This course is a complete guide to supervised and unsupervised learning using R, covering practical data science comprehensively. Companies globally use R to analyze vast data, and mastering it can enhance your career. Unlike other courses, this one provides in-depth knowledge of R's machine learning features, from data reading and cleaning to implementing and evaluating algorithms.

Offrez à votre carrière le cadeau de Coursera Plus avec $160 de réduction, facturé annuellement. Économisez aujourd’hui.
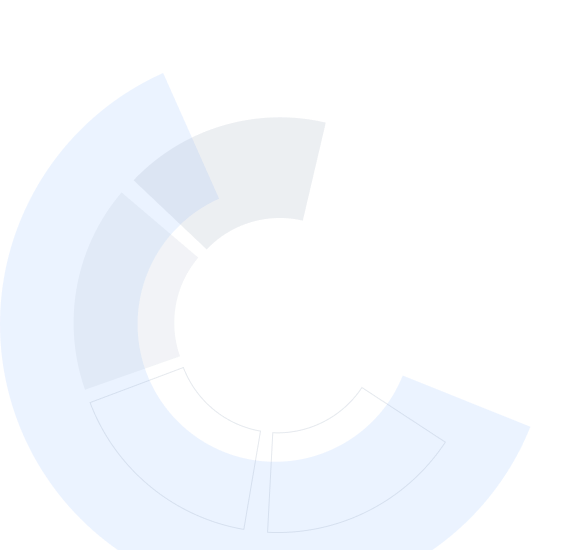
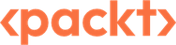
Expérience recommandée
Ce que vous apprendrez
Perform basic data pre-processing and wrangling in R Studio.
Implement and analyze unsupervised clustering techniques, such as K-means clustering.
Implement supervised learning techniques and classification methods, such as Random Forests.
Utilize dimensional reduction techniques (PCA) and feature selection.
