This course covers linear algebra, probability, and optimization. It begins with systems of equations, matrix operations, vector spaces, and eigenvalues. Advanced topics include Cholesky and singular value decomposition. Probability modules address Bayes' theorem, Gaussian distribution, and inference techniques. The course concludes with model selection methods and an introduction to optimization.

New year. Big goals. Bigger savings. Unlock a year of unlimited access to learning with Coursera Plus for $199. Save now.
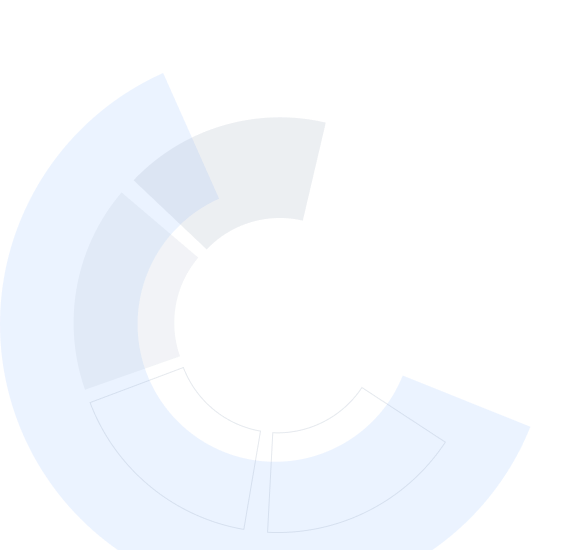
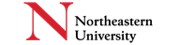
Details to know

Add to your LinkedIn profile
January 2025
6 assignments
See how employees at top companies are mastering in-demand skills
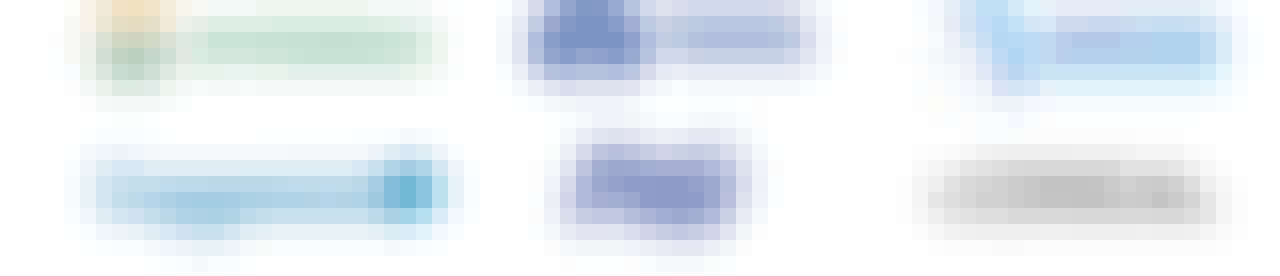
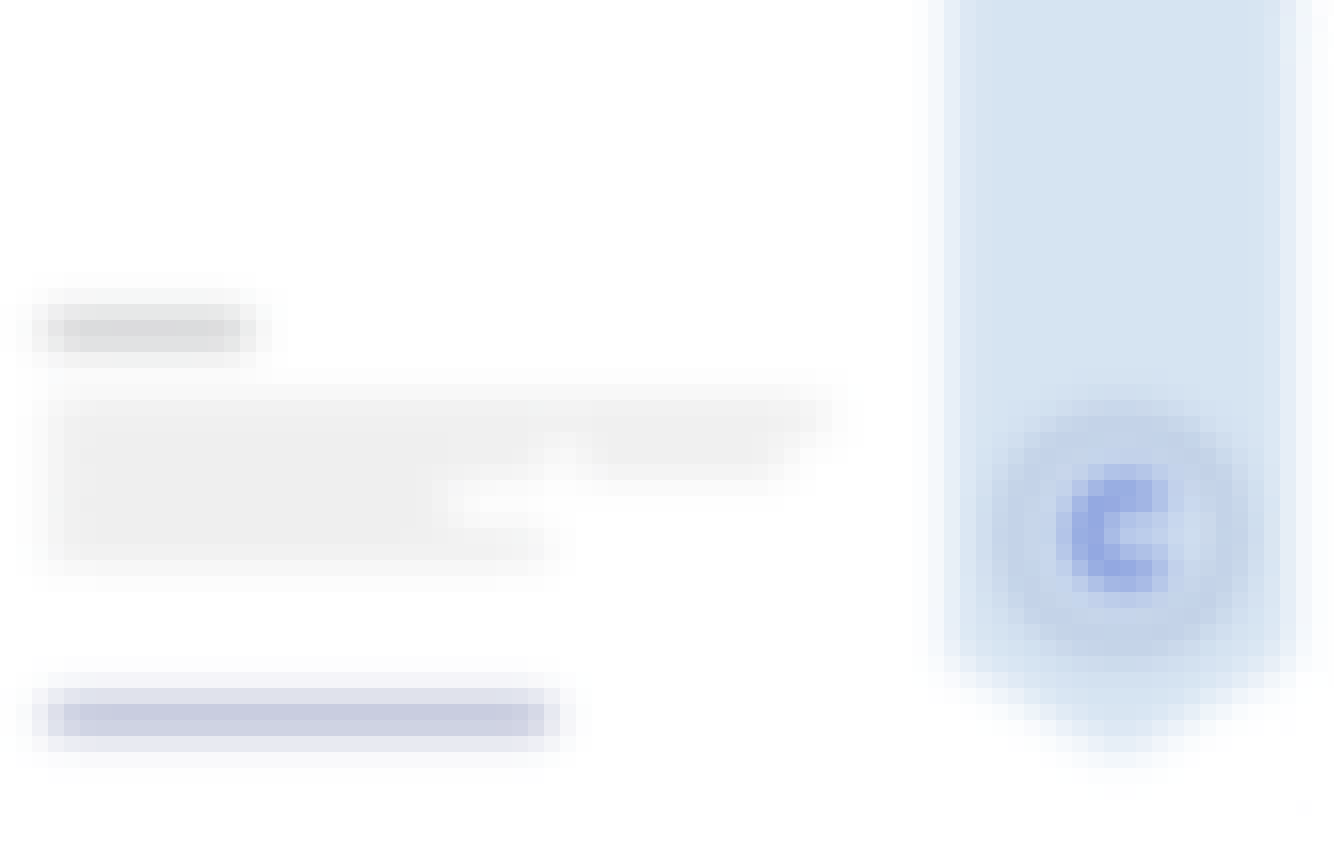
Earn a career certificate
Add this credential to your LinkedIn profile, resume, or CV
Share it on social media and in your performance review
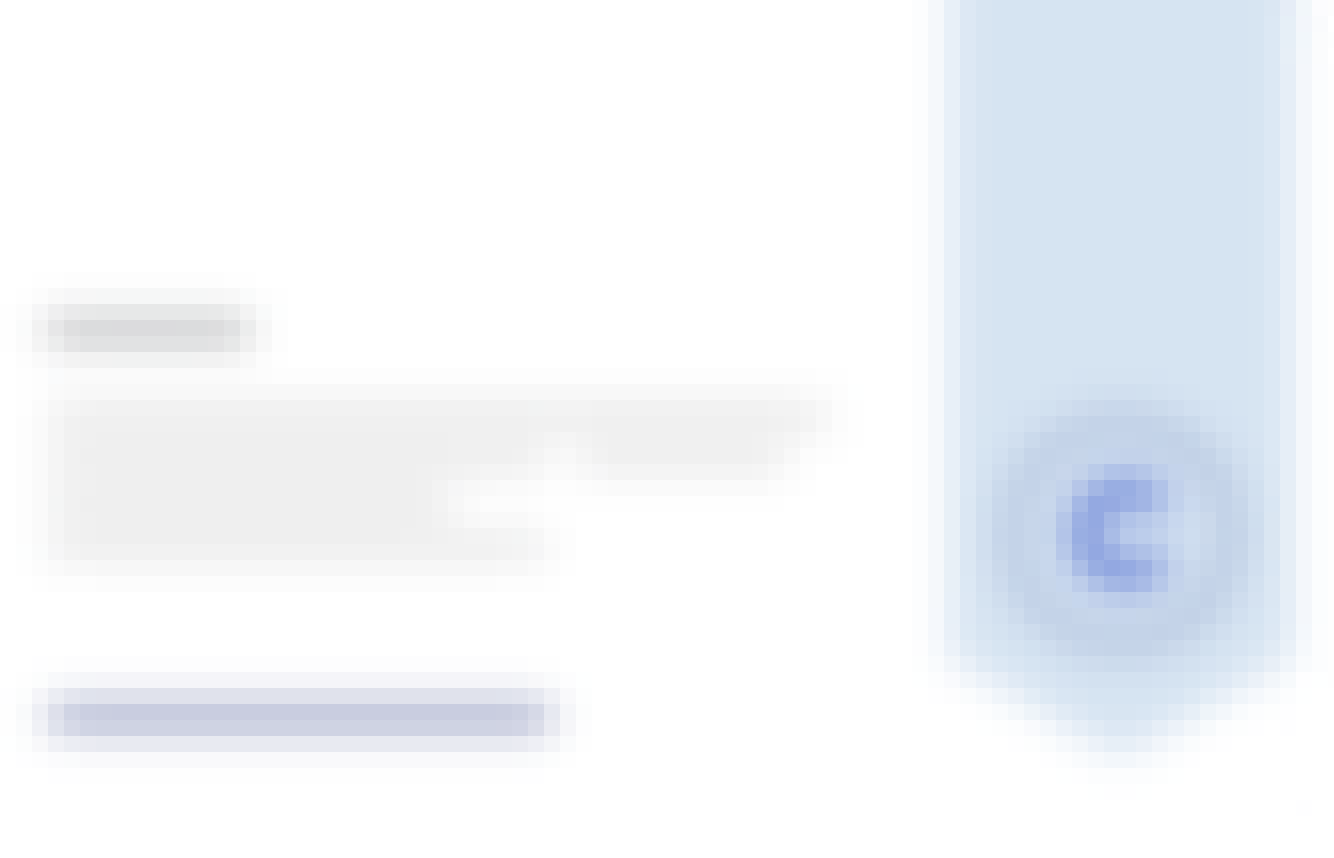
There are 4 modules in this course
This module provides a foundational understanding of linear algebra concepts essential for statistical learning and algorithms. You will explore the principles of linear systems, matrix operations, vector spaces, orthogonality, and projections. These topics will lay the groundwork for understanding more advanced machine learning and statistical modeling techniques.
What's included
4 videos20 readings3 assignments1 app item1 discussion prompt
This module covers essential linear algebra concepts, focusing on linear mappings, eigenvectors, eigenvalues, Cholesky decomposition, and singular value decomposition. You'll learn to apply linear mappings, interpret eigenvectors and eigenvalues, and explore the Cholesky decomposition for symmetric, positive