Many real-world algorithmic problems cannot be solved efficiently using traditional algorithmic tools, for example, because the problems are NP-hard. The goal of the Approximation Algorithms course is to become familiar with important algorithmic concepts and techniques needed to effectively deal with such problems. These techniques apply when we don't require the optimal solution to certain problems, but an approximation that is close to the optimal solution. We will see how to efficiently find such approximations.

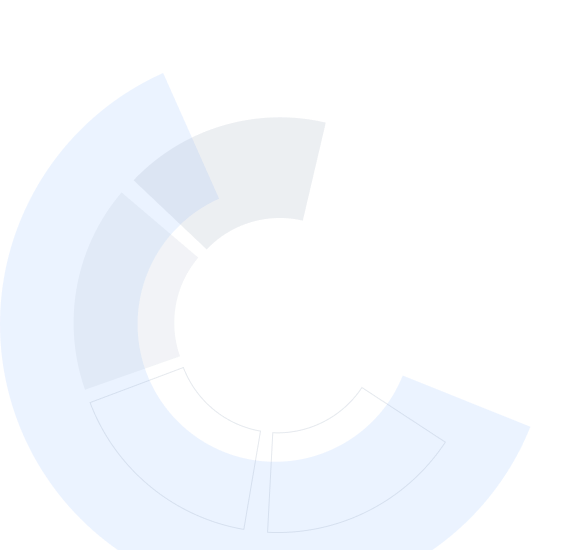

Approximation Algorithms

Instructor: Mark de Berg
Sponsored by Mojatu Foundation
6,660 already enrolled
(32 reviews)
Details to know

