Understanding Generative AI Risks: A Learning Leaders Guide to Ethical, Data, and Legal Concerns
Learn how to evaluate and safeguard your organization from risks—including ethical, data, and legal concerns—as you navigate generative AI transformation.
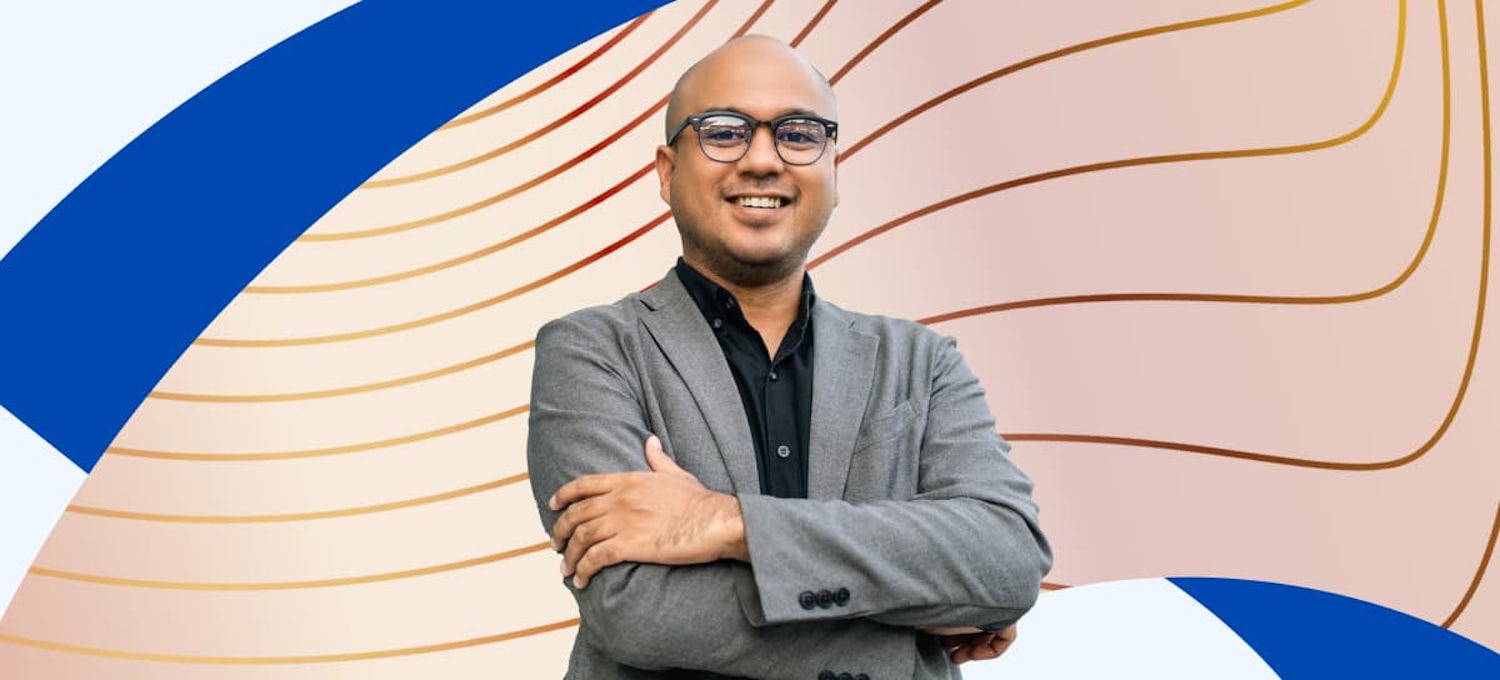
By Trena Minudri, VP & Chief Learning Officer
Key takeaways:
Despite GenAI being a top priority for leaders, two thirds are ambivalent or dissatisfied with their progress with the technology.
Considering legal, data, and ethical concerns up front will help leaders create a GenAI policy to prepare for the GenAI transformation.
Ethical considerations include hallucinations, intellectual property, training models, and bias amplification.
With rapidly shifting regulations, business leaders need to reassess their compliance and data risk.
Providing both leaders and employees with training from trusted institutions is key to navigating Gen AI risks and ethics.
The business upside of generative AI (GenAI) has become abundantly clear—and not just to me.
Between increased productivity and new levels of innovation, McKinsey estimates GenAI could generate up to $4.4 trillion in value across industries. It’s no surprise that over half of top executives rated GenAI as a “top priority” in 2024.
But incorporating GenAI into your organization doesn’t come without risks, and plenty of business leaders are frustrated with roadblocks. Two-thirds of leaders are ambivalent or dissatisfied with their progress on GenAI.
One of the top three underlying reasons executives are dissatisfied with their progress?
The absence of a strategy for responsible AI.
