The course "Applied Machine Learning: Techniques and Applications" focuses on the practical use of machine learning across various domains, particularly in computer vision, data feature analysis, and model evaluation. Learners will gain hands-on experience with key techniques, such as image processing and supervised learning methods while mastering essential skills in data pre-processing and model evaluation.

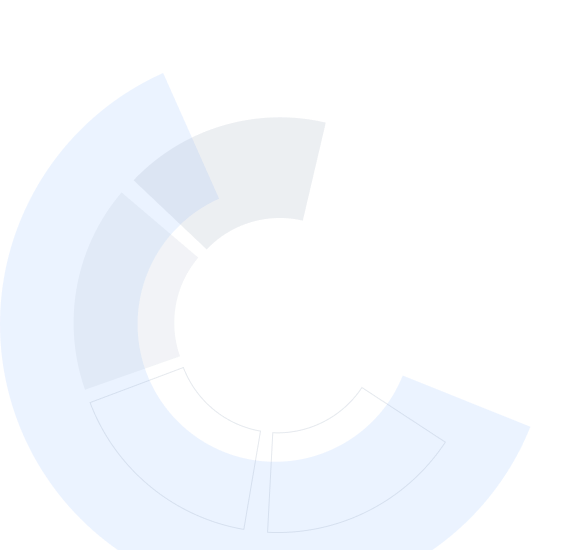

Applied Machine Learning: Techniques and Applications
This course is part of Applied Machine Learning Specialization

Instructor: Erhan Guven
Included with
Recommended experience
What you'll learn
Understand and implement machine learning techniques for computer vision tasks, including image recognition and object detection.
Analyze data features and evaluate machine learning model performance using appropriate metrics and evaluation techniques.
Apply data pre-processing methods to clean, transform, and prepare data for effective machine learning model training.
Implement and optimize supervised learning algorithms for classification and regression tasks.
Skills you'll gain
Details to know