Add to your LinkedIn profile
4 assignments
See how employees at top companies are mastering in-demand skills
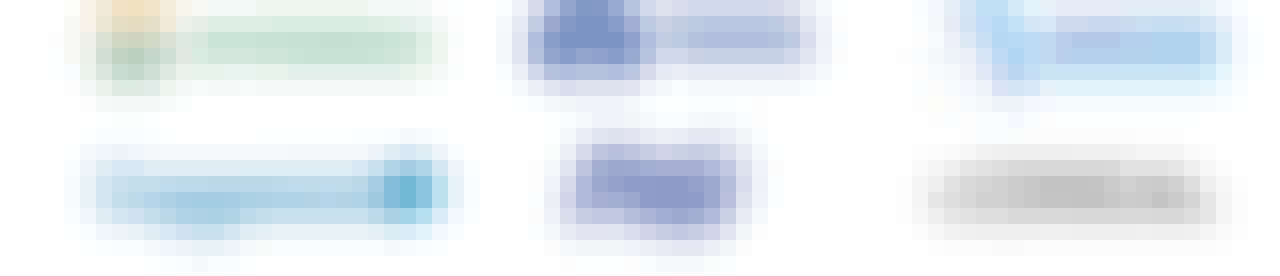
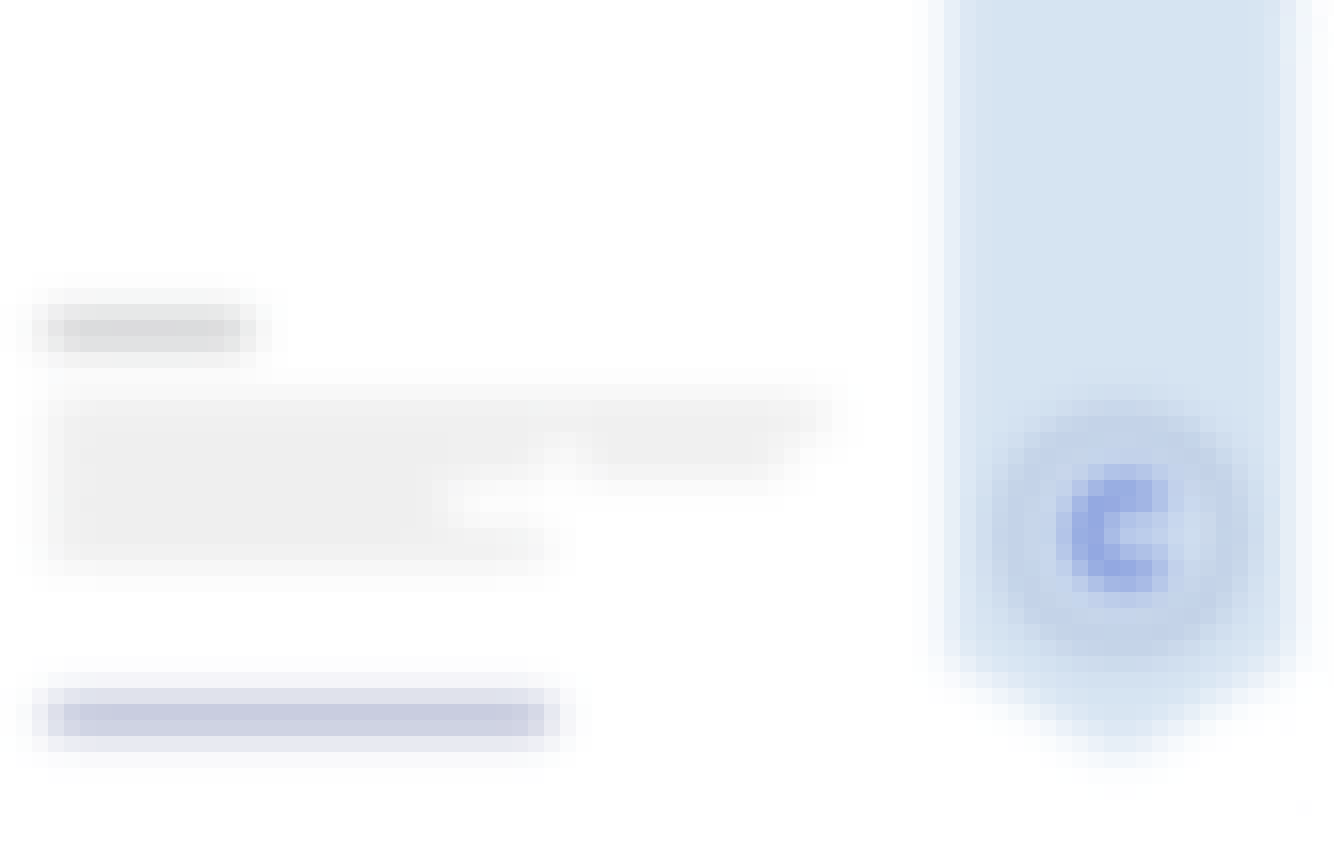
Earn a career certificate
Add this credential to your LinkedIn profile, resume, or CV
Share it on social media and in your performance review
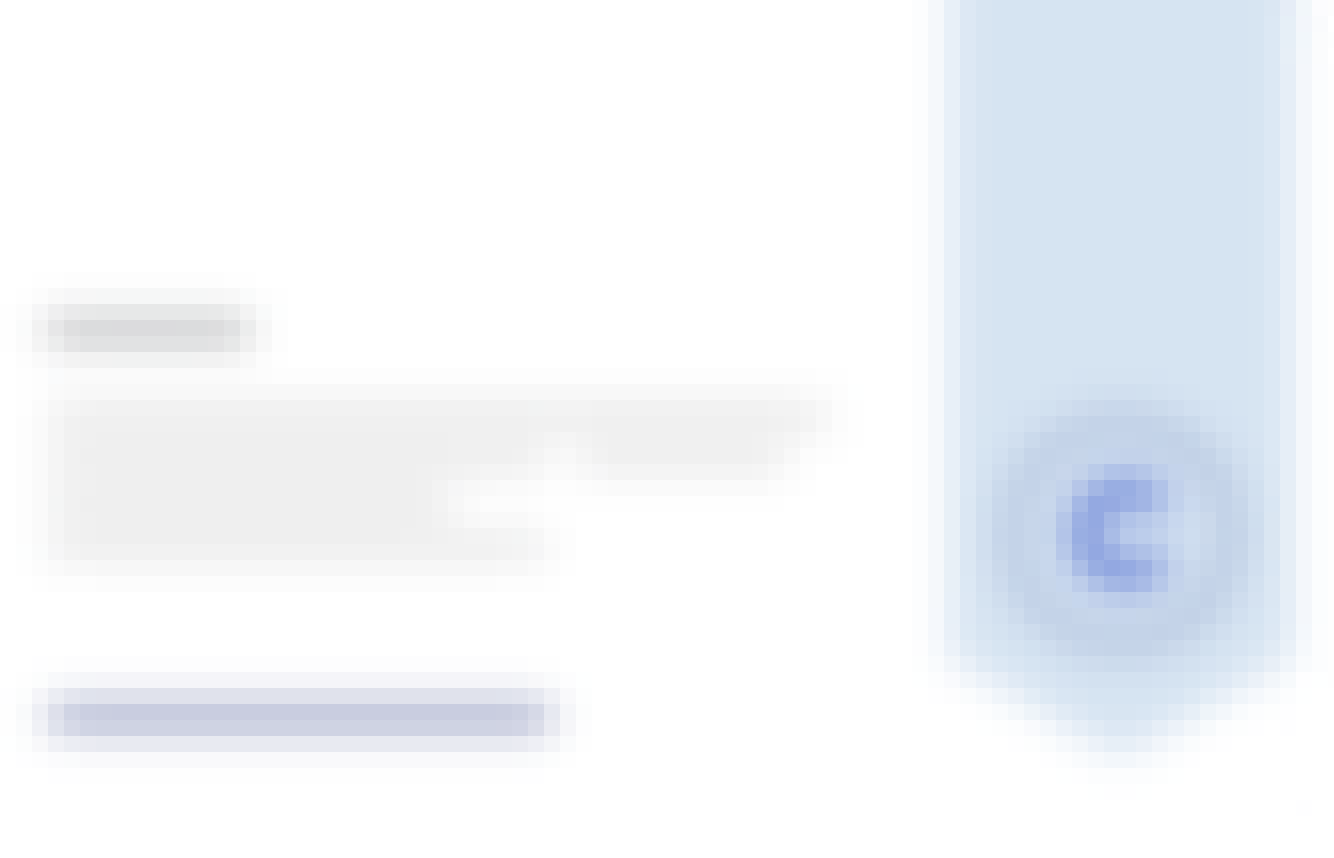
There are 4 modules in this course
In the module the motivation for studying approximation algorithms will be given. We will discuss what optimization problems are, and what the difference between heuristics and approximation algorithms is. Finally, we will introduce the concept of approximation ratio, which plays a central role in the analysis of the quality of approximation algorithms.
What's included
1 video1 reading1 assignment
In this module we will study various approximation algorithms for the load balancing problem. This problems asks to distribute a given set of jobs, each with a certain processing time, over a number of machine. The goal is to do this such that all jobs are finished as soon as possible. We will analyze the quality of the computed solutions computed using the concept of rho-approximation, which we saw in the previous lecture. In this analysis we will see that lower bounds on the optimal solution play a crucial role in the analysis (or, for maximization problems: upper bounds).
What's included
3 videos1 reading1 assignment1 programming assignment
In this module we will introduce the technique of LP relaxation to design approximation algorithms, and explain how to analyze the approximation ratio of an algorithm based in LP relaxation. We will do this using the (weighted) Vertex Cover problem as an example. Before we explain the technique of LP relaxation, however, we first give a simple 2-approximation algorithm for the unweighted Vertex Cover problem.
