In this first course of the AI Product Management Specialization offered by Duke University's Pratt School of Engineering, you will build a foundational understanding of what machine learning is, how it works and when and why it is applied. To successfully manage an AI team or product and work collaboratively with data scientists, software engineers, and customers you need to understand the basics of machine learning technology. This course provides a non-coding introduction to machine learning, with focus on the process of developing models, ML model evaluation and interpretation, and the intuition behind common ML and deep learning algorithms. The course will conclude with a hands-on project in which you will have a chance to train and optimize a machine learning model on a simple real-world problem.

Give your career the gift of Coursera Plus with $160 off, billed annually. Save today.
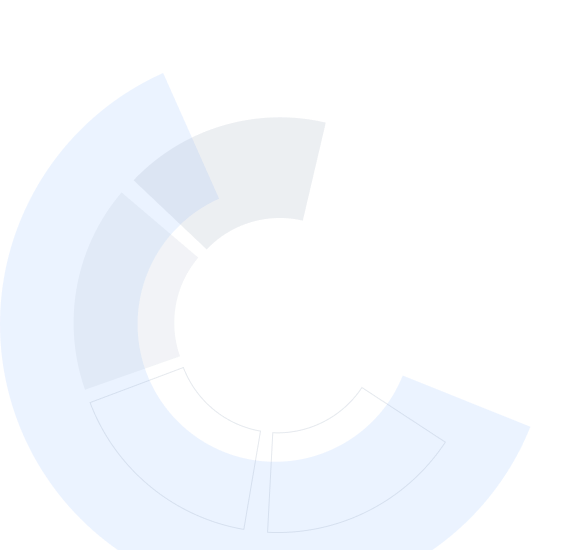
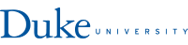
Machine Learning Foundations for Product Managers
This course is part of AI Product Management Specialization

Instructor: Jon Reifschneider
46,041 already enrolled
Included with
(472 reviews)
Recommended experience
Details to know

Add to your LinkedIn profile
6 assignments
See how employees at top companies are mastering in-demand skills
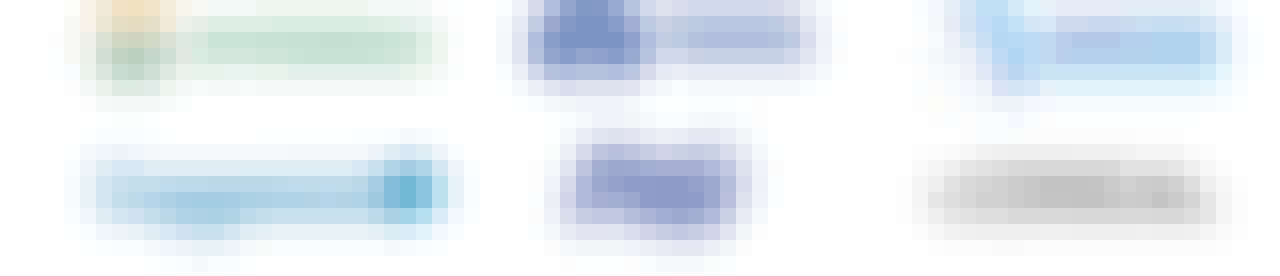
Build your subject-matter expertise
- Learn new concepts from industry experts
- Gain a foundational understanding of a subject or tool
