Unlock the power of deep learning and elevate your machine learning skills with our comprehensive deep neural networks course. This hands-on program covers deep learning fundamentals, including artificial neural networks, activation functions, bias, data, and loss functions.

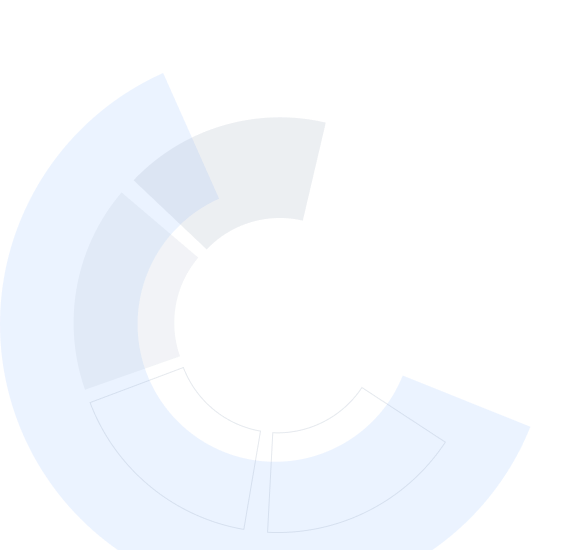
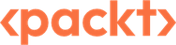
Recommended experience
What you'll learn
Explain the fundamentals of deep learning and neural networks.
Use Python to build and train your own deep neural network models.
Differentiate between various activation functions and optimization algorithms.
Assess techniques for improving model performance and reducing overfitting.
Details to know

