This course will provide you a foundational understanding of machine learning models (logistic regression, multilayer perceptrons, convolutional neural networks, natural language processing, etc.) as well as demonstrate how these models can solve complex problems in a variety of industries, from medical diagnostics to image recognition to text prediction. In addition, we have designed practice exercises that will give you hands-on experience implementing these data science models on data sets. These practice exercises will teach you how to implement machine learning algorithms with PyTorch, open source libraries used by leading tech companies in the machine learning field (e.g., Google, NVIDIA, CocaCola, eBay, Snapchat, Uber and many more).

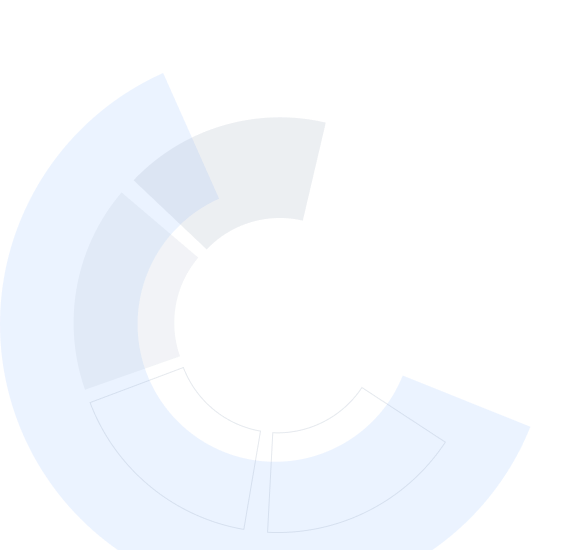
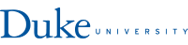
Introduction to Machine Learning



Instructors: Lawrence Carin
Sponsored by InternMart, Inc
216,721 already enrolled
(3,640 reviews)
Details to know

