Introduction to Computer Vision guides learners through the essential algorithms and methods to help computers 'see' and interpret visual data. You will first learn the core concepts and techniques that have been traditionally used to analyze images. Then, you will learn modern deep learning methods, such as neural networks and specific models designed for image recognition, and how it can be used to perform more complex tasks like object detection and image segmentation. Additionally, you will learn the creation and impact of AI-generated images and videos, exploring the ethical considerations of such technology.

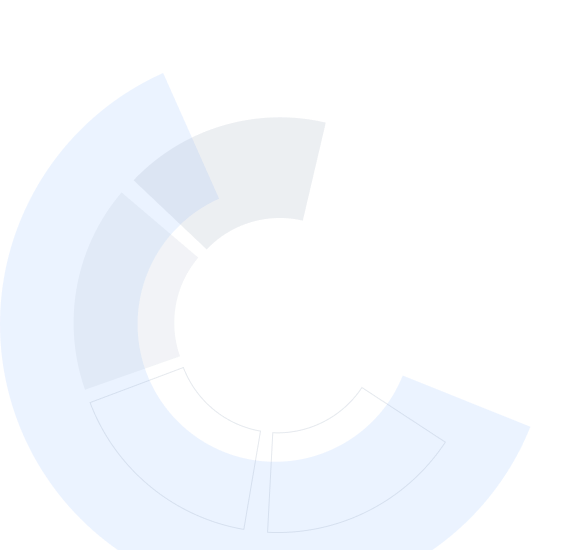
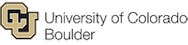
Recommended experience
What you'll learn
Understand the fundamental principles and algorithms of classical computer vision.
Apply deep learning models to various computer vision tasks.
Evaluate and implement computer vision solutions for real-world applications.
Details to know