Add to your LinkedIn profile
September 2024
7 assignments
See how employees at top companies are mastering in-demand skills
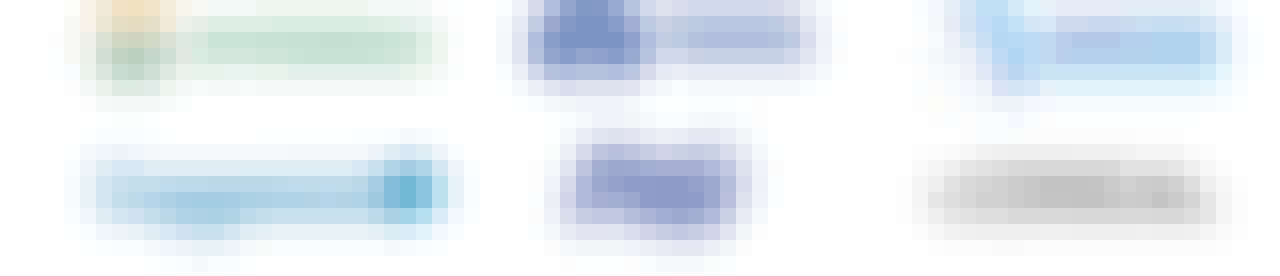
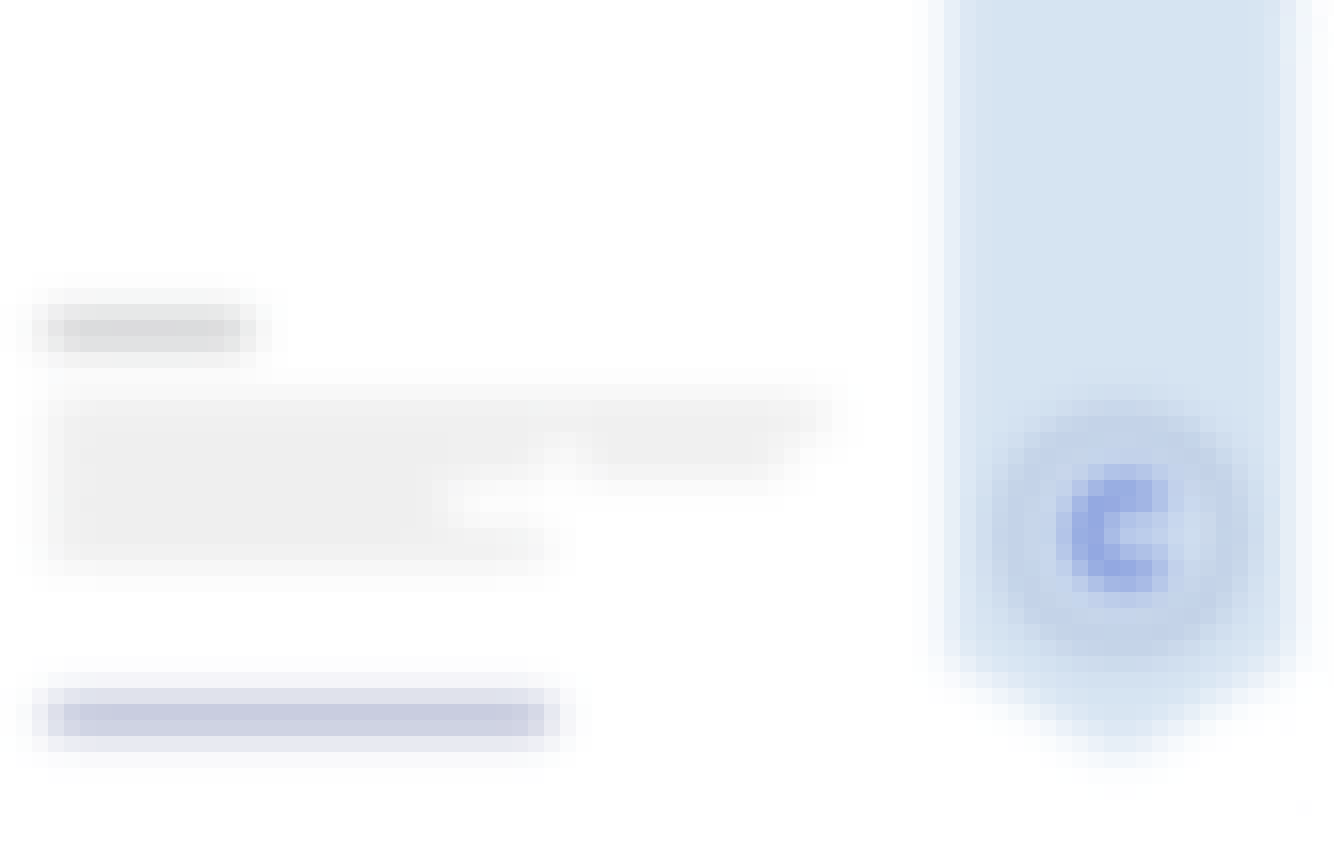
Earn a career certificate
Add this credential to your LinkedIn profile, resume, or CV
Share it on social media and in your performance review
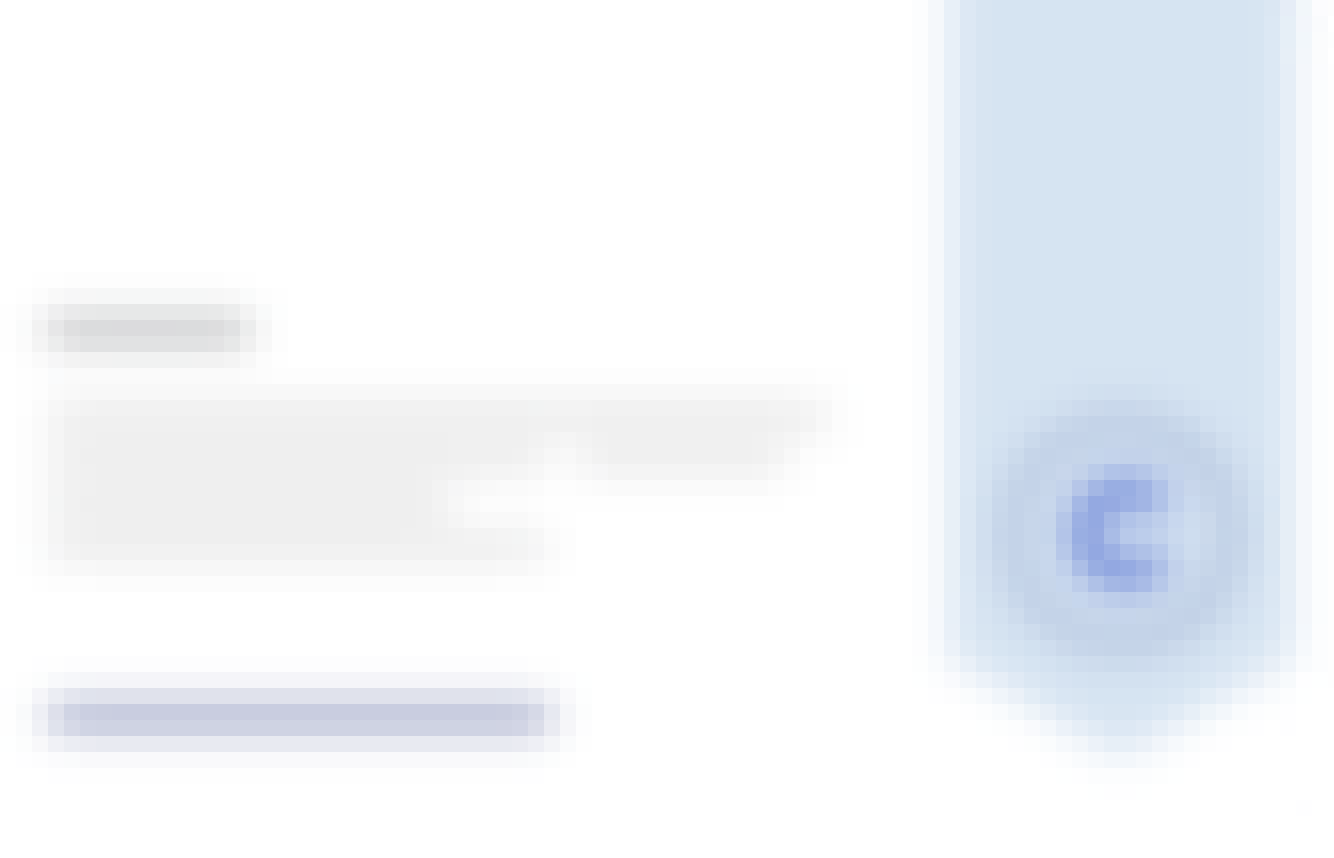
There are 17 modules in this course
In this module, we will welcome you to the course and provide an overview of deep learning. We will explain the course objectives, the structure of the content, and the skills and knowledge you will acquire throughout the course.
What's included
2 videos1 reading
In this module, we will lay the foundation for understanding deep learning by covering essential topics such as artificial neural networks, activation functions, and bias. We will also explore the role of data, various applications, models, loss functions, and learning algorithms crucial for model performance.
What's included
8 videos
In this module, we will provide a crash course on the basics of Python programming, essential for deep learning. You will learn how to install and use Jupyter Notebook and Google Colab, understand data types, containers, control statements, and implement functions and classes in Python.
What's included
7 videos1 assignment
In this module, we will delve into Python libraries crucial for data science. You will learn how to handle arrays with NumPy, manipulate data using Pandas, and visualize data with Matplotlib. We will cover topics from basic data structures to advanced data cleaning and plotting techniques.
What's included
8 videos
In this module, we will explore the MP Neuron model, also known as the McCulloch-Pitts model. You will gain an understanding of the data intuition, learn how to find parameters, and develop a mathematical intuition for this fundamental concept in neural networks.
What's included
4 videos
In this module, we will focus on implementing the MP Neuron model in Python. You will learn how to import datasets, apply train-test split, and modify data. By the end of this section, you will have created an MP Neuron class from scratch and practiced with an assignment.
What's included
5 videos1 assignment
In this module, we will summarize the key concepts and practical implementation of the MP Neuron model. We will review the important points and ensure you have a solid understanding through a recap and evaluation assignments.
What's included
1 video
In this module, we will cover the Perceptron model, discussing its representation, loss function, and parameter updates. You will understand how the update rule works and see its practical implementation in programs.
What's included
5 videos
In this module, we will implement the Perceptron model in Python. You will learn to program the model and visualize its accuracy and performance with increasing epochs, enhancing your practical skills in deep learning.
What's included
2 videos1 assignment
In this module, we will transition from Perceptron to Sigmoid Neuron. You will learn about the limitations of the Perceptron, the benefits of the Sigmoid Neuron, and gain insights into gradient descent for model optimization.
