Data never arrive in the condition that you need them in order to do effective data analysis. Data need to be re-shaped, re-arranged, and re-formatted, so that they can be visualized or be inputted into a machine learning algorithm. This course addresses the problem of wrangling your data so that you can bring them under control and analyze them effectively. The key goal in data wrangling is transforming non-tidy data into tidy data.

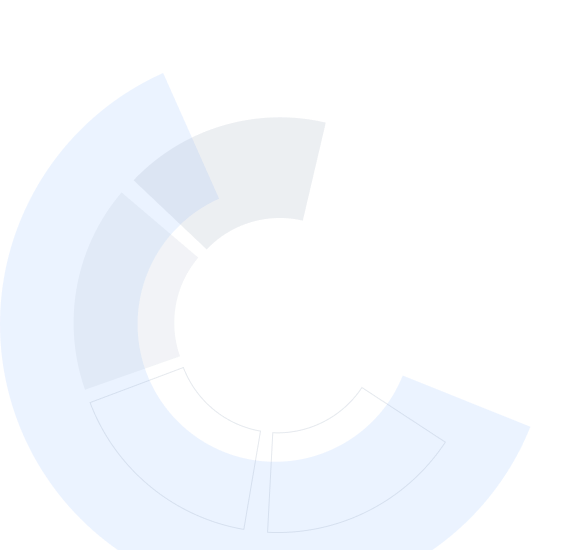
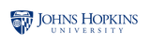
Wrangling Data in the Tidyverse
This course is part of Tidyverse Skills for Data Science in R Specialization



Instructors: Carrie Wright, PhD
Sponsored by MAHE Manipal
2,131 already enrolled
(31 reviews)
What you'll learn
Apply Tidyverse functions to transform non-tidy data to tidy data
Conduct basic exploratory data analysis
Conduct analyses of text data
Skills you'll gain
Details to know

Add to your LinkedIn profile
7 assignments
See how employees at top companies are mastering in-demand skills
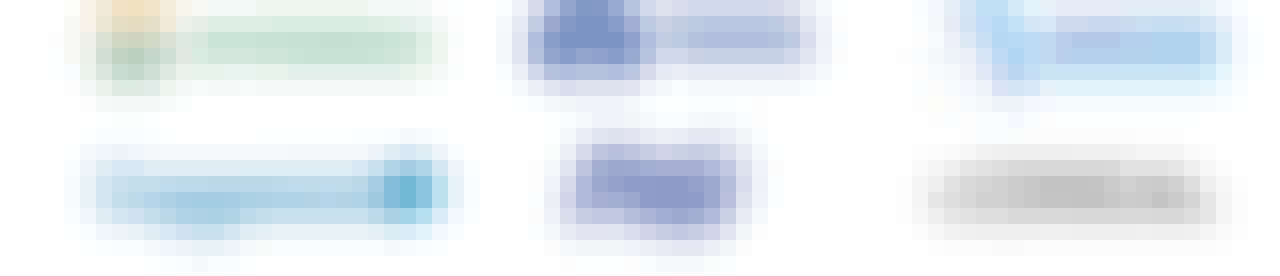
Build your subject-matter expertise
- Learn new concepts from industry experts
- Gain a foundational understanding of a subject or tool
- Develop job-relevant skills with hands-on projects