- Develop job-relevant skills with hands-on projects
- Earn a shareable career certificate
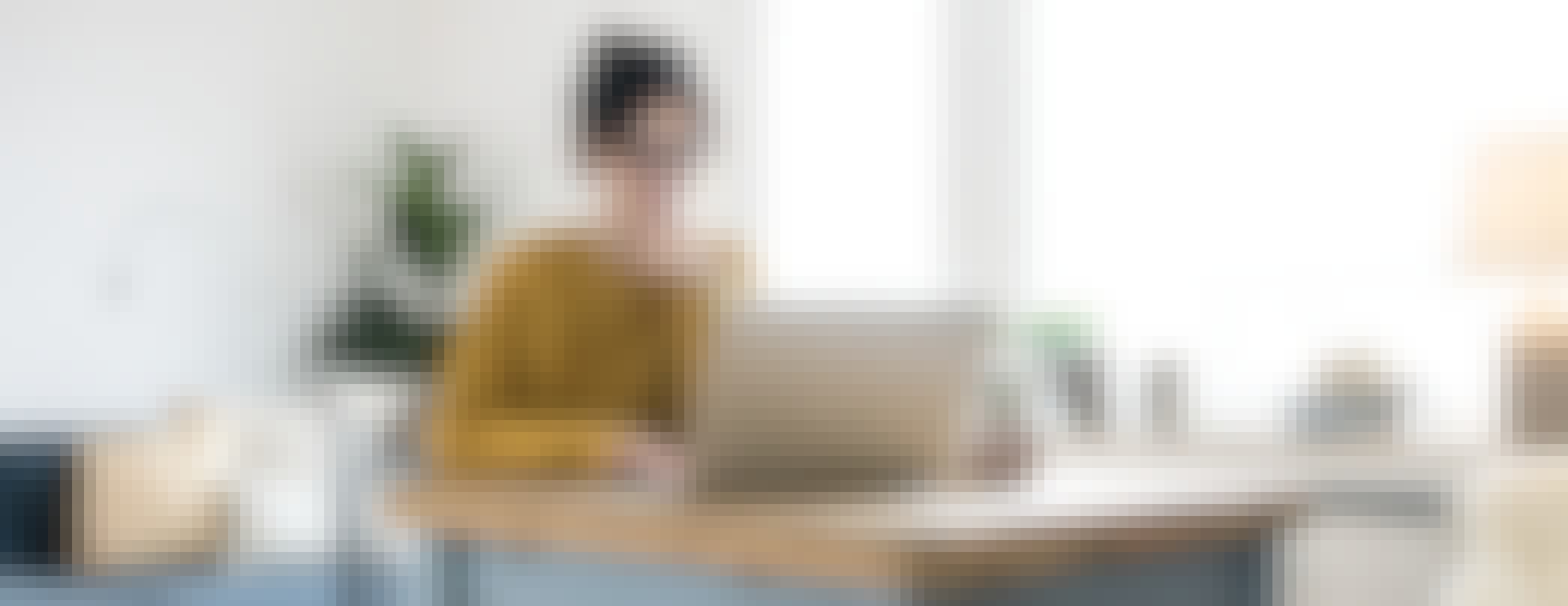
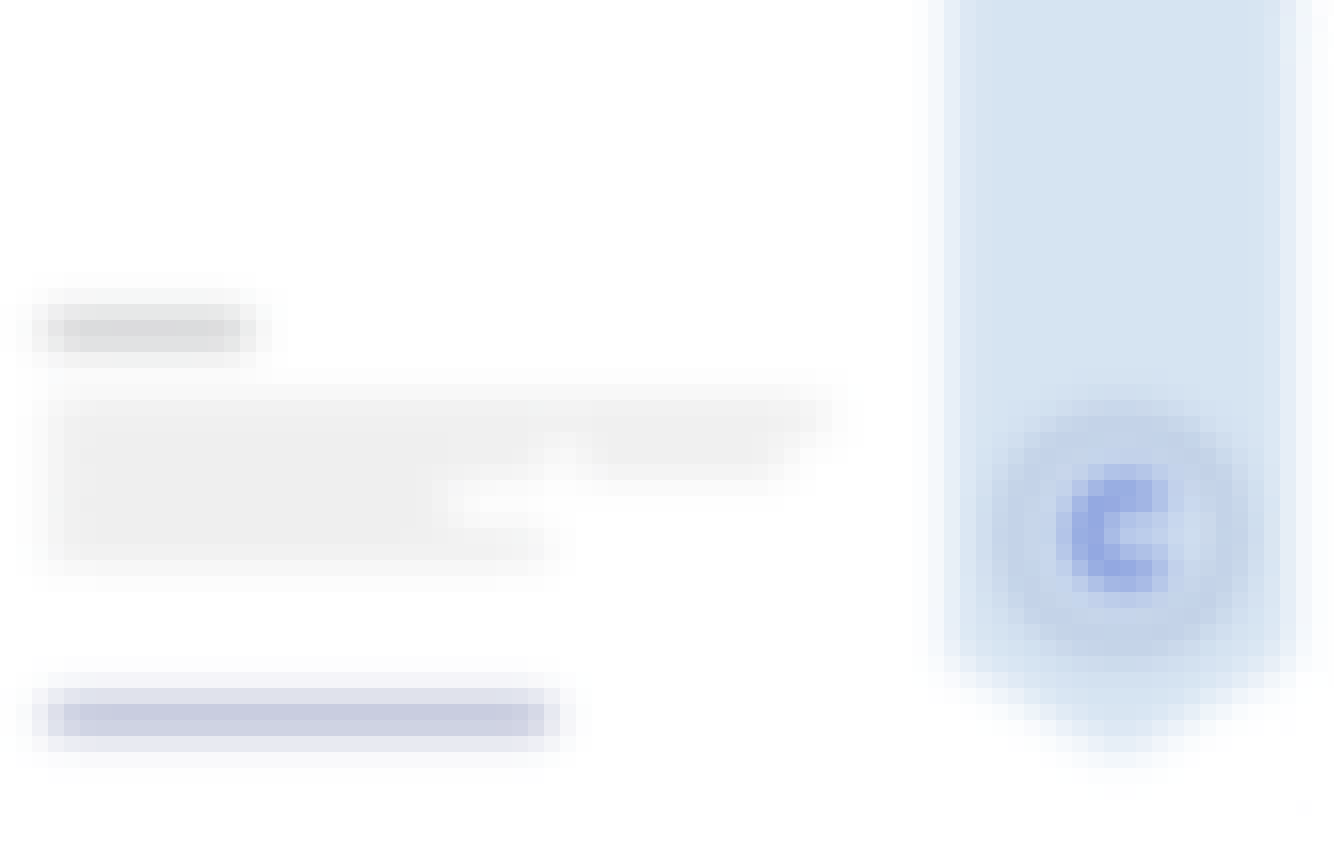
Earn a career certificate
Add this credential to your LinkedIn profile, resume, or CV
Share it on social media and in your performance review
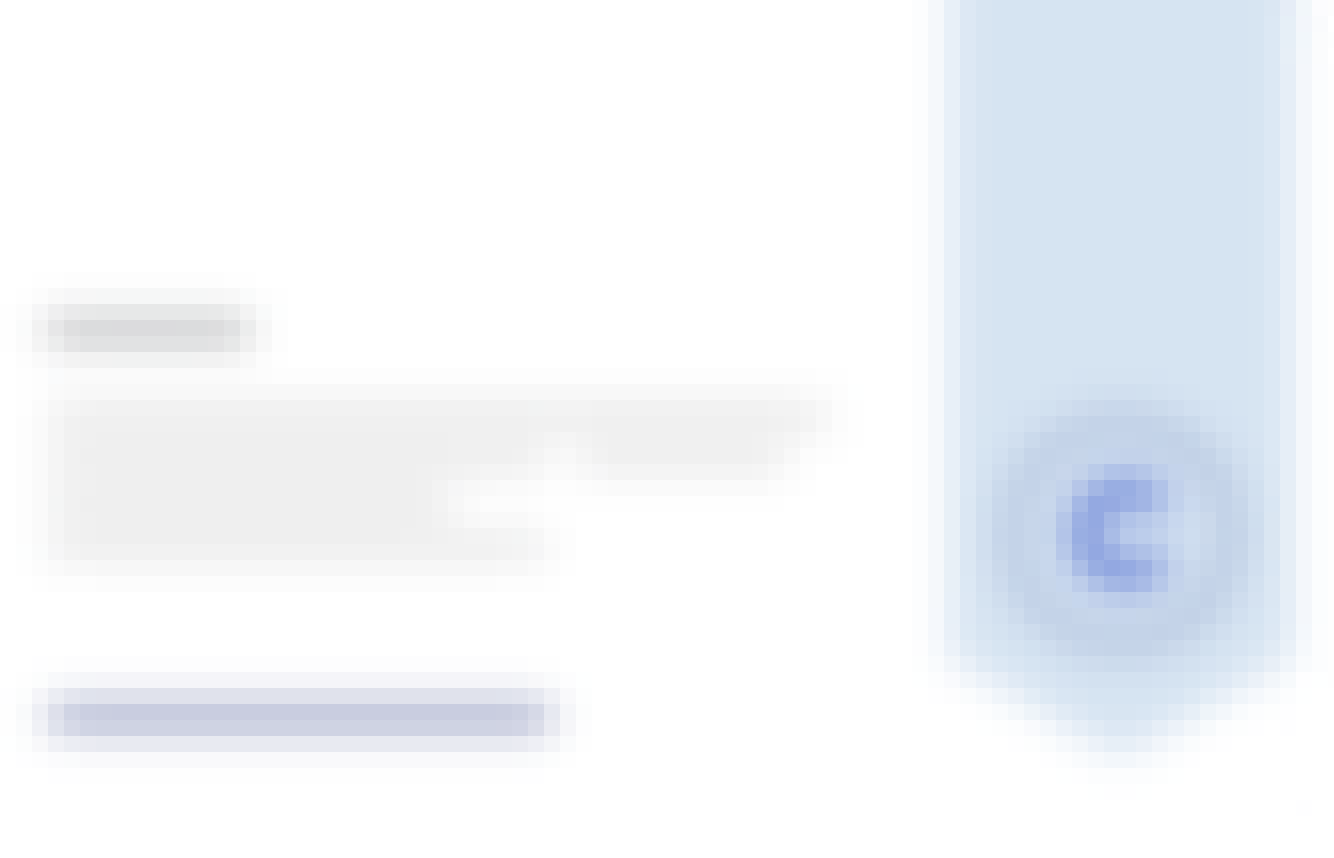
There are 6 modules in this course
In this module we will be introduced to what machine learning is and does. We will build the necessary vocabulary for working with data and models and develop an understanding of the different types of machine learning. We will conclude with a critical discussion of what machine learning can do well and cannot (or should not) do.
