This course introduces you to one of the main types of modelling families of supervised Machine Learning: Regression. You will learn how to train regression models to predict continuous outcomes and how to use error metrics to compare across different models. This course also walks you through best practices, including train and test splits, and regularization techniques.

Give your career the gift of Coursera Plus with $160 off, billed annually. Save today.
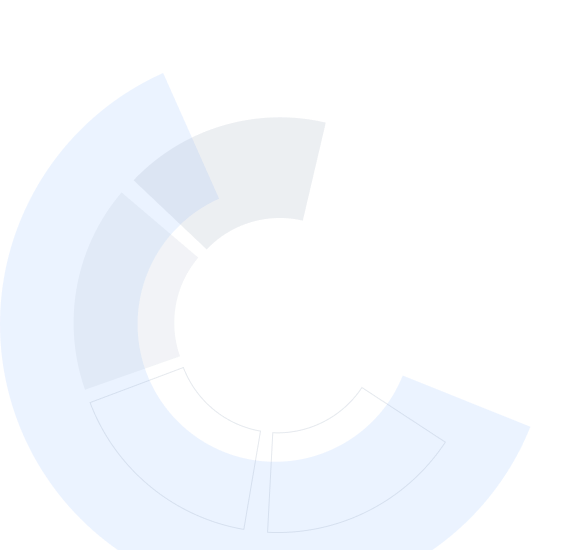
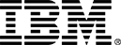
Supervised Machine Learning: Regression
This course is part of multiple programs.



Instructors: Mark J Grover
53,392 already enrolled
Included with
(620 reviews)
Skills you'll gain
Details to know

Add to your LinkedIn profile
13 assignments
See how employees at top companies are mastering in-demand skills
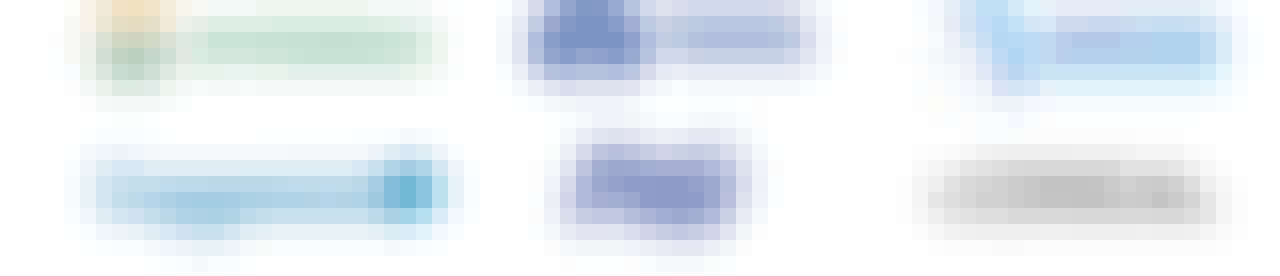
Build your subject-matter expertise
- Learn new concepts from industry experts
- Gain a foundational understanding of a subject or tool
- Develop job-relevant skills with hands-on projects
- Earn a shareable career certificate
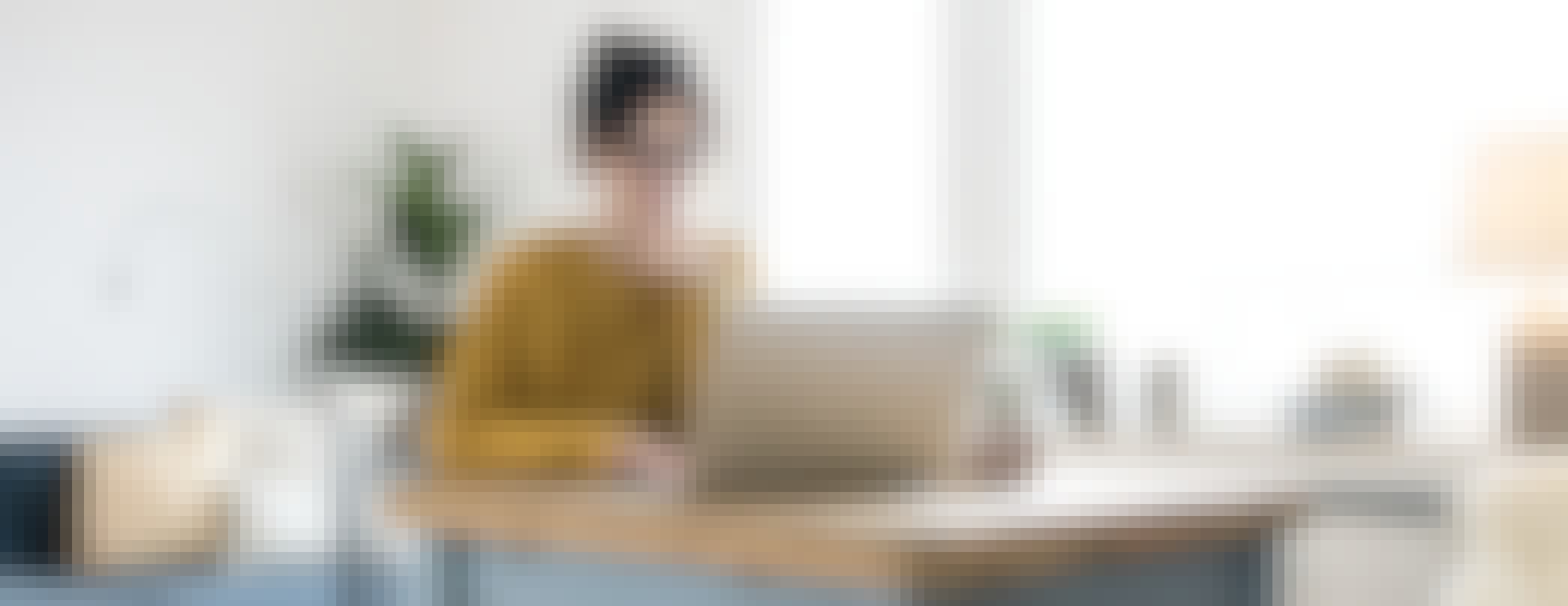
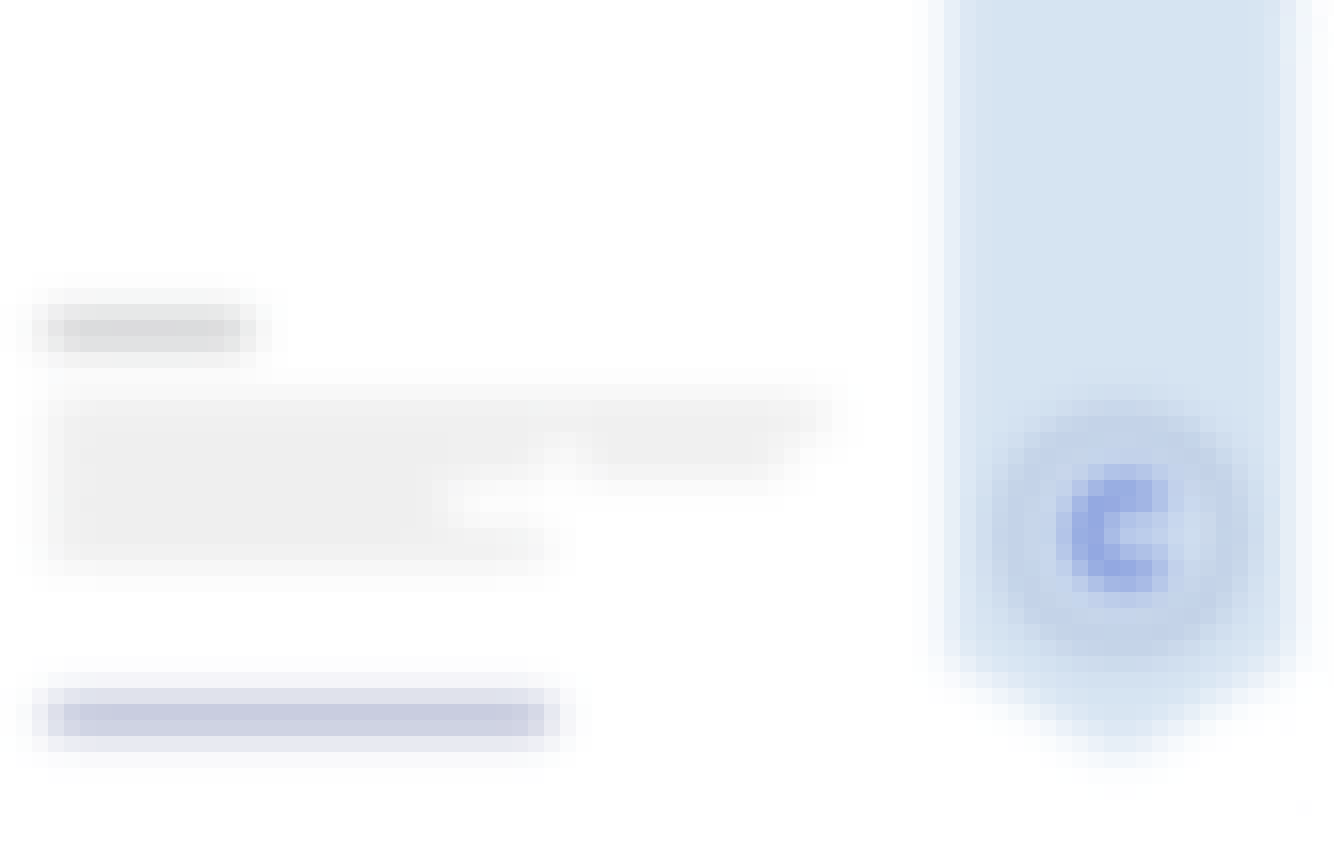
Earn a career certificate
Add this credential to your LinkedIn profile, resume, or CV
