This course will cover the steps used in weighting sample surveys, including methods for adjusting for nonresponse and using data external to the survey for calibration. Among the techniques discussed are adjustments using estimated response propensities, poststratification, raking, and general regression estimation. Alternative techniques for imputing values for missing items will be discussed. For both weighting and imputation, the capabilities of different statistical software packages will be covered, including R®, Stata®, and SAS®.

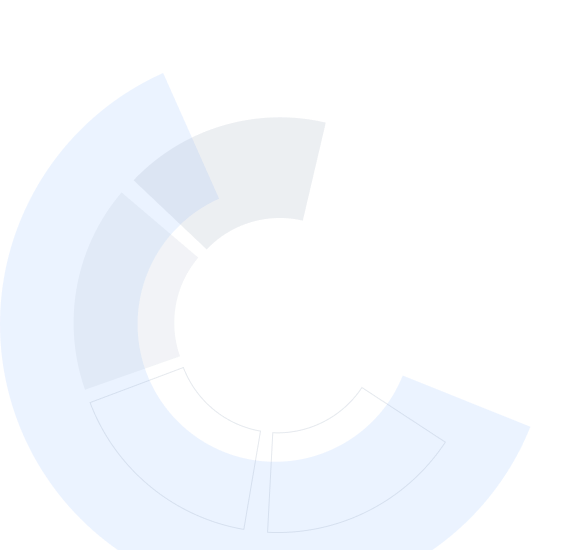
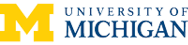
Dealing With Missing Data
This course is part of Survey Data Collection and Analytics Specialization

Instructor: Richard Valliant, Ph.D.
Sponsored by MAHE Manipal
11,735 already enrolled
(133 reviews)
Skills you'll gain
Details to know

Add to your LinkedIn profile
21 assignments
See how employees at top companies are mastering in-demand skills
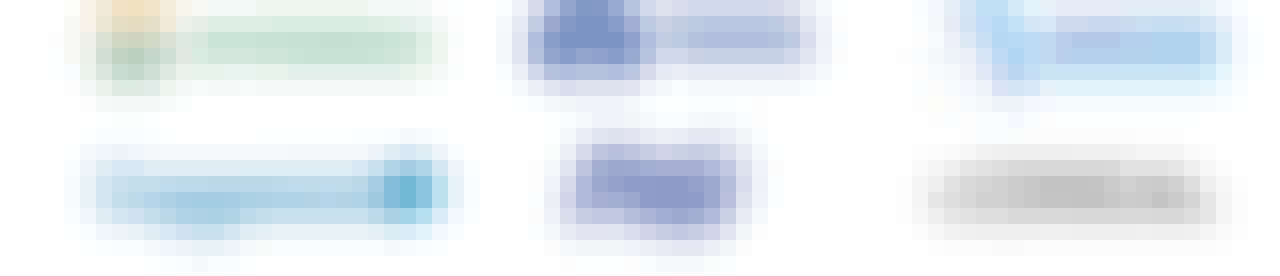
Build your subject-matter expertise