Add to your LinkedIn profile
September 2024
12 assignments
See how employees at top companies are mastering in-demand skills
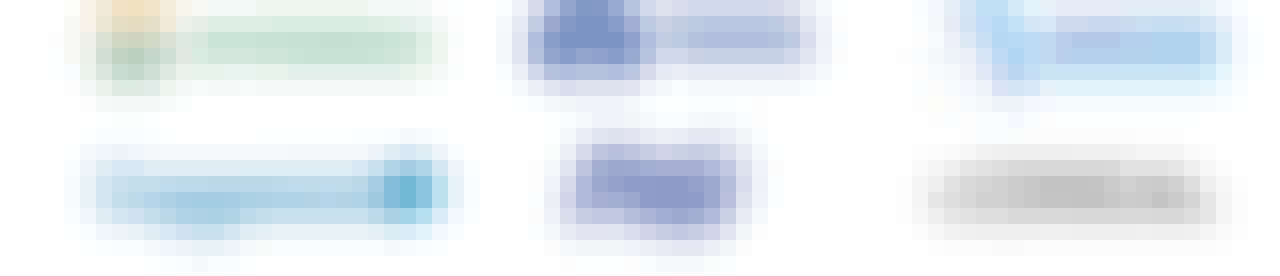
Build your subject-matter expertise
- Learn new concepts from industry experts
- Gain a foundational understanding of a subject or tool
- Develop job-relevant skills with hands-on projects
- Earn a shareable career certificate
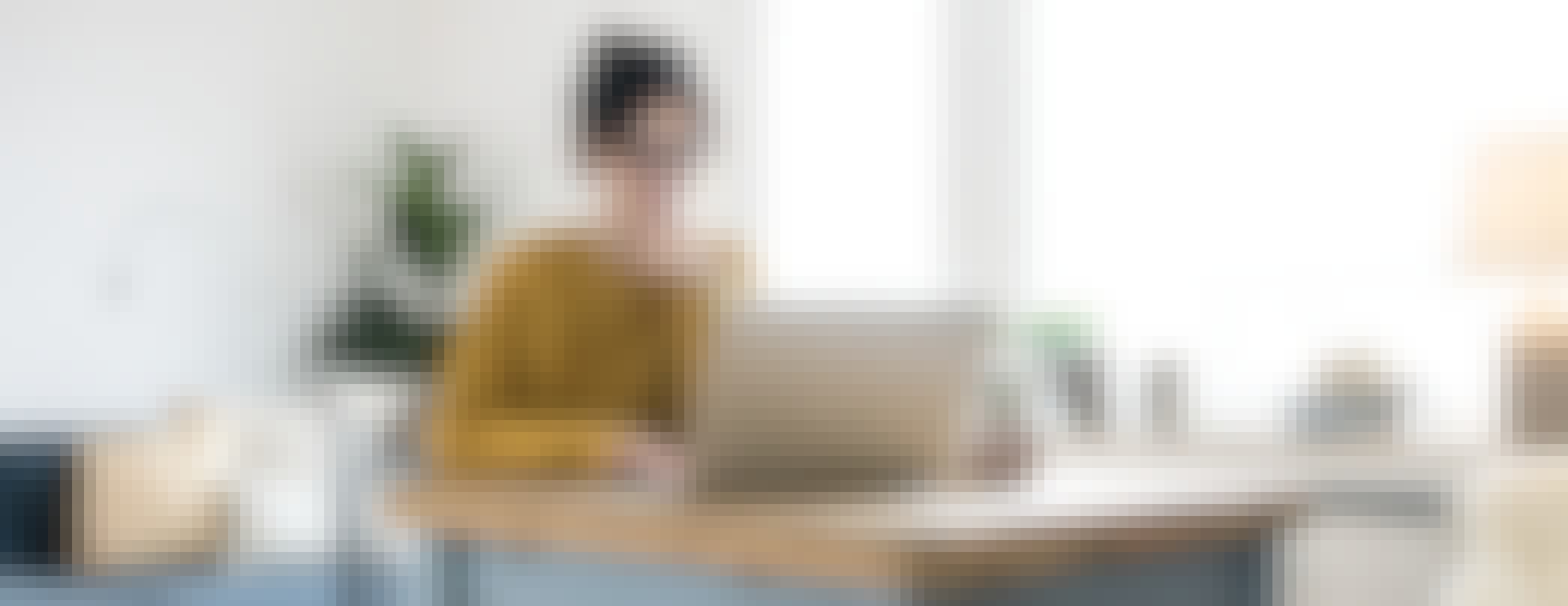
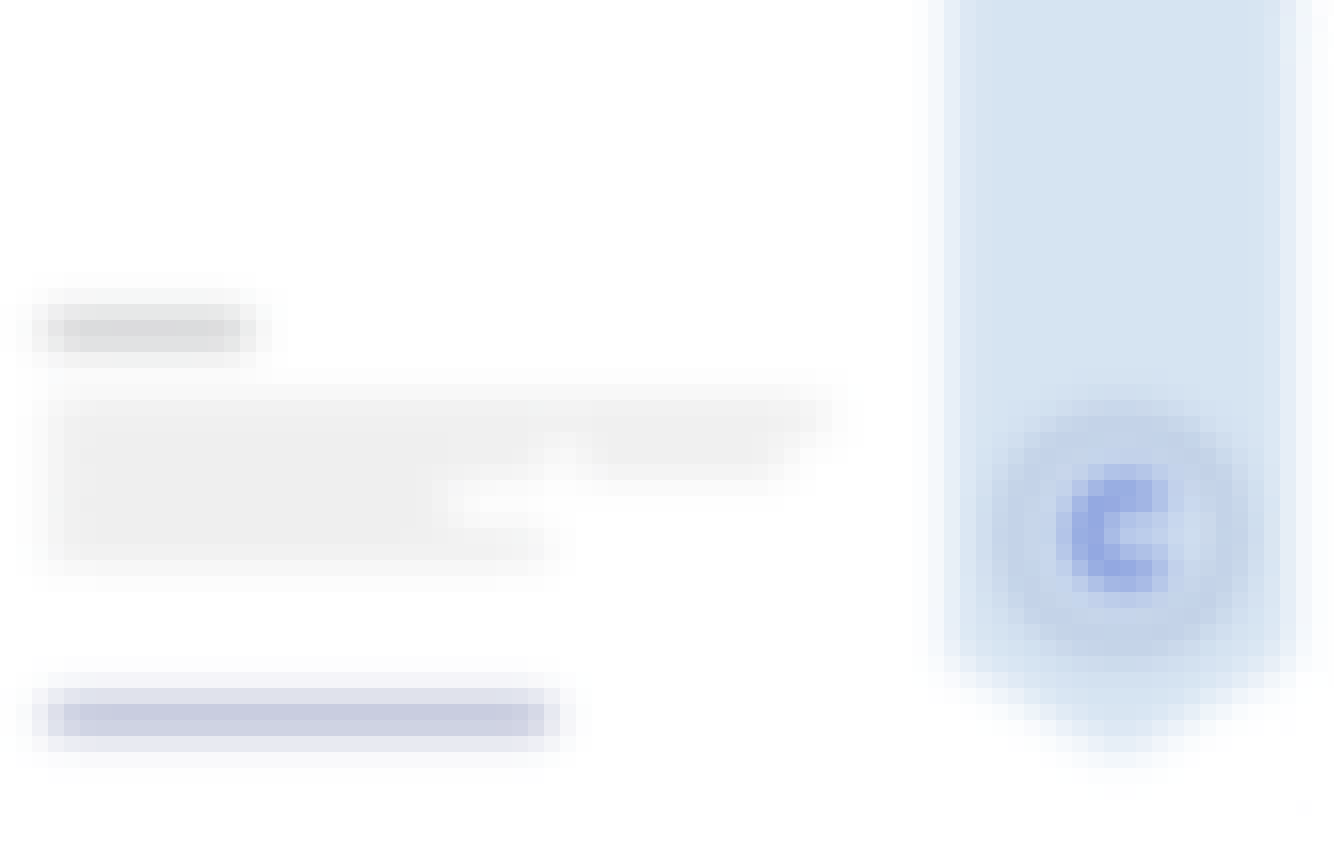
Earn a career certificate
Add this credential to your LinkedIn profile, resume, or CV
Share it on social media and in your performance review
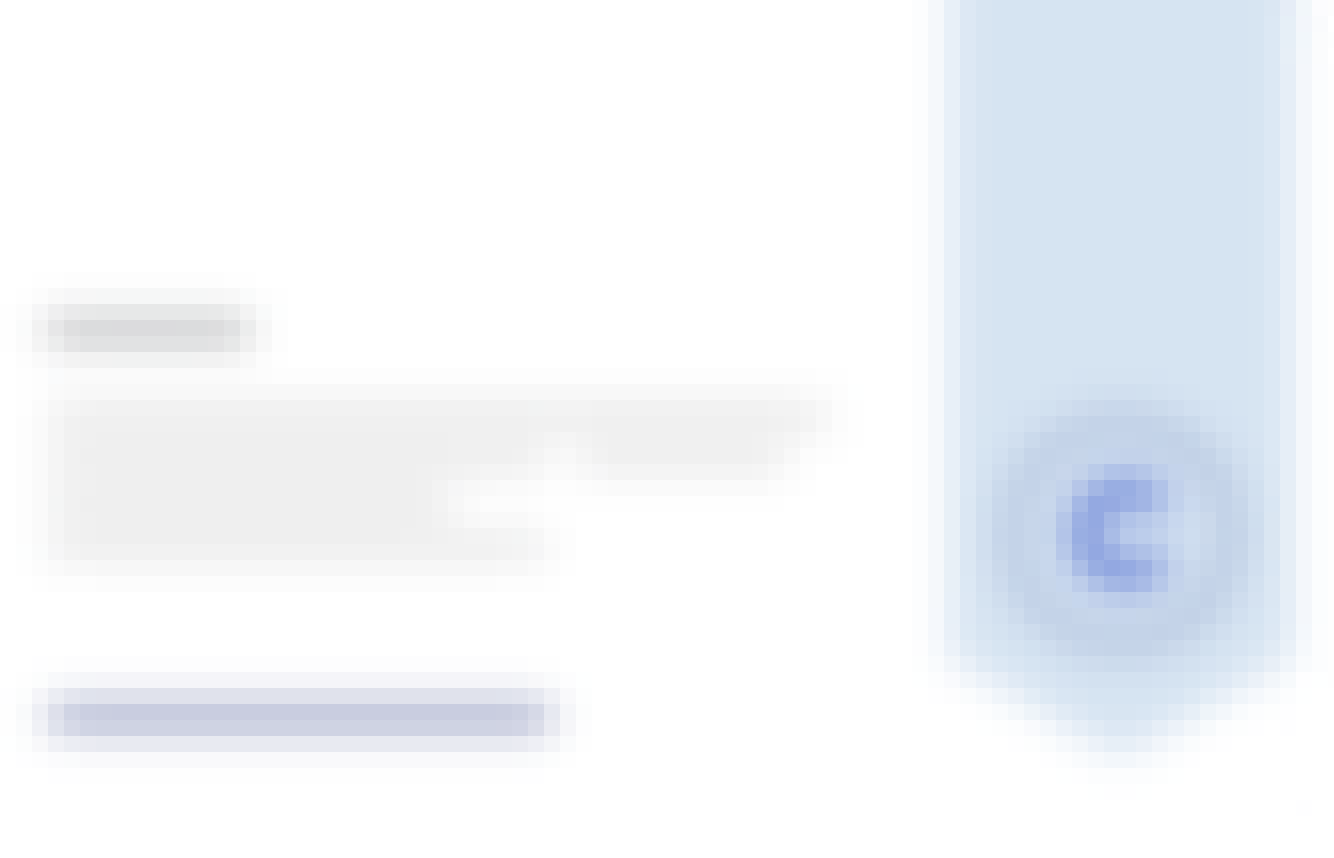
There are 5 modules in this course
Explore the practical applications of machine learning through hands-on modules covering data pre-processing, feature extraction, model evaluation, and supervised learning techniques. Delve into specialized topics such as computer vision and learn to implement and assess various machine learning models. This course combines theoretical insights with practical lab activities to equip you with essential skills in applied machine learning.
What's included
2 readings
Discover the foundational principles and practical applications of machine learning in the field of computer vision. This module covers essential concepts, including data preprocessing, dataset management, classification techniques, and model evaluation, providing a comprehensive introduction to applying machine learning to visual data.
What's included
5 videos2 readings3 assignments1 ungraded lab