What's included
10 videos3 readings1 assignment
In this module we will discuss the key steps in the process of building machine learning models. We will learn about the sources of model complexity and how complexity impacts a model's performance. We will wrap up with a discussion of strategies for comparing different models to select the optimal model for production.
What's included
8 videos1 reading1 assignment
In this module we will learn how to define appropriate outcome and output metrics for AI projects. We will then discuss key metrics for evaluating regression and classification models and how to select one for use. We will wrap up with a discussion of common sources of error in machine learning projects and how to troubleshoot poor performance.
What's included
8 videos1 reading1 assignment1 discussion prompt
In this module we will explore the use of linear models for regression and classification. We will begin with introducing linear regression and continue with a discussion on how to make linear regression work better through regularization. We will then switch to classification and introduce the logistic regression model for both binary and multi-class classification problems.
What's included
6 videos1 reading1 assignment
We will begin this model with a discussion of tree models and their value in modeling compex non-linear problems. We will then introduce the method of creating ensemble models and their benefits. We will wrap this module up by switching gears to unsupervised learning and discussing clustering and the popular K-Means clustering approach.
What's included
7 videos1 reading1 assignment
Our final module in this course will focus on a hot area of machine learning called deep learning, or the use of multi-layer neural networks. We will develop an understanding of the intuition and key mathematical principles behind how neural networks work. We will then discuss common applications of deep learning in computer vision and natural language processing. We will wrap up the course with our course project, where you will have an opportunity to apply the modeling process and best practices you have learned to create your own machine learning model.
What's included
9 videos2 readings1 assignment1 peer review
Instructor

Offered by
Recommended if you're interested in Machine Learning
Illinois Tech
Amazon Web Services
DeepLearning.AI
Why people choose Coursera for their career




Learner reviews
Showing 3 of 472
472 reviews
- 5 stars
77.58%
- 4 stars
15.22%
- 3 stars
2.74%
- 2 stars
1.90%
- 1 star
2.53%
New to Machine Learning? Start here.

Open new doors with Coursera Plus
Unlimited access to 7,000+ world-class courses, hands-on projects, and job-ready certificate programs - all included in your subscription
Advance your career with an online degree
Earn a degree from world-class universities - 100% online
Join over 3,400 global companies that choose Coursera for Business
Upskill your employees to excel in the digital economy
Frequently asked questions
Access to lectures and assignments depends on your type of enrollment. If you take a course in audit mode, you will be able to see most course materials for free. To access graded assignments and to earn a Certificate, you will need to purchase the Certificate experience, during or after your audit. If you don't see the audit option:
The course may not offer an audit option. You can try a Free Trial instead, or apply for Financial Aid.
The course may offer 'Full Course, No Certificate' instead. This option lets you see all course materials, submit required assessments, and get a final grade. This also means that you will not be able to purchase a Certificate experience.
When you enroll in the course, you get access to all of the courses in the Specialization, and you earn a certificate when you complete the work. Your electronic Certificate will be added to your Accomplishments page - from there, you can print your Certificate or add it to your LinkedIn profile. If you only want to read and view the course content, you can audit the course for free.
If you subscribed, you get a 7-day free trial during which you can cancel at no penalty. After that, we don’t give refunds, but you can cancel your subscription at any time. See our full refund policy.