This course for practicing and aspiring data scientists and statisticians. It is the fourth of a four-course sequence introducing the fundamentals of Bayesian statistics. It builds on the course Bayesian Statistics: From Concept to Data Analysis, Techniques and Models, and Mixture models.

Give your career the gift of Coursera Plus with $160 off, billed annually. Save today.
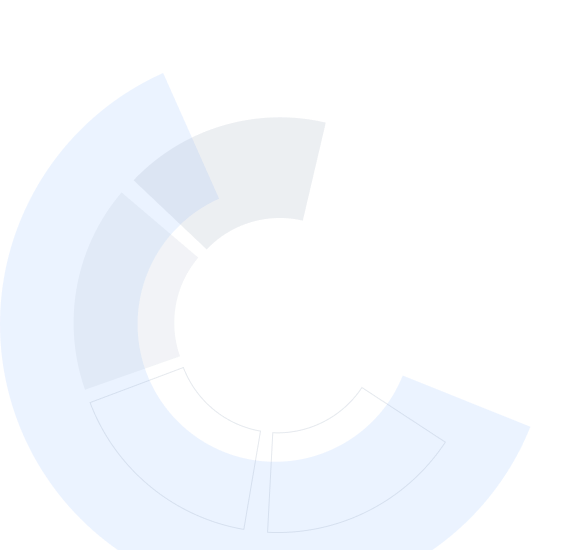
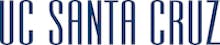
Bayesian Statistics: Time Series Analysis
This course is part of Bayesian Statistics Specialization

Instructor: Raquel Prado
4,659 already enrolled
Included with
(15 reviews)
Recommended experience
What you'll learn
Build models that describe temporal dependencies.
Use R for analysis and forecasting of times series.
Explain stationary time series processes.
Details to know

Add to your LinkedIn profile
10 assignments
See how employees at top companies are mastering in-demand skills
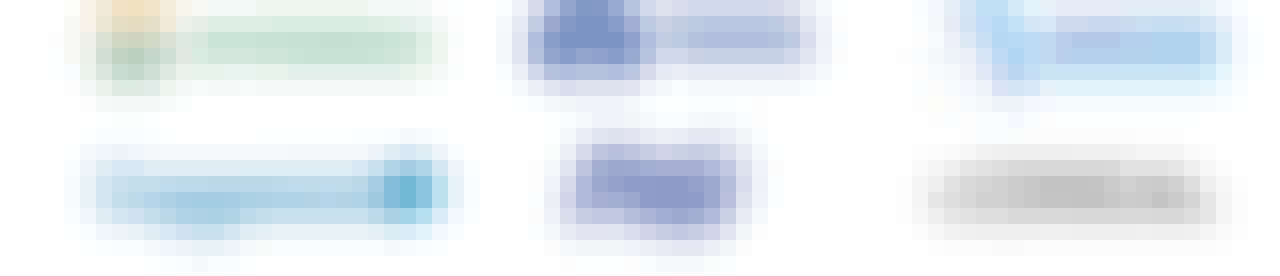
Build your subject-matter expertise
- Learn new concepts from industry experts
- Gain a foundational understanding of a subject or tool
- Develop job-relevant skills with hands-on projects
- Earn a shareable career certificate
