Artificial Intelligence is revolutionizing data analysis. This course delves into Recurrent Neural Networks (RNNs), starting with basic memory models and advancing to deep RNN structures. You'll explore RNN models like ManyToMany, ManyToOne, and OneToMany through practical exercises, culminating in sentiment classification for sophisticated text analysis and prediction.

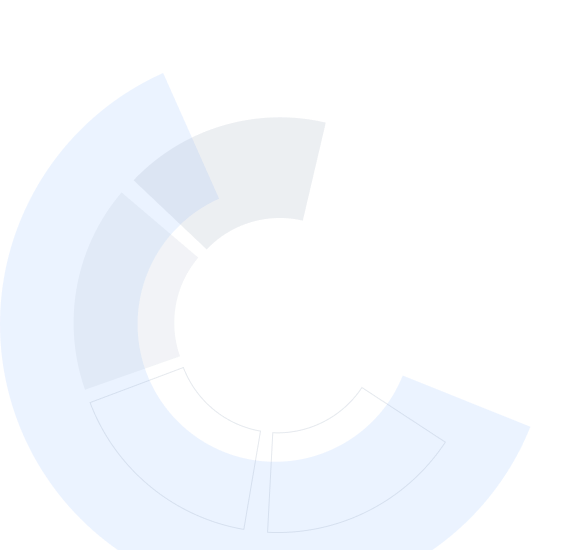
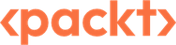
RNN Architecture and Sentiment Classification
This course is part of Deep Learning: Recurrent Neural Networks with Python Specialization

Instructor: Packt - Course Instructors
Sponsored by Coursera for Reliance Family
Recommended experience
What you'll learn
Identify different RNN architectures, including fixed-length and infinite memory models.
Examine the effectiveness of gradient descent and backpropagation through time in training RNN models.
Develop and apply RNN models for advanced tasks such as sentiment analysis and language modeling.
Skills you'll gain
- Machine Learning
