The course "Mastering Neural Networks and Model Regularization" dives deep into the fundamentals and advanced techniques of neural networks, from understanding perceptron-based models to implementing cutting-edge convolutional neural networks (CNNs). This course offers hands-on experience with real-world datasets, such as MNIST, and focuses on practical applications using the PyTorch framework. Learners will explore key regularization techniques like L1, L2, and drop-out to reduce model overfitting, as well as decision tree pruning.

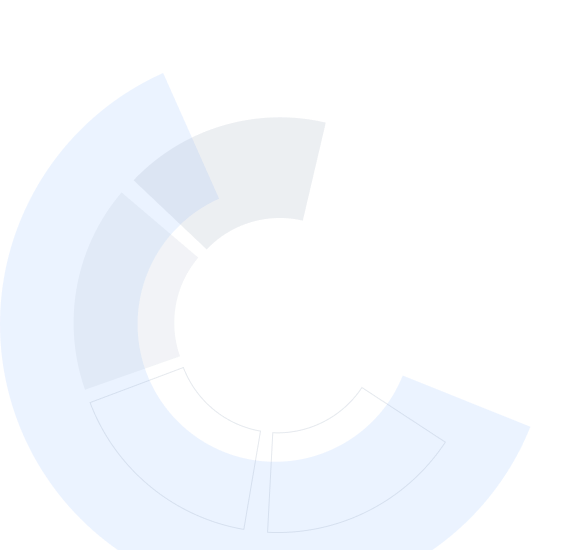

Mastering Neural Networks and Model Regularization
Ce cours fait partie de Spécialisation Applied Machine Learning

Instructeur : Erhan Guven
Inclus avec
Expérience recommandée
Ce que vous apprendrez
Build neural networks from scratch and apply them to real-world datasets like MNIST.
Apply back-propagation for optimizing neural network models and understand computational graphs.
Utilize L1, L2, drop-out regularization, and decision tree pruning to reduce model overfitting.
Implement convolutional neural networks (CNNs) and tensors using PyTorch for image and audio processing.
Compétences que vous acquerrez
- Catégorie : PyTorch Proficiency
- Catégorie : Regularization Techniques
- Catégorie : Neural Network Implementation
- Catégorie : Convolutional Neural Networks (CNNs)
- Catégorie : Back-Propagation Mastery
Détails à connaître

Ajouter à votre profil LinkedIn
septembre 2024
12 devoirs
Découvrez comment les employés des entreprises prestigieuses maîtrisent des compétences recherchées
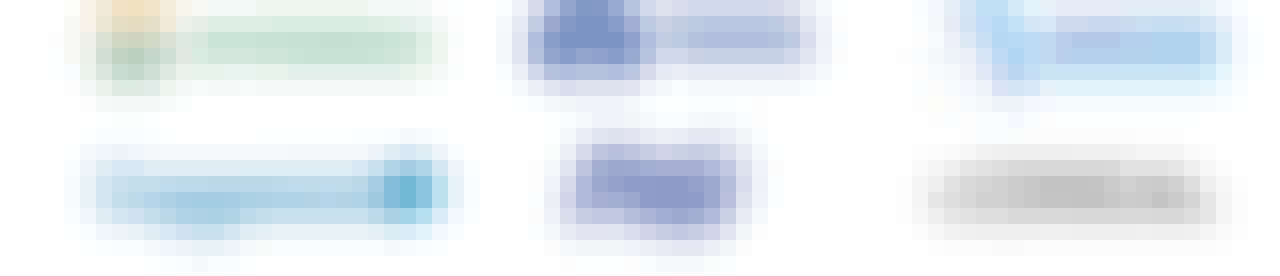
Élaborez votre expertise du sujet
