"Trees, SVM and Unsupervised Learning" is designed to provide working professionals with a solid foundation in support vector machines, neural networks, decision trees, and XG boost. Through in-depth instruction and practical hands-on experience, you will learn how to build powerful predictive models using these techniques and understand the advantages and disadvantages of each. The course will also cover how and when to apply them to different scenarios, including binary classification and K > 2 classes. Additionally, you will gain valuable experience in generating data representations through PCA and clustering. With a focus on practical, real-world applications, this course is a valuable asset for anyone looking to upskill or move into the field of data science.

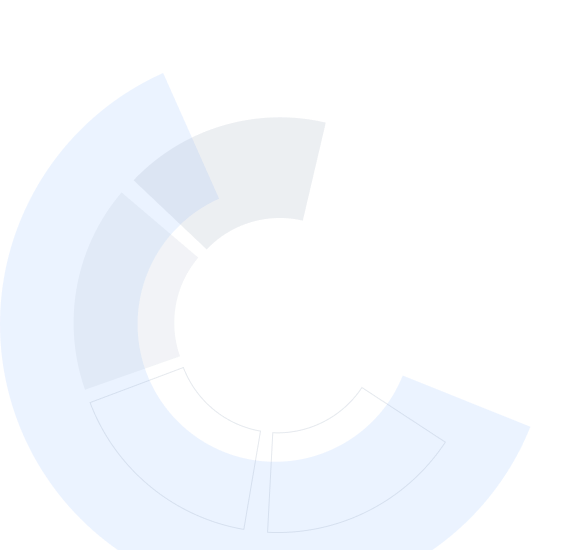
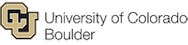
Trees, SVM and Unsupervised Learning
This course is part of Statistical Learning for Data Science Specialization

Instructor: Osita Onyejekwe
Sponsored by BrightStar Care
Recommended experience
What you'll learn
Describe the advantages and disadvantages of trees, and how and when to use them.
Apply SVMs for binary classification or K > 2 classes.
Analyze the strengths and weaknesses of neural networks compared to other machine learning algorithms, such as SVMs.
