Dieser Kurs stellt eine leistungsstarke Reihe von Data Science Tools vor, die als Tidyverse bekannt sind. Tidyverse hat die Art und Weise revolutioniert, in der Datenwissenschaftler fast jeden Aspekt ihrer Arbeit erledigen. Wir werden die einfache Idee der "aufgeräumten Daten" behandeln und wie diese Idee dazu dient, Daten für die Analyse und Modellierung zu organisieren. Außerdem werden wir uns damit befassen, wie nicht aufgeräumte Daten in aufgeräumte Daten umgewandelt werden können, mit dem Lebenszyklus von Data Science-Projekten und dem Ökosystem der Tidyverse R-Pakete, die zur Durchführung eines Data Science-Projekts verwendet werden können.

Pflegen Sie Ihre Karriere mit von Experten geleiteten Programmen, arbeitsplatztauglichen Zertifikaten und 10.000 Möglichkeiten zur Weiterentwicklung. Alles für 25 $/Monat, jährlich abgerechnet. Jetzt sparen
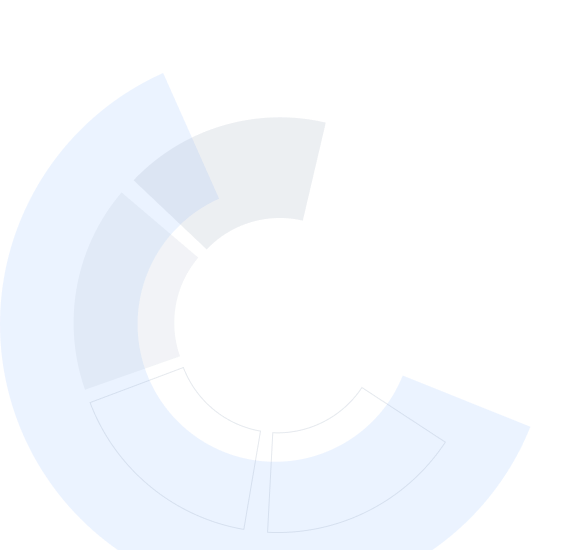
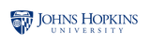
Einführung in das Tidyverse
Dieser Kurs ist Teil von Spezialisierung Tidyverse-Fähigkeiten für Datenwissenschaft in R



Dozenten: Carrie Wright, PhD
4.781 bereits angemeldet
Bei enthalten
(48 Bewertungen)
Empfohlene Erfahrung
Was Sie lernen werden
Unterscheiden Sie zwischen aufgeräumten und unaufgeräumten Daten
Beschreiben Sie, wie nicht aufgeräumte Daten in aufgeräumte Daten umgewandelt werden können
Beschreiben Sie das Tidyverse Ökosystem von Paketen
Organisieren und initiieren Sie ein Data Science-Projekt
Kompetenzen, die Sie erwerben
- Kategorie: Datenanalyse
- Kategorie: Führung und Management
- Kategorie: Grundsätze der Programmierung
- Kategorie: Datenbanken
Wichtige Details

Zu Ihrem LinkedIn-Profil hinzufügen
5 Aufgaben
Erfahren Sie, wie Mitarbeiter führender Unternehmen gefragte Kompetenzen erwerben.
