Get ready to put all your gen AI engineering skills into practice! This guided project will test and apply the knowledge and understanding you’ve gained throughout the previous courses in the program. You will build your own real-world gen AI application.

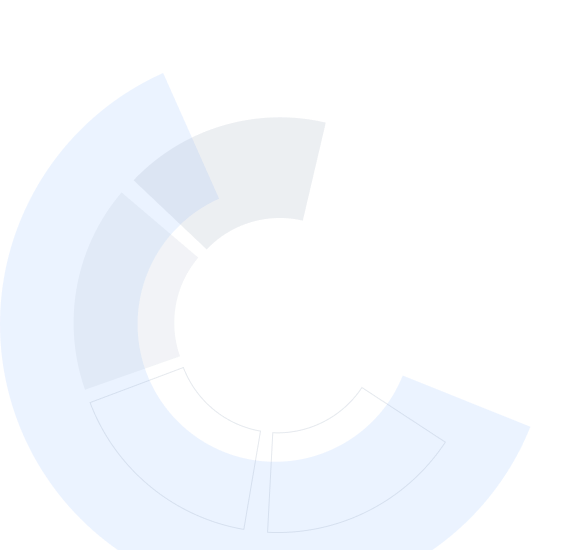
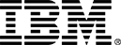
Project: Generative AI Applications with RAG and LangChain
This course is part of multiple programs.


Instructors: Kang Wang
Sponsored by Mojatu Foundation
3,029 already enrolled
(24 reviews)
Recommended experience
What you'll learn
Gain practical experience building your own real-world gen AI application that you can talk about in interviews.
Get hands-on using LangChain to load documents and apply text splitting techniques with RAG and LangChain to enhance model responsiveness.
Create and configure a vector database to store document embeddings and develop a retriever to fetch document segments based on queries.
Set up a simple Gradio interface for model interaction and construct a QA bot using LangChain and an LLM to answer questions from loaded documents.
