In this course, you will learn about several algorithms that can learn near optimal policies based on trial and error interaction with the environment---learning from the agent’s own experience. Learning from actual experience is striking because it requires no prior knowledge of the environment’s dynamics, yet can still attain optimal behavior. We will cover intuitively simple but powerful Monte Carlo methods, and temporal difference learning methods including Q-learning. We will wrap up this course investigating how we can get the best of both worlds: algorithms that can combine model-based planning (similar to dynamic programming) and temporal difference updates to radically accelerate learning.

Give your career the gift of Coursera Plus with $160 off, billed annually. Save today.
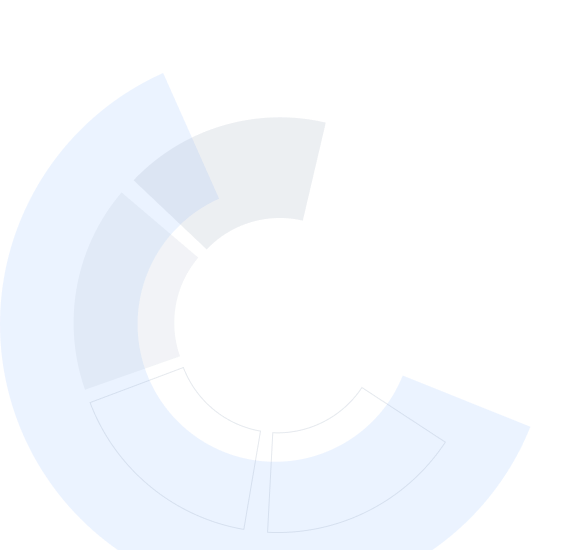
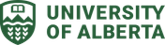
Sample-based Learning Methods
This course is part of Reinforcement Learning Specialization


Instructors: Martha White
33,662 already enrolled
Included with
(1,228 reviews)
Recommended experience
Skills you'll gain
Details to know

Add to your LinkedIn profile
5 assignments
See how employees at top companies are mastering in-demand skills
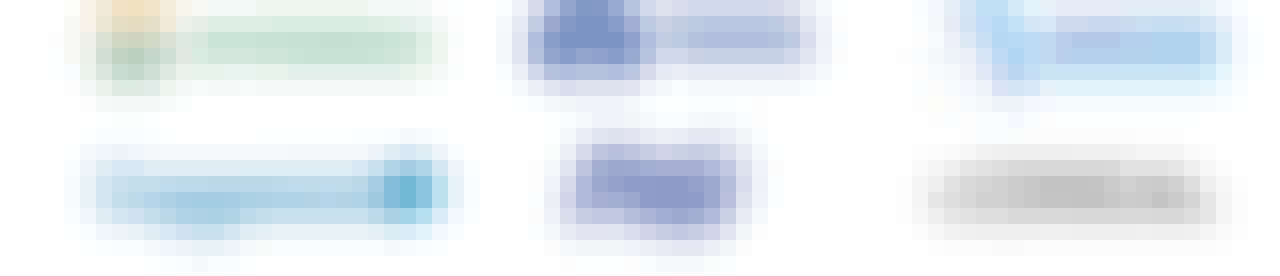
Build your subject-matter expertise
- Learn new concepts from industry experts
- Gain a foundational understanding of a subject or tool
- Develop job-relevant skills with hands-on projects