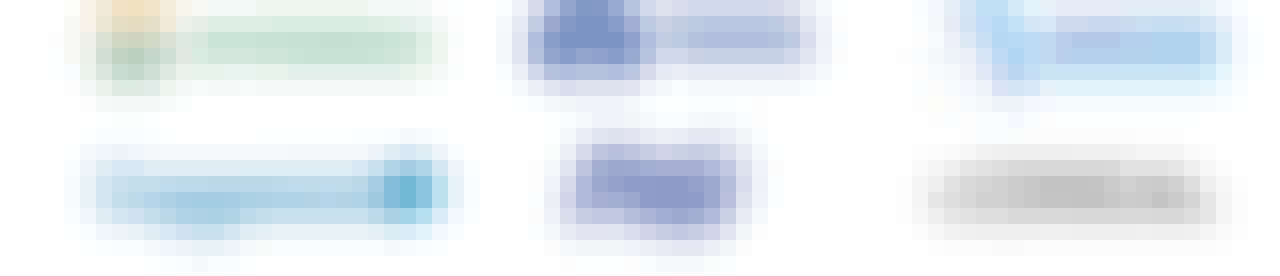
Erweitern Sie Ihre Fachkenntnisse
- Lernen Sie neue Konzepte von Branchenexperten
- Gewinnen Sie ein Grundverständnis bestimmter Themen oder Tools
- Erwerben Sie berufsrelevante Kompetenzen durch praktische Projekte
- Erwerben Sie ein Berufszertifikat zur Vorlage