Share it on social media and in your performance review
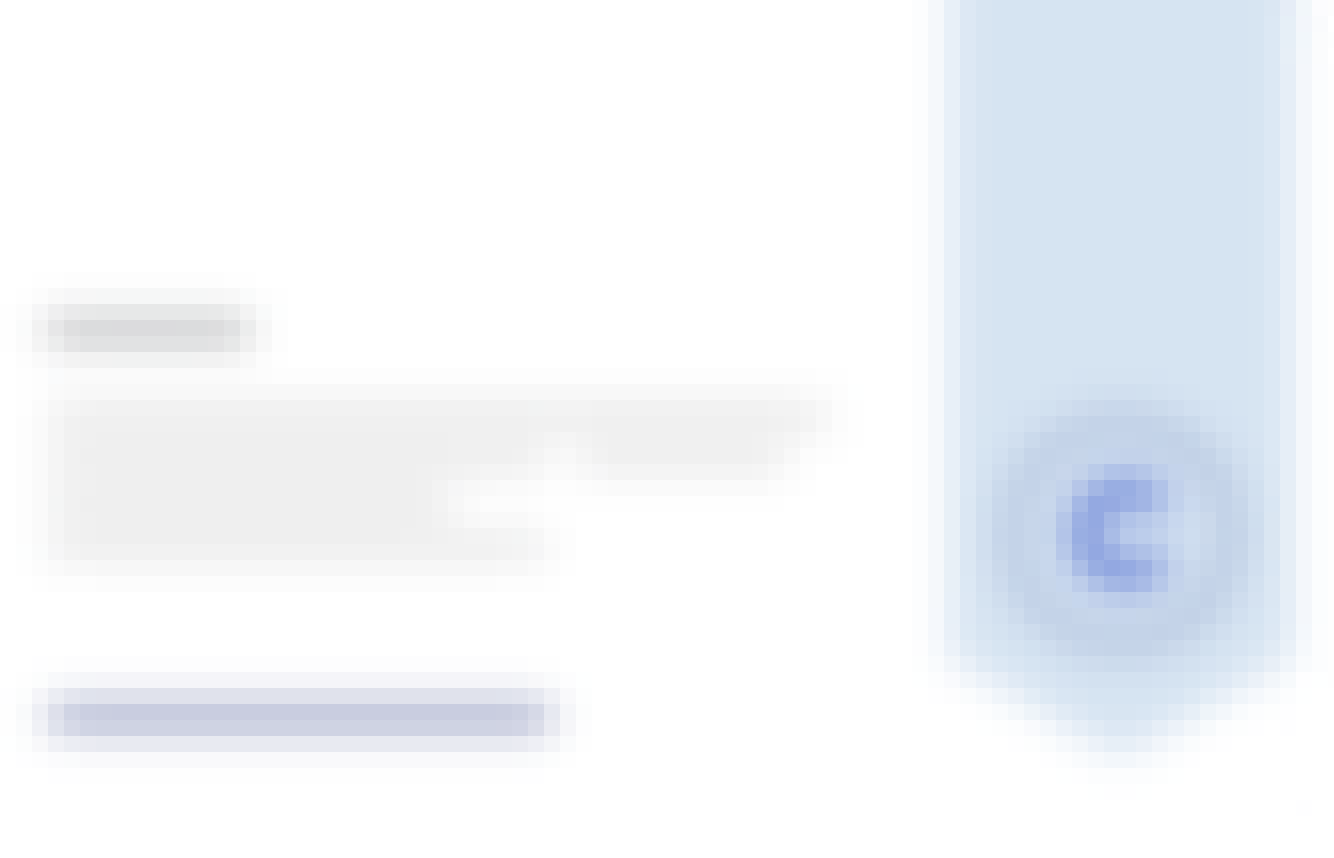
There are 6 modules in this course
This module introduces a brief overview of supervised machine learning and its main applications: classification and regression. After introducing the concept of regression, you will learn its best practices, as well as how to measure error and select the regression model that best suits your data.
What's included
11 videos2 readings3 assignments2 app items
There are a few best practices to avoid overfitting of your regression models. One of these best practices is splitting your data into training and test sets. Another alternative is to use cross validation. And a third alternative is to introduce polynomial features. This module walks you through the theoretical framework and a few hands-on examples of these best practices.
What's included
7 videos1 reading3 assignments2 app items
There is a trade-off between the size of your training set and your testing set. If you use most of your data for training, you will have fewer samples to validate your model. Conversely, if you use more samples for testing, you will have fewer samples to train your model. Cross Validation will allow you to reuse your data to use more samples for training and testing.
What's included
