This course teaches you to use Python, AI, machine learning, and deep learning to build recommender systems, from simple engines to hybrid ensemble recommenders. You'll start with an introduction to recommender systems and Python, evaluate systems, and explore the recommender engine framework.

Cultivate your career with expert-led programs, job-ready certificates, and 10,000 ways to grow. All for $25/month, billed annually. Save now
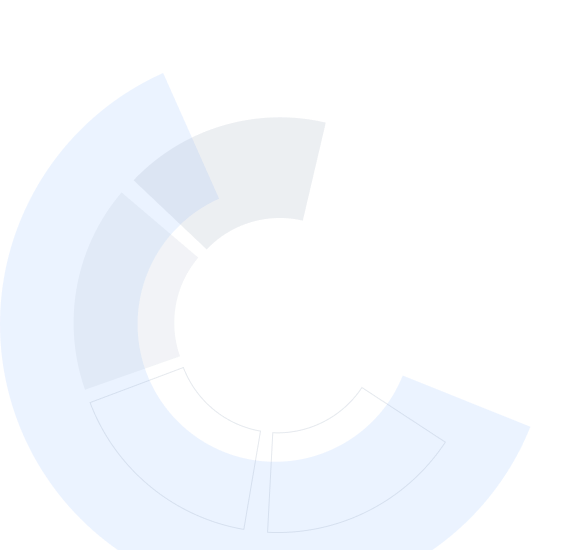
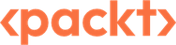
Building Recommender Systems with Machine Learning and AI

Instructor: Packt - Course Instructors
Included with
Recommended experience
Recommended experience
What you'll learn
Analyze and evaluate recommendation algorithms using Python.
Create session-based recommendations using recurrent neural networks.
Implement large-scale recommendation computations with Apache Spark.
Details to know