- Earn a shareable career certificate
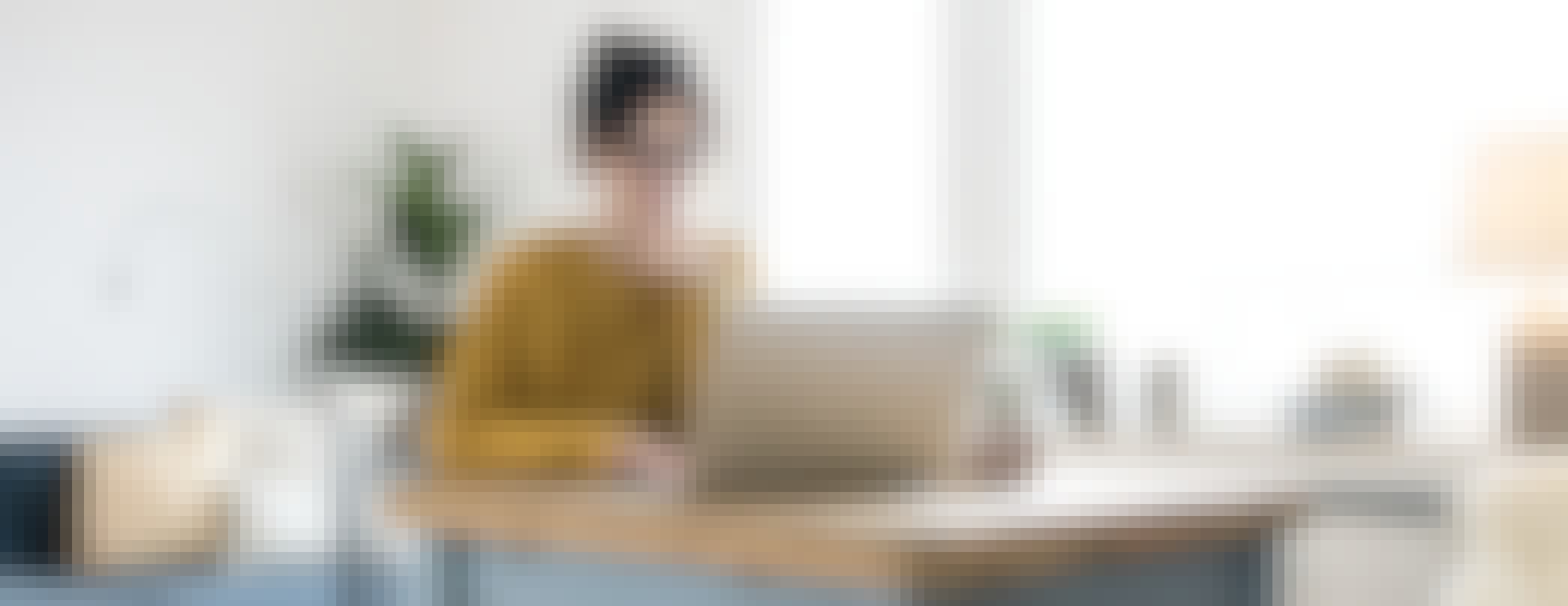
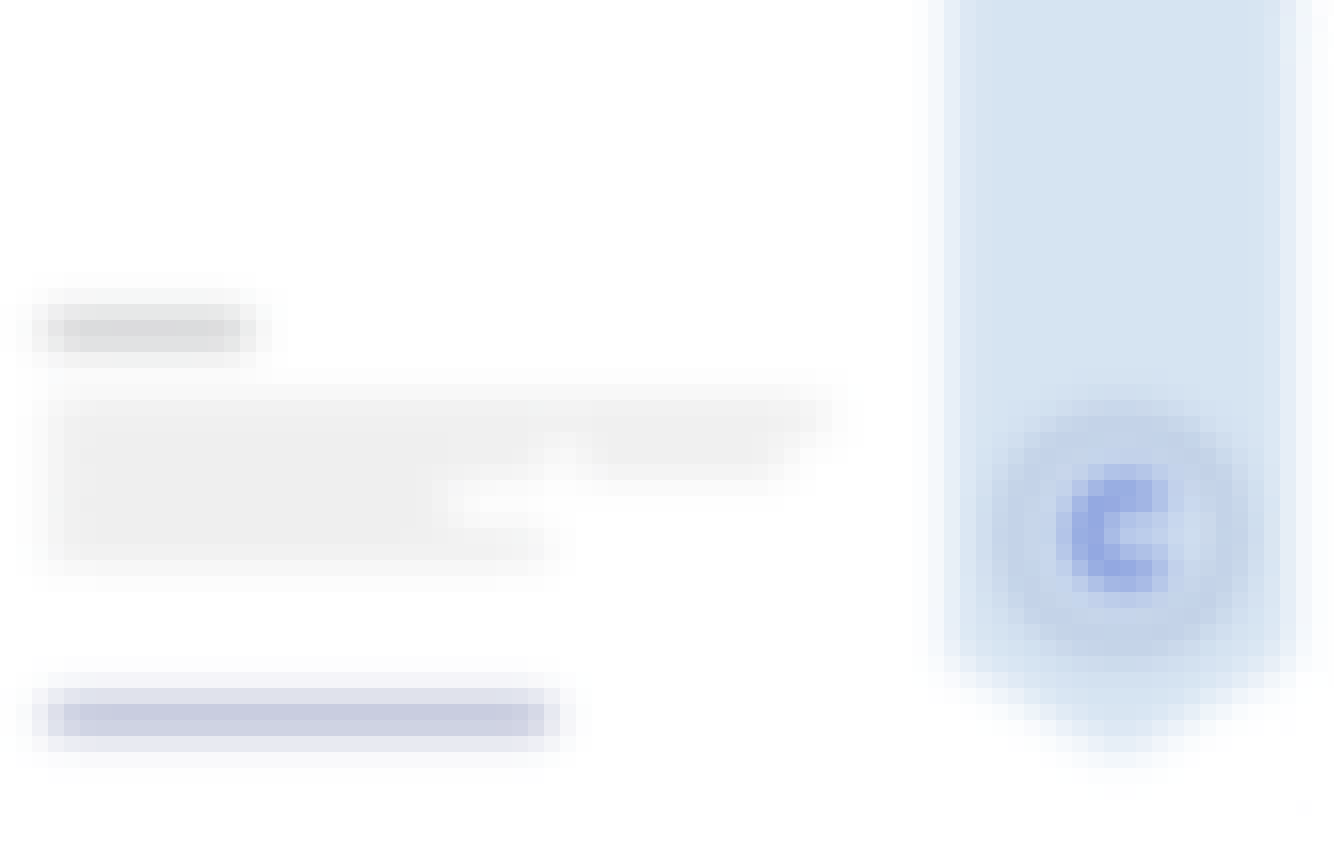
Earn a career certificate
Add this credential to your LinkedIn profile, resume, or CV
Share it on social media and in your performance review
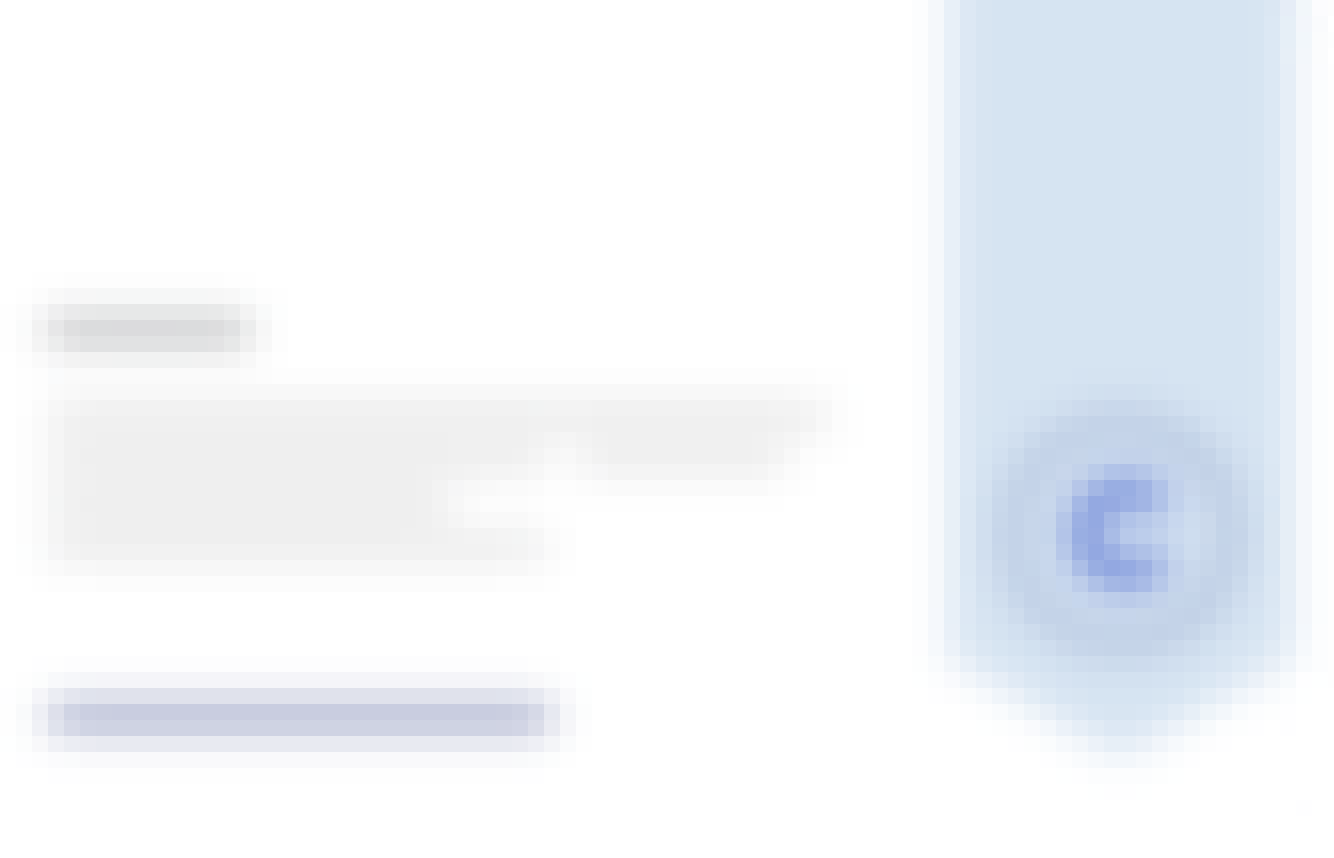
There are 6 modules in this course
Data never arrive in the condition that you need them in order to do effective data analysis. Data need to be re-shaped, re-arranged, and re-formatted, so that they can be visualized or be inputted into a machine learning algorithm. This module addresses the problem of wrangling your data so that you can bring them under control and analyze them effectively. The key goal in data wrangling is transforming non-tidy data into tidy data.
What's included
19 readings2 assignments
In R, categorical data are handled as factors. By definition, categorical data are limited in that they have a set number of possible values they can take. For example, there are 12 months in a calendar year. In a month variable, each observation is limited to taking one of these twelve values. Thus, with a limited number of possible values, month is a categorical variable. Categorical data, which will be referred to as factors for the rest of this lesson, are regularly found in data. Learning how to work with this type of variable effectively will be incredibly helpful.