Explore essential techniques in data feature analysis and model evaluation critical to effective machine learning applications. Learn to identify, preprocess, and integrate datasets from diverse sources like UCI KDD and Kaggle. Gain hands-on experience with the Weka framework for data preprocessing and classification, and understand evaluation metrics including Receiver Operating Characteristic curves. By the end of this module, you'll grasp the nuances of model overfitting and strategies to optimize model performance.
What's included
7 videos2 readings3 assignments1 ungraded lab
Master the essential techniques of data pre-processing to enhance machine learning model performance. This module covers the foundational aspects of data cleaning, various data formats, and processing methods. You'll delve into advanced topics like discretization, data transformation, and reduction techniques. By the end of this module, you'll be adept at engineering data features, applying feature selection, and refining datasets for optimal machine learning outcomes.
What's included
5 videos1 reading3 assignments1 ungraded lab
Delve into the core principles and mathematical foundations of supervised learning algorithms. This module covers essential techniques, including the Perceptron algorithm, Naive Bayes classifier, and Linear Regression methods. You'll gain practical experience implementing and visualizing these algorithms, and explore how classifier decision boundaries shift with parameter changes. Additionally, learn to apply text classification using real-world datasets for hands-on understanding of supervised learning applications.
What's included
6 videos2 readings3 assignments1 programming assignment
Instructor