- Earn a shareable career certificate
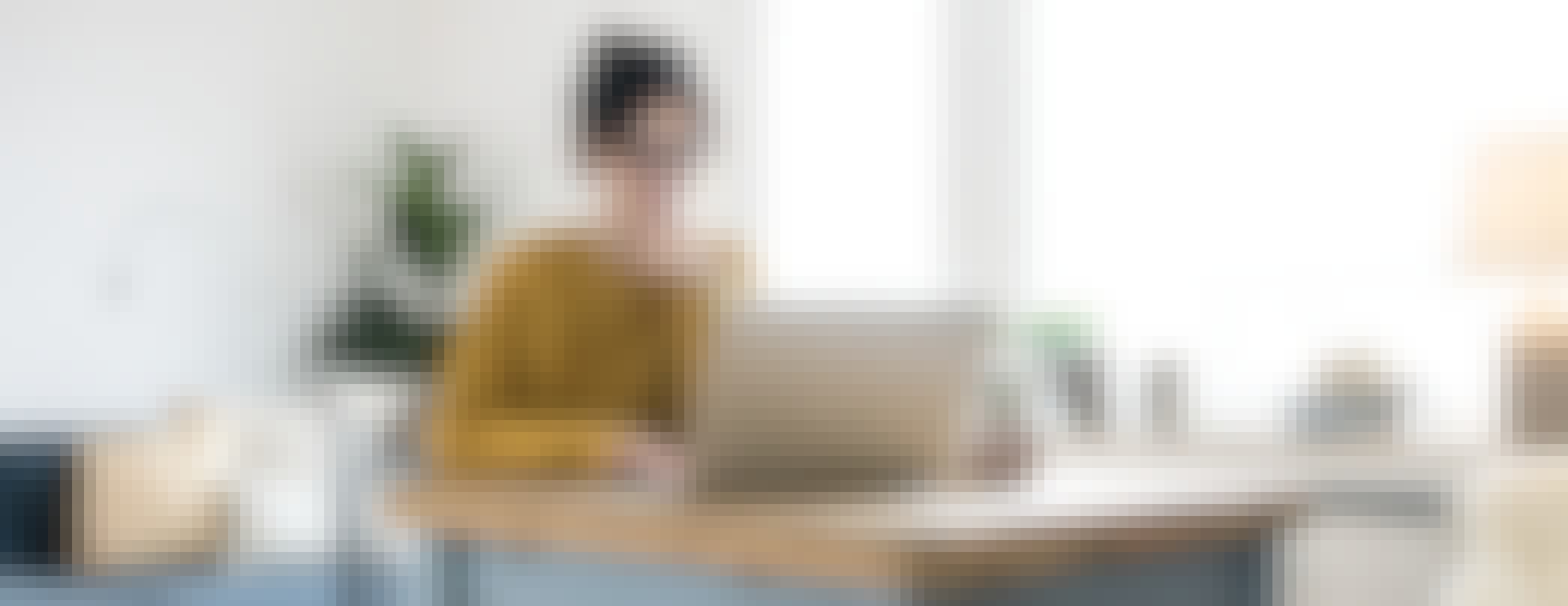
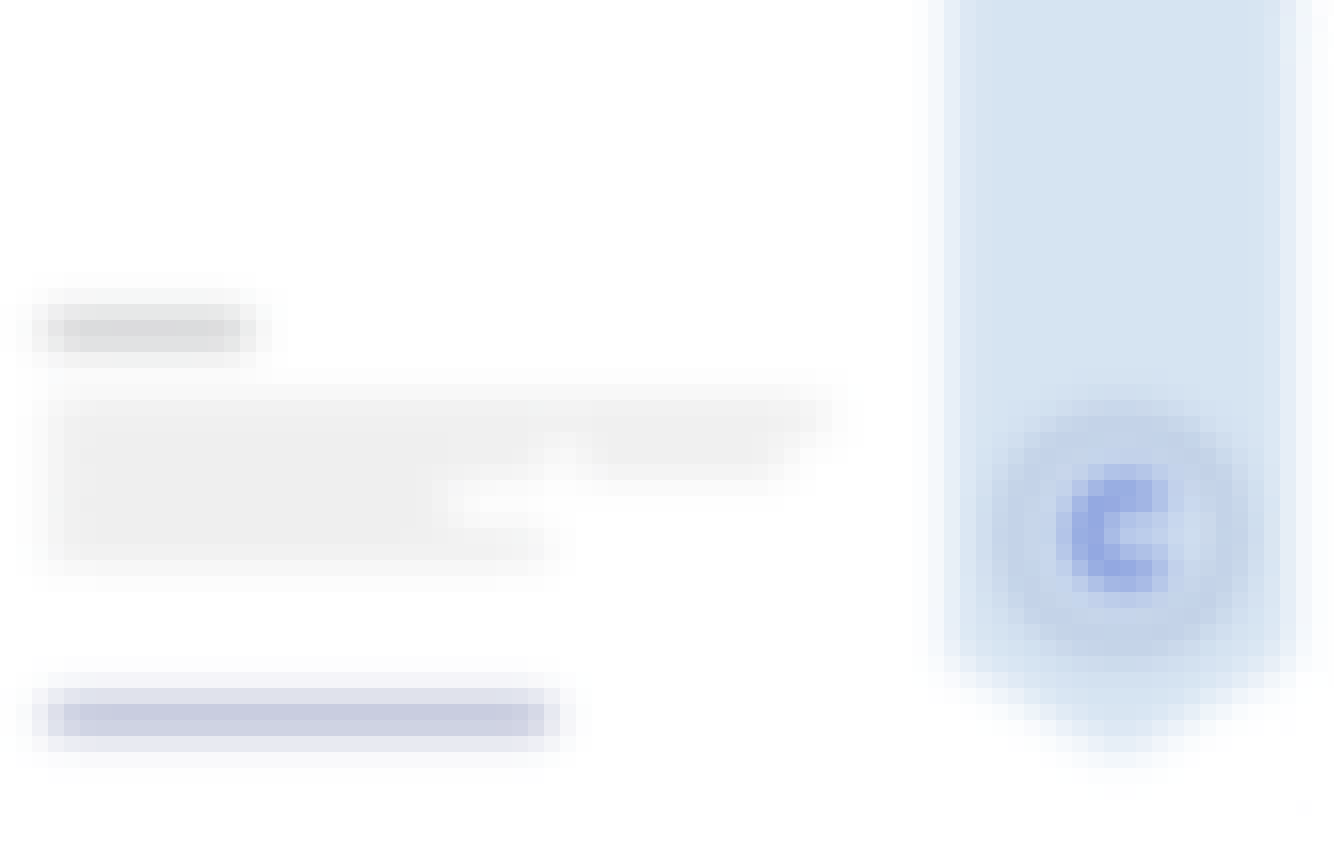
Earn a career certificate
Add this credential to your LinkedIn profile, resume, or CV
Share it on social media and in your performance review
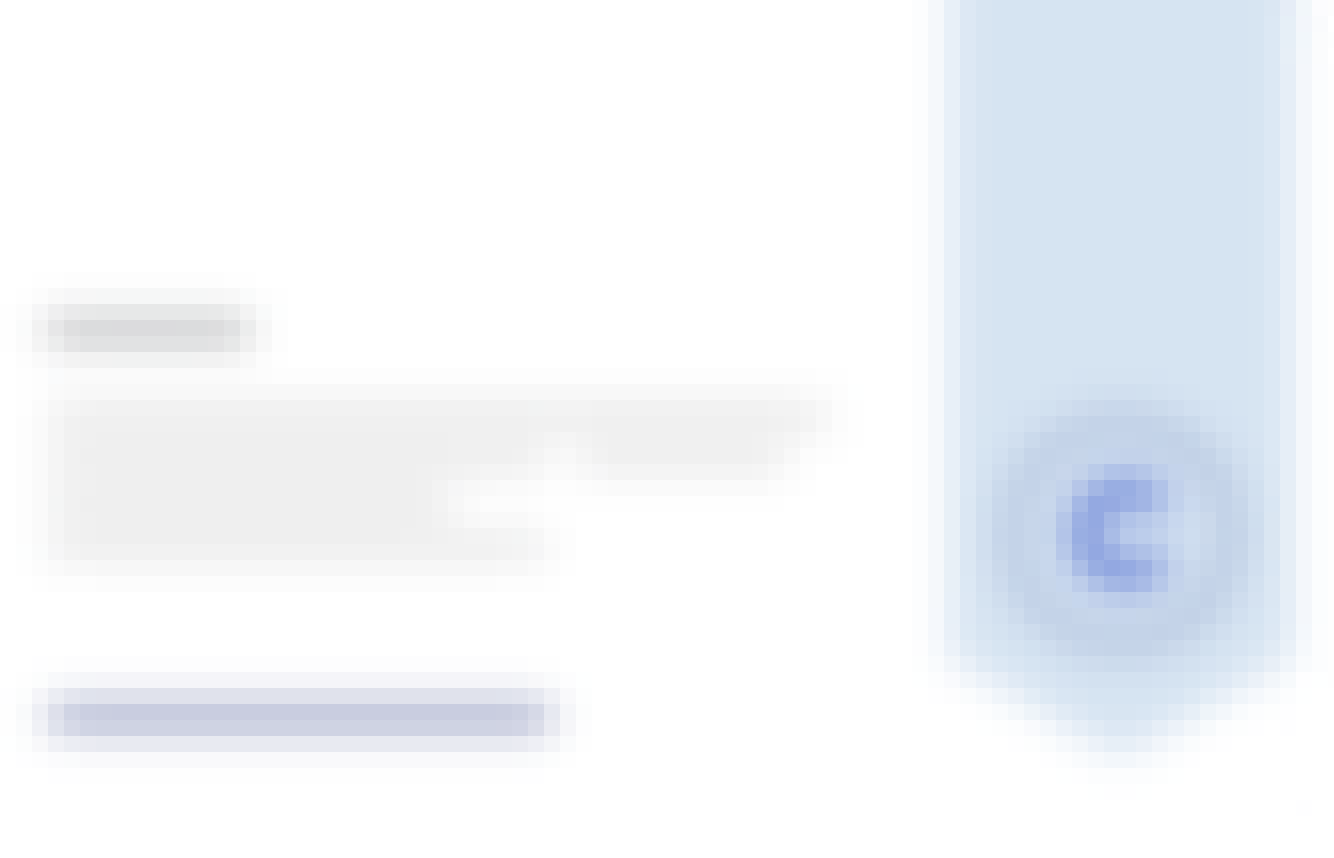
There are 5 modules in this course
Welcome to the second course in the Reinforcement Learning Specialization: Sample-Based Learning Methods, brought to you by the University of Alberta, Onlea, and Coursera. In this pre-course module, you'll be introduced to your instructors, and get a flavour of what the course has in store for you. Make sure to introduce yourself to your classmates in the "Meet and Greet" section!
