This course is the second in a specialization for Machine Learning for Supply Chain Fundamentals. In this course, we explore all aspects of time series, especially for demand prediction. We'll start by gaining a foothold in the basic concepts surrounding time series, including stationarity, trend (drift), cyclicality, and seasonality. Then, we'll spend some time analyzing correlation methods in relation to time series (autocorrelation). In the 2nd half of the course, we'll focus on methods for demand prediction using time series, such as autoregressive models. Finally, we'll conclude with a project, predicting demand using ARIMA models in Python.

Offrez à votre carrière le cadeau de Coursera Plus avec $160 de réduction, facturé annuellement. Économisez aujourd’hui.
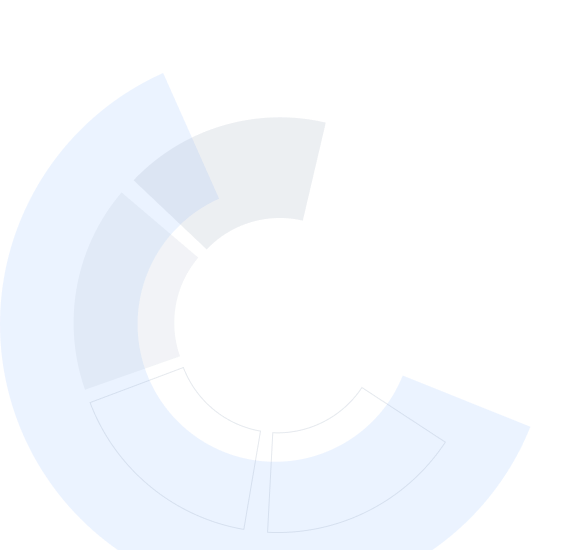
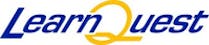
Demand Forecasting Using Time Series
