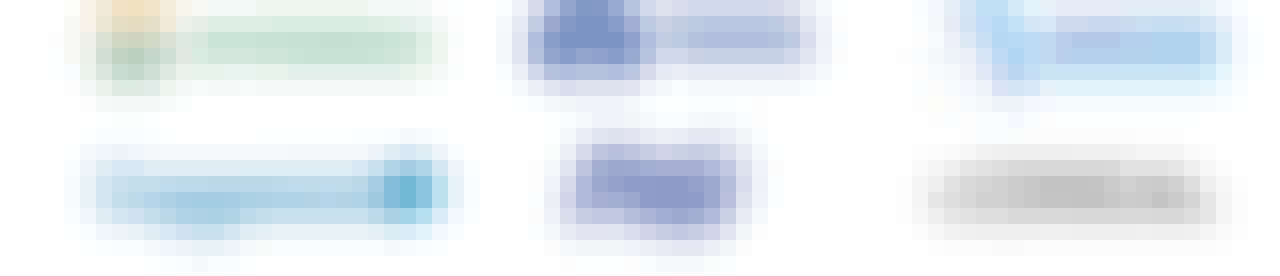
Erweitern Sie Ihre Fachkenntnisse
- Lernen Sie neue Konzepte von Branchenexperten
- Gewinnen Sie ein Grundverständnis bestimmter Themen oder Tools
- Erwerben Sie berufsrelevante Kompetenzen durch praktische Projekte
- Erwerben Sie ein Berufszertifikat zur Vorlage