Add to your LinkedIn profile
26 assignments
See how employees at top companies are mastering in-demand skills
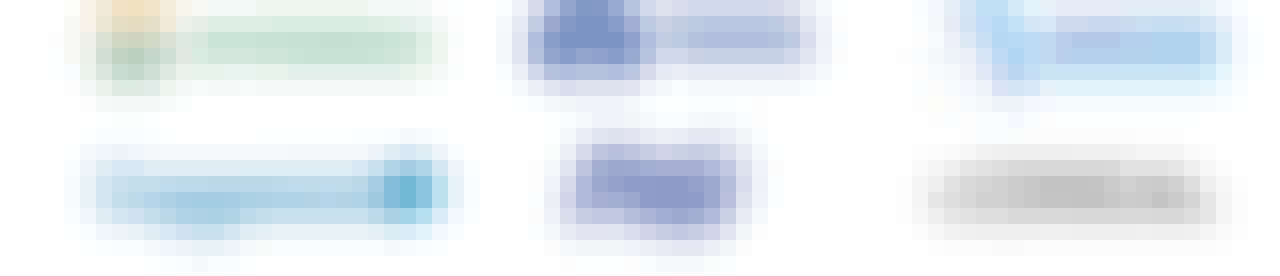
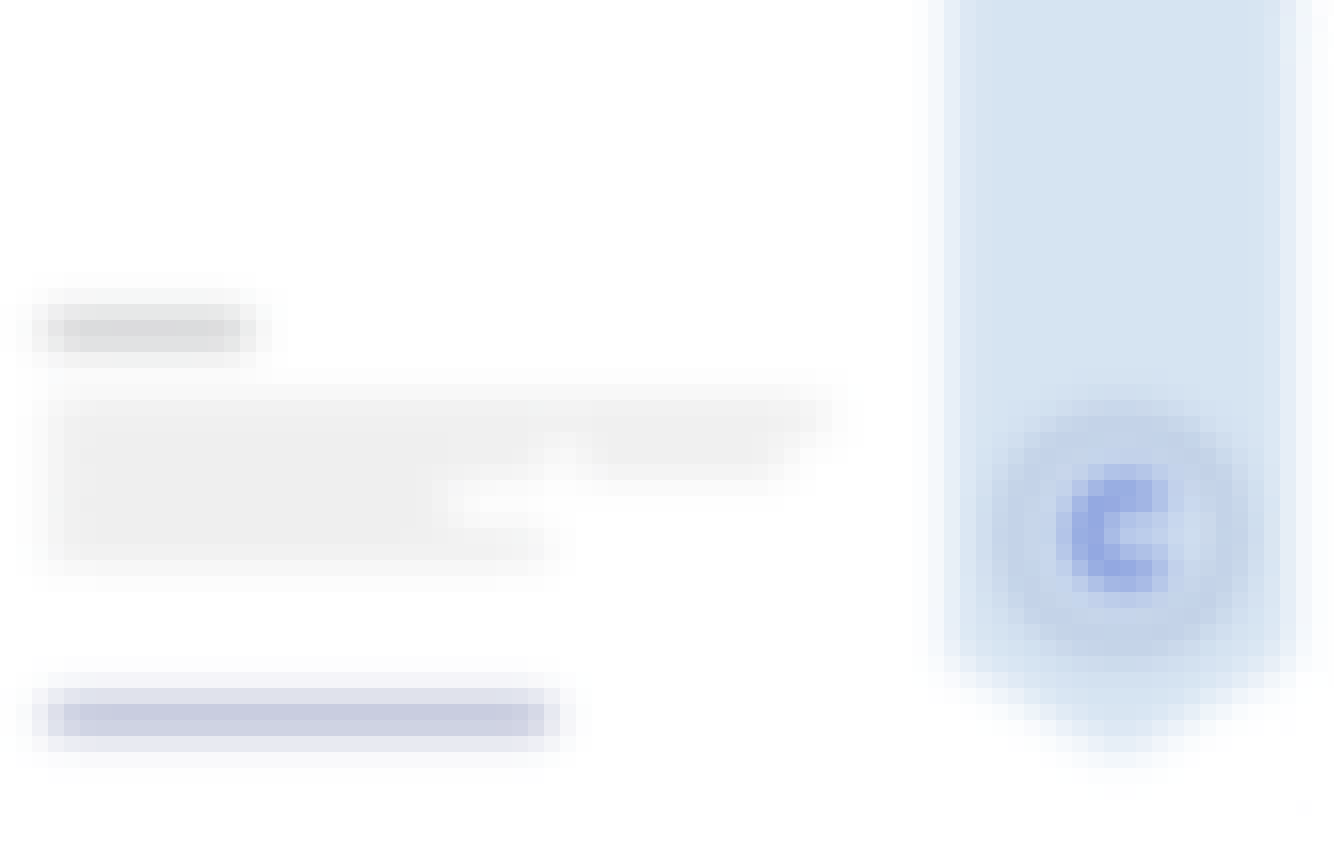
Earn a career certificate
Add this credential to your LinkedIn profile, resume, or CV
Share it on social media and in your performance review
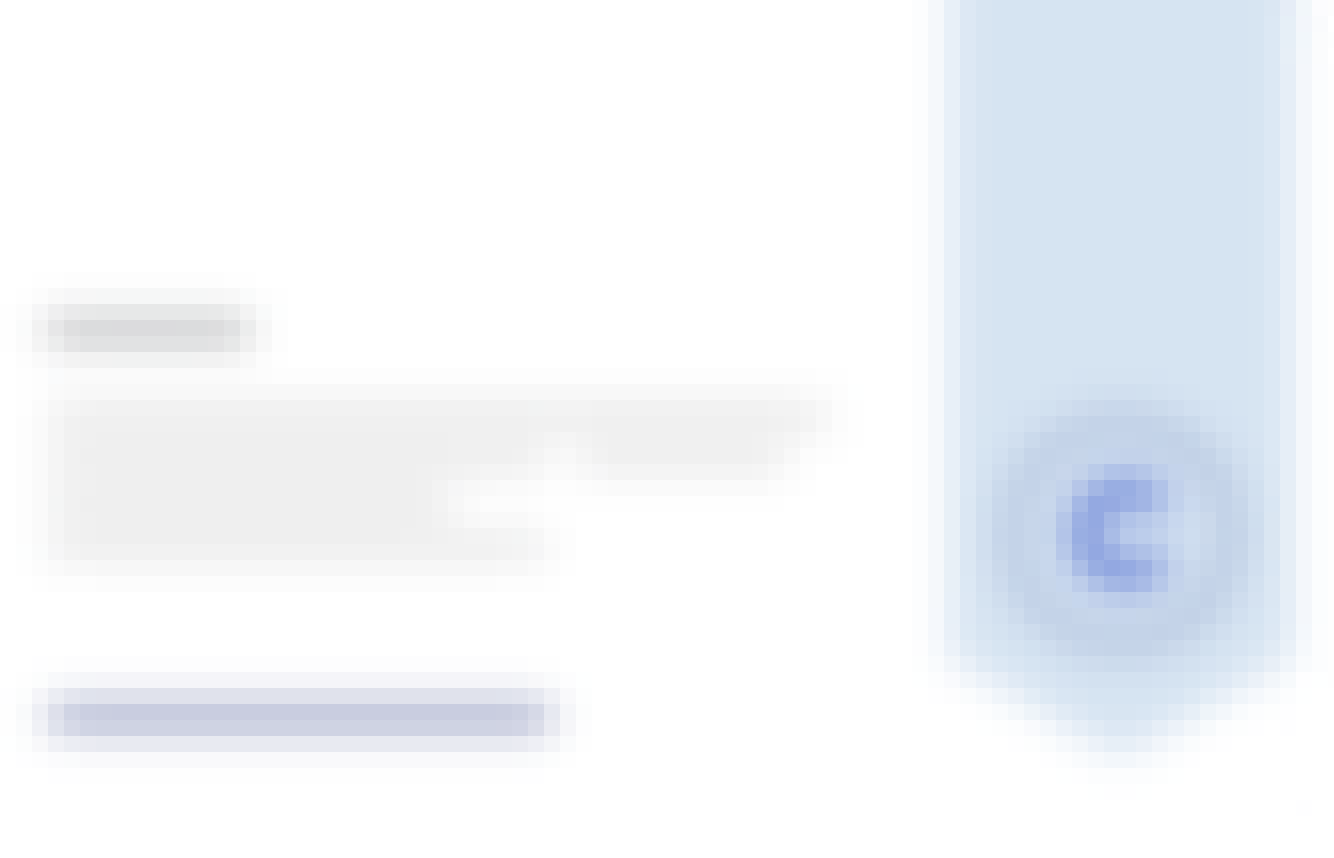
There are 4 modules in this course
This module introduces foundational concepts related to common image types and functions. It offers a comprehensive overview of different formats and their unique characteristics. This section establishes the context for understanding how images are represented and processed in various applications. Next, the module delves into image functions, explaining the basic operations that can be performed on images to enhance or manipulate them, such as cropping, resizing, or adjusting brightness. It also covers more advanced operations like filtering and thresholding, illustrating how these functions play a crucial role in image processing. Then the module explores the underlying mathematics of image transformations. It starts with linear transforms, highlighting their application in image scaling, rotation, and translation. The module then introduces homogeneous coordinates, providing a simplified approach to represent complex transformations with additional dimensions. This leads into a deeper exploration of homogeneous transformations, demonstrating how they are used to perform multiple transformations in a single step.
What's included
7 videos4 readings5 assignments
This module provides a deep dive into image analysis and similarity assessment techniques. It starts by exploring the basic concept of comparing pixels, highlighting how individual pixel values can be used to gauge similarity. This is followed by a detailed discussion on comparing multiple images by their features, emphasizing the advantages of feature-based analysis over pixel-by-pixel comparison. The module introduces the concept of image moments, revealing how these statistical properties help identify shapes and patterns within images. The module then addresses similarity and distance, offering a quick overview of how these concepts are calculated and applied in image processing. You'll also learn about converting pixels into distributions, an essential technique for more complex analysis. This leads to a comprehensive explanation of cross-entropy, providing insights into its role in measuring the dissimilarity between distributions. You'll explore cross-correlation in 1D, followed by a deeper examination of cross-correlation as matrix multiplication. The module wraps up by exploring cross-correlation in more detail, with a focus on the mathematics behind it.
