"Trees, SVM and Unsupervised Learning" is designed to provide working professionals with a solid foundation in support vector machines, neural networks, decision trees, and XG boost. Through in-depth instruction and practical hands-on experience, you will learn how to build powerful predictive models using these techniques and understand the advantages and disadvantages of each. The course will also cover how and when to apply them to different scenarios, including binary classification and K > 2 classes. Additionally, you will gain valuable experience in generating data representations through PCA and clustering. With a focus on practical, real-world applications, this course is a valuable asset for anyone looking to upskill or move into the field of data science.

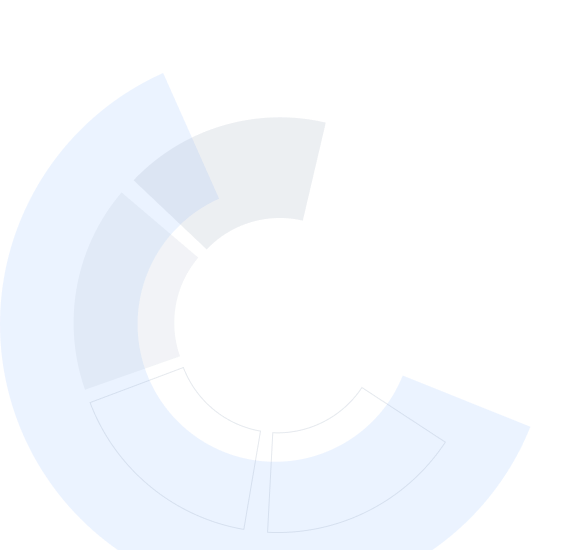
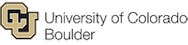
Trees, SVM and Unsupervised Learning
Ce cours fait partie de Spécialisation Statistical Learning for Data Science

Instructeur : Osita Onyejekwe
Inclus avec
Expérience recommandée
Expérience recommandée
Ce que vous apprendrez
Describe the advantages and disadvantages of trees, and how and when to use them.
Apply SVMs for binary classification or K > 2 classes.
Analyze the strengths and weaknesses of neural networks compared to other machine learning algorithms, such as SVMs.
Compétences que vous acquerrez
- Catégorie : Classification And Regression Tree (CART)
- Catégorie : Predictive Modeling
- Catégorie : Random Forest Algorithm
- Catégorie : Supervised Learning
- Catégorie : Artificial Neural Networks
- Catégorie : Feature Engineering
- Catégorie : Unsupervised Learning
- Catégorie : Statistics
- Catégorie : Applied Machine Learning
- Catégorie : Decision Tree Learning
- Catégorie : Machine Learning
- Catégorie : Dimensionality Reduction
Détails à connaître