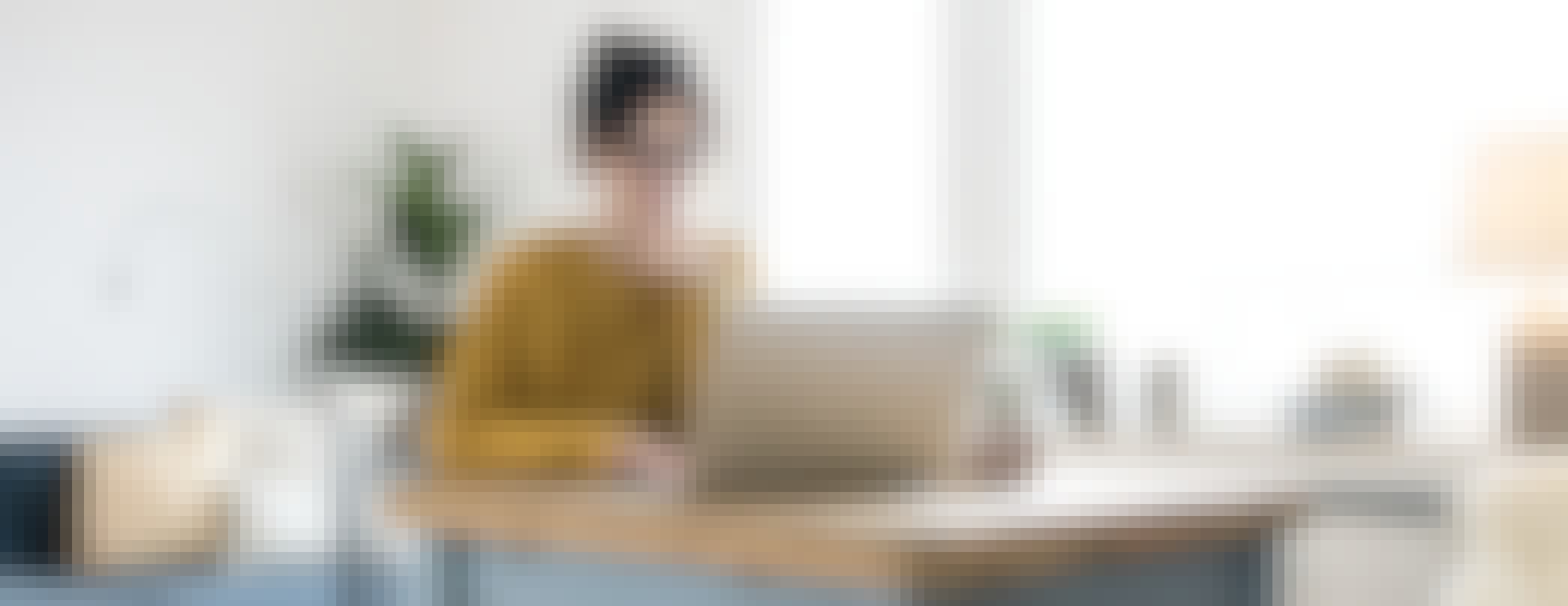
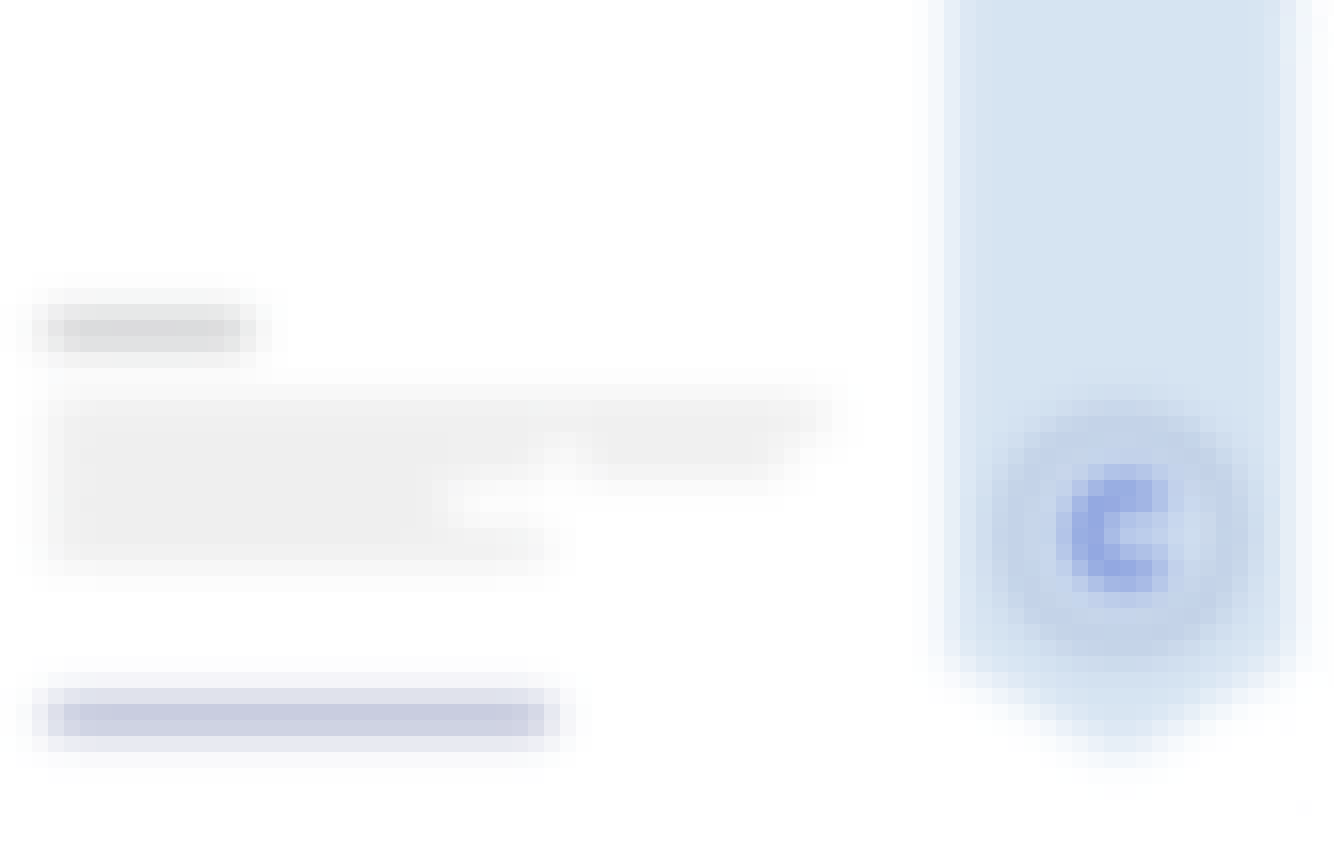
Erwerben Sie ein Karrierezertifikat.
Fügen Sie diese Qualifikation zur Ihrem LinkedIn-Profil oder Ihrem Lebenslauf hinzu.
Teilen Sie es in den sozialen Medien und in Ihrer Leistungsbeurteilung.