Ce cours fait partie de Spécialisation Machine Learning for Supply Chains


Instructeurs : Rajvir Dua
3 437 déjà inscrits
Inclus avec
(31 avis)
Expérience recommandée
Ce que vous apprendrez
Building ARIMA models in Python to make demand predictions
Developing the framework for more advanced neural netowrks (such as LSTMs) by understanding autocorrelation and autoregressive models.
Compétences que vous acquerrez
- Catégorie : Python Programming
- Catégorie : Autoregressive Integrated Moving Average (ARIMA)
- Catégorie : Time Series
- Catégorie : Machine Learning
- Catégorie : Demand Forecasting
Détails à connaître

Ajouter à votre profil LinkedIn
5 devoirs
Découvrez comment les employés des entreprises prestigieuses maîtrisent des compétences recherchées
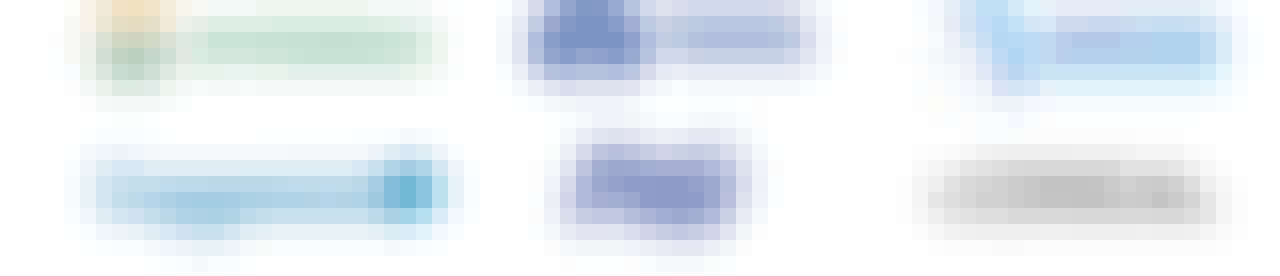
Élaborez votre expertise du sujet
- Apprenez de nouveaux concepts auprès d'experts du secteur