- Learn new concepts from industry experts
- Gain a foundational understanding of a subject or tool
- Develop job-relevant skills with hands-on projects
- Earn a shareable career certificate
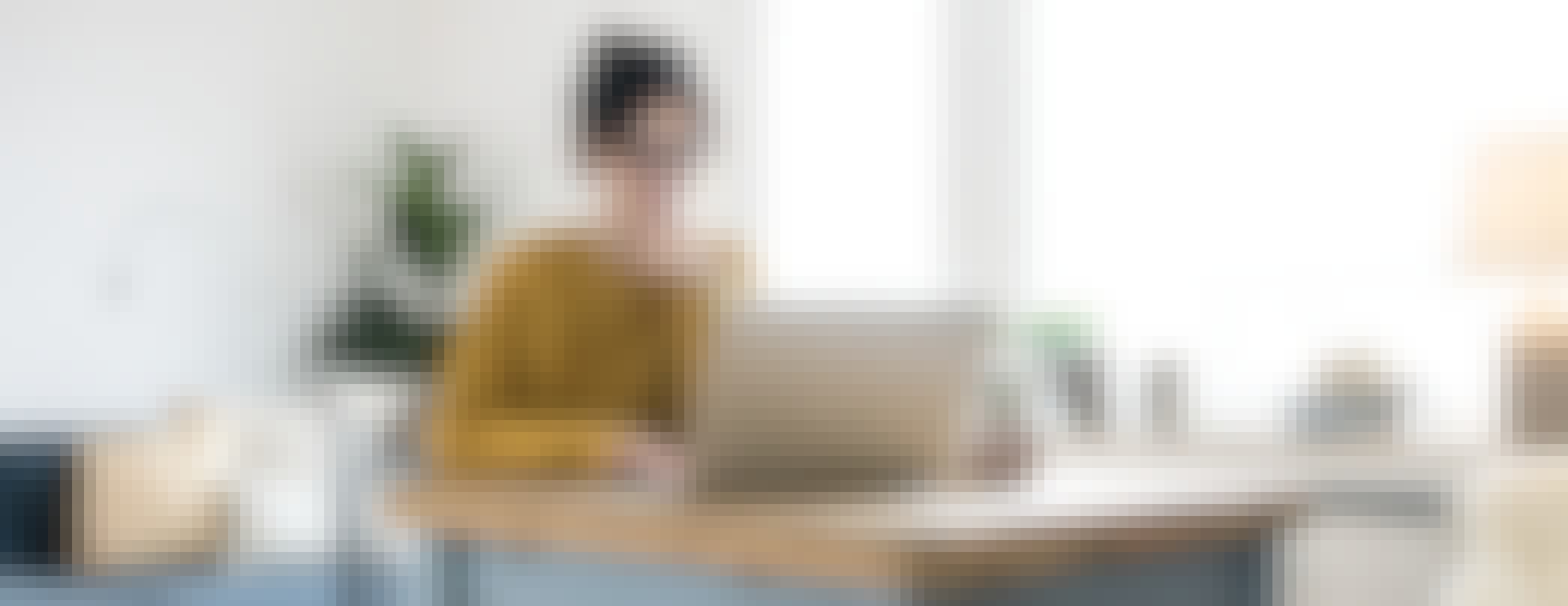
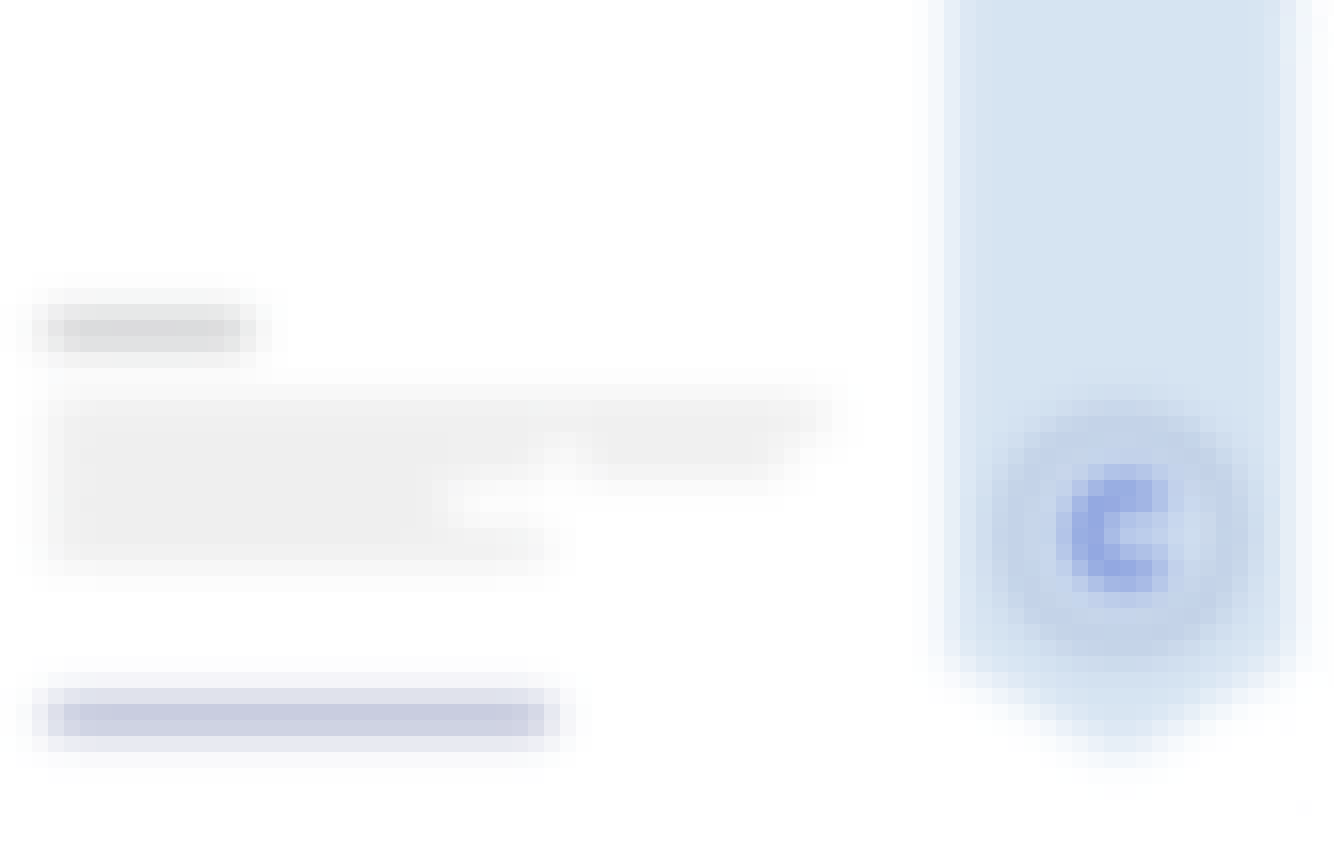
Earn a career certificate
Add this credential to your LinkedIn profile, resume, or CV
Share it on social media and in your performance review
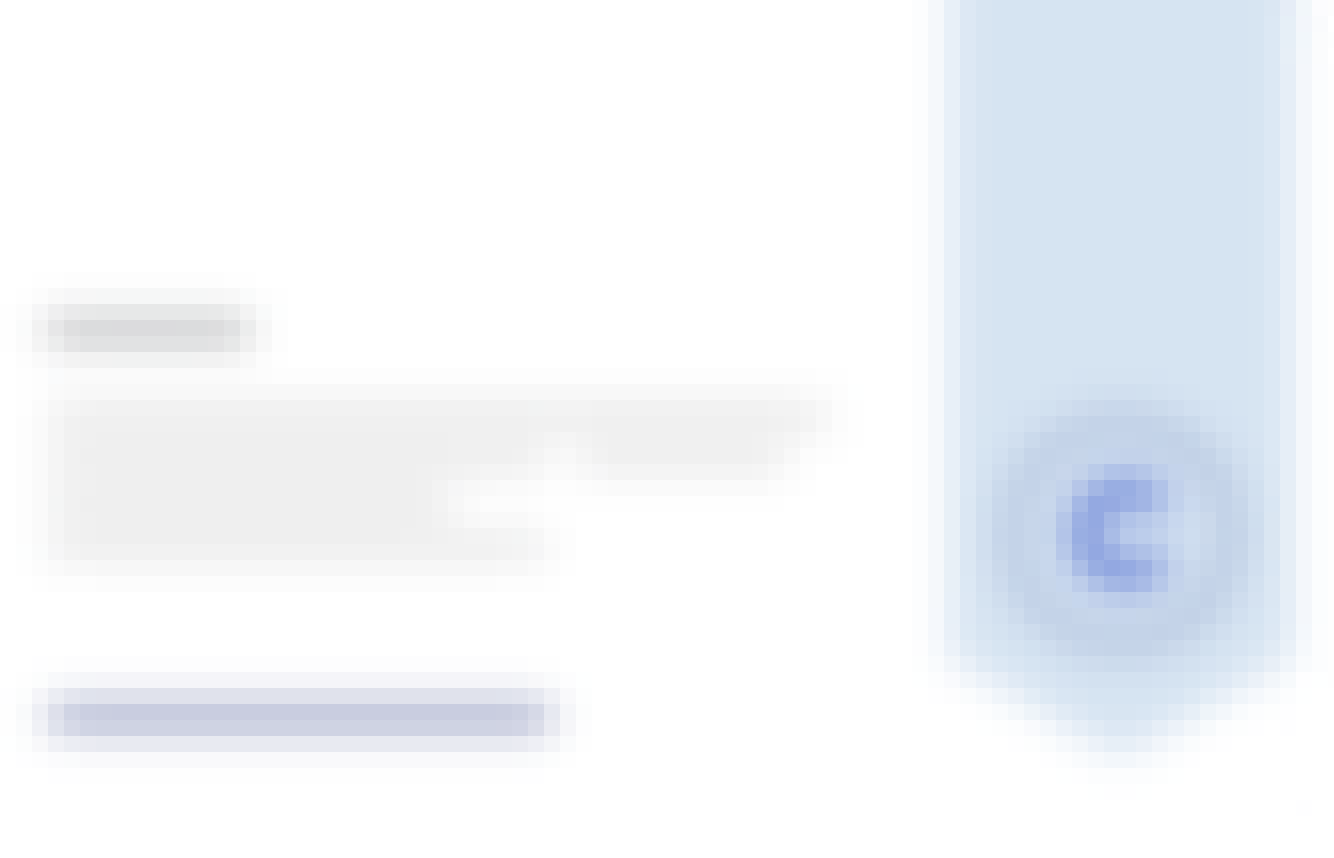
There are 5 modules in this course
Weights are used to expand a sample to a population. To accomplish this, the weights may correct for coverage errors in the sampling frame, adjust for nonresponse, and reduce variances of estimators by incorporating covariates. The series of steps needed to do this are covered in Module 1.
What's included
7 videos7 readings7 assignments
Specific steps in weighting include computing base weights, adjusting if there are cases whose eligibility we are unsure of, adjusting for nonresponse, and using covariates to calibrate the sample to external population controls. We flesh out the general steps with specific details here.