6 videos1 reading2 assignments2 app items1 plugin
This module walks you through the theory and a few hands-on examples of regularization regressions including ridge, LASSO, and elastic net. You will realize the main pros and cons of these techniques, as well as their differences and similarities.
What's included
10 videos1 reading3 assignments1 app item
In this section, you will understand the relationship between the loss function and the different regularization types.
What's included
5 videos1 reading2 assignments2 app items
In this section you will test everything you learned
What's included
1 reading1 peer review1 app item
Instructors



Offered by
Recommended if you're interested in Machine Learning
Coursera Project Network
Duke University
Why people choose Coursera for their career




Learner reviews
Showing 3 of 620
620 reviews
- 5 stars
76.92%
- 4 stars
16.82%
- 3 stars
4%
- 2 stars
0.80%
- 1 star
1.44%
New to Machine Learning? Start here.

Open new doors with Coursera Plus
Unlimited access to 7,000+ world-class courses, hands-on projects, and job-ready certificate programs - all included in your subscription
Advance your career with an online degree
Earn a degree from world-class universities - 100% online
Join over 3,400 global companies that choose Coursera for Business
Upskill your employees to excel in the digital economy
Frequently asked questions
Access to lectures and assignments depends on your type of enrollment. If you take a course in audit mode, you will be able to see most course materials for free. To access graded assignments and to earn a Certificate, you will need to purchase the Certificate experience, during or after your audit. If you don't see the audit option:
The course may not offer an audit option. You can try a Free Trial instead, or apply for Financial Aid.
The course may offer 'Full Course, No Certificate' instead. This option lets you see all course materials, submit required assessments, and get a final grade. This also means that you will not be able to purchase a Certificate experience.
When you enroll in the course, you get access to all of the courses in the Certificate, and you earn a certificate when you complete the work. Your electronic Certificate will be added to your Accomplishments page - from there, you can print your Certificate or add it to your LinkedIn profile. If you only want to read and view the course content, you can audit the course for free.
If you subscribed, you get a 7-day free trial during which you can cancel at no penalty. After that, we don’t give refunds, but you can cancel your subscription at any time. See our full refund policy.