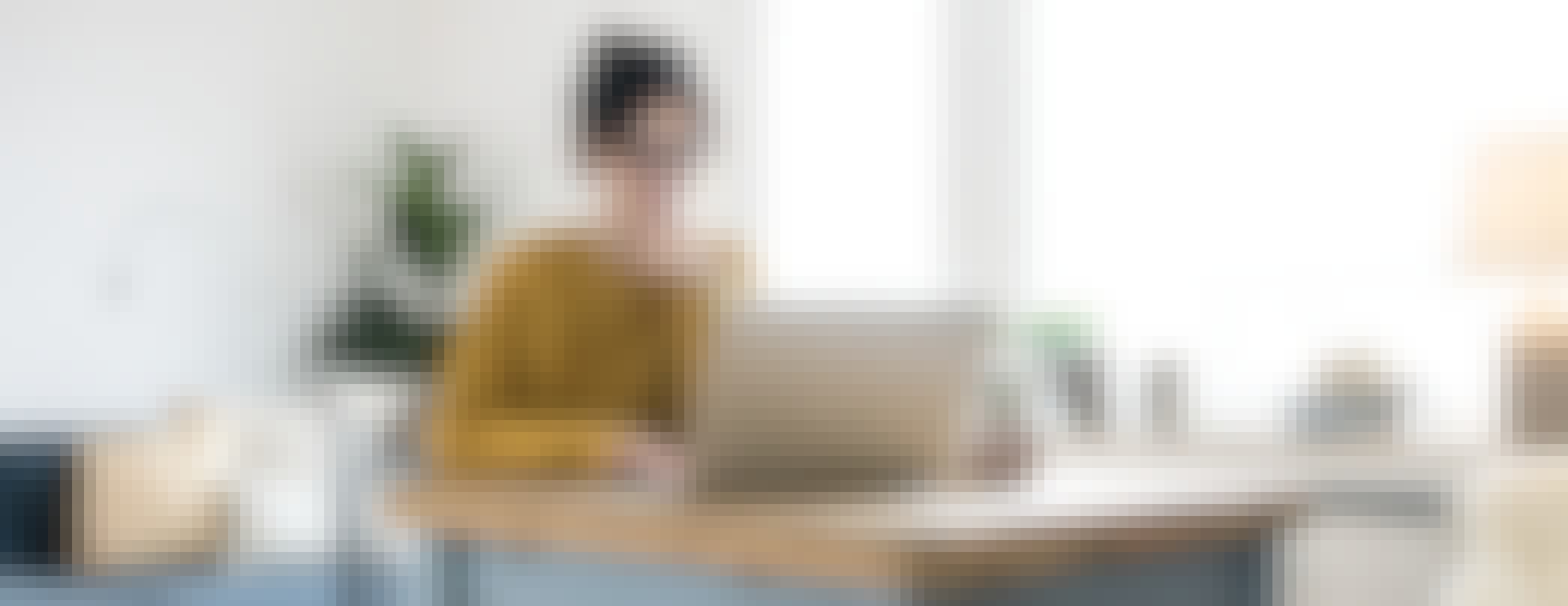
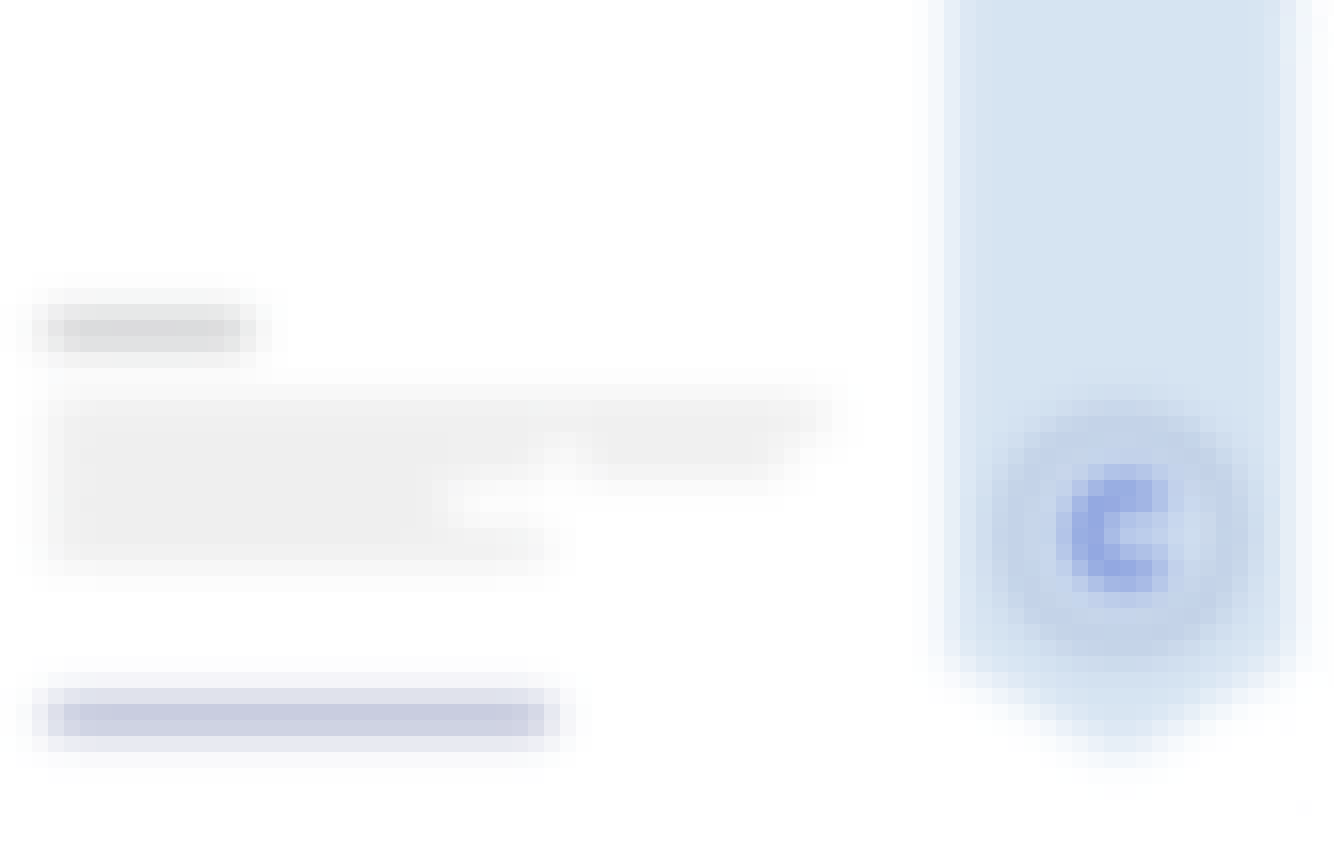
Earn a career certificate
Add this credential to your LinkedIn profile, resume, or CV
Share it on social media and in your performance review
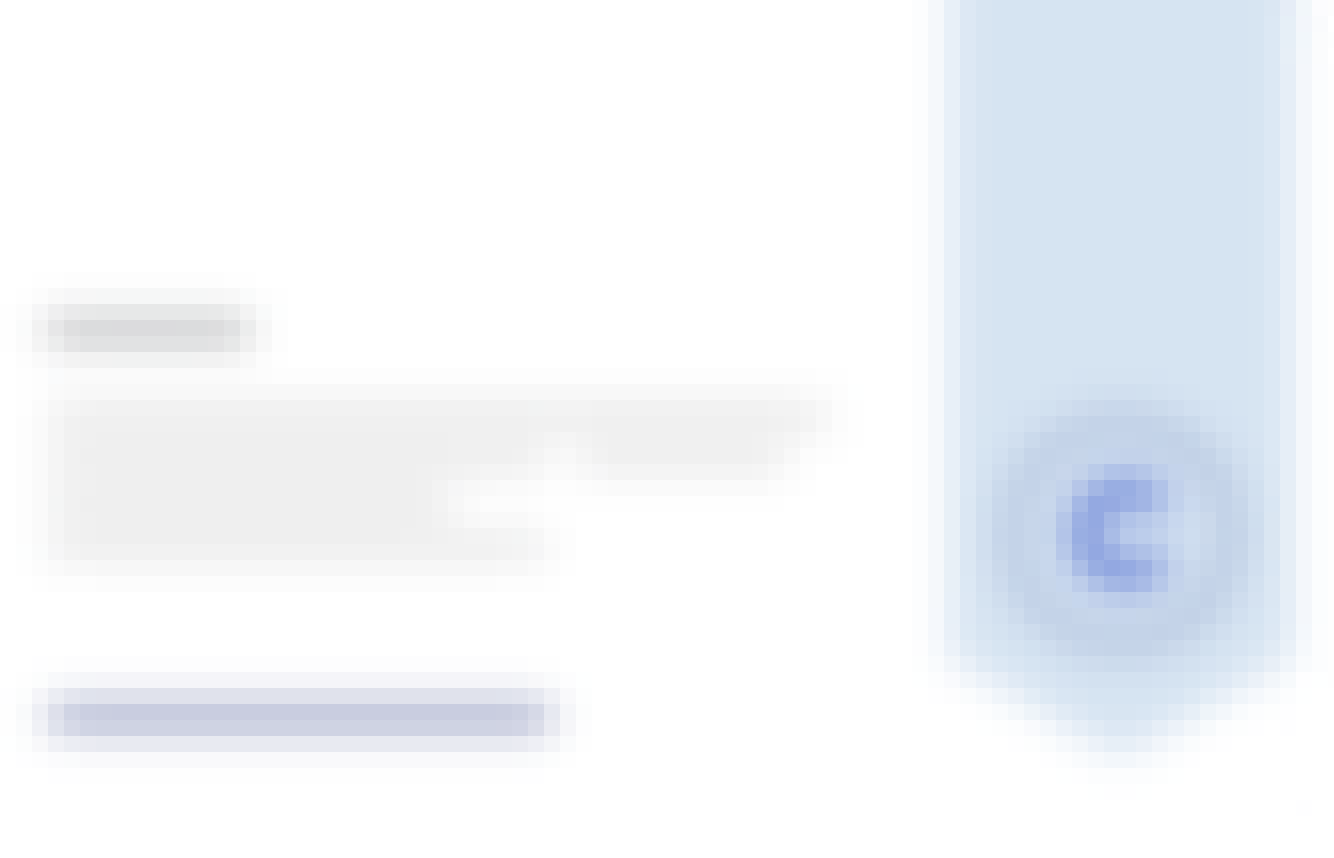
There are 5 modules in this course
This module defines stationary time series processes, the autocorrelation function and the autoregressive process of order one or AR(1). Parameter estimation via maximum likelihood and Bayesian inference in the AR(1) are also discussed.
What's included
9 videos12 readings4 assignments1 peer review
This module extends the concepts learned in Week 1 about the AR(1) process to the general case of the AR(p). Maximum likelihood estimation and Bayesian posterior inference in the AR(p) are discussed.
What's included
9 videos8 readings2 assignments1 peer review
Normal Dynamic Linear Models (NDLMs) are defined and illustrated in this module using several examples. Model building based on the forecast function via the superposition principle is explained. Methods for Bayesian filtering, smoothing and forecasting for NDLMs in the case of known observational variances and known system covariance matrices are discussed and illustrated.