- Artificial Intelligence and Machine Learning (AI/ML)
- Artificial Intelligence
- Natural Language Processing
- Network Planning And Design
- Network Architecture
- Artificial Neural Networks
- Applied Machine Learning
- Deep Learning
- Computer Networking
- PyTorch (Machine Learning Library)
- Machine Learning Methods
Details to know

Add to your LinkedIn profile
3 assignments
September 2024
See how employees at top companies are mastering in-demand skills
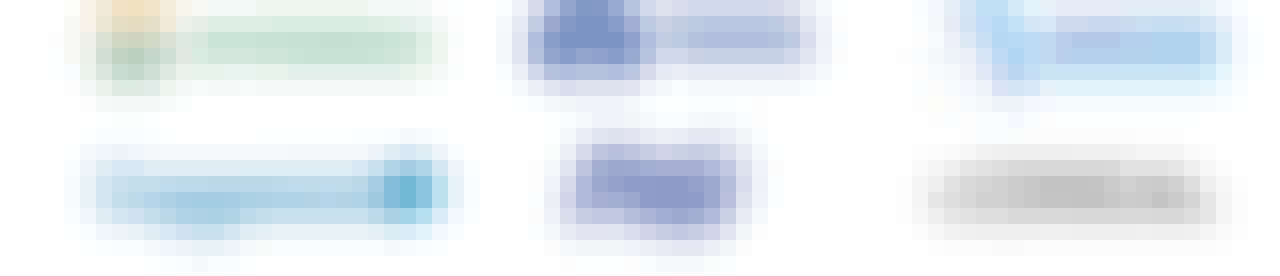
Build your subject-matter expertise
- Learn new concepts from industry experts
- Gain a foundational understanding of a subject or tool
- Develop job-relevant skills with hands-on projects
- Earn a shareable career certificate
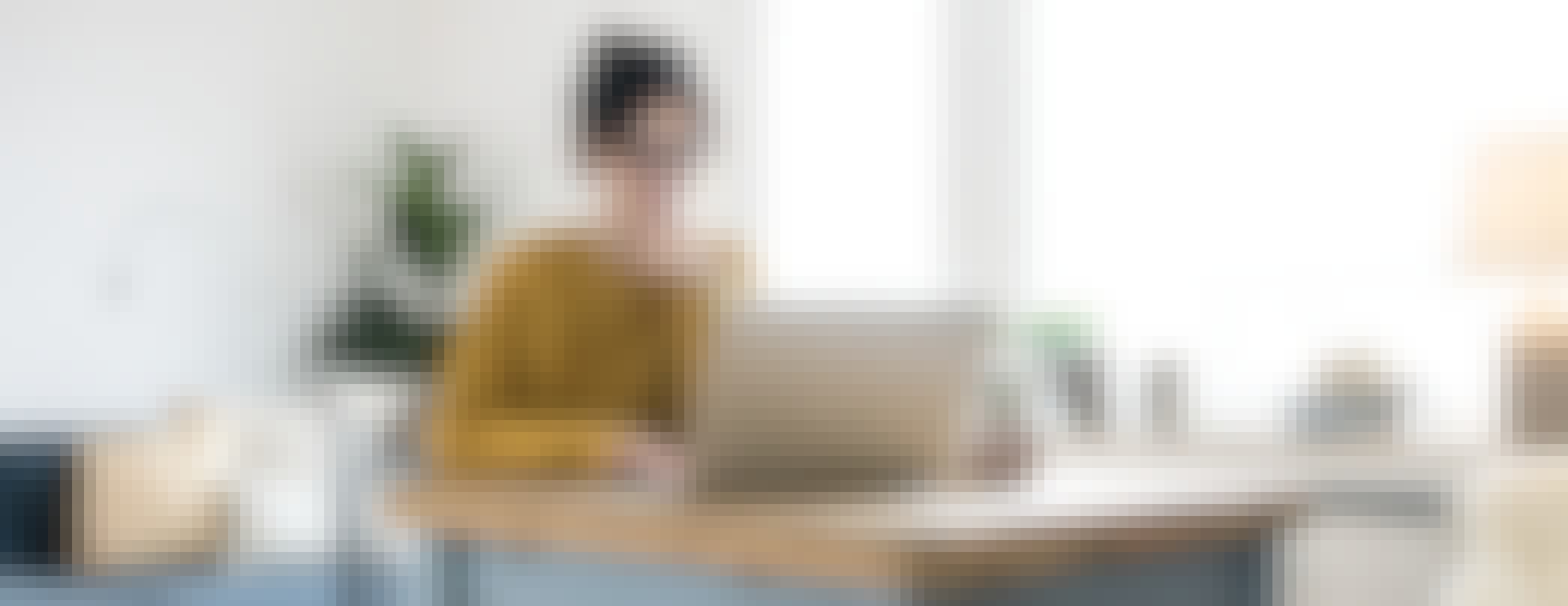
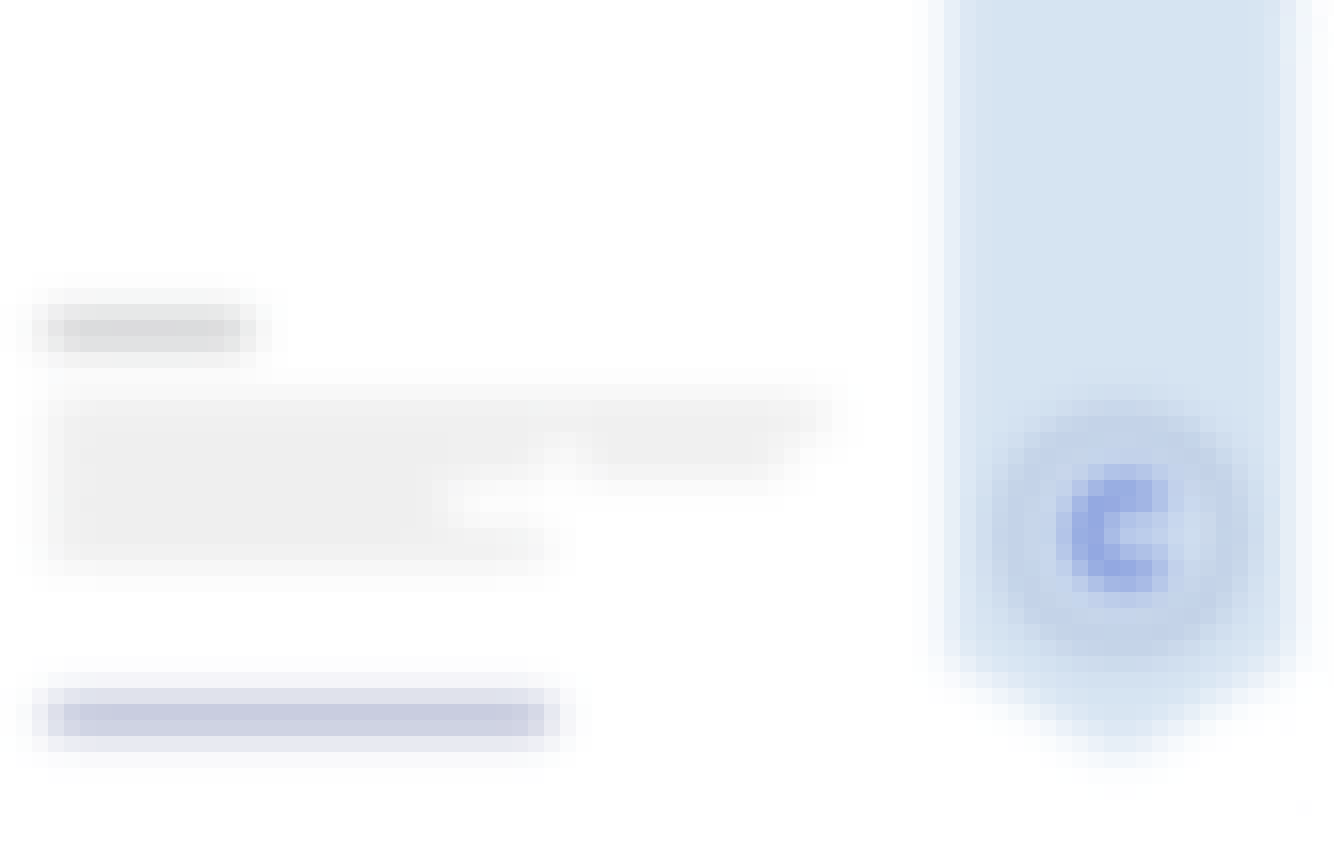
Earn a career certificate
Add this credential to your LinkedIn profile, resume, or CV
Share it on social media and in your performance review
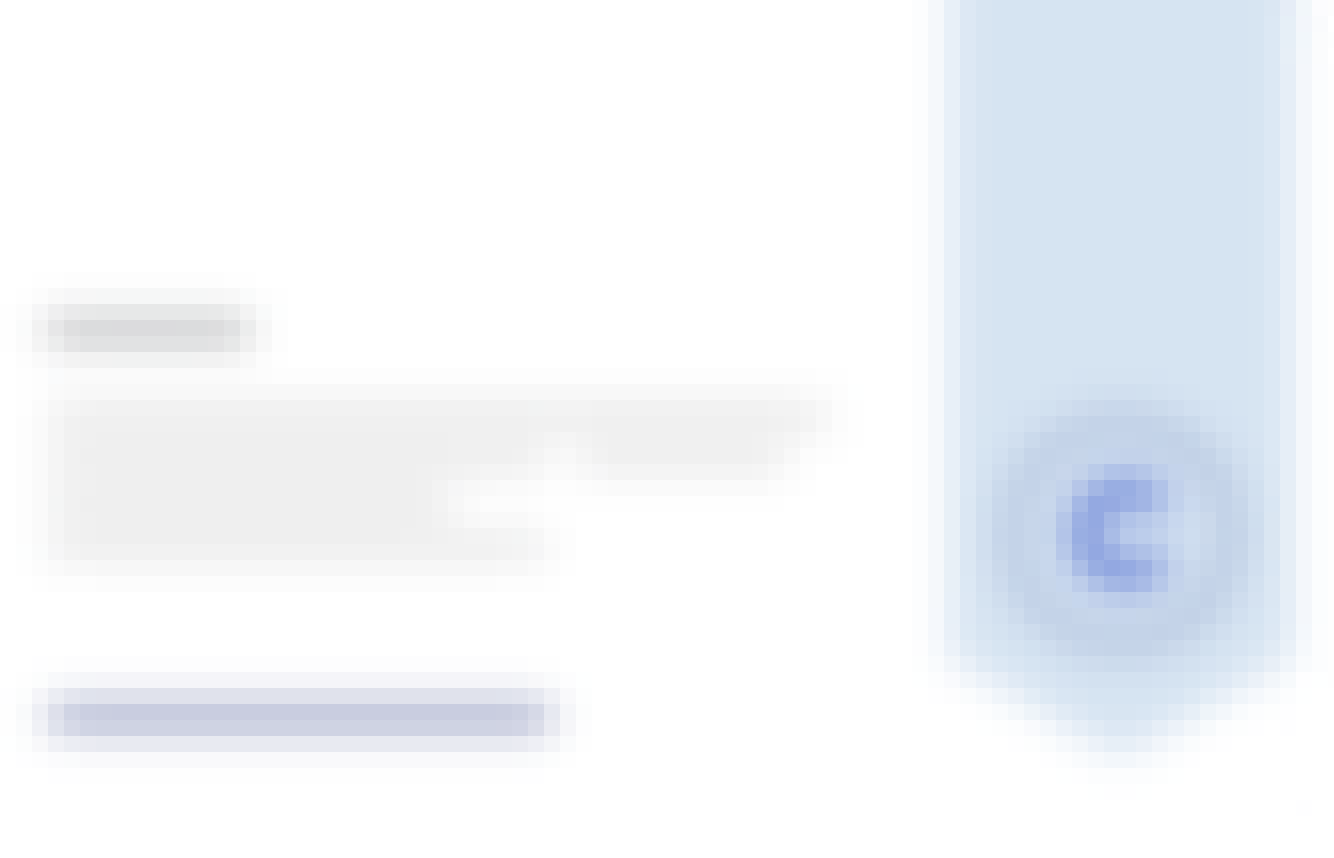
There are 4 modules in this course