- Acquérez une compréhension de base d'un sujet ou d'un outil
- Développez des compétences professionnelles avec des projets pratiques
- Obtenez un certificat professionnel partageable
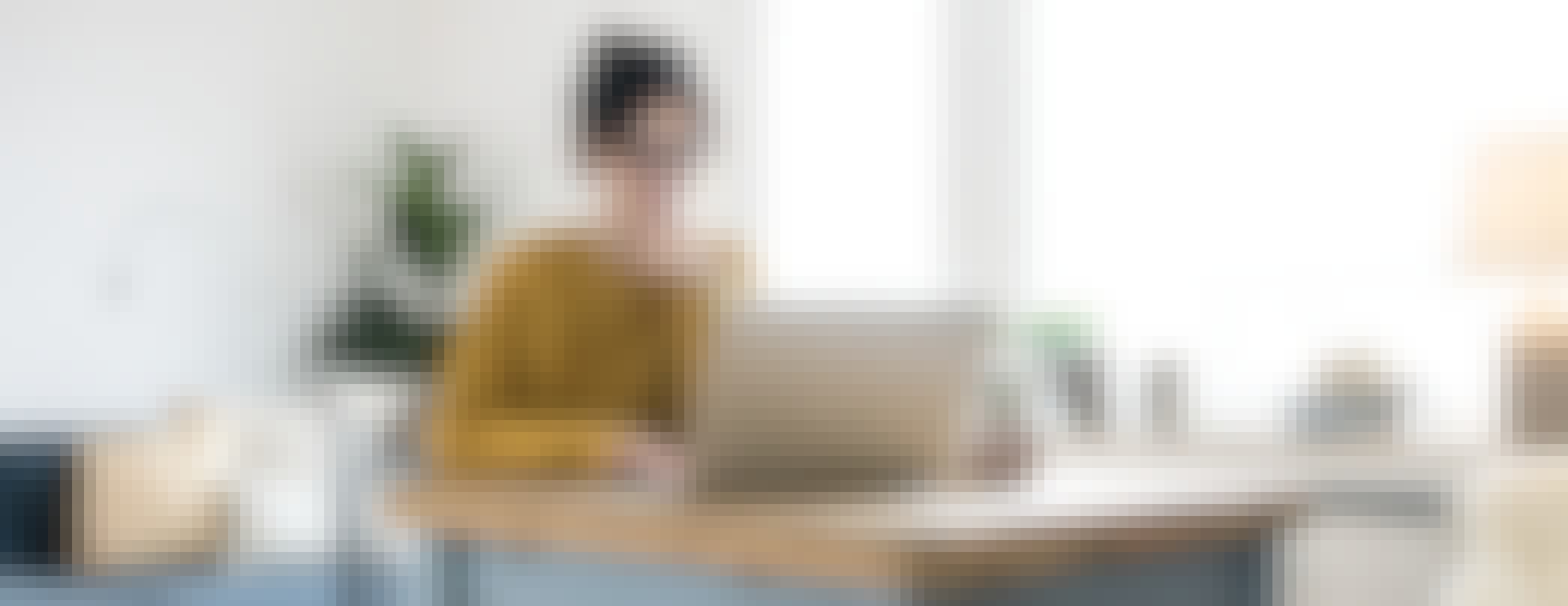
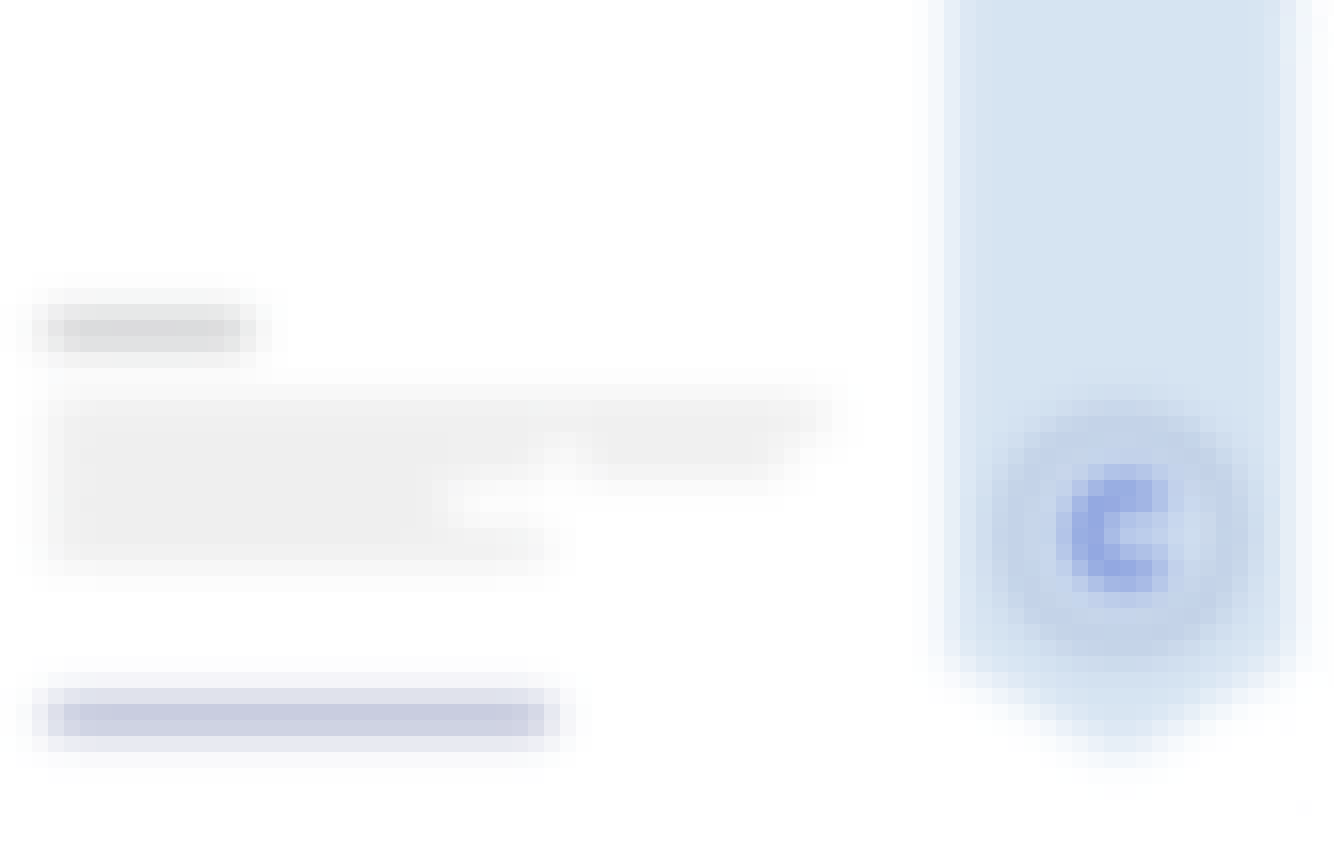
Obtenez un certificat professionnel
Ajoutez cette qualification à votre profil LinkedIn ou à votre CV
Partagez-le sur les réseaux sociaux et dans votre évaluation de performance
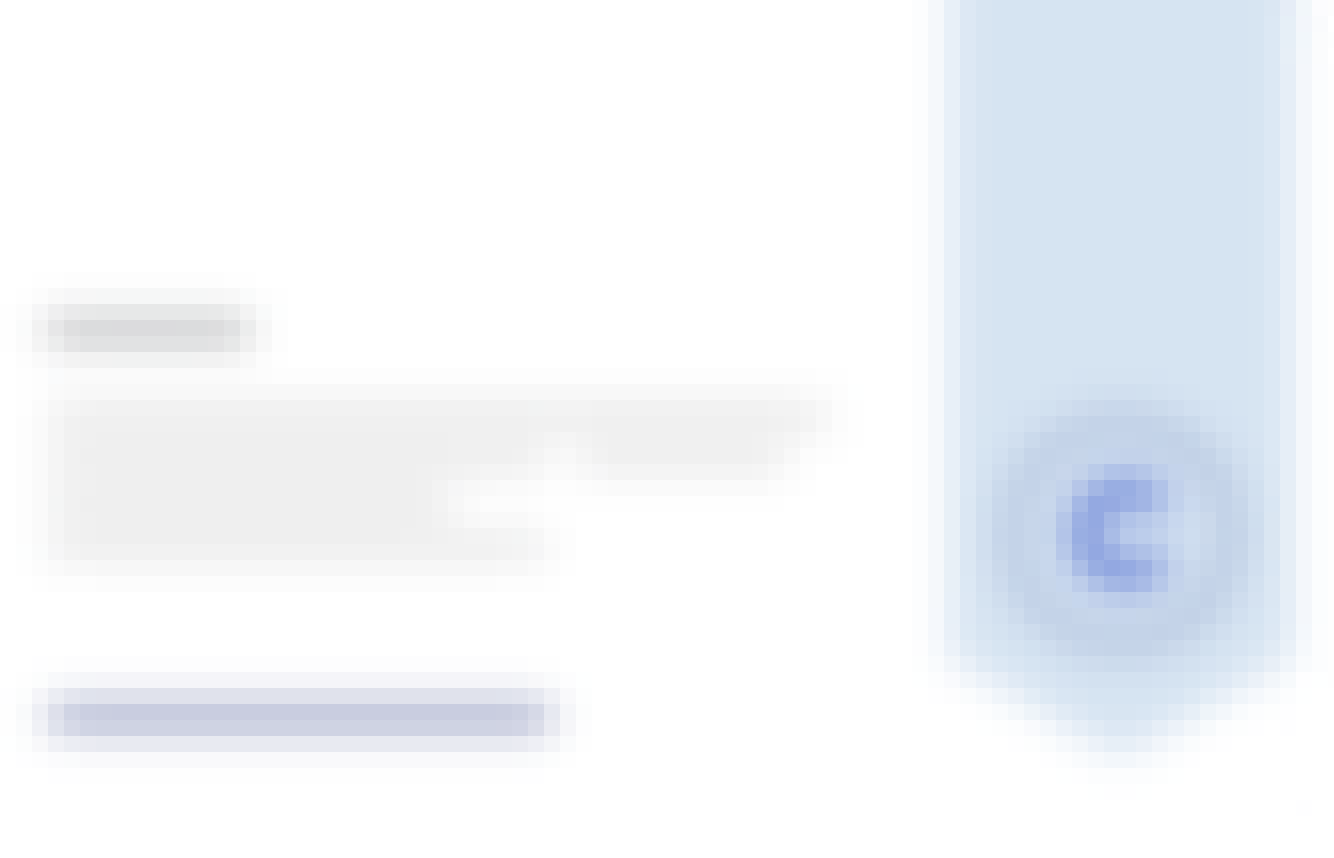
Il y a 4 modules dans ce cours
In this module, we'll get our feet wet with time series in Python. We'll start by getting familiar with where time series fits in to the machine learning landscape. Then, we'll learn about the main types of time series and their distinguishing factors, including period, frequency, and stationarity. After pausing to learn how to plot timeseries in Python, we'll explore the differences between seasonality and cyclicality.