What's included
10 videos7 readings2 assignments1 peer review
What's included
7 videos4 readings2 assignments1 peer review
In this final project you will use normal dynamic linear models to analyze a time series dataset downloaded from Google trend.
What's included
1 peer review
Instructor

Offered by
Recommended if you're interested in Probability and Statistics
Stanford University
Johns Hopkins University
Coursera Project Network
Queen Mary University of London
Why people choose Coursera for their career




Learner reviews
Showing 3 of 15
15 reviews
- 5 stars
73.33%
- 4 stars
0%
- 3 stars
20%
- 2 stars
0%
- 1 star
6.66%
Reviewed on Feb 5, 2024
New to Probability and Statistics? Start here.

Open new doors with Coursera Plus
Unlimited access to 7,000+ world-class courses, hands-on projects, and job-ready certificate programs - all included in your subscription
Advance your career with an online degree
Earn a degree from world-class universities - 100% online
Join over 3,400 global companies that choose Coursera for Business
Upskill your employees to excel in the digital economy
Frequently asked questions
Access to lectures and assignments depends on your type of enrollment. If you take a course in audit mode, you will be able to see most course materials for free. To access graded assignments and to earn a Certificate, you will need to purchase the Certificate experience, during or after your audit. If you don't see the audit option:
The course may not offer an audit option. You can try a Free Trial instead, or apply for Financial Aid.
The course may offer 'Full Course, No Certificate' instead. This option lets you see all course materials, submit required assessments, and get a final grade. This also means that you will not be able to purchase a Certificate experience.
When you enroll in the course, you get access to all of the courses in the Specialization, and you earn a certificate when you complete the work. Your electronic Certificate will be added to your Accomplishments page - from there, you can print your Certificate or add it to your LinkedIn profile. If you only want to read and view the course content, you can audit the course for free.
If you subscribed, you get a 7-day free trial during which you can cancel at no penalty. After that, we don’t give refunds, but you can cancel your subscription at any time. See our full refund policy.