Add to your LinkedIn profile
24 assignments
See how employees at top companies are mastering in-demand skills
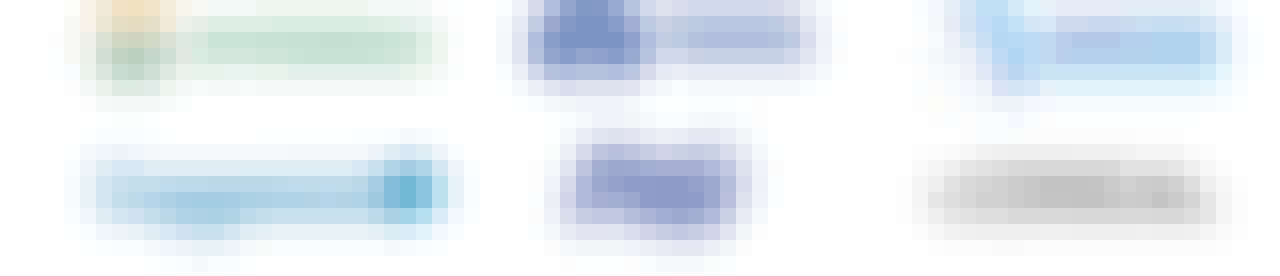
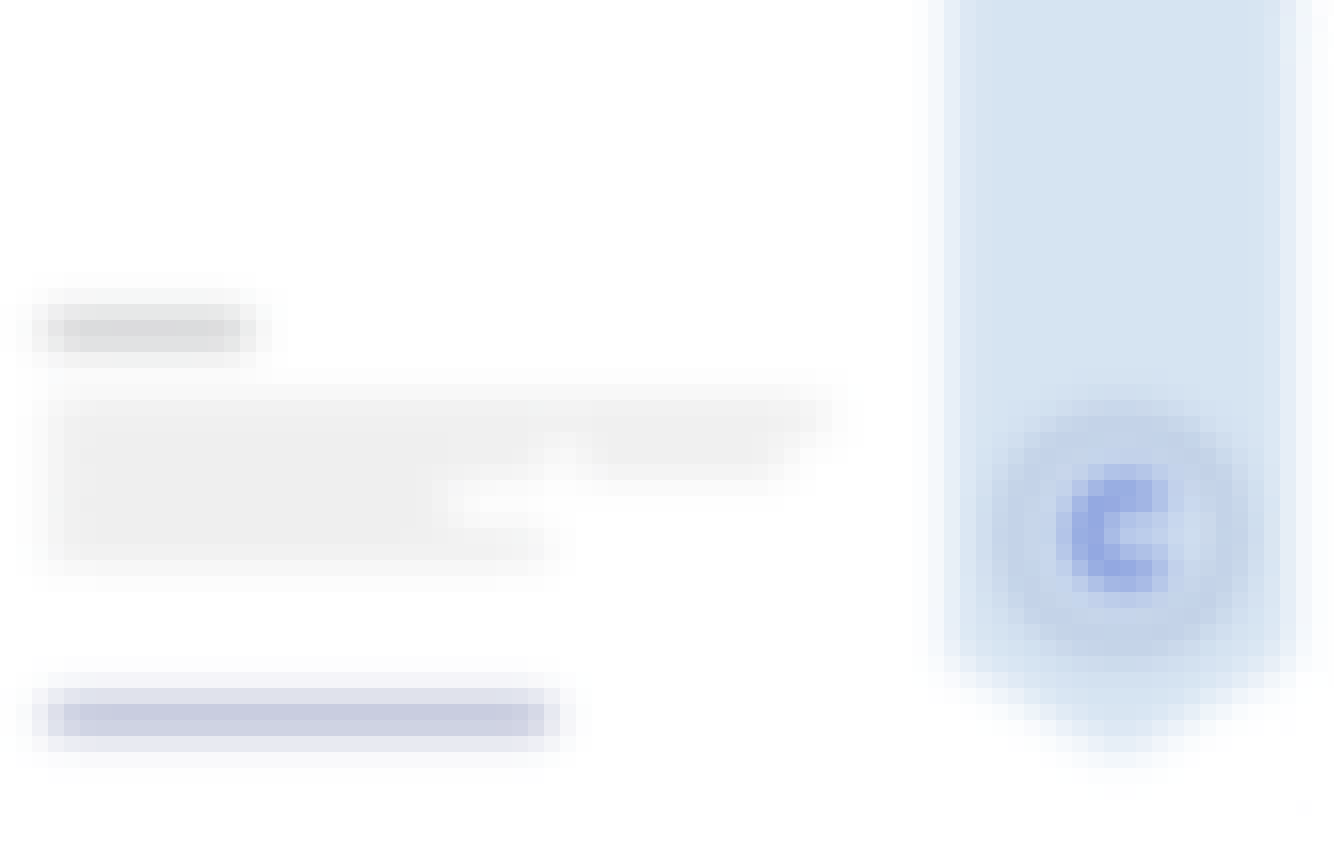
Earn a career certificate
Add this credential to your LinkedIn profile, resume, or CV
Share it on social media and in your performance review
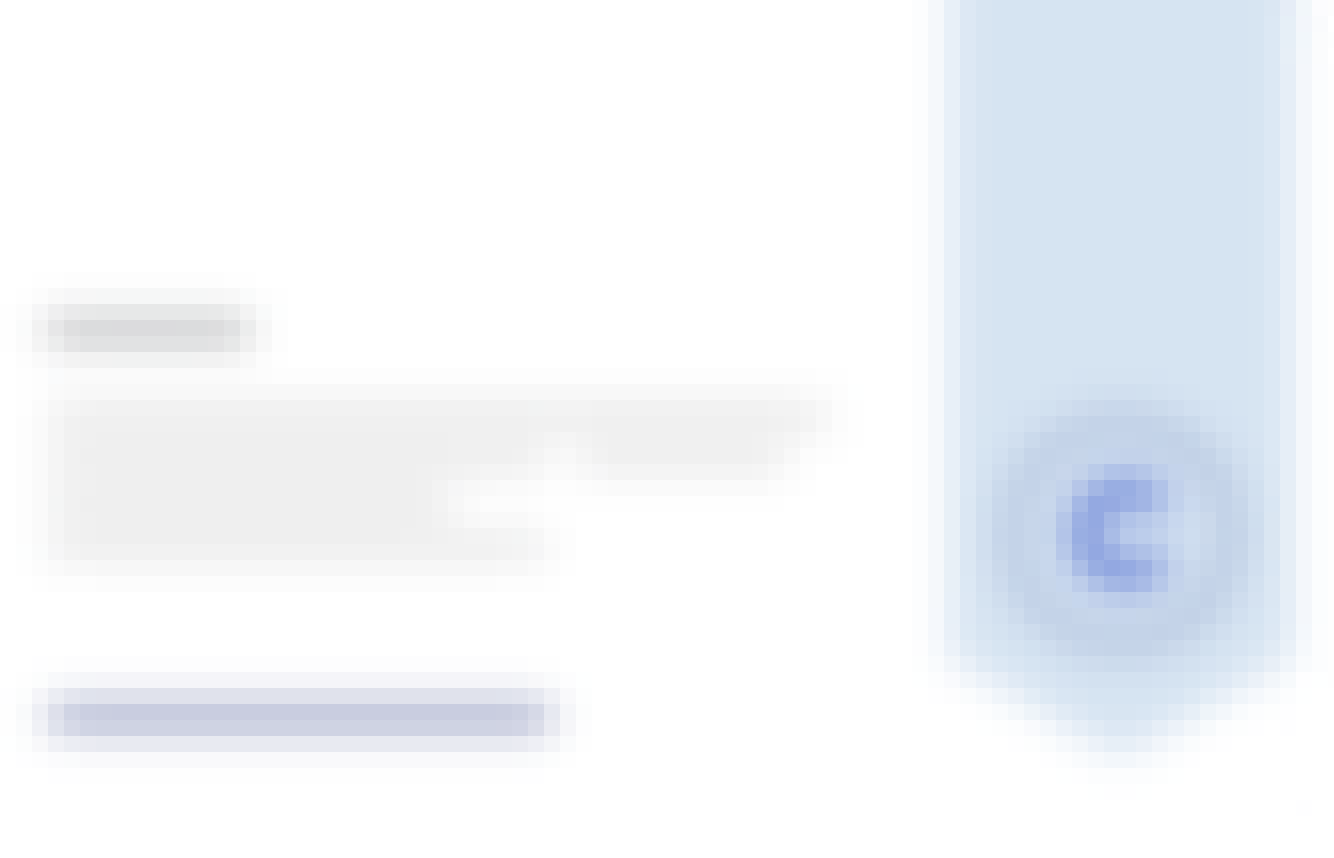
There are 6 modules in this course
The focus of this module is to introduce the concepts of machine learning with as little mathematics as possible. We will introduce basic concepts in machine learning, including logistic regression, a simple but widely employed machine learning (ML) method. Also covered is multilayered perceptron (MLP), a fundamental neural network. The concept of deep learning is discussed, and also related to simpler models.
What's included
23 videos2 readings10 assignments3 ungraded labs
In this module we will be discussing the mathematical basis of learning deep networks. We’ll first work through how we define the issue of learning deep networks as a minimization problem of a mathematical function. After defining our mathematical goal, we will introduce validation methods to estimate real-world performance of the learned deep networks. We will then discuss how gradient descent, a classical technique in optimization, can be used to achieve this mathematical goal. Finally, we will discuss both why and how stochastic gradient descent is used in practice to learn deep networks.
What's included
6 videos3 assignments2 ungraded labs
This week will cover model training, as well as transfer learning and fine-tuning. In addition to learning the fundamentals of a CNN and how it is applied, careful discussion is provided on the intuition of the CNN, with the goal of providing a conceptual understanding.
What's included
8 videos4 assignments2 ungraded labs
This week will cover the application of neural networks to natural language processing (NLP), from simple neural models to the more complex. The fundamental concept of word embeddings is discussed, as well as how such methods are employed within model learning and usage for several NLP applications. A wide range of neural NLP models are also discussed, including recurrent neural networks, and specifically long short-term memory (LSTM) models.