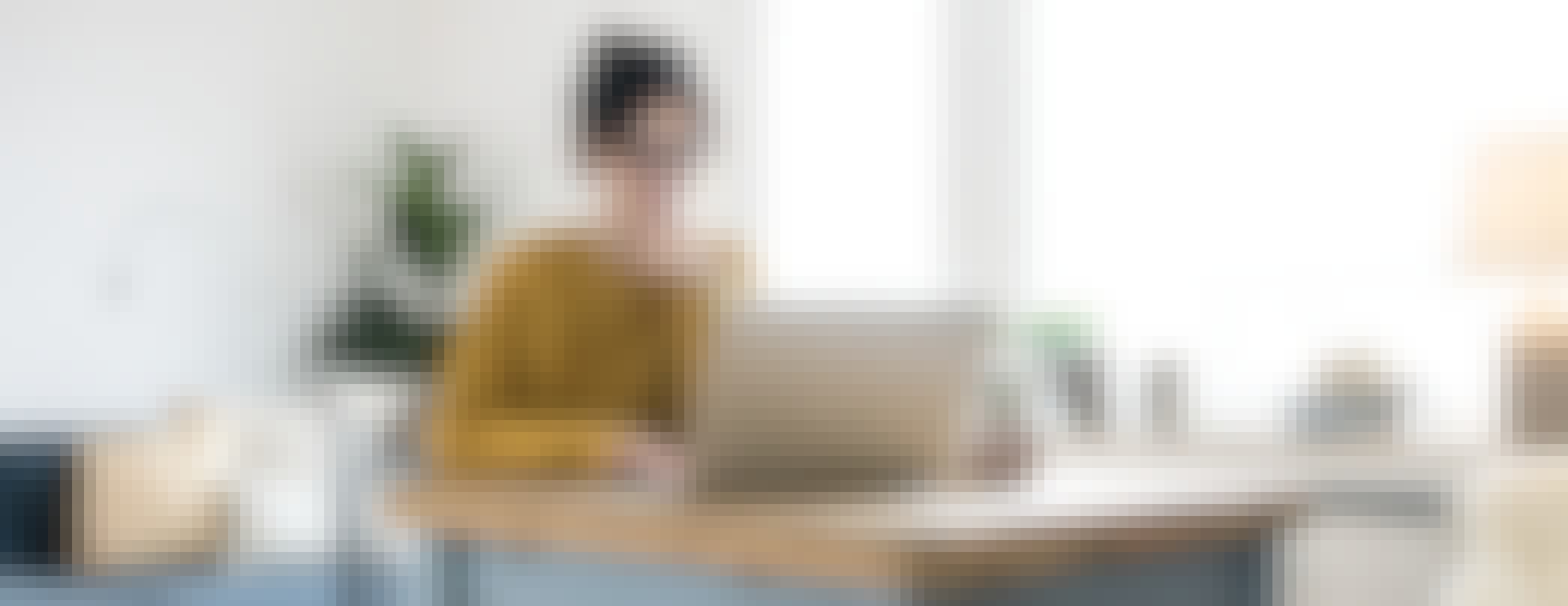
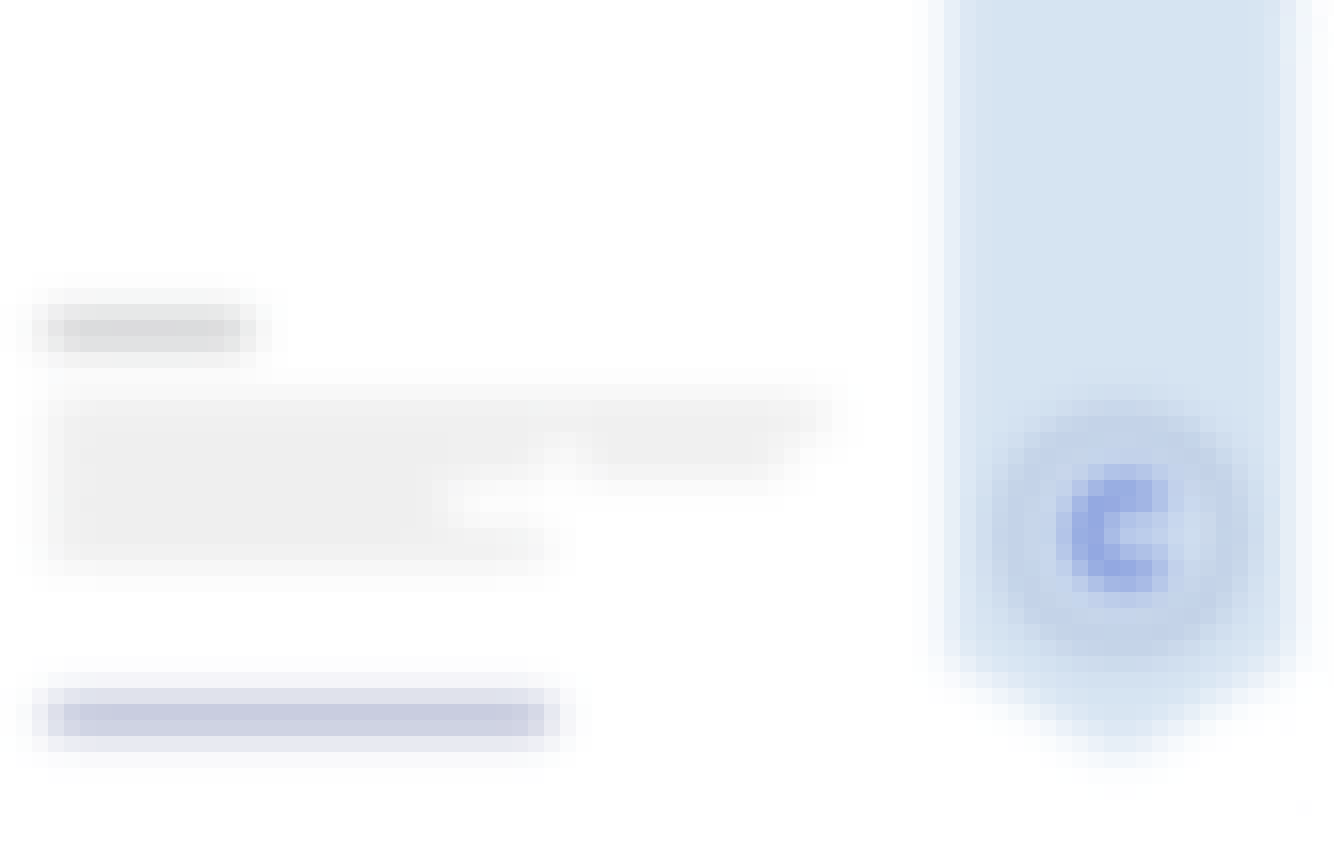
Erwerben Sie ein Karrierezertifikat.
Fügen Sie diese Qualifikation zur Ihrem LinkedIn-Profil oder Ihrem Lebenslauf hinzu.
Teilen Sie es in den sozialen Medien und in Ihrer Leistungsbeurteilung.