definite matrices. Additionally, you'll delve into singular value decomposition and its applications. The lessons include linear independence, linear mappings, eigenvalues and eigenvectors, Cholesky decomposition, and singular value decomposition, providing a comprehensive understanding of these critical topics.
What's included
2 videos11 readings1 assignment1 app item
This module focuses on essential probability concepts and their applications in machine learning. You will explore the sum rule, product rule, and Bayes' theorem, understanding how these principles are applied to solve complex problems. Additionally, you'll learn to apply Bayesian inference to estimate hidden variables from observed data, enhancing your ability to make informed predictions and decisions in machine learning contexts. These topics will provide a solid foundation for understanding and implementing probabilistic models in various machine learning scenarios.
What's included
11 readings1 assignment
This module covers key techniques for enhancing machine learning models. You will learn to minimize the error or loss of a model through various optimization methods. Additionally, you'll explore different cross-validation techniques to assess model performance and generalizability. By examining various optimization techniques, you'll improve model accuracy and efficiency. These topics will equip you with the skills to fine-tune and validate your machine learning models effectively.
What's included
15 readings1 assignment
Instructor

Offered by
Recommended if you're interested in Mechanical Engineering
Arizona State University
Alberta Machine Intelligence Institute
Duke University
National Taiwan University
Why people choose Coursera for their career





Open new doors with Coursera Plus