Compétences que vous acquerrez
- Catégorie : R
- Catégorie : CSV
- Catégorie : Classification
- Catégorie : Machine Learning
- Catégorie : Clustering
Détails à connaître

Ajouter à votre profil LinkedIn
août 2024
11 devoirs
Découvrez comment les employés des entreprises prestigieuses maîtrisent des compétences recherchées
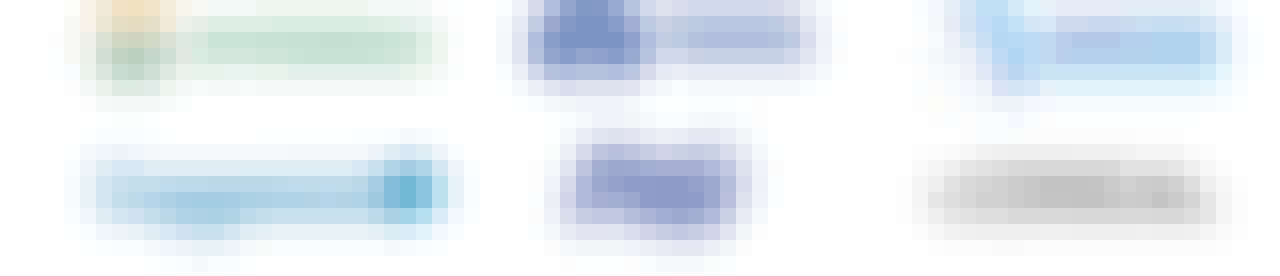
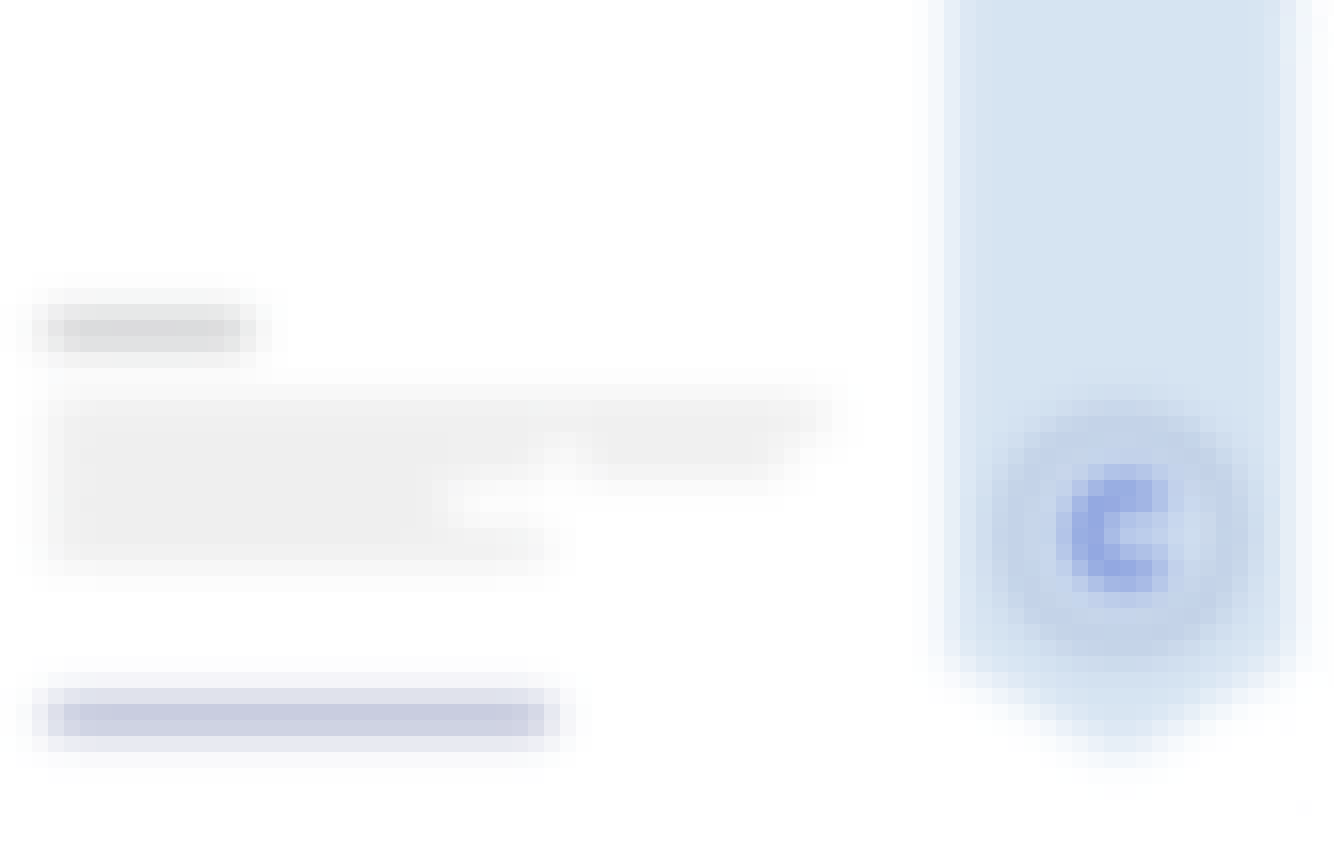
Obtenez un certificat professionnel
Ajoutez cette qualification à votre profil LinkedIn ou à votre CV
Partagez-le sur les réseaux sociaux et dans votre évaluation de performance
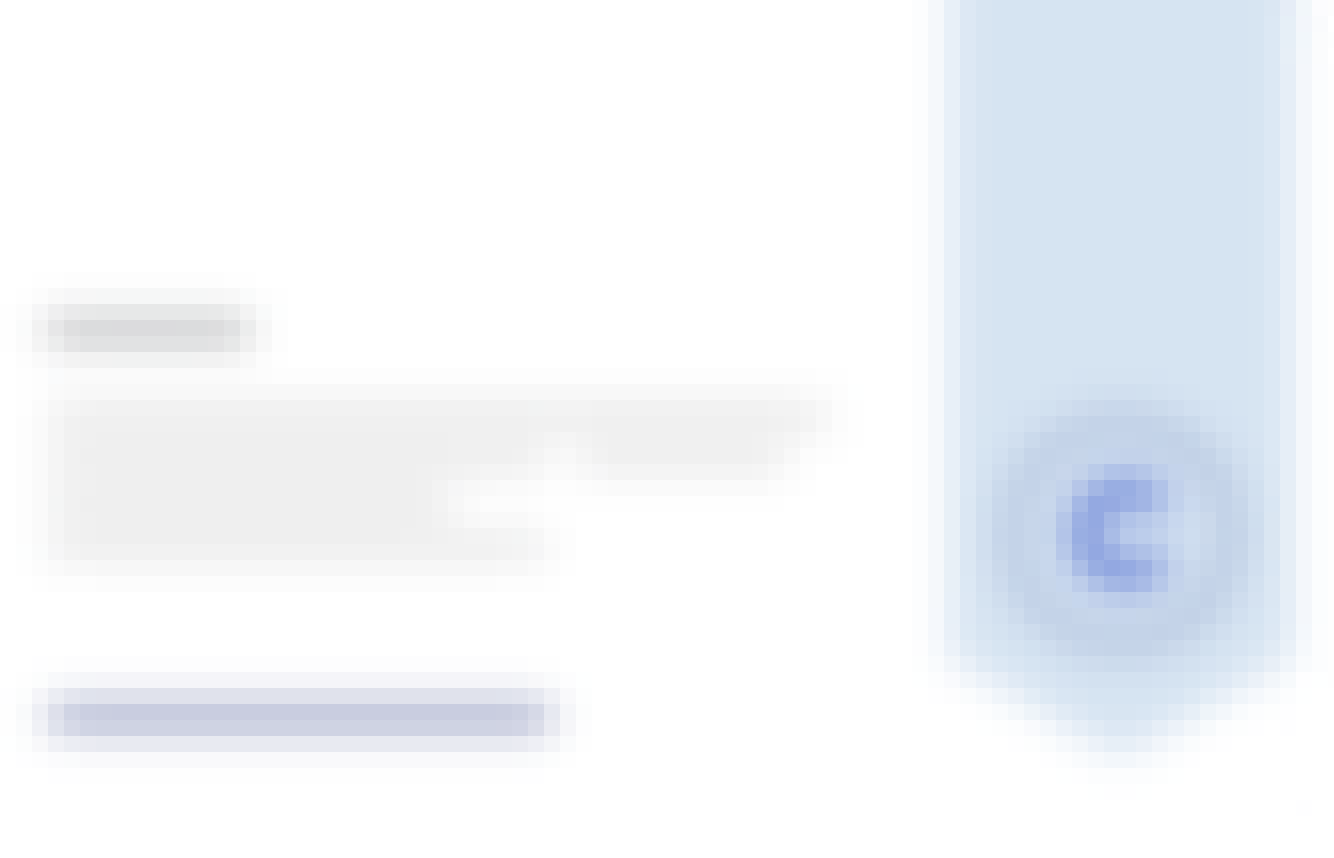
Il y a 10 modules dans ce cours
In this module, we will introduce the course, outlining the fundamental concepts of clustering and classification in machine learning. We will also guide you through the installation and setup of R and R Studio, ensuring you are prepared to dive into the practical aspects of the course.