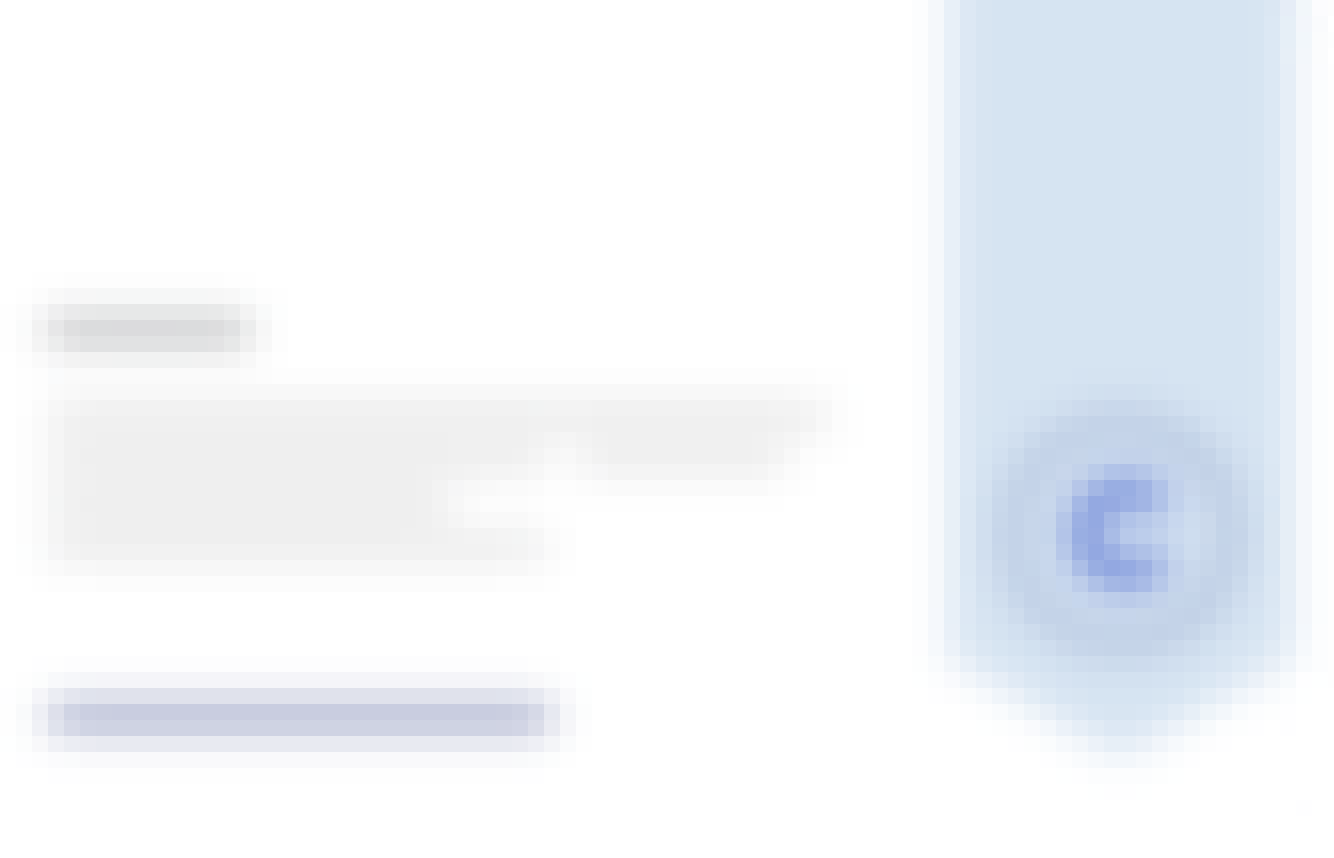
In diesem Kurs gibt es 9 Module
In this module, you will become familiar with the course, your instructor and your classmates, and our learning environment. This orientation will also help you obtain the technical skills required to navigate and be successful in this course.
Das ist alles enthalten
2 Videos4 Lektüren2 Diskussionsthemen1 Plug-in
This module provides the basis for the rest of the course by introducing the basic concepts behind machine learning, and, specifically, how to perform machine learning by using Python and the scikit-learn machine learning module. First, you will learn about the basic types of machine learning. Next, you will learn an important step before applying machine learning algorithms, data pre-processing. Finally, you will learn how to leverage different types of machine learning algorithms in a Python script.
Das ist alles enthalten
4 Videos1 Lektüre1 Quiz1 Programmieraufgabe1 Diskussionsthema4 Unbewertete Labore
This module introduces three machine learning algorithms. First, you will learn how linear regression can be considered a machine learning problem with parameters that must be determined computationally by minimizing a cost function. Next, you will learn Logistic Regression. Despite its name, Logistic Regression is a classification algorithm. Lastly, you will learn Decision Tree, which is a popular machine learning algorithm that can be used for both classification and regression. This module will dive deeper into the concept of machine classification, where algorithms learn from existing, labeled data to classify new, unseen data into specific categories; and, the concept of machine regression, where algorithms learn a model from data to make predictions for new, unseen continuous data. While these algorithms all differ in their mathematical underpinnings, they are often used for classifying numerical, text, and image data or performing regression in a variety of domains.
Das ist alles enthalten
4 Videos1 Lektüre1 Quiz1 Programmieraufgabe4 Unbewertete Labore
This module introduces three more machine learning algorithms, k-nearest neighbors, support vector machine and random forest. All of them can be used for either classification or regression tasks.
Das ist alles enthalten
4 Videos1 Lektüre1 Quiz1 Programmieraufgabe4 Unbewertete Labore
Model Evaluation is an integral component of any data analytics project. It helps to find out how well the model will work on predicting future (out-of-sample) data. This module introduces basic model evaluation metrics for machine learning algorithms. First, the evaluation metrics for regression is presented. Next the metrics and techniques to evaluate classification are introduced.