Skills you'll gain
- Artificial Intelligence and Machine Learning (AI/ML)
- Deep Learning
- Statistical Modeling
- Artificial Neural Networks
- Supervised Learning
- Machine Learning Methods
- Statistical Machine Learning
- Machine Learning
- Advanced Analytics
- Predictive Analytics
- Machine Learning Algorithms
- Random Forest Algorithm
- Data Science
- Predictive Modeling
- Computer Science
- Unsupervised Learning
- Artificial Intelligence
- Applied Machine Learning
- Decision Tree Learning
- Classification And Regression Tree (CART)
Details to know

Add to your LinkedIn profile
See how employees at top companies are mastering in-demand skills
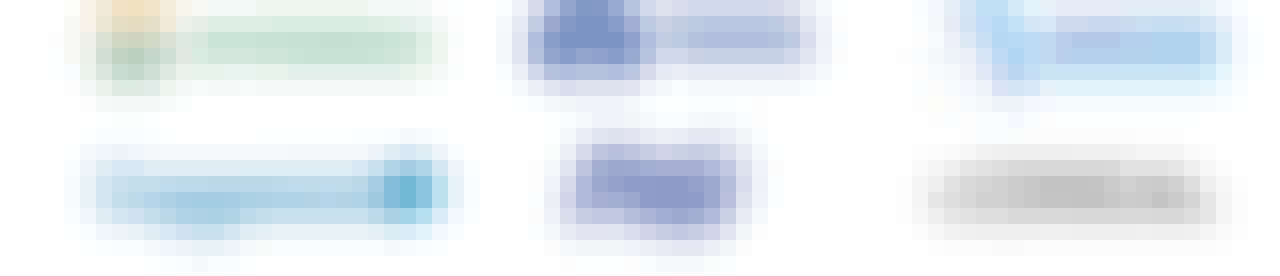
Build your subject-matter expertise
- Learn new concepts from industry experts
- Gain a foundational understanding of a subject or tool
- Develop job-relevant skills with hands-on projects
- Earn a shareable career certificate
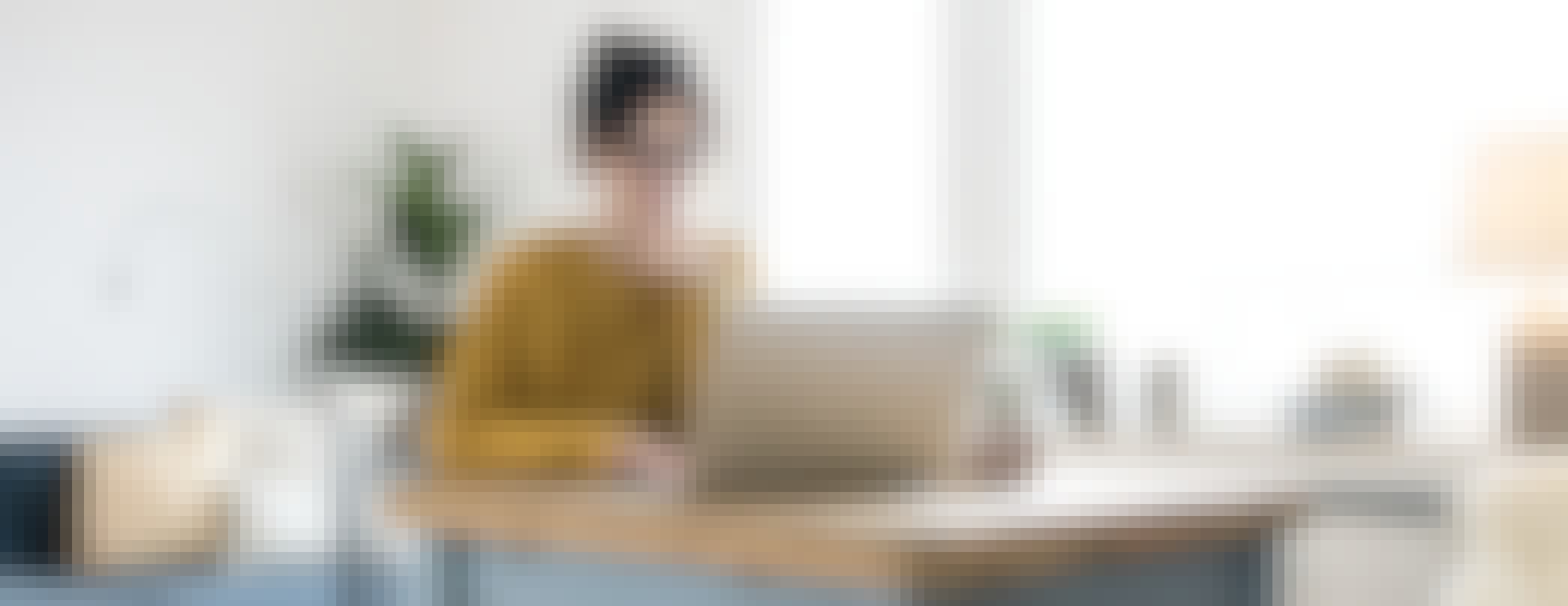
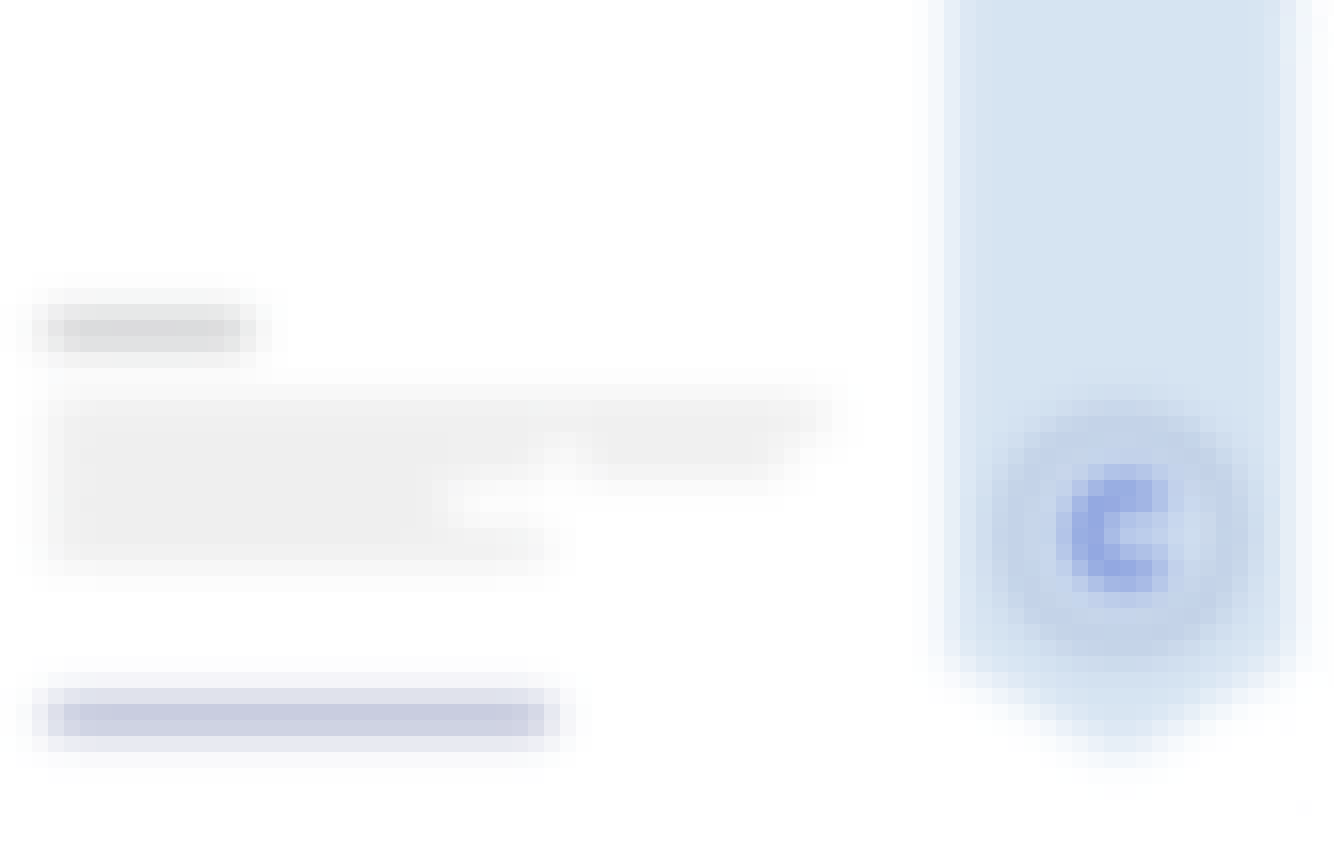
Earn a career certificate
Add this credential to your LinkedIn profile, resume, or CV
Share it on social media and in your performance review
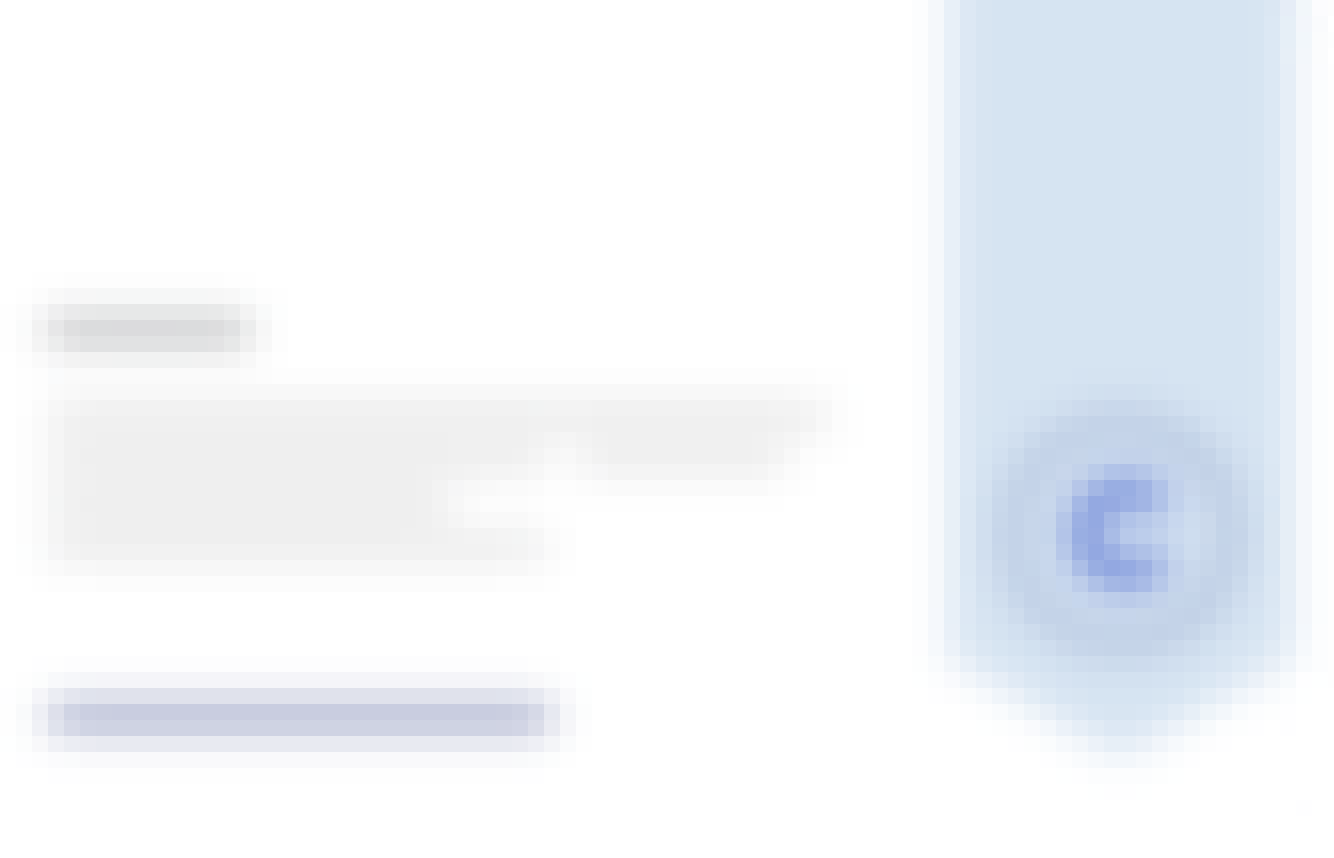
There are 4 modules in this course
The module provides an introductory overview of the course and introduces the course instructor.