- Apprenez de nouveaux concepts auprès d'experts du secteur
- Acquérez une compréhension de base d'un sujet ou d'un outil
- Développez des compétences professionnelles avec des projets pratiques
- Obtenez un certificat professionnel partageable
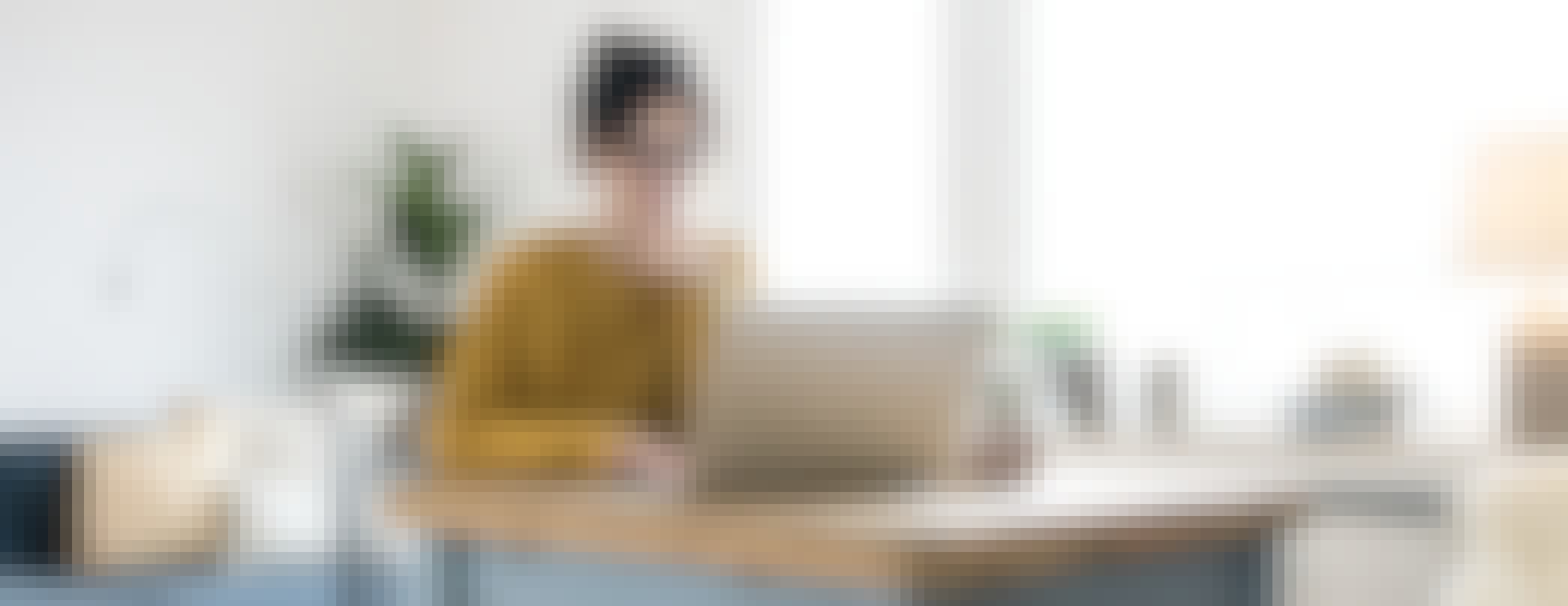
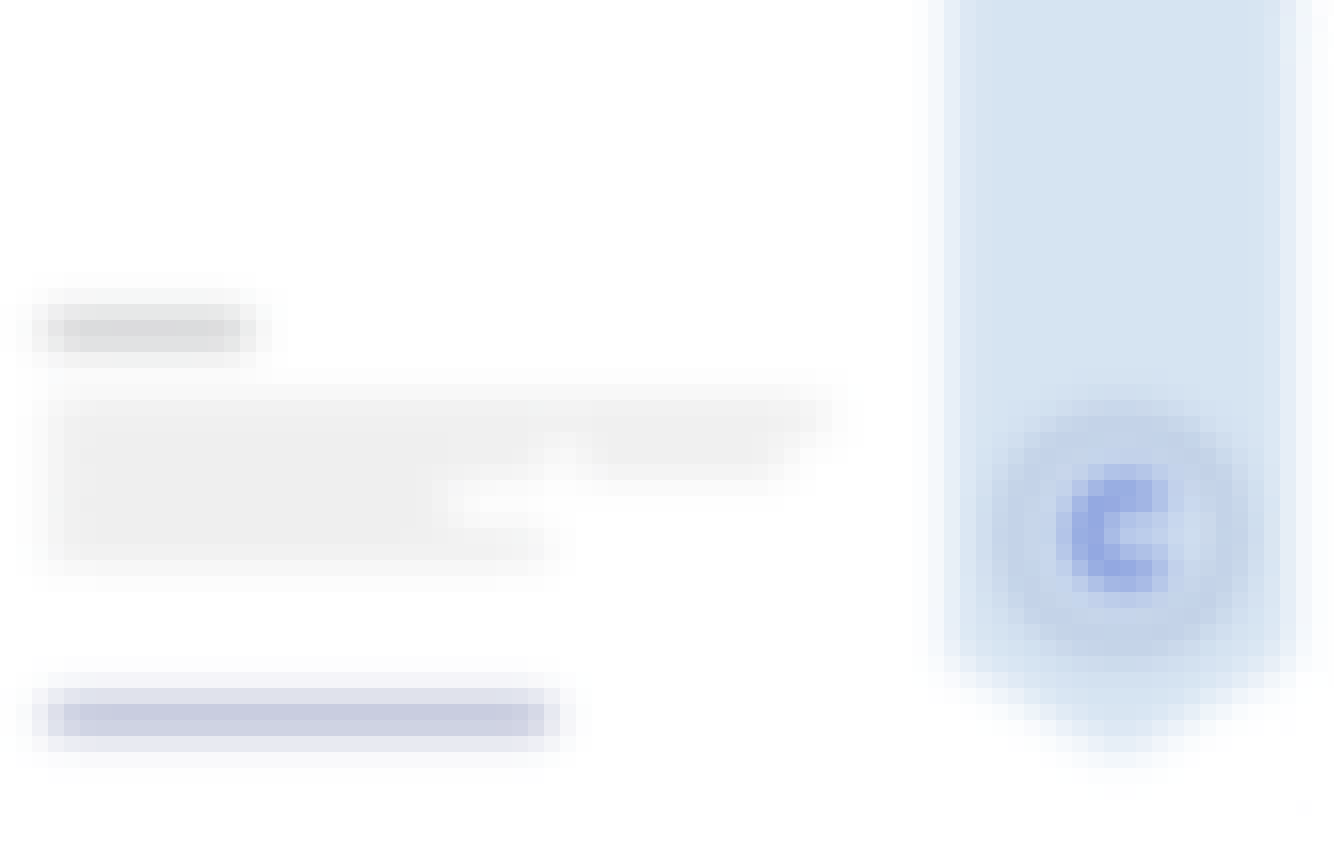
Obtenez un certificat professionnel
Ajoutez cette qualification à votre profil LinkedIn ou à votre CV
Partagez-le sur les réseaux sociaux et dans votre évaluation de performance
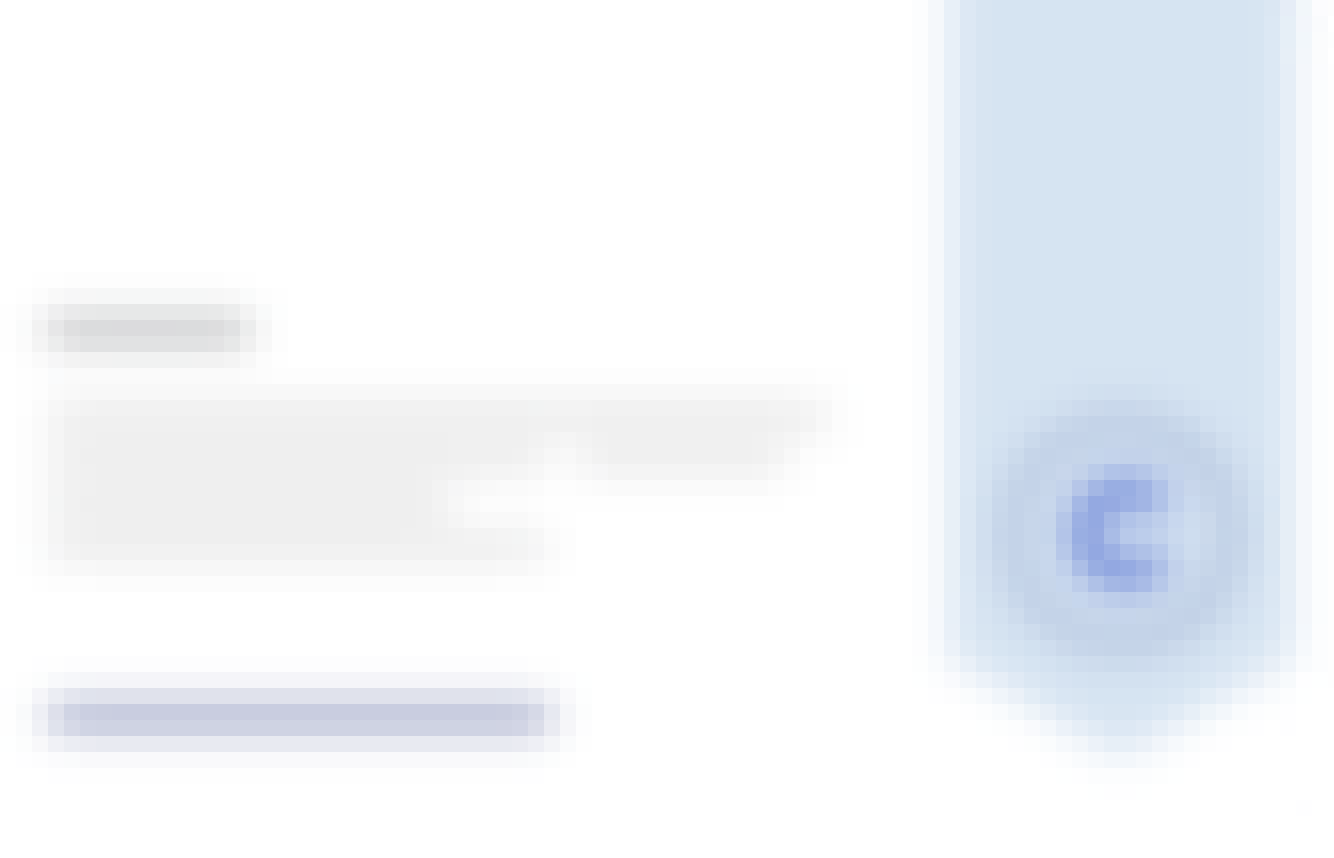
Il y a 5 modules dans ce cours
This course provides a comprehensive introduction to neural networks, focusing on the perceptron model, regularization techniques, and practical implementation using PyTorch. Students will build and evaluate neural networks, including convolutional architectures for image processing and audio signal modeling. Emphasis will be placed on comparing performance metrics and understanding advanced concepts like computational graphs and loss functions. By the end of the course, participants will be equipped with the skills to effectively design, implement, and optimize neural network models.
Inclus
2 lectures
In this module, you will learn about the fundamental concepts in neural networks, covering the perceptron model, model parameters, and the back-propagation algorithm. You'll also learn to implement a neural network from scratch and apply it to classify MNIST images, evaluating performance against sklearn's library function.
Inclus
4 vidéos2 lectures3 devoirs1 laboratoire non noté
