In the final course of the statistical modeling for data science program, learners will study a broad set of more advanced statistical modeling tools. Such tools will include generalized linear models (GLMs), which will provide an introduction to classification (through logistic regression); nonparametric modeling, including kernel estimators, smoothing splines; and semi-parametric generalized additive models (GAMs). Emphasis will be placed on a firm conceptual understanding of these tools. Attention will also be given to ethical issues raised by using complicated statistical models.

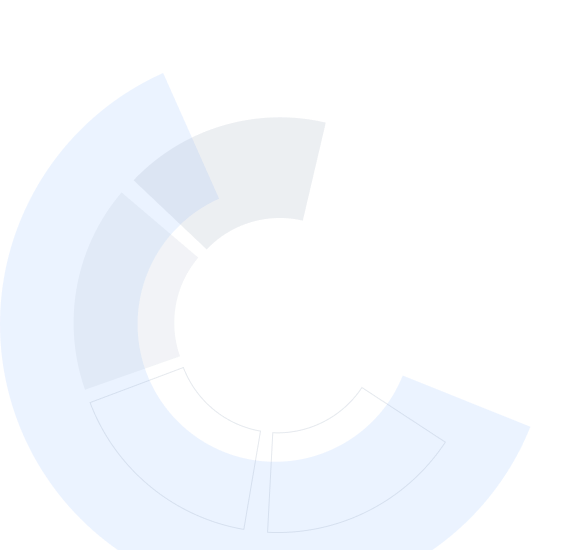
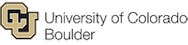
Generalized Linear Models and Nonparametric Regression
This course is part of Statistical Modeling for Data Science Applications Specialization

Instructor: Brian Zaharatos
Sponsored by ITC-Infotech
4,329 already enrolled
(20 reviews)
Recommended experience
What you'll learn
Describe how to generalize the linear model framework to accommodate data that is not suitable for the standard linear regression model.
State some advantages and disadvantages of (generalized) additive models.
Describe how an additive model can be generalized to incorporate non-normal response variables (i.e., define a generalized additive model).
Skills you'll gain
- Data Analysis
- Regression Analysis