What's included
2 videos2 readings1 discussion prompt
This week you will learn how to estimate value functions and optimal policies, using only sampled experience from the environment. This module represents our first step toward incremental learning methods that learn from the agent’s own interaction with the world, rather than a model of the world. You will learn about on-policy and off-policy methods for prediction and control, using Monte Carlo methods---methods that use sampled returns. You will also be reintroduced to the exploration problem, but more generally in RL, beyond bandits.
What's included
11 videos3 readings1 assignment1 programming assignment1 discussion prompt
This week, you will learn about one of the most fundamental concepts in reinforcement learning: temporal difference (TD) learning. TD learning combines some of the features of both Monte Carlo and Dynamic Programming (DP) methods. TD methods are similar to Monte Carlo methods in that they can learn from the agent’s interaction with the world, and do not require knowledge of the model. TD methods are similar to DP methods in that they bootstrap, and thus can learn online---no waiting until the end of an episode. You will see how TD can learn more efficiently than Monte Carlo, due to bootstrapping. For this module, we first focus on TD for prediction, and discuss TD for control in the next module. This week, you will implement TD to estimate the value function for a fixed policy, in a simulated domain.
What's included
6 videos2 readings1 assignment1 programming assignment1 discussion prompt
This week, you will learn about using temporal difference learning for control, as a generalized policy iteration strategy. You will see three different algorithms based on bootstrapping and Bellman equations for control: Sarsa, Q-learning and Expected Sarsa. You will see some of the differences between the methods for on-policy and off-policy control, and that Expected Sarsa is a unified algorithm for both. You will implement Expected Sarsa and Q-learning, on Cliff World.
What's included
9 videos3 readings1 assignment1 programming assignment1 discussion prompt
Up until now, you might think that learning with and without a model are two distinct, and in some ways, competing strategies: planning with Dynamic Programming verses sample-based learning via TD methods. This week we unify these two strategies with the Dyna architecture. You will learn how to estimate the model from data and then use this model to generate hypothetical experience (a bit like dreaming) to dramatically improve sample efficiency compared to sample-based methods like Q-learning. In addition, you will learn how to design learning systems that are robust to inaccurate models.
What's included
11 videos4 readings2 assignments1 programming assignment1 discussion prompt
Instructors


Recommended if you're interested in Machine Learning
Johns Hopkins University
University of Alberta
Johns Hopkins University
Wesleyan University
Why people choose Coursera for their career




Learner reviews
Showing 3 of 1228
1,228 reviews
- 5 stars
82.18%
- 4 stars
13.42%
- 3 stars
2.84%
- 2 stars
0.56%
- 1 star
0.97%
New to Machine Learning? Start here.

Open new doors with Coursera Plus
Unlimited access to 7,000+ world-class courses, hands-on projects, and job-ready certificate programs - all included in your subscription
Advance your career with an online degree
Earn a degree from world-class universities - 100% online
Join over 3,400 global companies that choose Coursera for Business
Upskill your employees to excel in the digital economy
Frequently asked questions
Access to lectures and assignments depends on your type of enrollment. If you take a course in audit mode, you will be able to see most course materials for free. To access graded assignments and to earn a Certificate, you will need to purchase the Certificate experience, during or after your audit. If you don't see the audit option:
The course may not offer an audit option. You can try a Free Trial instead, or apply for Financial Aid.
The course may offer 'Full Course, No Certificate' instead. This option lets you see all course materials, submit required assessments, and get a final grade. This also means that you will not be able to purchase a Certificate experience.
When you enroll in the course, you get access to all of the courses in the Specialization, and you earn a certificate when you complete the work. Your electronic Certificate will be added to your Accomplishments page - from there, you can print your Certificate or add it to your LinkedIn profile. If you only want to read and view the course content, you can audit the course for free.
If you subscribed, you get a 7-day free trial during which you can cancel at no penalty. After that, we don’t give refunds, but you can cancel your subscription at any time. See our full refund policy.