Inclus
2 vidéos1 lecture1 devoir
In this module, we will explore the different methods to import data into R from various sources. You will learn to read data from CSV and Excel files, unzipped folders, online CSVs, Google Sheets, HTML tables, and databases, setting the foundation for data manipulation and analysis.
Inclus
7 vidéos1 devoir
In this module, we will delve into data cleaning and preprocessing, ensuring your data is ready for analysis. You will learn to summarize and explore data using the dplyr package and create visualizations with ggplot2. Additionally, we'll cover methods to evaluate associations between variables and test for correlation.
Inclus
11 vidéos1 devoir
In this module, we will explore the differences between machine learning and traditional statistical analysis, providing a theoretical overview of machine learning. You will gain a foundational understanding of machine learning concepts and their relevance to data science.
Inclus
2 vidéos1 devoir
In this module, we will cover unsupervised learning techniques, focusing on clustering algorithms. You will learn to implement and evaluate different clustering methods, including K-Means, Fuzzy K-Means, DBSCAN, and more. We'll also discuss how to select the best algorithm for your specific data needs.
Inclus
12 vidéos1 devoir
In this module, we will explore techniques for reducing the dimensionality of your data. You will learn the theoretical aspects of dimension reduction and how to apply methods such as PCA, Multidimensional Scaling, and SVD in R to simplify your datasets while preserving essential information.
Inclus
5 vidéos1 devoir
In this module, we will focus on feature selection techniques to identify the most relevant predictors for your models. You will learn to remove correlated variables and use methods like LASSO regression, FSelector, and Boruta analysis to select important features, enhancing your model's performance.
Inclus
4 vidéos1 devoir
In this module, we will introduce the fundamental concepts of supervised learning. You will learn how to preprocess data for supervised learning and gain insights into various types of supervised learning problems, preparing you for more advanced classification and regression techniques.
Inclus
2 vidéos1 devoir
In this module, we will delve into classification techniques in supervised learning. You will learn to implement logistic regression, Decision Trees, Random Forests, and Support Vector Machines (SVM). We will also cover methods to evaluate classification accuracy and understand variable importance in your models.
Inclus
18 vidéos1 devoir
In this module, we will provide additional lectures focusing on advanced clustering methods. You will learn about Fuzzy C-Means Clustering, understanding its theoretical underpinnings and practical applications in R, further enhancing your clustering analysis skills.
Inclus
1 vidéo2 devoirs
Instructeur

Offert par
Recommandé si vous êtes intéressé(e) par Machine Learning
University of Washington
University of Illinois Urbana-Champaign
Pour quelles raisons les étudiants sur Coursera nous choisissent-ils pour leur carrière ?





Ouvrez de nouvelles portes avec Coursera Plus
Accès illimité à plus de 7 000 cours de renommée internationale, à des projets pratiques et à des programmes de certificats reconnus sur le marché du travail, tous inclus dans votre abonnement
Faites progresser votre carrière avec un diplôme en ligne
Obtenez un diplôme auprès d’universités de renommée mondiale - 100 % en ligne
Rejoignez plus de 3 400 entreprises mondiales qui ont choisi Coursera pour les affaires
Améliorez les compétences de vos employés pour exceller dans l’économie numérique
Foire Aux Questions
Yes, you can preview the first video and view the syllabus before you enroll. You must purchase the course to access content not included in the preview.
If you decide to enroll in the course before the session start date, you will have access to all of the lecture videos and readings for the course. You’ll be able to submit assignments once the session starts.
Once you enroll and your session begins, you will have access to all videos and other resources, including reading items and the course discussion forum. You’ll be able to view and submit practice assessments, and complete required graded assignments to earn a grade and a Course Certificate.