Getting business value out of GenAI is a big undertaking, made more complicated by how quickly the space is evolving and the legal, data, and ethical concerns involved. "I think the big change that came with generative AI was simply the pace at which change was happening and perhaps even the scale of the impact of these changes,” shares Dr. Robert Brunner, Associate Dean of Innovation at the University of Illinois, in the course Setting a Generative AI Strategy.
At Coursera, we’re confident GenAI’s future can be determined by how we as business leaders use it—if we do so fairly, ethically, and responsibly.
To realize the business impact of GenAI, you need to define a strategy around the ethics, data privacy, and legality of large language models (LLMs) first. When you do, you’ll be able to cut through the chatter around GenAI concerns and confidently assess threats as you act on the opportunity genAI presents.
We recently explored how business leaders are navigating the change in our playbook How to Lead through Generative AI Transformation: Insights from Industry Experts.
In this follow-up, I further break down GenAI’s risks—and how they can be mitigated—with insights from expert practitioners at Microsoft, Dow, Vanderbilt University, and more.
You’ll become aware of the most pressing AI issues, so you can bring GenAI into your organization with minimized risk and an informed perspective.
[Disclaimer: These are suggestions from my experience and are intended for informational purposes. Please consult with your internal legal counsel and technical teams to determine the best course of action for you and your organization.]
Consider these ethical factors before you roll out GenAI
Hallucinations
Like humans, the internet, and most knowledge repositories, GenAI also provides inaccurate information at times—otherwise known as hallucinations. This often occurs when AI models are not used correctly or asked to complete tasks outside of their functional limitations.
In his course Navigating Generative AI Risks for Leaders, Coursera CEO Jeff Maggioncalda frames this well: “If you’re going to start using these models and expect that almost all of your employees will, they need to understand the limitations and the role that they play as individuals in making sure they validate and reflect on what comes out of these models.”
Intellectual property
When LLMs are trained on internet-wide source material without expressed user or owner permission, this calls into question the intellectual property rights of trademarked, copyrighted, and sensitive material.
Who does data actually belong to, and do we have the right to use it freely?
“When machines can manipulate language created by other people, those machines can get a lot more value out of that language,” says Jeff. “LLMs can be trained with knowledge and intellectual property can be infused into derivative pieces of work” without the end user being aware.
Responsible GenAI use starts at the very beginning—how do we ensure we’re evaluating data outputs even as we use and train models? This brings us to our next point.
Training models
GenAI comes with an inherent set of risks, but it’s up to leaders to determine how much risk they’d like the business to take on.
If your org trains models for internal use only, you’ll lead with minimal risk. This risk increases exponentially when external partners like stakeholders, vendors, or even customers use the LLMs you train.
So where to start?
“I think you start internally,” shares Dr. Jules White, a Coursera instructor and expert in GenAI at Vanderbilt University. “First, you build up the expertise, the responsibility within your own workforce, and then you start figuring out the safe ways to take it outside.” Dr. White points to an example: adopting Microsoft Copilot as a start to train employees on the capabilities and limitations of GenAI within your domain.
[Side note: for a fantastic introduction to trustworthy GenAI, head over to Dr. White’s course.]
When training models, business leaders need to consider not only what data they input into LLMs to do so, but also how they integrate company ethos and values to minimize digital harm and pave the way for quality outputs.
Bias amplification
When used properly, GenAI enables smarter decision-making by becoming a second brain of sorts: LLMs can evaluate every element of a scenario and share alternate viewpoints.
Yet there’s an unfortunate consequence if the source data fed into LLMs is biased—the outputs become biased and amplified. This is called machine bias, which can lead to increased stereotyping and inaccurate information.
GenAI models are susceptible to multiple kinds of biases, including availability bias, or the favoring of more widespread data, and confirmation bias, in which all prompts heavily generate one desired or stereotypical output.
This is just one of the reasons an established AI governance policy to proactively address this risk is so important for companies. IBM proposes a few initial best practices for avoiding bias amplification, including a “human-in-the-loop” system and paying close attention to compliance, trust, and fairness standards in your GenAI governance.
Get familiar with data security and regulation concerns
Rapidly shifting regulations
As GenAI tools continue to improve and evolve, regulations and laws governing their actions will follow suit and shift often in the coming years.
Laws vary based on the country your company is based in, and sometimes even the state or region. Keep an eye on credible sources, like emerging public policy shifts or legal cases, to stay agile as regulations change.
“Part of the ethical responsibility of CEOs is to not only understand how GenAI works today but to have some anticipation for how it’s changing,” Jeff shares. “Because we don’t know what capabilities will exist in a year or two or three from now. We need to anticipate how they might impact employees and customers and society.”
Compliance risk
As your organization integrates GenAI, you need to consider liability and compliance risks to avoid hefty fines and data infractions that could harm your customers or company.
Communicate closely with your Legal team and AI leaders to understand how your organization can stay compliant as new GenAI capabilities continue to unfold.