What's included
13 videos4 assignments2 ungraded labs
This week we'll cover an Introduction to the Transformer Network, a deep machine learning model designed to be more flexible and robust than Recurrent Neural Network (RNN). We'll start by reviewing several machine learning building blocks of a Transformer Network: the Inner products of word vectors, attention mechanisms, and sequence-to-sequence encoders and decoders. Then, we'll put all of these components together to explore the complete Transformer Network.
What's included
12 videos
This week will cover Reinforcement Learning, a fundamental concept in machine learning that is concerned with taking suitable actions to maximize rewards in a particular situation. After learning the initial steps of Reinforcement Learning, we'll move to Q Learning, as well as Deep Q Learning. We'll discuss the difference between the concepts of Exploration and Exploitation and why they are important.
What's included
10 videos3 assignments2 ungraded labs
Instructors



Offered by
Why people choose Coursera for their career




Learner reviews
3,640 reviews
- 5 stars
74.69%
- 4 stars
20.54%
- 3 stars
2.88%
- 2 stars
0.65%
- 1 star
1.20%
Showing 3 of 3640
Reviewed on Jul 8, 2019
Very good introductory course, I highly recommend it to anyone looking to get a flavour of the methods behind the recent advances in AI without going into super-technical details.
Reviewed on Apr 27, 2021
Its really a helpful course to my career. I got to learn various things about machine learning from this course all thanks to Coursera. A valuable course for every machine learning aspirant.
Reviewed on Jun 26, 2021
Thanks to Coursera I now know the basic machine learning models as well as how I can implement them to solve real world problems. Excellent instructors and learning resources!
Recommended if you're interested in Data Science
Google Cloud
Alberta Machine Intelligence Institute

Open new doors with Coursera Plus
Unlimited access to 10,000+ world-class courses, hands-on projects, and job-ready certificate programs - all included in your subscription
Advance your career with an online degree
Earn a degree from world-class universities - 100% online
Join over 3,400 global companies that choose Coursera for Business
Upskill your employees to excel in the digital economy