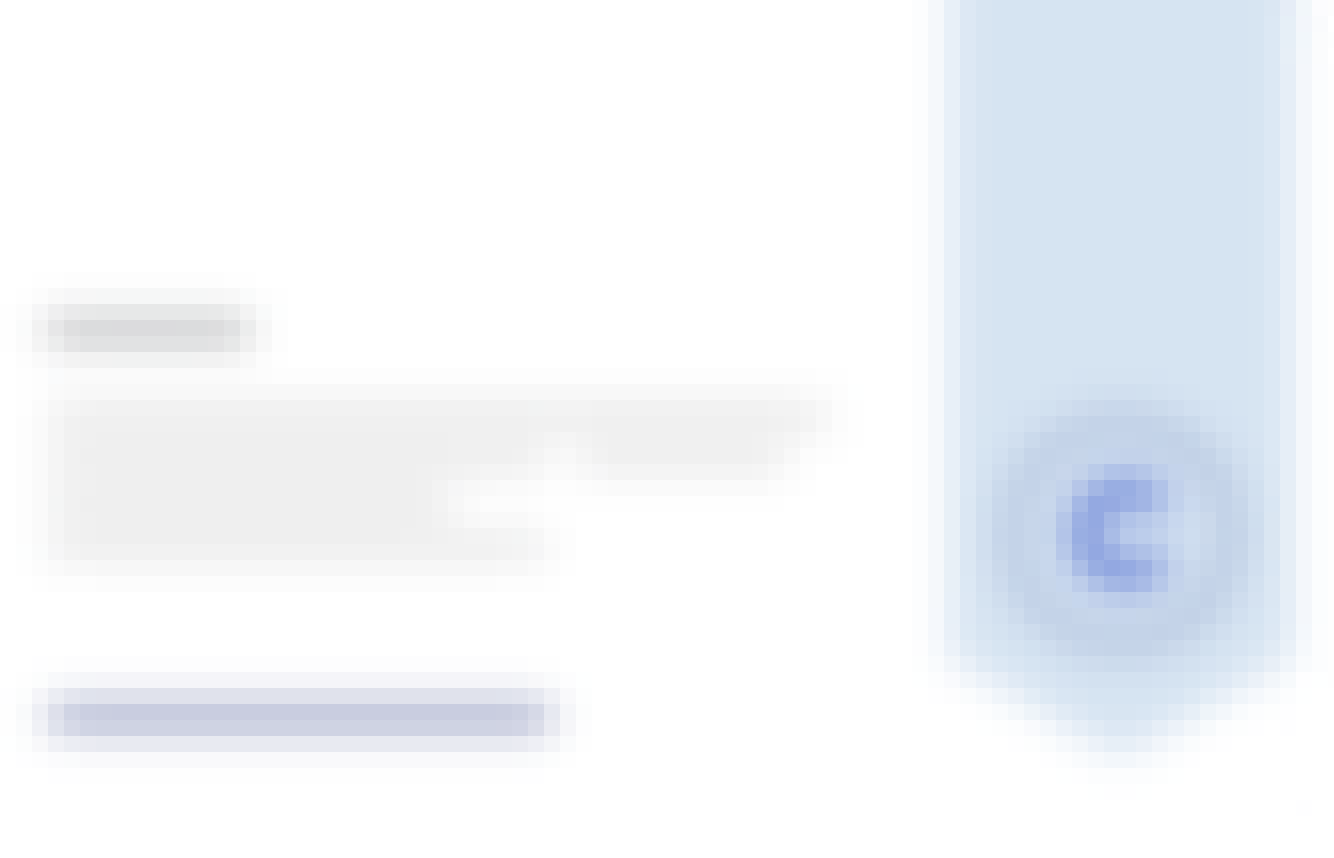
In diesem Kurs gibt es 6 Module
Bevor wir all die Möglichkeiten erörtern können, mit denen R die Arbeit mit aufgeräumten Daten erleichtert, müssen wir zunächst wissen, was aufgeräumte Daten sind. Aufgeräumte Datensätze sind einfacher zu bearbeiten, zu modellieren und zu visualisieren, da die Grundsätze für aufgeräumte Daten, die wir in diesem Kurs besprechen werden, den Daten einen allgemeinen Rahmen und eine Reihe von konsistenten Regeln auferlegen. Ein bekanntes Zitat von Hadley Wickham lautet: "Aufgeräumte Datensätze sind alle gleich, aber jeder unordentliche Datensatz ist auf seine eigene Weise unordentlich." Die Verwendung eines konsistenten, aufgeräumten Datenformats ermöglicht die Entwicklung von Tools, die innerhalb dieses Rahmens gut funktionieren und letztlich die Datenverarbeitung, Visualisierung und Analyse vereinfachen. Wenn Sie mit Daten beginnen, die bereits in einem aufgeräumten Format vorliegen, oder wenn Sie sich zu Beginn eines Projekts die Zeit nehmen, die Daten in ein aufgeräumtes Format zu bringen, werden die verbleibenden Schritte Ihres Data Science-Projekts einfacher sein.