Add to your LinkedIn profile
6 assignments
See how employees at top companies are mastering in-demand skills
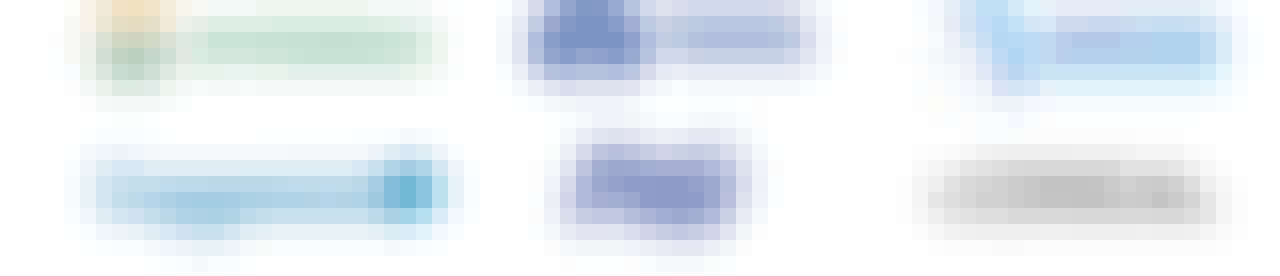
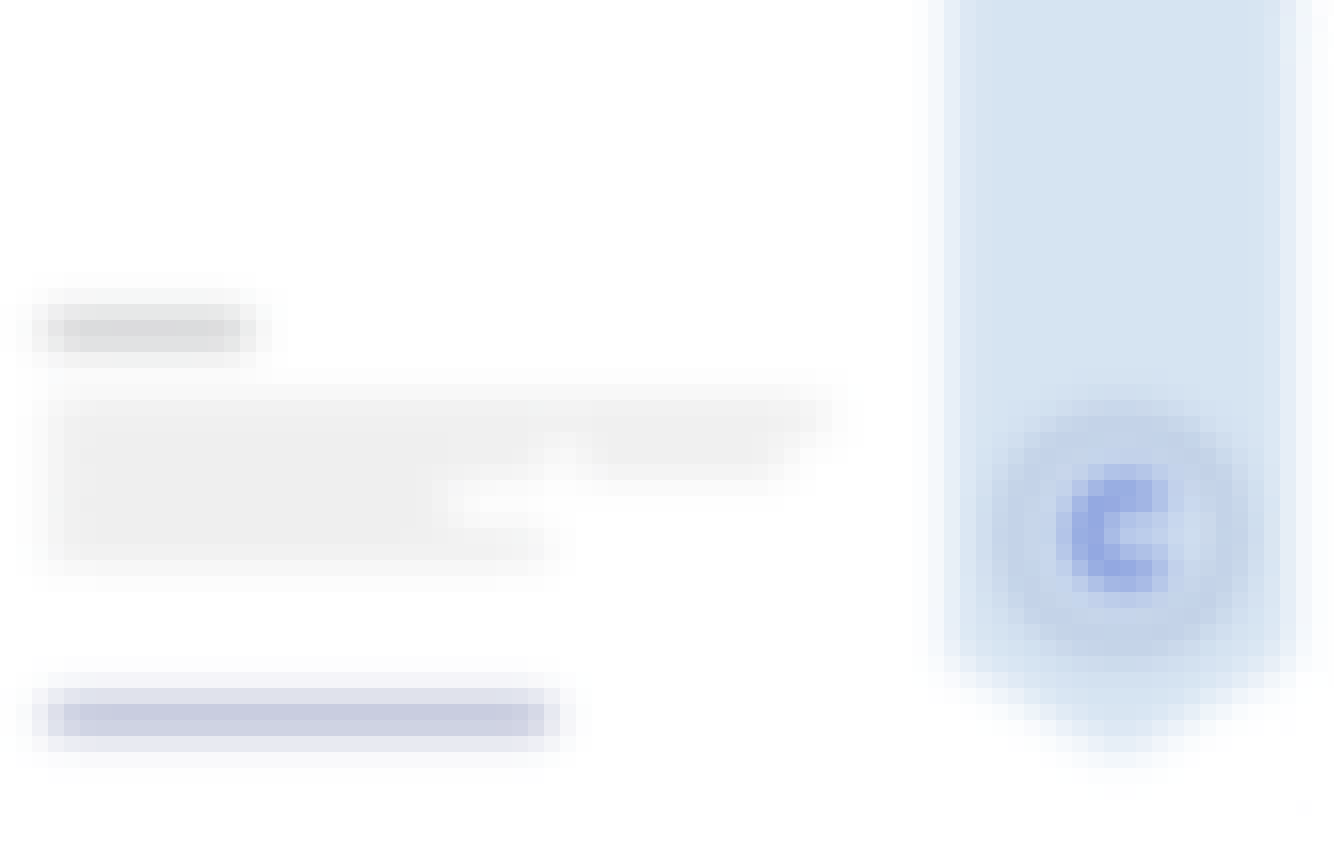
Earn a career certificate
Add this credential to your LinkedIn profile, resume, or CV
Share it on social media and in your performance review
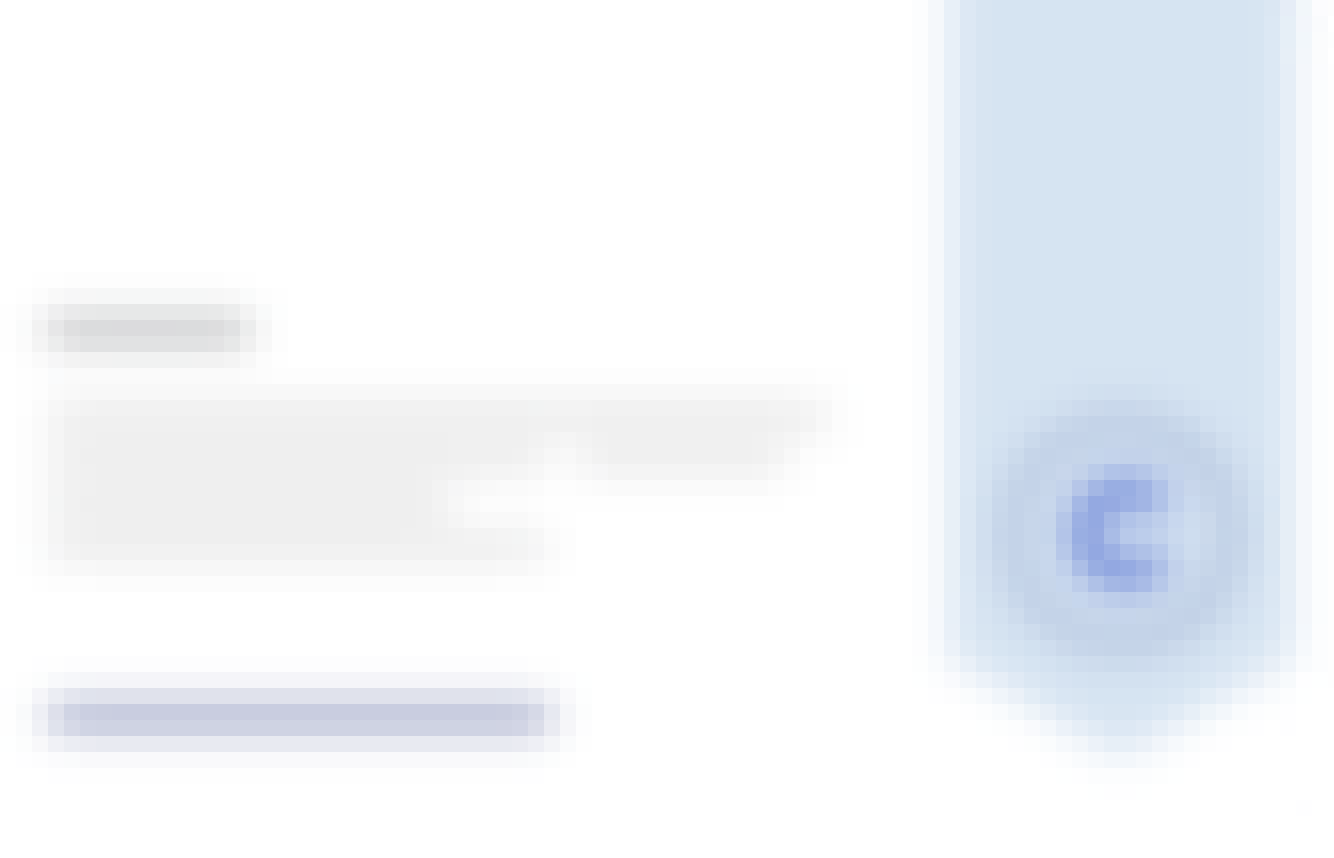
There are 14 modules in this course
In this module, we will lay the foundation for the course by setting up the development environment with Anaconda, familiarizing you with the course materials, and introducing you to creating simple movie recommendations.
What's included
7 videos1 reading
In this module, we will cover the essentials of Python programming, including basic syntax, data structures, and functions. We will also delve into Boolean expressions and loops through hands-on challenges.
What's included
4 videos
In this module, we will explore various methods for evaluating recommender systems, including accuracy metrics, hit rates, and diversity measures. We will also review practical examples and quizzes to reinforce learning.
What's included
9 videos1 assignment
In this module, we will focus on the architecture of a recommender engine framework, guiding you through code walkthroughs and activities to implement and test various recommendation algorithms.
What's included
4 videos
In this module, we will dive into content-based filtering methods, exploring metrics like cosine similarity and KNN. We will also conduct hands-on activities to produce and evaluate movie recommendations.
What's included
6 videos
In this module, we will cover neighborhood-based collaborative filtering techniques, including user-based and item-based methods. Practical exercises and activities will help solidify your understanding of these approaches.
What's included
13 videos1 assignment
In this module, we will explore matrix factorization methods like PCA and SVD, demonstrating how to apply these techniques to movie rating datasets. We will also focus on improving these methods through hyperparameter tuning.
What's included
6 videos
In this module, we will provide an optional deep dive into deep learning, covering fundamental concepts, neural network architectures, and practical implementations using TensorFlow and Keras.
What's included
25 videos
In this module, we will focus on applying deep learning to recommender systems, exploring techniques like Restricted Boltzmann Machines (RBM) and auto-encoders. We will also cover practical evaluation and tuning methods.
What's included
19 videos1 assignment