Details to know

Add to your LinkedIn profile
7 assignments
October 2024
See how employees at top companies are mastering in-demand skills
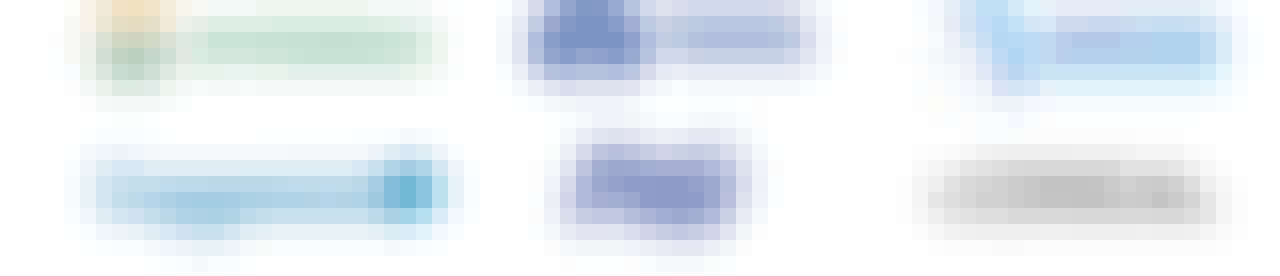
Build your subject-matter expertise
- Learn new concepts from industry experts
- Gain a foundational understanding of a subject or tool
- Develop job-relevant skills with hands-on projects
- Earn a shareable career certificate
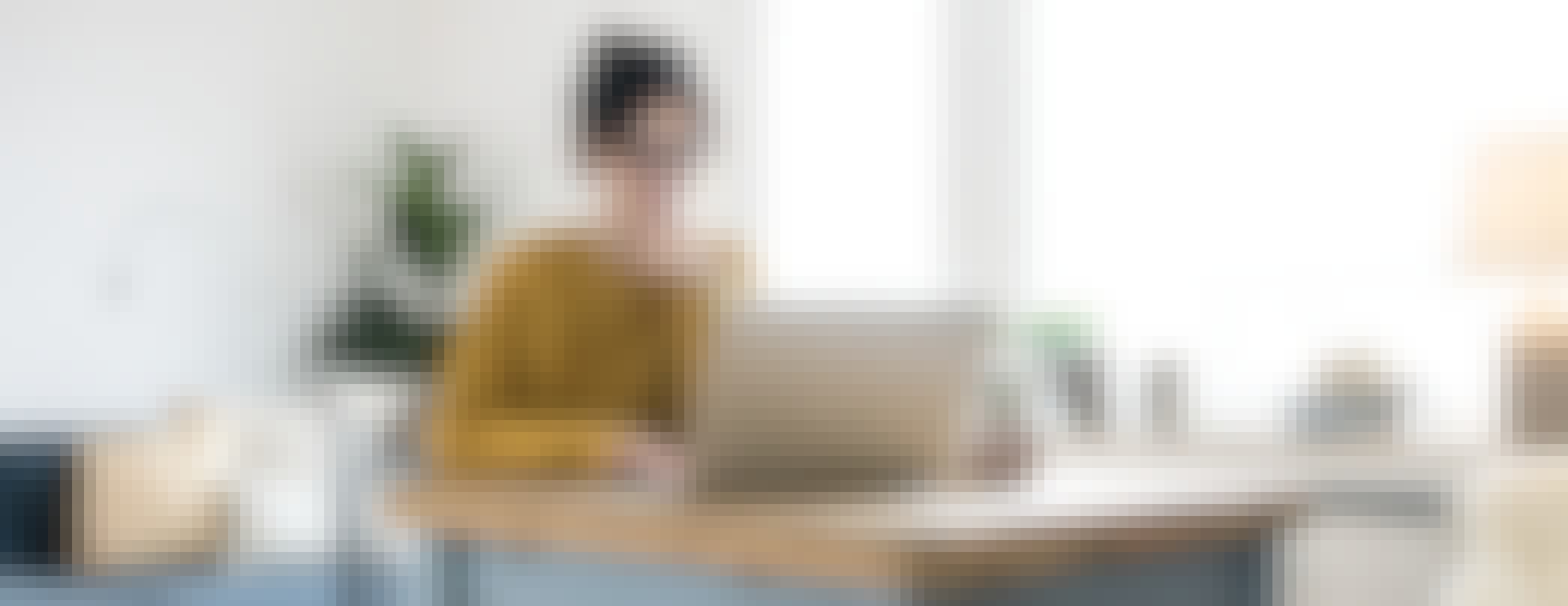
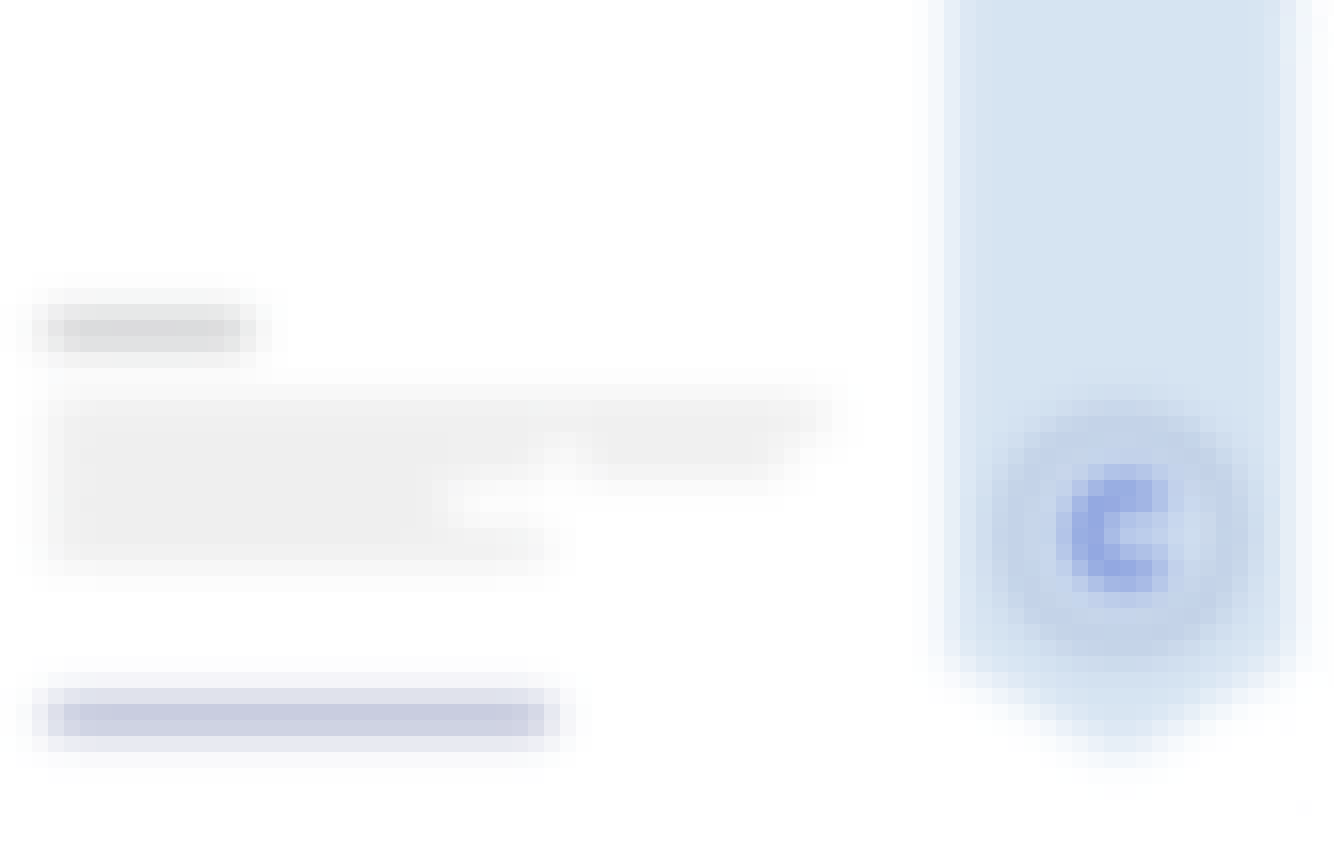
Earn a career certificate
Add this credential to your LinkedIn profile, resume, or CV
Share it on social media and in your performance review
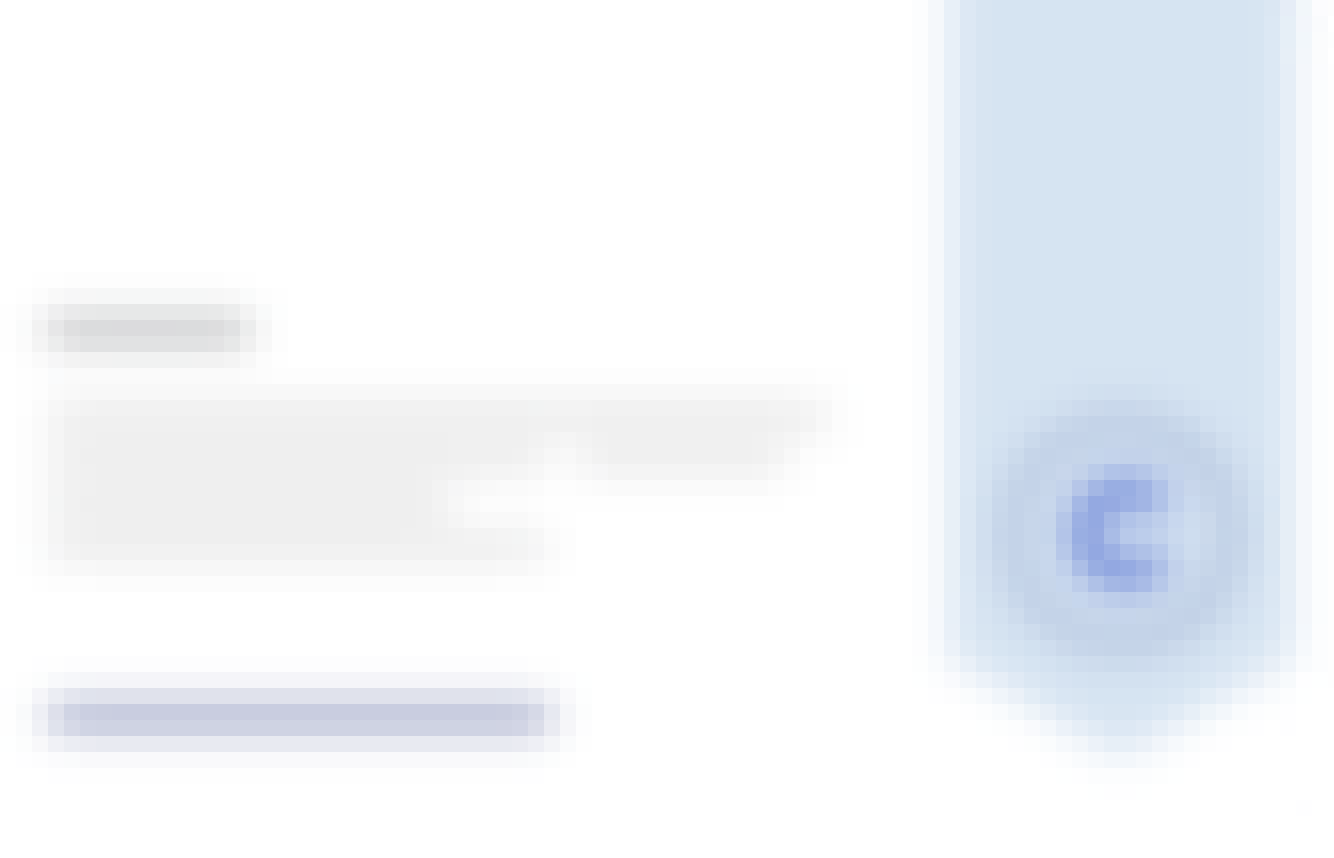
There are 3 modules in this course
In this module, you will learn all about document loaders from LangChain and then use that knowledge to load your document from various sources. You will also explore the various text splitting strategies with RAG and LangChain and apply them to enhance model responsiveness. Hands-on labs will provide you an opportunity to practice loading documents as well as implement the text-splitting techniques you have learned.