In this module, you'll delve into techniques to enhance machine learning model performance and generalization. You'll grasp the necessity of regularization to mitigate overfitting, compare L1 and L2 regularization methods, understand decision tree pruning, explore dropout regularization in neural networks, and observe how regularization shapes model decision boundaries.
Inclus
3 vidéos3 lectures3 devoirs1 laboratoire non noté
In this module, you'll cover essential concepts and practical skills in deep learning using PyTorch. You'll also learn computational graphs in supervised learning, create and manipulate tensors in PyTorch, compare activation and loss functions, learn implementation steps and library functions for neural network training, and optimize models by running them on GPU for enhanced performance.
Inclus
3 vidéos2 lectures3 devoirs1 laboratoire non noté
In this module, you'll focus on advanced applications of convolutional neural networks (CNNs) using PyTorch. You'll also learn to implement CNN filters, compare different CNN architectures, develop models for image processing tasks in PyTorch, and explore techniques for modeling audio time signals using Spectrogram features for enhanced analysis and classification.
Inclus
2 vidéos3 lectures3 devoirs1 devoir de programmation
Instructeur

Offert par
Recommandé si vous êtes intéressé(e) par Machine Learning
Coursera Project Network
Pour quelles raisons les étudiants sur Coursera nous choisissent-ils pour leur carrière ?





Ouvrez de nouvelles portes avec Coursera Plus
Accès illimité à plus de 7 000 cours de renommée internationale, à des projets pratiques et à des programmes de certificats reconnus sur le marché du travail, tous inclus dans votre abonnement
Faites progresser votre carrière avec un diplôme en ligne
Obtenez un diplôme auprès d’universités de renommée mondiale - 100 % en ligne
Rejoignez plus de 3 400 entreprises mondiales qui ont choisi Coursera pour les affaires
Améliorez les compétences de vos employés pour exceller dans l’économie numérique
Foire Aux Questions
Access to lectures and assignments depends on your type of enrollment. If you take a course in audit mode, you will be able to see most course materials for free. To access graded assignments and to earn a Certificate, you will need to purchase the Certificate experience, during or after your audit. If you don't see the audit option:
The course may not offer an audit option. You can try a Free Trial instead, or apply for Financial Aid.
The course may offer 'Full Course, No Certificate' instead. This option lets you see all course materials, submit required assessments, and get a final grade. This also means that you will not be able to purchase a Certificate experience.
When you enroll in the course, you get access to all of the courses in the Specialization, and you earn a certificate when you complete the work. Your electronic Certificate will be added to your Accomplishments page - from there, you can print your Certificate or add it to your LinkedIn profile. If you only want to read and view the course content, you can audit the course for free.
If you subscribed, you get a 7-day free trial during which you can cancel at no penalty. After that, we don’t give refunds, but you can cancel your subscription at any time. See our full refund policy.