What's included
6 videos6 readings5 assignments
Software is critical to implementing the steps, but the R system is an excellent source of free routines. This module covers several R packages, including sampling, survey, and PracTools that will select samples and compute weights.
What's included
6 videos5 readings4 assignments
In most surveys there will be items for which respondents do not provide information, even though the respondent completed enough of the data collection instrument to be considered "complete". If only the cases with all items present are retained when fitting a model, quite a few cases may be excluded from the analysis. Imputing for the missing items avoids dropping the missing cases. We cover methods of doing the imputing and of reflecting the effects of imputations on standard errors in this module.
What's included
6 videos5 readings5 assignments
We briefly summarize the methods of weighting and imputation that were covered in Course 5.
What's included
1 video1 reading
Instructor

Offered by
Why people choose Coursera for their career




Learner reviews
133 reviews
- 5 stars
41.35%
- 4 stars
25.56%
- 3 stars
13.53%
- 2 stars
8.27%
- 1 star
11.27%
Showing 3 of 133
Reviewed on Dec 24, 2017
This is a higher level course. Good for beginners.
Reviewed on Apr 13, 2017
Aside from a little hiccup with one of the quiz questions during week 1, this course was worth my time.
Reviewed on Nov 18, 2017
This course was hard to follow, hard to complete (quizzes), poorly designed and with little useful content. In other words, not worth the money I paid for it!
Recommended if you're interested in Data Science
DeepLearning.AI
Eindhoven University of Technology

Open new doors with Coursera Plus
Unlimited access to 10,000+ world-class courses, hands-on projects, and job-ready certificate programs - all included in your subscription
Advance your career with an online degree
Earn a degree from world-class universities - 100% online
Join over 3,400 global companies that choose Coursera for Business
Upskill your employees to excel in the digital economy