What's included
3 videos4 readings2 assignments3 app items1 plugin
In this module, you will learn how to store embeddings using a vector store and how to use Chroma DB to save embeddings. You’ll gain insights into LangChain retrievers like the Vector Store-Based, Multi-Query, Self-Query, and Parent Document Retriever. In hands-on labs, you’ll prepare and preprocess documents for embedding and use watsonx.ai to generate embeddings for your documents. You’ll use vector databases such as Chroma DB and FAISS to store embeddings generated from textual data using LangChain. Finally, you’ll use various retrievers to efficiently extract relevant document segments from text using LangChain.
What's included
3 videos1 reading2 assignments3 app items2 plugins
In this module, you will learn how to implement RAG to improve retrieval. You will become familiar with Gradio and how to set up a simple Gradio interface to interact with your models. You will also learn how to construct a QA bot to answer questions from loaded documents using LangChain and LLMs. Using hands-on labs, you will have the opportunity to practice setting up a Gradio interface, as well as constructing a QA bot. In the final project, you will build an AI application using RAG and LangChain.
What's included
1 video4 readings3 assignments1 peer review2 app items4 plugins
Why people choose Coursera for their career




Learner reviews
24 reviews
- 5 stars
92%
- 4 stars
4%
- 3 stars
0%
- 2 stars
4%
- 1 star
0%
Showing 3 of 24
Reviewed on Dec 18, 2024
The best of the one of AI foundation courser, Thanks a lot, only this course give code detail material, really learned a lot, Super, Bravo!
Recommended if you're interested in Data Science
SkillUp EdTech
Board Infinity

Open new doors with Coursera Plus
Unlimited access to 10,000+ world-class courses, hands-on projects, and job-ready certificate programs - all included in your subscription
Advance your career with an online degree
Earn a degree from world-class universities - 100% online
Join over 3,400 global companies that choose Coursera for Business
Upskill your employees to excel in the digital economy