What's included
1 video2 readings1 discussion prompt
To begin the course, we will learn about support vector machines (SVMs). SVMs have become a popular method in the field of statistical learning due to their ability to handle non-linear and high-dimensional data. SVMs seek to maximize the margin, or distance between the decision boundary and the closest data points, to improve generalization performance. Throughout the week, you will learn how to apply SVMs to classify or predict outcomes in a given dataset, select appropriate kernel functions and parameters, and evaluate model performance
What's included
4 videos1 reading1 programming assignment1 ungraded lab
Neural Networks have become increasingly popular in the field of statistical learning due to their ability to model complex relationships in data. In this module, we will cover introductory concepts of neural networks, such as activation functions and backpropagation. You will have the opportunity to apply Neural Networks to classify or predict outcomes in a given dataset and evaluate model performance in the labs for this module.
What's included
5 videos1 reading1 programming assignment
Welcome to the final module for the course. This module will focus on the ensemble methods decision trees, bagging, and random forests, which combine multiple models to improve prediction accuracy and reduce overfitting. Decision Trees are a popular machine learning method that partitions the feature space into smaller regions and models the response variable in each region using simple rules. However, Decision Trees can suffer from high variance and instability, which can be addressed by Bagging and Random Forests. Bagging involves generating multiple trees on bootstrapped samples of the data and averaging their predictions, while Random Forests further decorrelate the trees by randomly selecting subsets of features for each tree.
What's included
1 video1 reading1 programming assignment1 ungraded lab
Instructor

Offered by
Why people choose Coursera for their career




Recommended if you're interested in Data Science
University of Colorado Boulder
University of Colorado Boulder
University of Colorado Boulder

Open new doors with Coursera Plus
Unlimited access to 10,000+ world-class courses, hands-on projects, and job-ready certificate programs - all included in your subscription
Advance your career with an online degree
Earn a degree from world-class universities - 100% online
Join over 3,400 global companies that choose Coursera for Business
Upskill your employees to excel in the digital economy