Das ist alles enthalten
4 Videos1 Lektüre1 Quiz1 Programmieraufgabe4 Unbewertete Labore
This module introduces the techniques of model optimization. First, the basic techniques of feature selection is presented. Next, the technique of cross-validation is introduced, which can provide a more accurate evaluation on models. Finally, model selection, or hyperparameter tuning, which uses cross-validation, is introduced.
Das ist alles enthalten
4 Videos1 Lektüre1 Quiz1 Programmieraufgabe4 Unbewertete Labore
In this module, you will start applying your new machine learning skills to an exciting data analytic topic: Text Analysis. First, we will review the process by which textual data is converted into numerical data that can be processed by a computer. Along with this are a number of new concepts that focus on manipulating these data to generate improved machine learning predictions. Second, we will apply machine learning algorithms, specifically classification, to text data. Finally, we will explore the more advanced concepts in text analysis and introduce a special kind of text classification: sentiment analysis.
Das ist alles enthalten
4 Videos1 Lektüre1 Quiz1 Programmieraufgabe4 Unbewertete Labore
This module introduces clustering, where data points are assigned to sub groups of points based on some specific properties, such as spatial distance or the local density of points. While humans often find clusters visually with ease in a given data sets, computationally the problem is more challenging. This module starts by exploring the basic ideas behind this unsupervised learning technique. One of the most popular clustering techniques, K-means, is introduced. Next, a K-means case study is provided. Finally the density-based DBSCAN technique is introduced.
Das ist alles enthalten
4 Videos1 Lektüre1 Quiz1 Programmieraufgabe4 Unbewertete Labore
This module introduces time and date data, which provide unique learning opportunities and challenges. First, we will discuss how to properly handle time and date features within a Python program. Next, we will extend this discussion to handle data indexed by time and date information, which is known as time series data.
Das ist alles enthalten
4 Videos3 Lektüren1 Quiz1 Programmieraufgabe3 Unbewertete Labore1 Plug-in
Dozent

Empfohlen, wenn Sie sich für Business Strategy interessieren
University of Illinois Urbana-Champaign
University of Illinois Urbana-Champaign
The Hong Kong University of Science and Technology
Auf einen Abschluss hinarbeiten
Dieses Kurs ist Teil des/der folgenden Studiengangs/Studiengänge, die von University of Illinois Urbana-Champaignangeboten werden. Wenn Sie zugelassen werden und sich immatrikulieren, können Ihre abgeschlossenen Kurse auf Ihren Studienabschluss angerechnet werden und Ihre Fortschritte können mit Ihnen übertragen werden.¹
Warum entscheiden sich Menschen für Coursera für ihre Karriere?




Bewertungen von Lernenden
Zeigt 3 von 43
43 Bewertungen
- 5 stars
69,76 %
- 4 stars
23,25 %
- 3 stars
4,65 %
- 2 stars
0 %
- 1 star
2,32 %
Geprüft am 31. Jan. 2022
Geprüft am 16. Apr. 2022
Geprüft am 26. Aug. 2022

Neue Karrieremöglichkeiten mit Coursera Plus
Unbegrenzter Zugang zu über 7.000 erstklassigen Kursen, praktischen Projekten und Zertifikatsprogrammen, die Sie auf den Beruf vorbereiten – alles in Ihrem Abonnement enthalten
Bringen Sie Ihre Karriere mit einem Online-Abschluss voran.
Erwerben Sie einen Abschluss von erstklassigen Universitäten – 100 % online
Schließen Sie sich mehr als 3.400 Unternehmen in aller Welt an, die sich für Coursera for Business entschieden haben.
Schulen Sie Ihre Mitarbeiter*innen, um sich in der digitalen Wirtschaft zu behaupten.
Häufig gestellte Fragen
Access to lectures and assignments depends on your type of enrollment. If you take a course in audit mode, you will be able to see most course materials for free. To access graded assignments and to earn a Certificate, you will need to purchase the Certificate experience, during or after your audit. If you don't see the audit option:
The course may not offer an audit option. You can try a Free Trial instead, or apply for Financial Aid.
The course may offer 'Full Course, No Certificate' instead. This option lets you see all course materials, submit required assessments, and get a final grade. This also means that you will not be able to purchase a Certificate experience.
When you enroll in the course, you get access to all of the courses in the Specialization, and you earn a certificate when you complete the work. Your electronic Certificate will be added to your Accomplishments page - from there, you can print your Certificate or add it to your LinkedIn profile. If you only want to read and view the course content, you can audit the course for free.
If you subscribed, you get a 7-day free trial during which you can cancel at no penalty. After that, we don’t give refunds, but you can cancel your subscription at any time. See our full refund policy.