This course introduces the Bayesian approach to statistics, starting with the concept of probability and moving to the analysis of data. We will learn about the philosophy of the Bayesian approach as well as how to implement it for common types of data. We will compare the Bayesian approach to the more commonly-taught Frequentist approach, and see some of the benefits of the Bayesian approach. In particular, the Bayesian approach allows for better accounting of uncertainty, results that have more intuitive and interpretable meaning, and more explicit statements of assumptions. This course combines lecture videos, computer demonstrations, readings, exercises, and discussion boards to create an active learning experience. For computing, you have the choice of using Microsoft Excel or the open-source, freely available statistical package R, with equivalent content for both options. The lectures provide some of the basic mathematical development as well as explanations of philosophy and interpretation. Completion of this course will give you an understanding of the concepts of the Bayesian approach, understanding the key differences between Bayesian and Frequentist approaches, and the ability to do basic data analyses.

New year. Big goals. Bigger savings. Unlock a year of unlimited access to learning with Coursera Plus for $199. Save now.
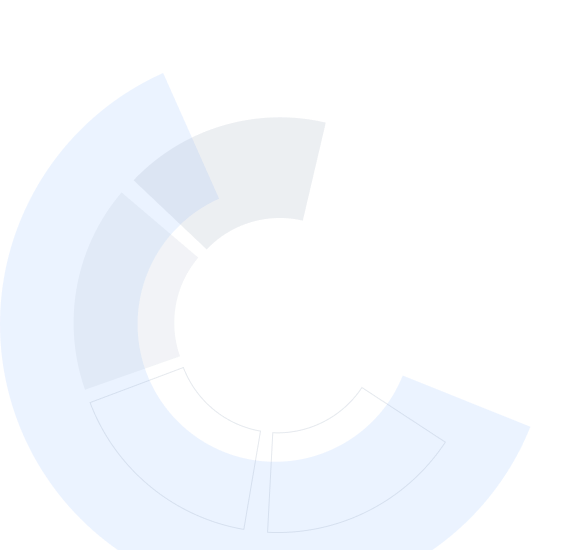
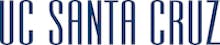
Bayesian Statistics: From Concept to Data Analysis
This course is part of Bayesian Statistics Specialization

Instructor: Herbert Lee
151,869 already enrolled
Included with
(3,176 reviews)
What you'll learn
Describe & apply the Bayesian approach to statistics.
Explain the key differences between Bayesian and Frequentist approaches.
Master the basics of the R computing environment.
Skills you'll gain
Details to know

Add to your LinkedIn profile
18 assignments
See how employees at top companies are mastering in-demand skills
