By the end of this project, you will be able to develop intepretable machine learning applications explaining individual predictions rather than explaining the behavior of the prediction model as a whole. This will be done via the well known Local Interpretable Model-agnostic Explanations (LIME) as a machine learning interpretation and explanation model. In particular, in this project, you will learn how to go beyond the development and use of machine learning (ML) models, such as regression classifiers, in that we add on explainability and interpretation aspects for individual predictions.

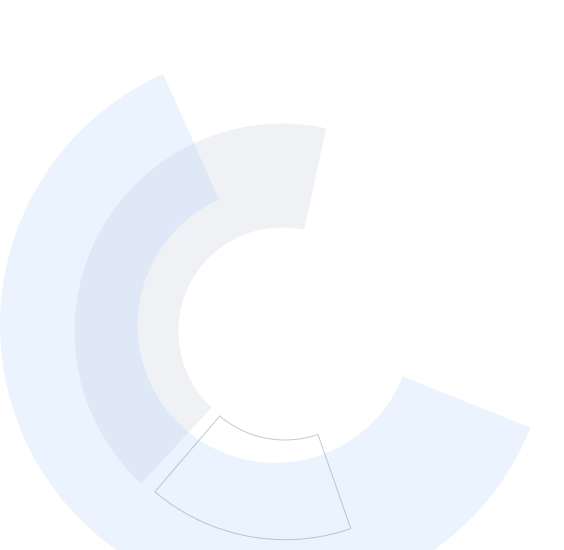
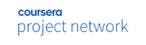
Interpretable Machine Learning Applications: Part 2

Instructor: Epaminondas Kapetanios
Sponsored by Abu Dhabi National Oil Company
2,200 already enrolled
(21 reviews)
Recommended experience
What you'll learn
Apply Local Interpretable Model-agnostic Explanations (LIME) as a machine learning interpretation
Explain individual predictions being made by a trained machine learning model.
Add aspects for individual predictions in your Machine Learning applications.
Skills you'll practice
- Machine Learning
- Statistics
- Data Science
- Advanced Analytics
- Probability & Statistics
- Artificial Intelligence and Machine Learning (AI/ML)
- Predictive Analytics
- Applied Machine Learning
- Predictive Modeling
- Artificial Intelligence
- Statistical Modeling
- Mathematical Modeling
- Computer Science
- Data Analysis
- Statistical Analysis
- Business Analytics
Details to know