- Statistical Programming
- Statistical Modeling
- Mathematical Modeling
- Data Science
- Probability & Statistics
- Statistical Inference
- R Programming
- Applied Mathematics
- Machine Learning
- Machine Learning Methods
- Mathematics and Mathematical Modeling
- Business Analytics
- Applied Machine Learning
- Statistical Analysis
- Statistics
- Statistical Machine Learning
- Statistical Methods
- Analytics
Details to know

Add to your LinkedIn profile
8 assignments
See how employees at top companies are mastering in-demand skills
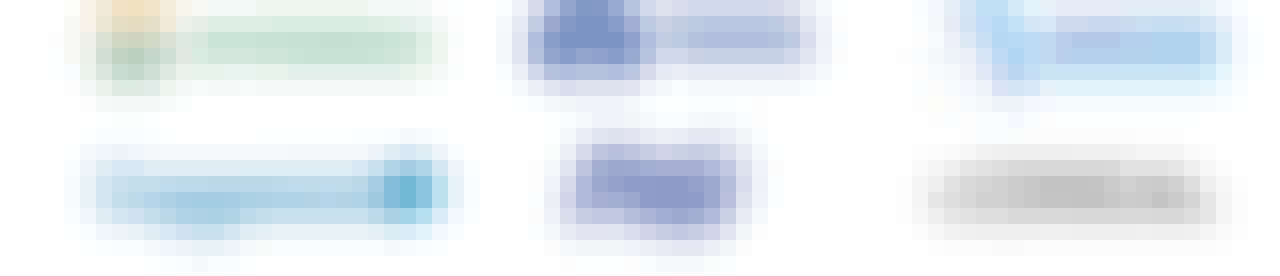
Build your subject-matter expertise
- Learn new concepts from industry experts
- Gain a foundational understanding of a subject or tool
- Develop job-relevant skills with hands-on projects
- Earn a shareable career certificate
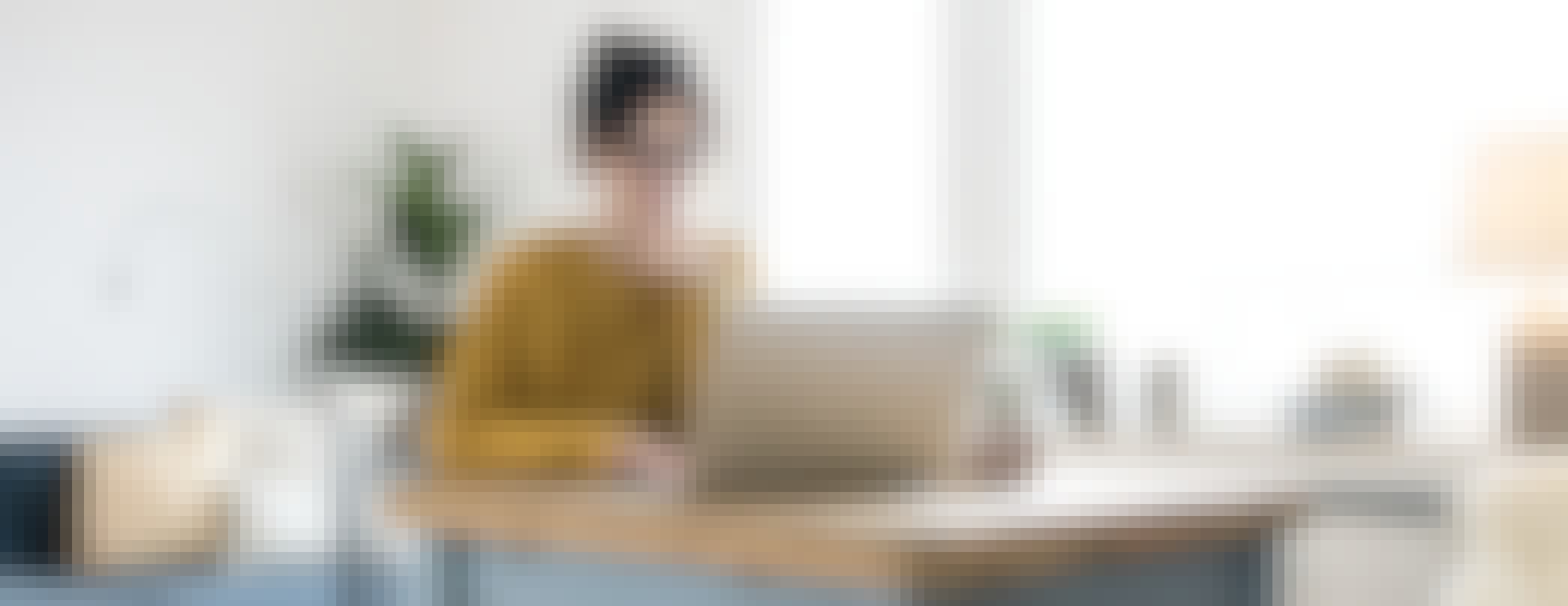
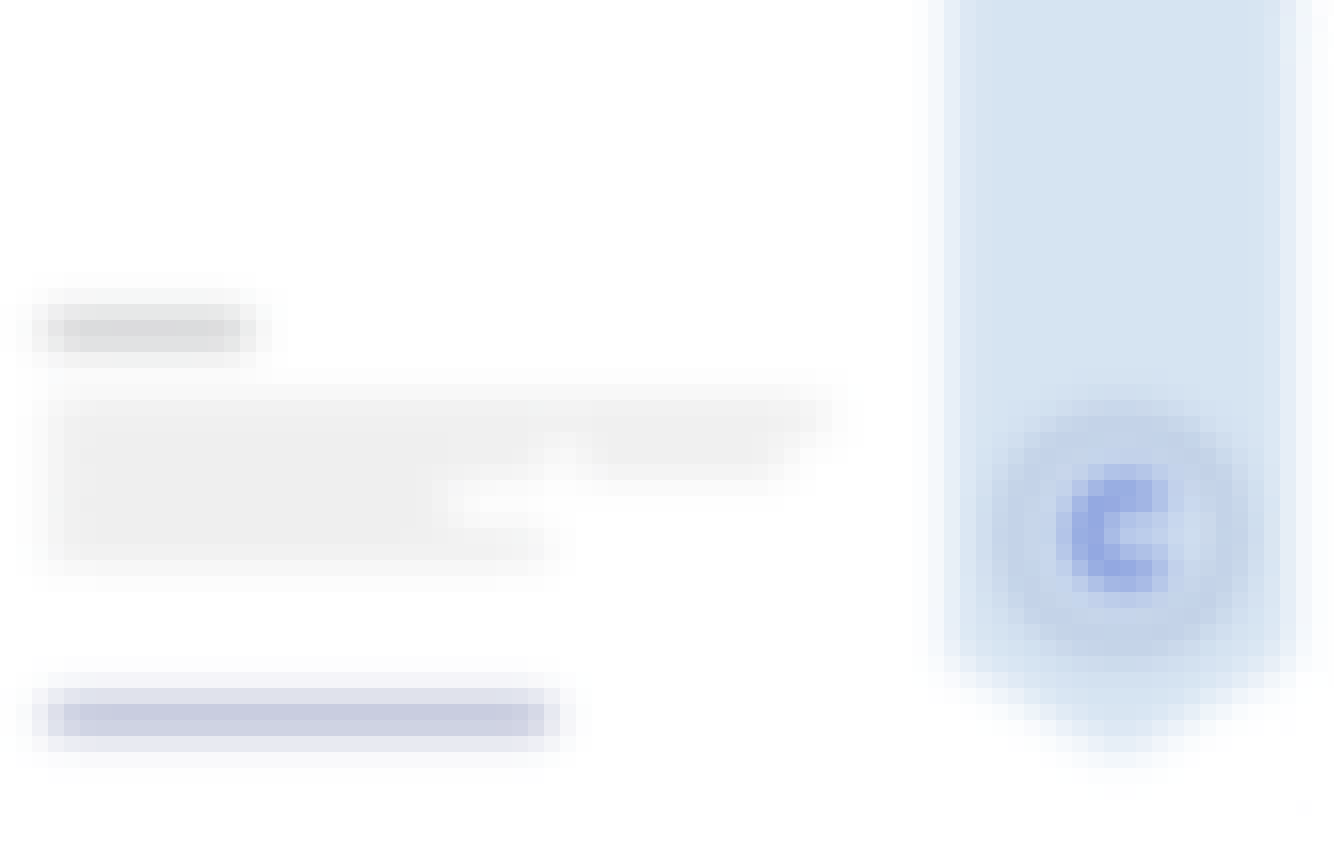
Earn a career certificate
Add this credential to your LinkedIn profile, resume, or CV
Share it on social media and in your performance review
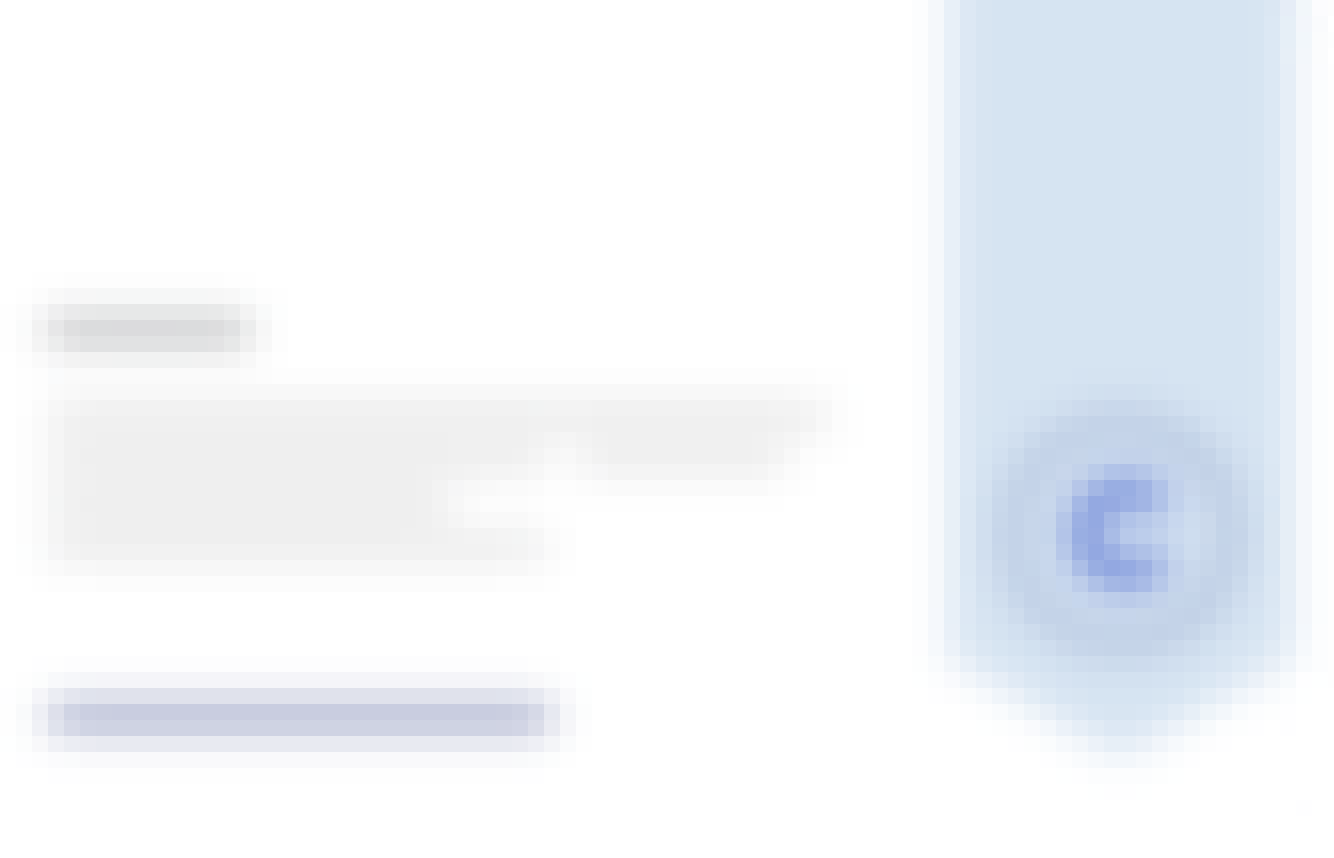
There are 4 modules in this course
In this module, we will introduce generalized linear models (GLMs) through the study of binomial data. In particular, we will motivate the need for GLMs; introduce the binomial regression model, including the most common binomial link functions; correctly interpret the binomial regression model; and consider various methods for assessing the fit and predictive power of the binomial regression model.