What's included
6 videos2 readings1 assignment
In this module we will introduce the concept of Polynomial-Time Approximation Scheme (PTAS), which are algorithms that can get arbitrarily close to an optimal solution. We describe a general technique to design PTASs, and apply it to the famous Knapsack problem. Finally we will see how to analyze PTASs that are designed with the general technique.
What's included
6 videos2 readings1 assignment1 programming assignment
Instructor

Offered by
Why people choose Coursera for their career




Learner reviews
32 reviews
- 5 stars
78.12%
- 4 stars
15.62%
- 3 stars
3.12%
- 2 stars
3.12%
- 1 star
0%
Showing 3 of 32
Reviewed on Feb 24, 2021
Very good course! A nice introduction to approximation algorithms.
Reviewed on Jan 26, 2021
Excellent short course on approximation algorithms. Good course material, presentations and exercises.
Reviewed on Oct 10, 2020
Please try to include some more numeric example like load balancing problem in the vertex cover and rest topics
Recommended if you're interested in Computer Science
Tsinghua University
DeepLearning.AI
Microsoft
Tsinghua University

Open new doors with Coursera Plus
Unlimited access to 10,000+ world-class courses, hands-on projects, and job-ready certificate programs - all included in your subscription
Advance your career with an online degree
Earn a degree from world-class universities - 100% online
Join over 3,400 global companies that choose Coursera for Business
Upskill your employees to excel in the digital economy