Add to your LinkedIn profile
Only available on desktop
See how employees at top companies are mastering in-demand skills
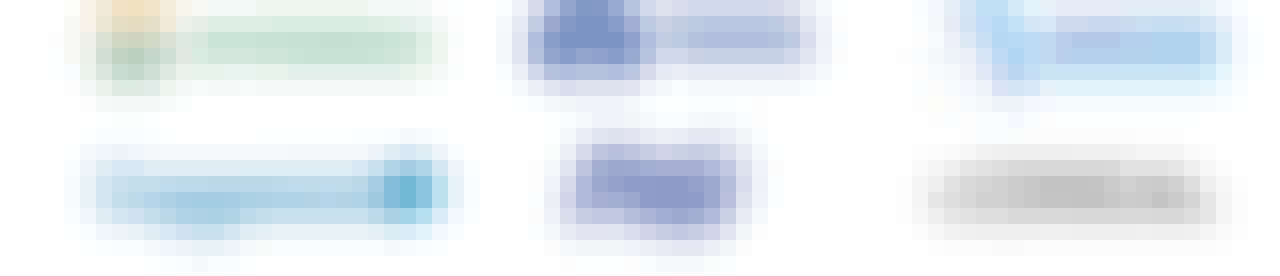
Learn, practice, and apply job-ready skills in less than 2 hours
- Receive training from industry experts
- Gain hands-on experience solving real-world job tasks
- Build confidence using the latest tools and technologies
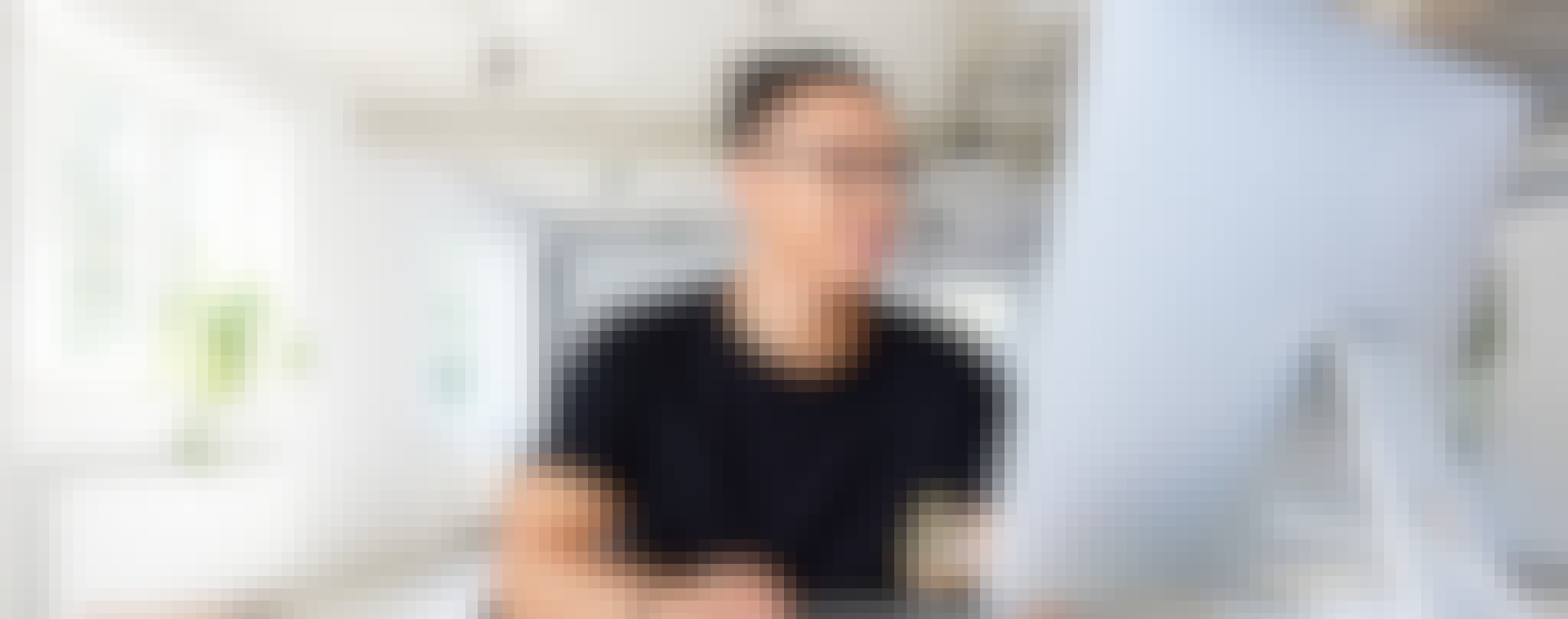
About this Guided Project
Learn step-by-step
In a video that plays in a split-screen with your work area, your instructor will walk you through these steps:
Explore and understand the features and values from the available data about red wine quality
Transform the available data into a classification dataset and problem
Prepare the data for training and validation purposes
Train, validate, estimate, and contrast the performance of three regression classifiers: Decision Tree, Random Forest, AdaBoost
Prepare and train the “explainer” in terms of the LIME library
Display and interpret explanations of individual predictions made by the three classifiers
Recommended experience
Some prior knowledge of machine learning basics and programming in Python
5 project images
Instructor

Offered by
How you'll learn
Skill-based, hands-on learning
Practice new skills by completing job-related tasks.
Expert guidance
Follow along with pre-recorded videos from experts using a unique side-by-side interface.
No downloads or installation required
Access the tools and resources you need in a pre-configured cloud workspace.
Available only on desktop
This Guided Project is designed for laptops or desktop computers with a reliable Internet connection, not mobile devices.
Why people choose Coursera for their career




You might also like
Google Cloud
DeepLearning.AI
Sungkyunkwan University

Open new doors with Coursera Plus
Unlimited access to 10,000+ world-class courses, hands-on projects, and job-ready certificate programs - all included in your subscription
Advance your career with an online degree
Earn a degree from world-class universities - 100% online
Join over 3,400 global companies that choose Coursera for Business
Upskill your employees to excel in the digital economy