Offered by
Recommended if you're interested in Machine Learning
Why people choose Coursera for their career




New to Machine Learning? Start here.

Open new doors with Coursera Plus
Unlimited access to 10,000+ world-class courses, hands-on projects, and job-ready certificate programs - all included in your subscription
Advance your career with an online degree
Earn a degree from world-class universities - 100% online
Join over 3,400 global companies that choose Coursera for Business
Upskill your employees to excel in the digital economy
Frequently asked questions
Access to lectures and assignments depends on your type of enrollment. If you take a course in audit mode, you will be able to see most course materials for free. To access graded assignments and to earn a Certificate, you will need to purchase the Certificate experience, during or after your audit. If you don't see the audit option:
The course may not offer an audit option. You can try a Free Trial instead, or apply for Financial Aid.
The course may offer 'Full Course, No Certificate' instead. This option lets you see all course materials, submit required assessments, and get a final grade. This also means that you will not be able to purchase a Certificate experience.
When you enroll in the course, you get access to all of the courses in the Specialization, and you earn a certificate when you complete the work. Your electronic Certificate will be added to your Accomplishments page - from there, you can print your Certificate or add it to your LinkedIn profile. If you only want to read and view the course content, you can audit the course for free.
If you subscribed, you get a 7-day free trial during which you can cancel at no penalty. After that, we don’t give refunds, but you can cancel your subscription at any time. See our full refund policy.