Unlimited access to 10,000+ world-class courses, hands-on projects, and job-ready certificate programs - all included in your subscription
Advance your career with an online degree
Earn a degree from world-class universities - 100% online
Join over 3,400 global companies that choose Coursera for Business
Upskill your employees to excel in the digital economy
Frequently asked questions
Access to lectures and assignments depends on your type of enrollment. If you take a course in audit mode, you will be able to see most course materials for free. To access graded assignments and to earn a Certificate, you will need to purchase the Certificate experience, during or after your audit. If you don't see the audit option:
The course may not offer an audit option. You can try a Free Trial instead, or apply for Financial Aid.
The course may offer 'Full Course, No Certificate' instead. This option lets you see all course materials, submit required assessments, and get a final grade. This also means that you will not be able to purchase a Certificate experience.
When you purchase a Certificate you get access to all course materials, including graded assignments. Upon completing the course, your electronic Certificate will be added to your Accomplishments page - from there, you can print your Certificate or add it to your LinkedIn profile. If you only want to read and view the course content, you can audit the course for free.
You will be eligible for a full refund until two weeks after your payment date, or (for courses that have just launched) until two weeks after the first session of the course begins, whichever is later. You cannot receive a refund once you’ve earned a Course Certificate, even if you complete the course within the two-week refund period. See our full refund policy.