What's included
14 readings2 assignments
Working with text data is increasingly common in data science projects. Text manipulation is often needed to clean up messy datasets and to create numerical measurements out of text input. In addition, often the text themselves are the data and this module covers tools to extract information from the text.
What's included
13 readings2 assignments
The goal of an exploratory analysis is to examine, or explore the data and find relationships that weren’t previously known. Exploratory analyses explore how different measures might be related to each other but do not confirm that relationship as causal, i.e., one variable causing another. You’ve probably heard the phrase “Correlation does not imply causation,” and exploratory analyses lie at the root of this saying. Just because you observe a relationship between two variables during exploratory analysis, it does not mean that one necessarily causes the other.
What's included
2 readings
Now we will demonstrate how to import data using our case study examples. When working through the steps of the case studies, you can use either RStudio on your own computer or Coursera lab spaces provided for each case study.
What's included
11 readings2 ungraded labs
In this project, you will practice data exploration and data wrangling with the tidyverse using consumer complaint data from the Consumer Financial Protection Bureau (CFPB).
What's included
1 reading1 assignment
Instructors



Offered by
Why people choose Coursera for their career




Learner reviews
31 reviews
- 5 stars
68.75%
- 4 stars
18.75%
- 3 stars
9.37%
- 2 stars
3.12%
- 1 star
0%
Showing 3 of 31
Reviewed on Apr 24, 2021
Excellent course! I've learned so many useful R techniques/codes!
Reviewed on Apr 18, 2022
Great course to get yourself acquanted with data wrangling in Tidyverse.
Recommended if you're interested in Data Science
Duke University
Duke University
University of Michigan
University of Colorado Boulder

Open new doors with Coursera Plus
Unlimited access to 10,000+ world-class courses, hands-on projects, and job-ready certificate programs - all included in your subscription
Advance your career with an online degree
Earn a degree from world-class universities - 100% online
Join over 3,400 global companies that choose Coursera for Business
Upskill your employees to excel in the digital economy