Uncertainty Quantification (UQ) is the science of mathematically quantifying and reducing uncertainty in systems of all types. Students will learn the nature and role of uncertainty in physical, mathematical, and engineering systems along with the basics of probability theory necessary to quantify uncertainty. The course provides an introduction to various sub-topics of UQ including uncertainty propagation, surrogate modeling, reliability analysis, random processes and random fields, and Bayesian inverse UQ methods.

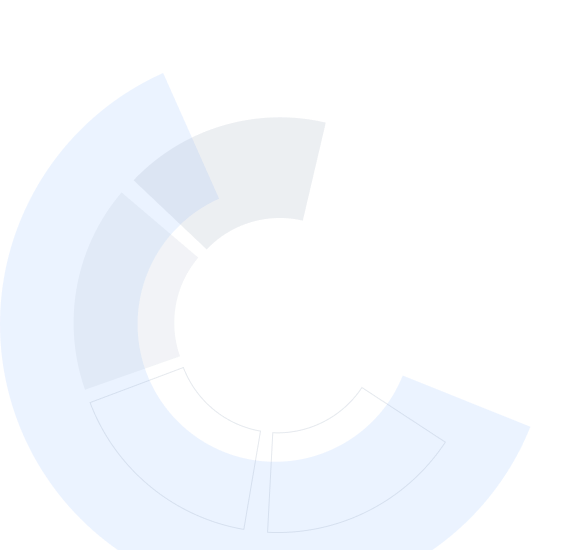
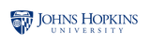
Introduction to Uncertainty Quantification

Instructor: Michael Shields
Sponsored by Abu Dhabi National Oil Company
Recommended experience
Skills you'll gain
- Simulations
- Mathematics and Mathematical Modeling
- Data Collection
- Applied Mathematics
- Bayesian Statistics
- Estimation
- Statistics
- Engineering Software
- Statistical Modeling
- Probability
- Statistical Inference
- Statistical Methods
- Probability Distribution
- Mathematical Modeling
- Probability & Statistics
- Advanced Mathematics
- Statistical Analysis
- Simulation and Simulation Software
- Sampling (Statistics)
Details to know