In this module, we will explore the fundamental structures of Recurrent Neural Network (RNN) architectures. You'll learn about fixed length memory models, infinite memory architectures, and various model configurations such as Many-to-Many, Many-to-One, and One-to-Many. Through exercises and practical activities, you'll gain a deep understanding of these architectures and their applications.
What's included
30 videos2 readings
In this module, we will delve into the gradient descent algorithm as it applies to Recurrent Neural Networks. You'll learn the fundamental equations, understand the role of gradients, and apply the chain rule. Practical exercises and examples will illustrate backpropagation through time, ensuring a comprehensive grasp of these essential techniques.
What's included
13 videos1 assignment
In this module, we will focus on the practical implementation of RNNs. You'll learn about automatic differentiation in PyTorch, and apply RNNs to language modeling and next word prediction tasks. Through step-by-step coding exercises, you'll develop hands-on skills in building and training RNN models for language-related applications.
What's included
11 videos
In this module, we will tackle sentiment classification using Recurrent Neural Networks. You'll learn how to implement vocabulary and vectorizers, set up RNN models, and train them for sentiment analysis. Practical exercises will guide you through each step, ensuring you can effectively classify text data based on sentiment.
What's included
7 videos1 reading2 assignments
Instructor

Offered by
Why people choose Coursera for their career




Recommended if you're interested in Data Science

Open new doors with Coursera Plus
Unlimited access to 10,000+ world-class courses, hands-on projects, and job-ready certificate programs - all included in your subscription
Advance your career with an online degree
Earn a degree from world-class universities - 100% online
Join over 3,400 global companies that choose Coursera for Business
Upskill your employees to excel in the digital economy