What's included
8 videos
In this module, we will implement the Sigmoid Neuron using Python. You will learn to download and standardize datasets, and create a class for the Sigmoid activation function, solidifying your understanding through practical assignments.
What's included
4 videos
In this module, we will cover basic probability concepts. You will learn about random variables, their importance, types, and probability distribution tables, as well as the concept of entropy loss in the context of deep learning.
What's included
5 videos1 assignment
In this module, we will explore deep neural networks. You will learn why they are important, and through practical programming, understand the concept of linear separation of data, preparing you for more complex deep learning models.
What's included
2 videos
In this module, we will delve into the Universal Approximation Theorem. You will learn its significance, confirm its effectiveness with practical examples, and discuss the challenges of building deep neural networks from scratch.
What's included
4 videos
In this module, we will focus on TensorFlow 2.x for deep learning. You will learn to build, train, and evaluate neural networks using TensorFlow, with a recap of deep learning concepts and a summary to prepare for more advanced topics.
What's included
7 videos1 assignment
In this module, we will cover activation functions in deep learning. You will learn about different activation functions provided by TensorFlow and understand common network configurations used in deep learning tasks.
What's included
4 videos
In this module, we will apply deep learning concepts. You will transition from shallow to deep learning, understand Keras basics, solve classification and regression problems, and explore advanced TensorFlow techniques and subclassing methods.
What's included
8 videos2 assignments
Instructor

Offered by
Recommended if you're interested in Mobile and Web Development
DeepLearning.AI
University of Colorado Boulder
Coursera Project Network
DeepLearning.AI
Why people choose Coursera for their career




New to Mobile and Web Development? Start here.

Open new doors with Coursera Plus
Unlimited access to 10,000+ world-class courses, hands-on projects, and job-ready certificate programs - all included in your subscription
Advance your career with an online degree
Earn a degree from world-class universities - 100% online
Join over 3,400 global companies that choose Coursera for Business
Upskill your employees to excel in the digital economy
Frequently asked questions
Yes, you can preview the first video and view the syllabus before you enroll. You must purchase the course to access content not included in the preview.
If you decide to enroll in the course before the session start date, you will have access to all of the lecture videos and readings for the course. You’ll be able to submit assignments once the session starts.
Once you enroll and your session begins, you will have access to all videos and other resources, including reading items and the course discussion forum. You’ll be able to view and submit practice assessments, and complete required graded assignments to earn a grade and a Course Certificate.