Add to your LinkedIn profile
25 assignments
December 2024
See how employees at top companies are mastering in-demand skills
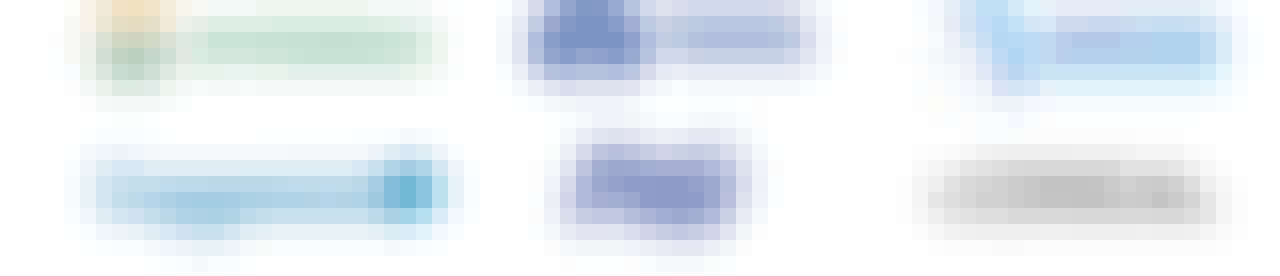
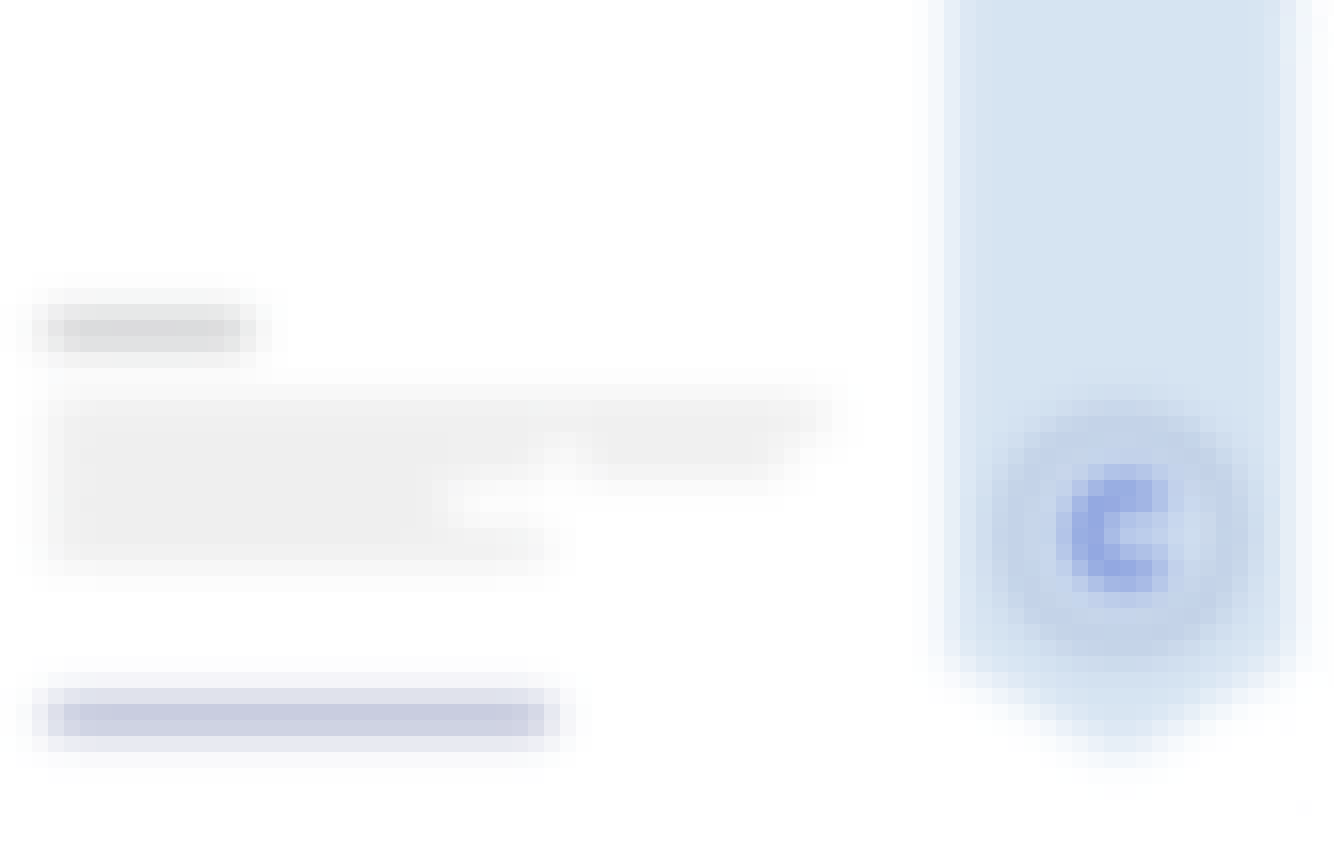
Earn a career certificate
Add this credential to your LinkedIn profile, resume, or CV
Share it on social media and in your performance review
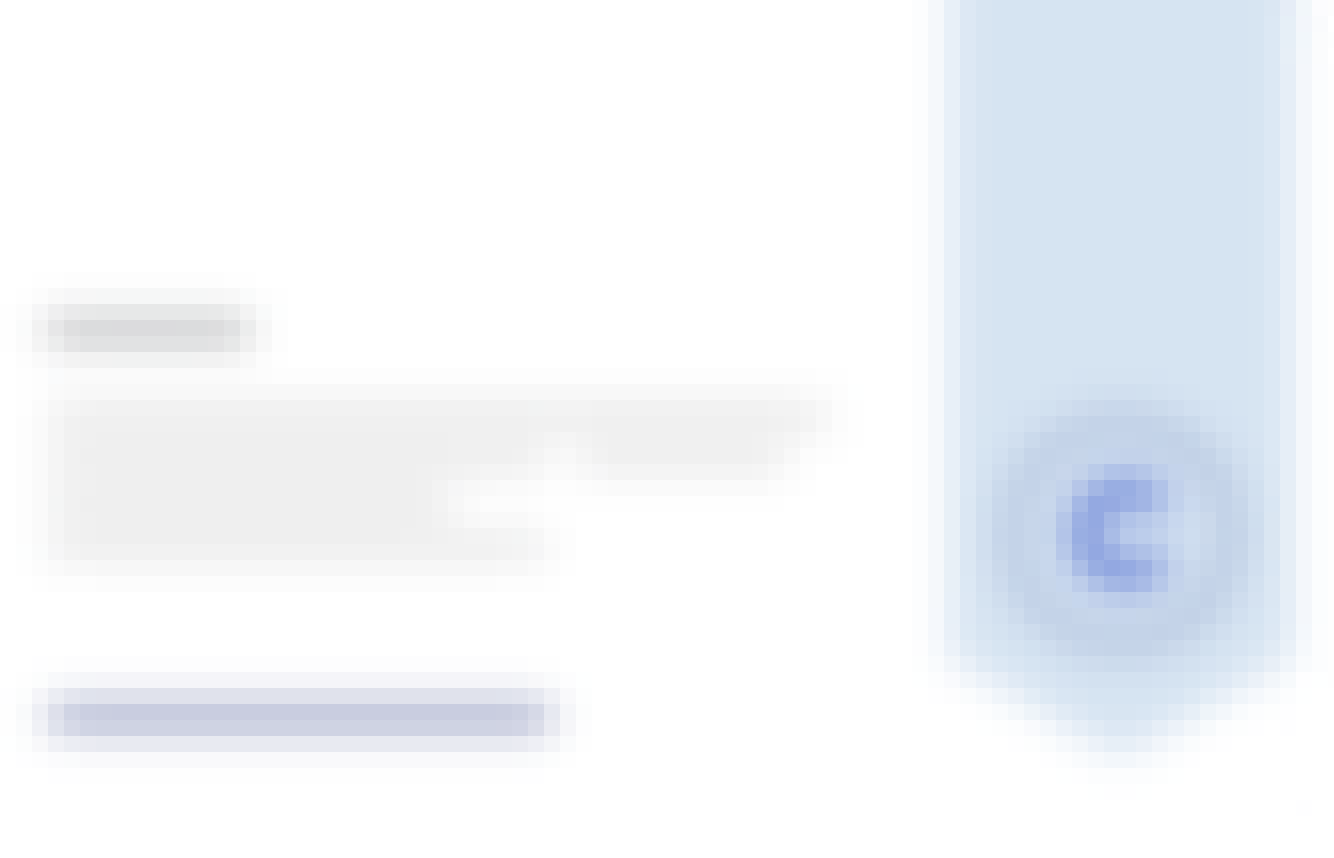
There are 4 modules in this course
This module sets the stage for uncertainty quantification by carefully defining the different types of uncertainties that may be present. Lesson one introduces aleatory and epistemic uncertainties. The second lesson helps to build your understanding of aleatory and epistemic uncertainties and tell the difference between the two. The third, and final module discusses from a board perspective how we mathematically treat aleatory and epistemic uncertainty.
What's included
12 videos3 readings3 assignments2 discussion prompts1 ungraded lab
This module provides an introduction to probability that will be necessary for conducting probabilistic uncertainty quantification. The first lesson introduces basic concepts in probability starting with preliminaries in Set Theory and building up the Axioms of Probability. The second lesson then introduces random variables and defines them through their probability density function and cumulative distribution function. Moments of random variables are also introduced. The third lesson introduces random vectors and random processes to begin extending toward higher dimensional problems. Again, random vectors and random processes are defined through their distribution functions and moments are defined.
What's included
14 videos4 readings11 assignments1 discussion prompt
In this module, we discuss the propagation of uncertainty through a general model. We begin in Lesson 1 with simple systems where uncertainty can be propagated analytically as a function of random variables. Lesson 2 then introduces the Taylor series expansion and demonstrates how we can make a Taylor series approximation for some systems, which allows us to analytically estimate the moments of a function of random