What's included
7 videos4 readings3 assignments2 programming assignments2 peer reviews1 discussion prompt2 ungraded labs
In this module, we will consider how to model count data. When the response variable is a count of some phenomenon, and when that count is thought to depend on a set of predictors, we can use Poisson regression as a model. We will describe the Poisson regression in some detail and use Poisson regression on real data. Then, we will describe situations in which Poisson regression is not appropriate, and briefly present solutions to those situations.
What's included
7 videos2 assignments1 programming assignment1 peer review3 ungraded labs
In this module, we will introduce the concept of a nonparametric regression model. We will contrast this notion with the parametric models that we have studied so far. Then, we’ll study particular nonparametric regression models: kernel estimators and splines. Finally, we will introduce additive models as a blending of parametric and nonparametric methods.
What's included
6 videos1 assignment1 programming assignment1 peer review3 ungraded labs
Some models, such as linear regression, are easily interpretable, but inflexible, in that they don't capture many real-world relationships accurately. Other models, such as neural networks, are quite flexible, but very difficult to interpret. Generalized additive models (GAMs) are a nice balance between flexibility and interpretability. In this module, we will further motivate GAMs, learn the basic mathematics of fitting GAMs, and implementing them on simulated and real data in R.
What's included
6 videos1 reading2 assignments1 programming assignment1 peer review3 ungraded labs
Instructor

Offered by
Why people choose Coursera for their career




Learner reviews
20 reviews
- 5 stars
75%
- 4 stars
0%
- 3 stars
10%
- 2 stars
5%
- 1 star
10%
Showing 3 of 20
Reviewed on Jun 27, 2023
The pace of instruction is excellent and the assignments make it easy to translate theory to practice.
Recommended if you're interested in Data Science
DeepLearning.AI
Coursera Project Network
University of Colorado Boulder
University of Colorado Boulder

Open new doors with Coursera Plus
Unlimited access to 10,000+ world-class courses, hands-on projects, and job-ready certificate programs - all included in your subscription
Advance your career with an online degree
Earn a degree from world-class universities - 100% online
Join over 3,400 global companies that choose Coursera for Business
Upskill your employees to excel in the digital economy