In this module, we will explore methods to scale up recommendation systems, including using Apache Spark for large-scale data processing and Amazon's DSSTNE and SageMaker for deploying scalable machine learning models.
What's included
11 videos
In this module, we will tackle real-world challenges faced by recommender systems, such as the cold start problem, filtering bubbles, and fraud. We will also explore solutions to these issues through practical exercises.
What's included
11 videos1 assignment
In this module, we will study real-world case studies of YouTube and Netflix, focusing on their recommendation strategies and the use of deep learning and hybrid approaches to enhance recommendation quality.
What's included
4 videos
In this module, we will explore hybrid recommendation approaches, combining multiple algorithms to improve recommendation accuracy and diversity. Practical exercises will guide you through implementing and evaluating hybrid systems.
What's included
2 videos1 assignment
In this module, we will wrap up the course by summarizing key points, providing resources for further study, and introducing advanced topics and emerging trends in recommender systems to keep you up-to-date.
What's included
1 video1 assignment
Instructor

Offered by
Recommended if you're interested in Machine Learning
University of Minnesota
Sungkyunkwan University
Why people choose Coursera for their career




New to Machine Learning? Start here.

Open new doors with Coursera Plus
Unlimited access to 10,000+ world-class courses, hands-on projects, and job-ready certificate programs - all included in your subscription
Advance your career with an online degree
Earn a degree from world-class universities - 100% online
Join over 3,400 global companies that choose Coursera for Business
Upskill your employees to excel in the digital economy
Frequently asked questions
Yes, you can preview the first video and view the syllabus before you enroll. You must purchase the course to access content not included in the preview.
If you decide to enroll in the course before the session start date, you will have access to all of the lecture videos and readings for the course. You’ll be able to submit assignments once the session starts.
Once you enroll and your session begins, you will have access to all videos and other resources, including reading items and the course discussion forum. You’ll be able to view and submit practice assessments, and complete required graded assignments to earn a grade and a Course Certificate.
More questions
Financial aid available,