Das ist alles enthalten
6 Lektüren2 Aufgaben
Der Grund, warum es wichtig ist, darüber zu sprechen, was ordentliche Daten sind und wie sie aussehen, ist, dass die meisten Daten in der Welt da draußen unordentlich sind. Wenn Sie nicht derjenige sind, der die Daten eingibt, sondern wenn Sie die Daten von jemand anderem für ein Projekt erhalten, sind diese Daten in den meisten Fällen unordentlich. Unordentliche Daten werden oft einfach als unordentliche Daten bezeichnet. Um mit diesen Daten problemlos arbeiten zu können, müssen Sie sie in ein ordentliches Datenformat bringen. Das bedeutet, dass Sie unordentliche Daten genau erkennen und wissen müssen, wie Sie sie in ein ordentliches Format bringen können. Die folgenden häufigen Probleme, die in unordentlichen Datensätzen auftreten, stammen wiederum aus Hadley Wickhams Aufsatz über ordentliche Daten (http://vita.had.co.nz/papers/tidy-data.pdf). Nach einer kurzen Erläuterung der einzelnen Probleme werden wir uns einige unordentliche Datensätze ansehen. Schließlich werden wir uns mit den Konzepten für die Bereinigung unordentlicher Daten befassen, aber wir werden noch keine praktischen Übungen machen. Das kommt bald!
Das ist alles enthalten
3 Lektüren1 Aufgabe
Mit einem soliden Verständnis von aufgeräumten Daten und wie aufgeräumte Daten in den Lebenszyklus der Datenwissenschaft passen, werden wir uns ein wenig Zeit nehmen, um Ihnen die tidyverse und tidyverse-verwandten Pakete vorzustellen, die wir in dieser Spezialisierung lehren und verwenden werden. Zusammen bilden diese Pakete das tidyverse-Ökosystem, wie wir es nennen. Im weiteren Verlauf dieses Kurses geht es nicht darum, dass Sie verstehen, wie jedes dieser Pakete zu verwenden ist (das kommt noch!), sondern vielmehr darum, dass Sie sich damit vertraut machen, welche Pakete in welchen Teil des Lebenszyklus der Datenwissenschaft passen. Beachten Sie, dass die offiziellen tidyverse-Pakete unten fett gedruckt sind. Alle anderen Pakete sind tidyverse-adjacent, d.h. sie folgen denselben Konventionen wie die offiziellen tidyverse-Pakete und funktionieren gut innerhalb des tidy-Rahmens und der Struktur der Datenanalyse.
Das ist alles enthalten
5 Lektüren
Data-Science-Projekte sind sehr unterschiedlich, so dass es schwierig sein kann, allgemeingültige Regeln für ihre Organisation aufzustellen. Es gibt jedoch einige Möglichkeiten, Projekte zu organisieren, die im Allgemeinen nützlich sind. Insbesondere haben fast alle Projekte mit verschiedenen Arten von Dateien zu tun - Datendateien, Codedateien, Ausgabedateien usw. In diesem Abschnitt erfahren Sie, wie Dateien funktionieren und wie Projekte organisiert und angepasst werden können.
Das ist alles enthalten
6 Lektüren2 Aufgaben
In dieser Specialization werden wir eine Reihe von Fallstudien aus Open Case Studies verwenden, um die im Kurs vorgestellten Konzepte zu demonstrieren. Im Allgemeinen werden wir während der gesamten Spezialisierung dieselben Fallstudien verwenden, um Kontinuität zu gewährleisten, damit Sie sich auf die Konzepte und Fähigkeiten konzentrieren können, die gelehrt werden (und nicht auf den Kontext), während Sie mit interessanten Daten arbeiten. Diese Fallstudien befassen sich mit einer Frage des öffentlichen Gesundheitswesens und verwenden allesamt echte Daten.