variables. Lesson 3 presents the Monte Carlo method, which is the most robust and widely-used method for propagation of uncertainty and generally serves as a benchmark against which other methods are compared. Finally, we discuss the propagation of uncertainty through the construction of surrogate models in Lesson 4. In particular, we introduce Gaussian process and polynomial chaos expansions surrogates, which are the two most commonly used approaches for uncertainty quantification.
What's included
15 videos5 readings7 assignments6 ungraded labs
This module provides a brief introduction into some of the more advanced topics in uncertainty quantification. Each of these topics could be covered in a course of their own, so we are only able to briefly introduce the most important concepts. The module begins by staying on the topic of uncertainty propagation and discussing advanced numerical methods - namely the spectral stochastic methods - in Lesson 1. Lesson 2 introduces reliability analysis, which is concerned with estimating small failure probabilities. Both approximate and Monte Carlo methods for reliability analysis are introduced. Lesson 3 introduces global sensitivity analysis, which aims to identify which random variables make the most significant contributions to uncertainty in the output of the model. Finally, Lesson 4 provides a brief introduction to Bayesian inference with a focus on Bayesian parameter estimation.
What's included
14 videos3 readings4 assignments4 ungraded labs
Instructor

Offered by
Why people choose Coursera for their career




Recommended if you're interested in Physical Science and Engineering
Johns Hopkins University
American Psychological Association
Johns Hopkins University
Johns Hopkins University

Open new doors with Coursera Plus
Unlimited access to 10,000+ world-class courses, hands-on projects, and job-ready certificate programs - all included in your subscription
Advance your career with an online degree
Earn a degree from world-class universities - 100% online
Join over 3,400 global companies that choose Coursera for Business
Upskill your employees to excel in the digital economy