What's included
10 videos9 assignments
This module delves into multiview geometry, a pivotal concept in computer vision and 3D modeling. It starts with a brief overview of the motivation behind multiview systems, highlighting the advantages of capturing scenes from multiple viewpoints. The module then discusses multiple coordinate systems, exploring how different reference frames can describe points and transformations in 3D space. You'll also learn about multiple viewing planes, which play a crucial role in multiview setups by providing unique perspectives for scene reconstruction. The focus shifts to multiview projection, examining how distinct images from multiple cameras can be used to create a cohesive 3D scene. You'll gain insights into the principles of translation and rotation in 3D, crucial for understanding camera movement and orientation. The module also covers camera translation and camera rotation, offering practical examples to illustrate how camera motion affects the geometry and visual representation of a scene.
What's included
8 videos6 assignments
This module delves into key concepts of camera models and their role in computer vision and photogrammetry. Learn about the Extrinsic Matrix, exploring how it defines the position and orientation of a camera in 3D space. Understand the Pinhole Camera Model, a simplified optical system that forms the basis for many computer vision applications, alongside the Intrinsic Matrix, which captures the internal parameters of the camera. Epipolar geometry is examined, with a focus on its significance in 3D reconstruction and stereo vision. The module covers the motivation behind epipolar geometry, breaking down its basic components, and explaining the Essential Matrix, which encapsulates the geometric relationship between camera views, as well as the Fundamental Matrix, a core component in epipolar geometry that represents the relationship between two cameras in stereo vision.
What's included
6 videos6 assignments
Instructor

Offered by
Why people choose Coursera for their career




Recommended if you're interested in Computer Science
Coursera Instructor Network
Amazon Web Services
LearnQuest
Birla Institute of Technology & Science, Pilani

Open new doors with Coursera Plus
Unlimited access to 10,000+ world-class courses, hands-on projects, and job-ready certificate programs - all included in your subscription
Advance your career with an online degree
Earn a degree from world-class universities - 100% online
Join over 3,400 global companies that choose Coursera for Business
Upskill your employees to excel in the digital economy