Ajouter à votre profil LinkedIn
Découvrez comment les employés des entreprises prestigieuses maîtrisent des compétences recherchées
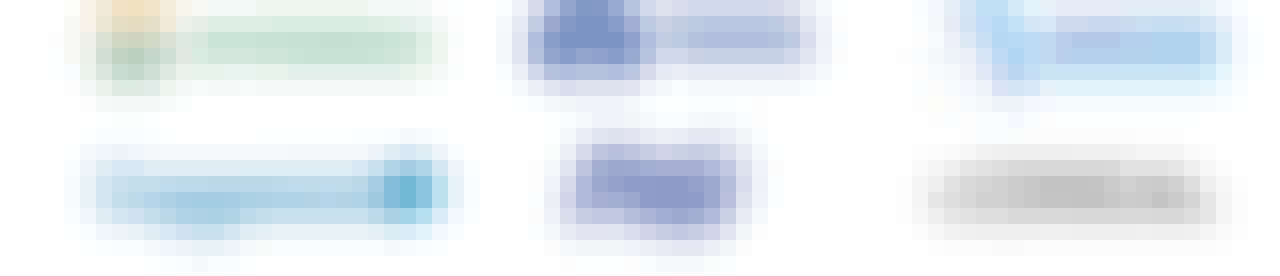
Élaborez votre expertise du sujet
- Apprenez de nouveaux concepts auprès d'experts du secteur
- Acquérez une compréhension de base d'un sujet ou d'un outil
- Développez des compétences professionnelles avec des projets pratiques
- Obtenez un certificat professionnel partageable
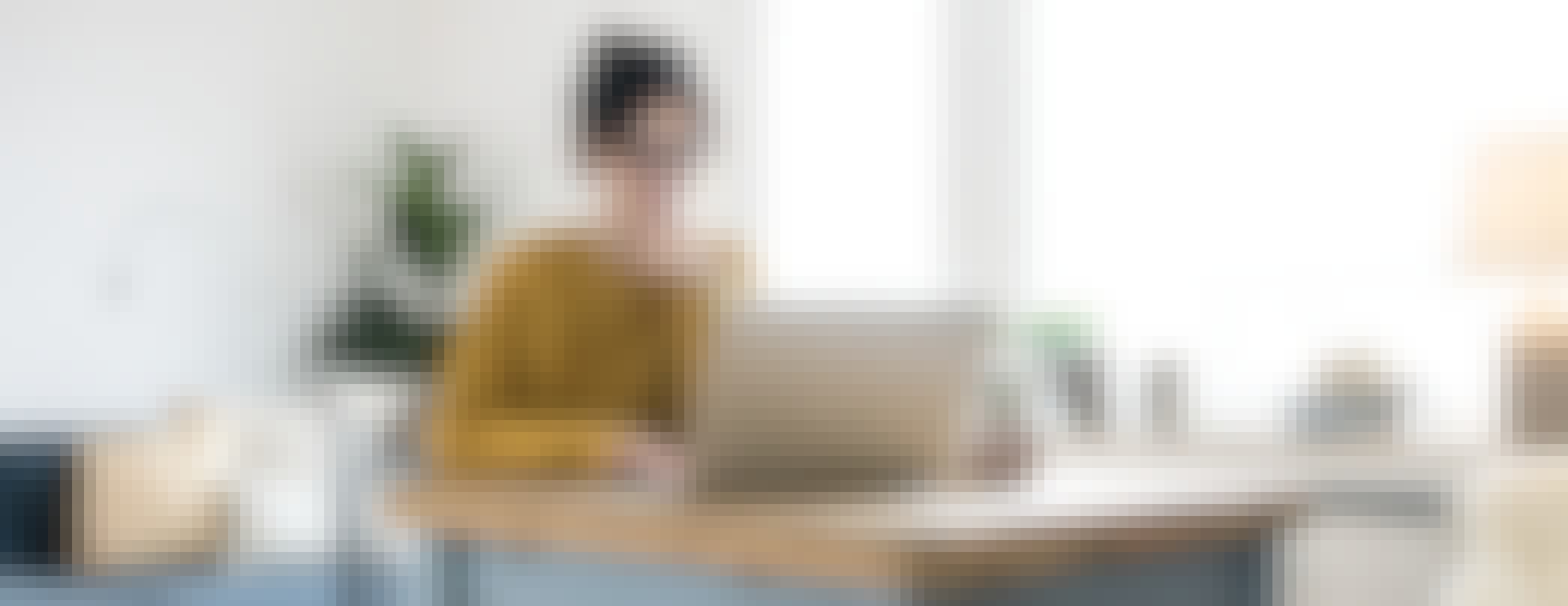
Il y a 4 modules dans ce cours
The module provides an introductory overview of the course and introduces the course instructor.
Inclus
1 vidéo2 lectures1 sujet de discussion
To begin the course, we will learn about support vector machines (SVMs). SVMs have become a popular method in the field of statistical learning due to their ability to handle non-linear and high-dimensional data. SVMs seek to maximize the margin, or distance between the decision boundary and the closest data points, to improve generalization performance. Throughout the week, you will learn how to apply SVMs to classify or predict outcomes in a given dataset, select appropriate kernel functions and parameters, and evaluate model performance
Inclus
4 vidéos1 lecture1 devoir de programmation1 laboratoire non noté
Neural Networks have become increasingly popular in the field of statistical learning due to their ability to model complex relationships in data. In this module, we will cover introductory concepts of neural networks, such as activation functions and backpropagation. You will have the opportunity to apply Neural Networks to classify or predict outcomes in a given dataset and evaluate model performance in the labs for this module.
Inclus
5 vidéos1 lecture1 devoir de programmation
Welcome to the final module for the course. This module will focus on the ensemble methods decision trees, bagging, and random forests, which combine multiple models to improve prediction accuracy and reduce overfitting. Decision Trees are a popular machine learning method that partitions the feature space into smaller regions and models the response variable in each region using simple rules. However, Decision Trees can suffer from high variance and instability, which can be addressed by Bagging and Random Forests. Bagging involves generating multiple trees on bootstrapped samples of the data and averaging their predictions, while Random Forests further decorrelate the trees by randomly selecting subsets of features for each tree.
Inclus
1 vidéo1 lecture1 devoir de programmation1 laboratoire non noté
Obtenez un certificat professionnel
Ajoutez ce titre à votre profil LinkedIn, à votre curriculum vitae ou à votre CV. Partagez-le sur les médias sociaux et dans votre évaluation des performances.
Instructeur

Offert par
En savoir plus sur Probability and Statistics
- Statut : Essai gratuit
University of Colorado Boulder
- Statut : Essai gratuit
- Statut : Essai gratuit
University of Colorado Boulder
- Statut : Essai gratuit
University of Colorado Boulder
Pour quelles raisons les étudiants sur Coursera nous choisissent-ils pour leur carrière ?





Ouvrez de nouvelles portes avec Coursera Plus
Accès illimité à 10,000+ cours de niveau international, projets pratiques et programmes de certification prêts à l'emploi - tous inclus dans votre abonnement.
Faites progresser votre carrière avec un diplôme en ligne
Obtenez un diplôme auprès d’universités de renommée mondiale - 100 % en ligne
Rejoignez plus de 3 400 entreprises mondiales qui ont choisi Coursera pour les affaires
Améliorez les compétences de vos employés pour exceller dans l’économie numérique
Foire Aux Questions
Access to lectures and assignments depends on your type of enrollment. If you take a course in audit mode, you will be able to see most course materials for free. To access graded assignments and to earn a Certificate, you will need to purchase the Certificate experience, during or after your audit. If you don't see the audit option:
The course may not offer an audit option. You can try a Free Trial instead, or apply for Financial Aid.
The course may offer 'Full Course, No Certificate' instead. This option lets you see all course materials, submit required assessments, and get a final grade. This also means that you will not be able to purchase a Certificate experience.
When you enroll in the course, you get access to all of the courses in the Specialization, and you earn a certificate when you complete the work. Your electronic Certificate will be added to your Accomplishments page - from there, you can print your Certificate or add it to your LinkedIn profile. If you only want to read and view the course content, you can audit the course for free.
If you subscribed, you get a 7-day free trial during which you can cancel at no penalty. After that, we don’t give refunds, but you can cancel your subscription at any time. See our full refund policy.
Plus de questions
Aide financière disponible,