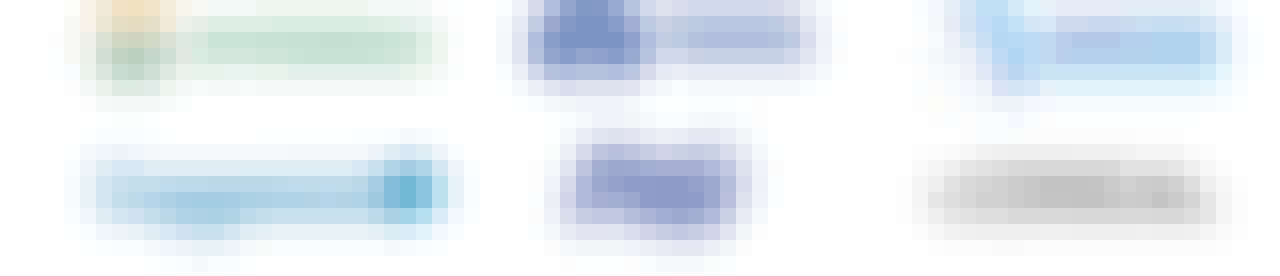
Build your subject-matter expertise
- Learn new concepts from industry experts
- Gain a foundational understanding of a subject or tool
- Develop job-relevant skills with hands-on projects
- Earn a shareable career certificate
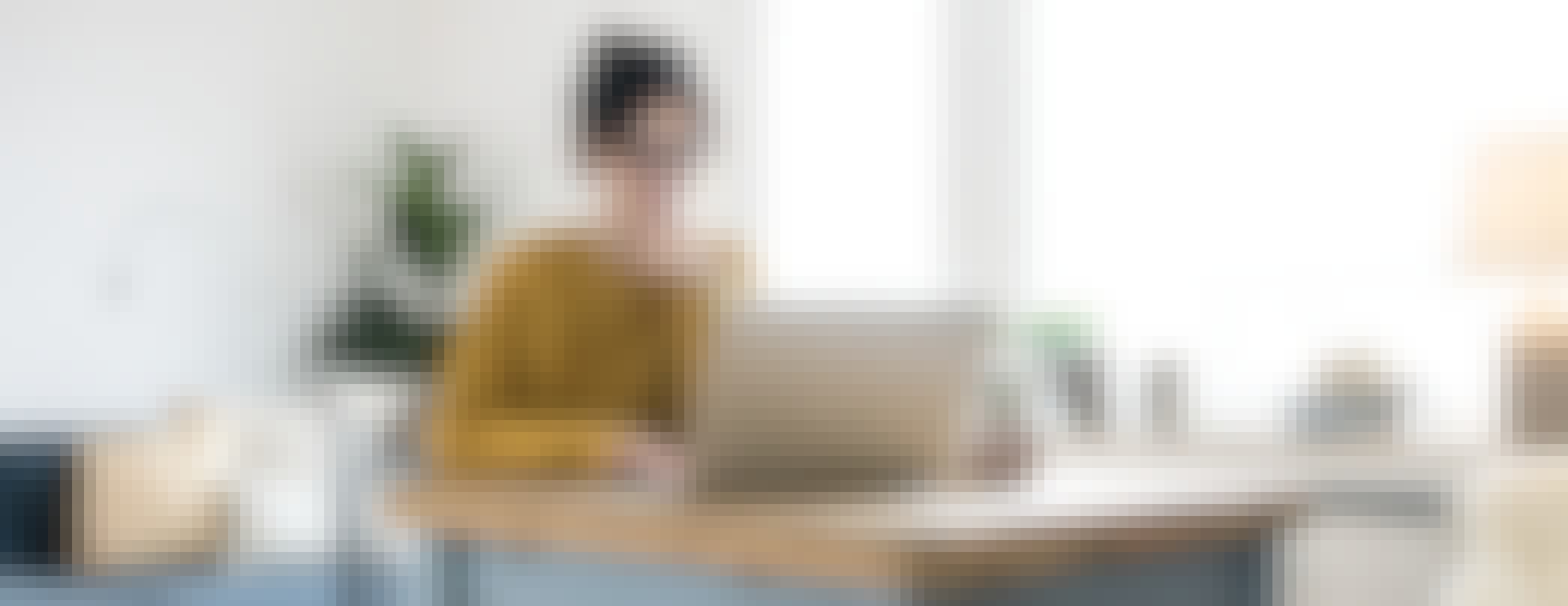
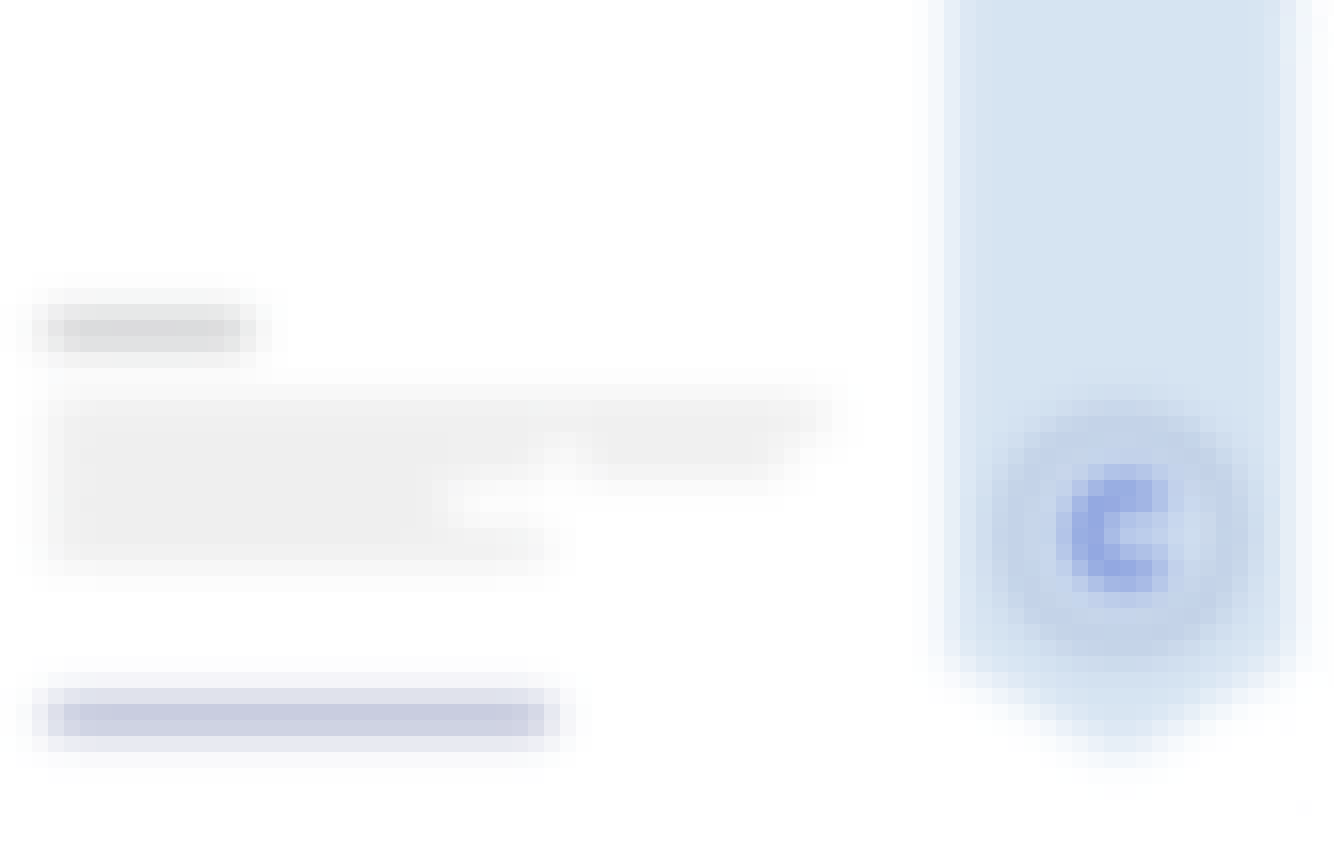
Earn a career certificate
Add this credential to your LinkedIn profile, resume, or CV
Share it on social media and in your performance review
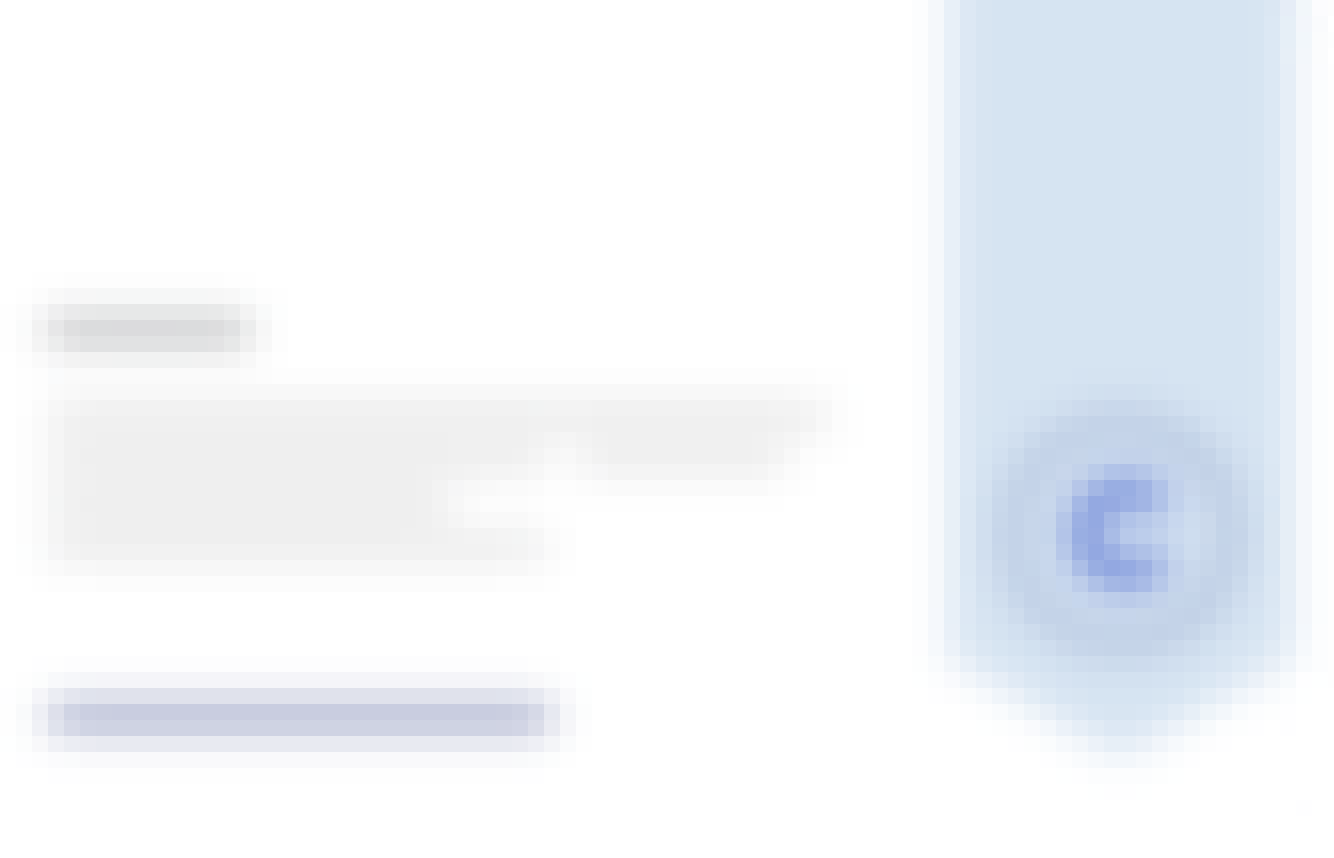
There are 4 modules in this course
In this module, we review the basics of probability and Bayes’ theorem. In Lesson 1, we introduce the different paradigms or definitions of probability and discuss why probability provides a coherent framework for dealing with uncertainty. In Lesson 2, we review the rules of conditional probability and introduce Bayes’ theorem. Lesson 3 reviews common probability distributions for discrete and continuous random variables.