“Engage the appropriate groups within your company, early and often,” says Alison Klein, Information Systems Talent Manager at Dow. “This looks different for each organization, but we’re working closely with our legal team to understand the protocols we need and what training employees need to complete.”
AI leaders within your organization should also implement ongoing risk assessments to stay ahead of any emerging threats.
Data risk
Your employees will likely be inputting potentially sensitive or proprietary company data into LLMs, so data risk via cyberattack is a major concern. Executive teams will need to provide critical oversight for data protection measures and risk management strategies. As Graeme Malcolm, Principal Content Development Manager, Data and AI at Microsoft shares, this problem is twofold: “There's ‘how do I, as an organization, ensure that we use this technology responsibly, and then there’s ‘how do we guard against those who might not?’”
From an internal standpoint, business leaders should start by defining how LLMs are used across the organization.
“Where will we be using these tools? Who will be using them? How do we want to think about the way that tools will be used over time in different kinds of contexts?” asks Dr. Alondra Nelson, a leading author of the White House-sanctioned AI Bill of Rights. Getting a level set on which tools your team is using and where they’re being used gives you a starting point for controlling data risk as teams adopt GenAI.
Key principles for responsibly adopting GenAI
A lack of knowledge about GenAI and fear-mongering discourse can keep business leaders stuck. But inaction isn’t the solution; it will only lead to missed growth opportunities and productivity losses.
1. Tighten up data practices
Business leaders should start by creating data practice guidelines and segmenting them by function across the organization to reduce the risk of inappropriate use, cyber threats, and privacy breaches. While teams can adopt data practices for their respective job duties, the CEO and other business leaders are both instrumental in driving home the importance of data safety more broadly through effective communication and frequent follow-up.
Start by making data privacy a priority. You’ll want to oversee how different teams interact with data and develop use cases around what data can and cannot be shared with LLMs. For instance, at Coursera, we use a safe and secure Playground environment for working with LLMs.
2. Create an AI ethics policy
“Data privacy and security for AI starts by having a really good understanding of the new risks posed by LLMs in particular because GenAI is so new,” notes Clara Shih, CEO of Salesforce AI, in the course Empowering and Transforming Your Organization with GenAI. “Organizations need to have safeguards, both through systems and technology, but also policies and procedures,”
Since GenAI will be used in myriad ways across your company, it’s key to create standards and policies regarding when it’s appropriate to use the tool in the first place. Enter a GenAI ethics policy framework.
“Putting those boundaries and frameworks in place sends a signal to your company that this really matters,” emphasizes Jeff. “It gives the guardrails for what people can and cannot do.”
3. Monitor the landscape, and keep learning
Keep up with emerging trends, the discourse surrounding GenAI, and policy updates. By staying up to speed on different angles and opportunities with GenAI, you’ll make better-informed decisions that will positively impact your organization, your employees, and your stakeholders.
Course recommendations:
Google AI Essentials: Google AI Essentials is a self-paced course designed to help people across roles and industries get essential AI skills to boost their productivity, zero experience required. The course is taught by AI experts at Google who are working to make the technology helpful for everyone.
Generative AI: Impact, Considerations, and Ethical Issues: Led by IBM’s Rav Ahuja, this course will help you identify the ethical issues, concerns, and misuses associated with generative AI.
Responsible AI in the Generative AI Era: In this course from Fractal, you will explore the fundamental principles of responsible AI, and understand the need for developing Generative AI tools responsibly.
4. Train your team
At a base level, you need to understand three things:
How GenAI tools work
Who they impact
How to train your team on using GenAI responsibly and ethically
Cross-functional teams and executives alike must do the work to learn how LLM outputs impact employees and customers—and that work should start today. But it doesn’t end there. Business leaders should also prioritize training their employees on GenAI—including legal, data, and ethical concerns—early on. Alison Klein agrees: “Offering appropriate training in conjunction with the GenAI rollout will be the key to successful adoption.”
Lead with confidence in the age of GenAI
Even the most respected thought leaders out there can’t 100% predict where GenAI is going next. That’s why business leaders need to move forward with an informed perspective, so they can make the most of benefits data scientists are hopeful about, like increased organizational productivity thanks to automation and better strategic thinking.
Discover more in-depth tips and case studies in How to Lead through Generative AI Transformation: Insights from Industry Experts.
- Expert content from leading GenAI innovators such as Microsoft, Google, Stanford Online, and IBM.
- Curated programs for different teams and career levels, including executives.
- Hands-on practice in secure GenAI Playgrounds, allowing employees to apply their learning in real-world scenarios.
- Interactive guidance from Coursera Coach for effective learning.
- Learning in preferred language with 20+ translations
- Accelerated course customization with AI-assisted Course Builder
This content has been made available for informational purposes only. Learners are advised to conduct additional research to ensure that courses and other credentials pursued meet their personal, professional, and financial goals.