Inclus
7 vidéos3 lectures2 devoirs1 sujet de discussion
In this module, we'll dive into the ideas behind autocorrelation and independence. We'll start by digging into the math of correlation and how it can be used to characterize the relationship between two variables. Next, we'll define its relationship to independence and explain where these ideas can be used. Finally, we'll combine correlation with time series attributes, such as trend, seasonality, and stationarity to derive autocorrelation. We'll go through both some of the theory behind autocorrelation, and how to code it in Python.
Inclus
8 vidéos2 lectures2 devoirs1 sujet de discussion
In this module, we'll start by reviewing some of the basic concepts behind linear regression. Then, we'll extend this knowledge to feed into lagged regression, an effective way to use regression techniques on time series. Once we have a solid foothold in basic and lagged regression, we'll explore modern methods such as ARIMA (autoregressive integrated moving average). All of this is building the framework for more advanced machine learning models such as LSTMs (long short-term memory network).
Inclus
4 vidéos1 lecture1 devoir1 devoir de programmation1 sujet de discussion1 laboratoire non noté
In the final course project, we'll make demand predictions using ARIMA models.
Inclus
1 devoir de programmation1 laboratoire non noté
Instructeurs

Offert par
Recommandé si vous êtes intéressé(e) par Machine Learning
Google Cloud
LearnQuest
Queen Mary University of London
Pour quelles raisons les étudiants sur Coursera nous choisissent-ils pour leur carrière ?




Avis des étudiants
Affichage de 3 sur 31
31 avis
- 5 stars
46,87 %
- 4 stars
6,25 %
- 3 stars
9,37 %
- 2 stars
15,62 %
- 1 star
21,87 %
Révisé le 12 sept. 2022

Ouvrez de nouvelles portes avec Coursera Plus
Accès illimité à plus de 7 000 cours de renommée internationale, à des projets pratiques et à des programmes de certificats reconnus sur le marché du travail, tous inclus dans votre abonnement
Faites progresser votre carrière avec un diplôme en ligne
Obtenez un diplôme auprès d’universités de renommée mondiale - 100 % en ligne
Rejoignez plus de 3 400 entreprises mondiales qui ont choisi Coursera pour les affaires
Améliorez les compétences de vos employés pour exceller dans l’économie numérique
Foire Aux Questions
Access to lectures and assignments depends on your type of enrollment. If you take a course in audit mode, you will be able to see most course materials for free. To access graded assignments and to earn a Certificate, you will need to purchase the Certificate experience, during or after your audit. If you don't see the audit option:
The course may not offer an audit option. You can try a Free Trial instead, or apply for Financial Aid.
The course may offer 'Full Course, No Certificate' instead. This option lets you see all course materials, submit required assessments, and get a final grade. This also means that you will not be able to purchase a Certificate experience.
When you enroll in the course, you get access to all of the courses in the Specialization, and you earn a certificate when you complete the work. Your electronic Certificate will be added to your Accomplishments page - from there, you can print your Certificate or add it to your LinkedIn profile. If you only want to read and view the course content, you can audit the course for free.
If you subscribed, you get a 7-day free trial during which you can cancel at no penalty. After that, we don’t give refunds, but you can cancel your subscription at any time. See our full refund policy.