What's included
8 videos4 readings5 assignments1 discussion prompt
This module introduces concepts of statistical inference from both frequentist and Bayesian perspectives. Lesson 4 takes the frequentist view, demonstrating maximum likelihood estimation and confidence intervals for binomial data. Lesson 5 introduces the fundamentals of Bayesian inference. Beginning with a binomial likelihood and prior probabilities for simple hypotheses, you will learn how to use Bayes’ theorem to update the prior with data to obtain posterior probabilities. This framework is extended with the continuous version of Bayes theorem to estimate continuous model parameters, and calculate posterior probabilities and credible intervals.
What's included
11 videos5 readings4 assignments1 discussion prompt
In this module, you will learn methods for selecting prior distributions and building models for discrete data. Lesson 6 introduces prior selection and predictive distributions as a means of evaluating priors. Lesson 7 demonstrates Bayesian analysis of Bernoulli data and introduces the computationally convenient concept of conjugate priors. Lesson 8 builds a conjugate model for Poisson data and discusses strategies for selection of prior hyperparameters.
What's included
9 videos2 readings4 assignments1 discussion prompt
This module covers conjugate and objective Bayesian analysis for continuous data. Lesson 9 presents the conjugate model for exponentially distributed data. Lesson 10 discusses models for normally distributed data, which play a central role in statistics. In Lesson 11, we return to prior selection and discuss ‘objective’ or ‘non-informative’ priors. Lesson 12 presents Bayesian linear regression with non-informative priors, which yield results comparable to those of classical regression.
What's included
9 videos5 readings5 assignments1 discussion prompt
Instructor

Offered by
Recommended if you're interested in Probability and Statistics
University of Colorado Boulder
Coursera Project Network
IIMA - IIM Ahmedabad
Why people choose Coursera for their career




Learner reviews
3,176 reviews
- 5 stars
67.30%
- 4 stars
25.17%
- 3 stars
5.16%
- 2 stars
1.29%
- 1 star
1.06%
Showing 3 of 3176
Reviewed on Jun 26, 2018
Great course. The content moves at a nice pace and the videos are really good to follow. The Quizzes are also set at a good level. You can't pass this course unless you have understood the material.
Reviewed on Jul 20, 2020
Prof. Lee's approach is simple and intuitive. There are exercises throughout the videos, which help you make sure you and the professor are on the same track. I would certainly recommend it!
Reviewed on Oct 16, 2024
The concepts could have been made much more easier, simpler and more examples could have been inculcated. But overall, a great course to gain in-depth insights for a starter on Bayesian Statistics
New to Probability and Statistics? Start here.

Open new doors with Coursera Plus
Unlimited access to 10,000+ world-class courses, hands-on projects, and job-ready certificate programs - all included in your subscription
Advance your career with an online degree
Earn a degree from world-class universities - 100% online
Join over 3,400 global companies that choose Coursera for Business
Upskill your employees to excel in the digital economy
Frequently asked questions
You should have exposure to the concepts from a basic statistics class (for example, probability, the Central Limit Theorem, confidence intervals, linear regression) and calculus (integration and differentiation), but it is not expected that you remember how to do all of these items. The course will provide some overview of the statistical concepts, which should be enough to remind you of the necessary details if you've at least seen the concepts previously. On the calculus side, the lectures will include some use of calculus, so it is important that you understand the concept of an integral as finding the area under a curve, or differentiating to find a maximum, but you will not be required to do any integration or differentiation yourself.
Data analysis is done using computer software. This course provides the option of Excel or R. Equivalent content is provided for both options. A very brief introduction to R is provided for people who have never used it before, but this is not meant to be a course on R. Learners using Excel are expected to already have basic familiarity of Excel.
Access to lectures and assignments depends on your type of enrollment. If you take a course in audit mode, you will be able to see most course materials for free. To access graded assignments and to earn a Certificate, you will need to purchase the Certificate experience, during or after your audit. If you don't see the audit option:
The course may not offer an audit option. You can try a Free Trial instead, or apply for Financial Aid.
The course may offer 'Full Course, No Certificate' instead. This option lets you see all course materials, submit required assessments, and get a final grade. This also means that you will not be able to purchase a Certificate experience.