Das ist alles enthalten
2 Lektüren2 Unbewertete Labore
Dieses Projekt ermöglicht es Ihnen, ein neues Projekt zu erstellen und die Dateien zu organisieren, die für eine zukünftige Datenanalyse benötigt werden
Das ist alles enthalten
1 peer review
Dozenten



Empfohlen, wenn Sie sich für Datenanalyse interessieren
Johns Hopkins University
Johns Hopkins University
University of Colorado Boulder
University of Colorado Boulder
Warum entscheiden sich Menschen für Coursera für ihre Karriere?




Bewertungen von Lernenden
48 Bewertungen
- 5 stars
70,83 %
- 4 stars
14,58 %
- 3 stars
4,16 %
- 2 stars
2,08 %
- 1 star
8,33 %
Zeigt 3 von 48 an
Geprüft am 17. Apr. 2024
The course is a breeze to follow because it aligns seamlessly with the book. As such, rather than watching videos, you get to read the book; it's really a convenient approach.
Bravo!
Geprüft am 30. Okt. 2022
Covers really important concepts and procedures for managing data science projects. Very helpful.

Neue Karrieremöglichkeiten mit Coursera Plus
Unbegrenzter Zugang zu 10,000+ Weltklasse-Kursen, praktischen Projekten und berufsqualifizierenden Zertifikatsprogrammen - alles in Ihrem Abonnement enthalten
Bringen Sie Ihre Karriere mit einem Online-Abschluss voran.
Erwerben Sie einen Abschluss von erstklassigen Universitäten – 100 % online
Schließen Sie sich mehr als 3.400 Unternehmen in aller Welt an, die sich für Coursera for Business entschieden haben.
Schulen Sie Ihre Mitarbeiter*innen, um sich in der digitalen Wirtschaft zu behaupten.
Häufig gestellte Fragen
Der Zugang zu Vorlesungen und Aufgaben hängt von der Art Ihrer Einschreibung ab. Wenn Sie einen Kurs im Prüfungsmodus belegen, können Sie die meisten Kursmaterialien kostenlos einsehen. Um auf benotete Aufgaben zuzugreifen und ein Zertifikat zu erwerben, müssen Sie die Zertifikatserfahrung während oder nach Ihrer Prüfung erwerben. Wenn Sie die Prüfungsoption nicht sehen:
Der Kurs bietet möglicherweise keine Prüfungsoption. Sie können stattdessen eine kostenlose Testversion ausprobieren oder finanzielle Unterstützung beantragen.
Der Kurs bietet möglicherweise stattdessen die Option 'Vollständiger Kurs, kein Zertifikat'. Mit dieser Option können Sie alle Kursmaterialien einsehen, die erforderlichen Bewertungen abgeben und eine Abschlussnote erhalten. Dies bedeutet auch, dass Sie kein Zertifikat erwerben können.
Wenn Sie sich für den Kurs einschreiben, erhalten Sie Zugang zu allen Kursen der Specializations, und Sie erhalten ein Zertifikat, wenn Sie die Arbeit abgeschlossen haben. Ihr elektronisches Zertifikat wird Ihrer Erfolgsseite hinzugefügt - von dort aus können Sie Ihr Zertifikat ausdrucken oder zu Ihrem LinkedIn-Profil hinzufügen. Wenn Sie die Kursinhalte nur lesen und ansehen möchten, können Sie den Kurs kostenlos besuchen.
Wenn Sie ein Abonnement abgeschlossen haben, erhalten Sie eine kostenlose 7-tägige Testphase, in der Sie kostenlos kündigen können. Danach gewähren wir keine Rückerstattung, aber Sie können Ihr Abonnement jederzeit kündigen. Siehe unsere vollständigen Rückerstattungsbedingungen.
Weitere Fragen
Finanzielle Unterstützung verfügbar,