This course introduces the Bayesian approach to statistics, starting with the concept of probability and moving to the analysis of data. We will learn about the philosophy of the Bayesian approach as well as how to implement it for common types of data. We will compare the Bayesian approach to the more commonly-taught Frequentist approach, and see some of the benefits of the Bayesian approach. In particular, the Bayesian approach allows for better accounting of uncertainty, results that have more intuitive and interpretable meaning, and more explicit statements of assumptions. This course combines lecture videos, computer demonstrations, readings, exercises, and discussion boards to create an active learning experience. For computing, you have the choice of using Microsoft Excel or the open-source, freely available statistical package R, with equivalent content for both options. The lectures provide some of the basic mathematical development as well as explanations of philosophy and interpretation. Completion of this course will give you an understanding of the concepts of the Bayesian approach, understanding the key differences between Bayesian and Frequentist approaches, and the ability to do basic data analyses.

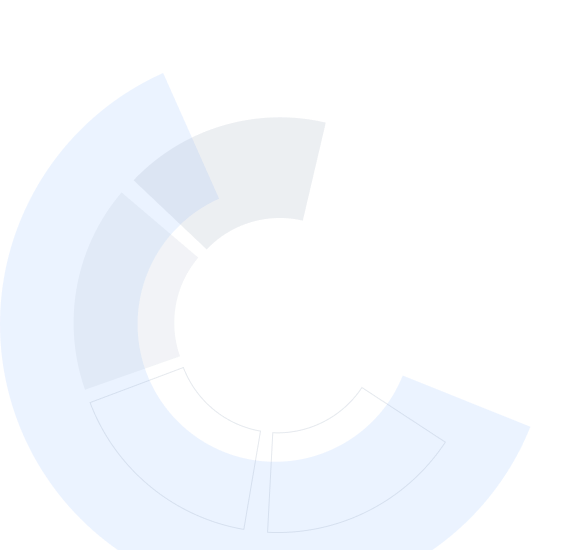
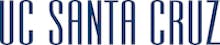
Bayesian Statistics: From Concept to Data Analysis
This course is part of Bayesian Statistics Specialization

Instructor: Herbert Lee
Sponsored by FutureX
152,521 already enrolled
(3,179 reviews)
What you'll learn
Describe & apply the Bayesian approach to statistics.
Explain the key differences between Bayesian and Frequentist approaches.
Master the basics of the R computing environment.
Skills you'll gain
- Business Analytics
- Statistical Analysis
- Analytics
- Statistical Inference
- Advanced Mathematics
- Mathematical Modeling
- Data Analysis
- Data Science
- Bayesian Statistics
- Probability Distribution
- Statistics
- Statistical Modeling
- Mathematics and Mathematical Modeling
- Probability
- Applied Mathematics
- Statistical Methods
- Probability & Statistics
- Regression Analysis
Details to know

Add to your LinkedIn profile
18 assignments
See how employees at top companies are mastering in-demand skills
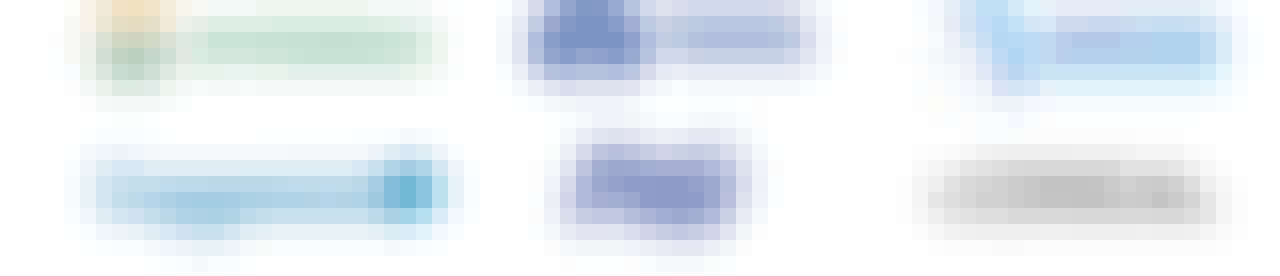
Build your subject-matter expertise
- Learn new concepts from industry experts
- Gain a foundational understanding of a subject or tool
- Develop job-relevant skills with hands-on projects
- Earn a shareable career certificate
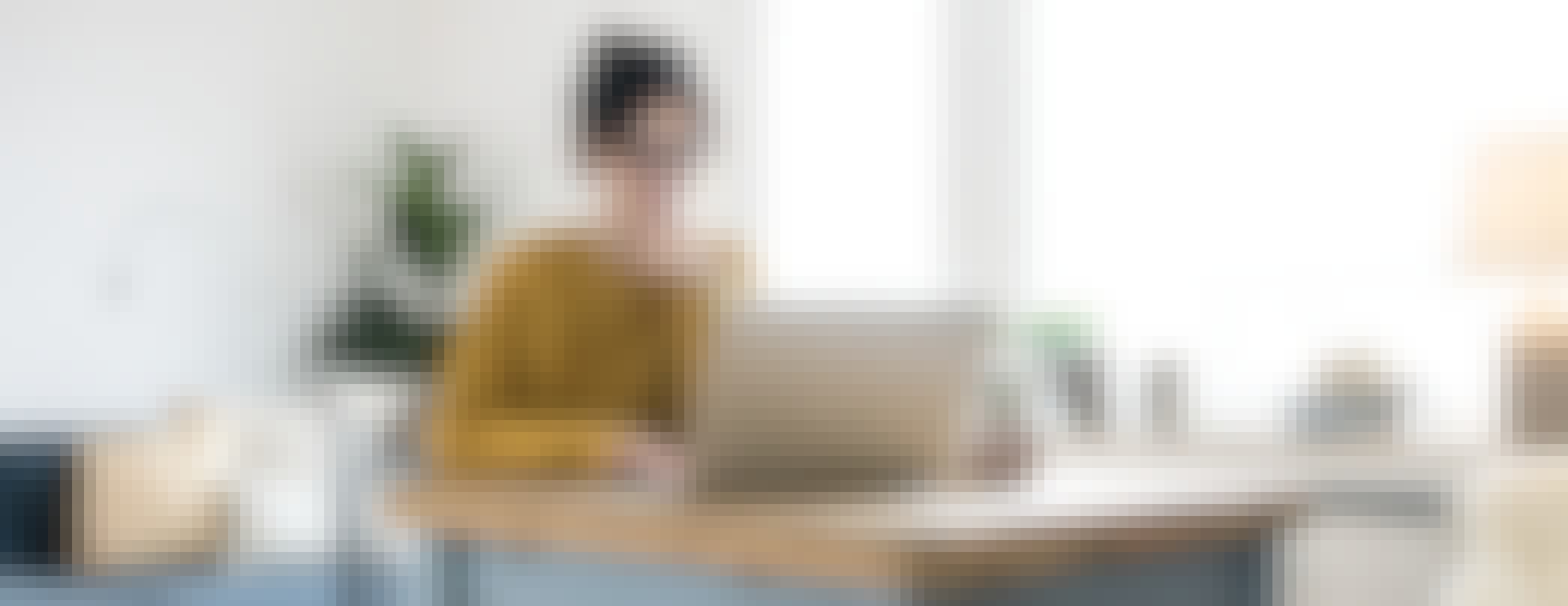
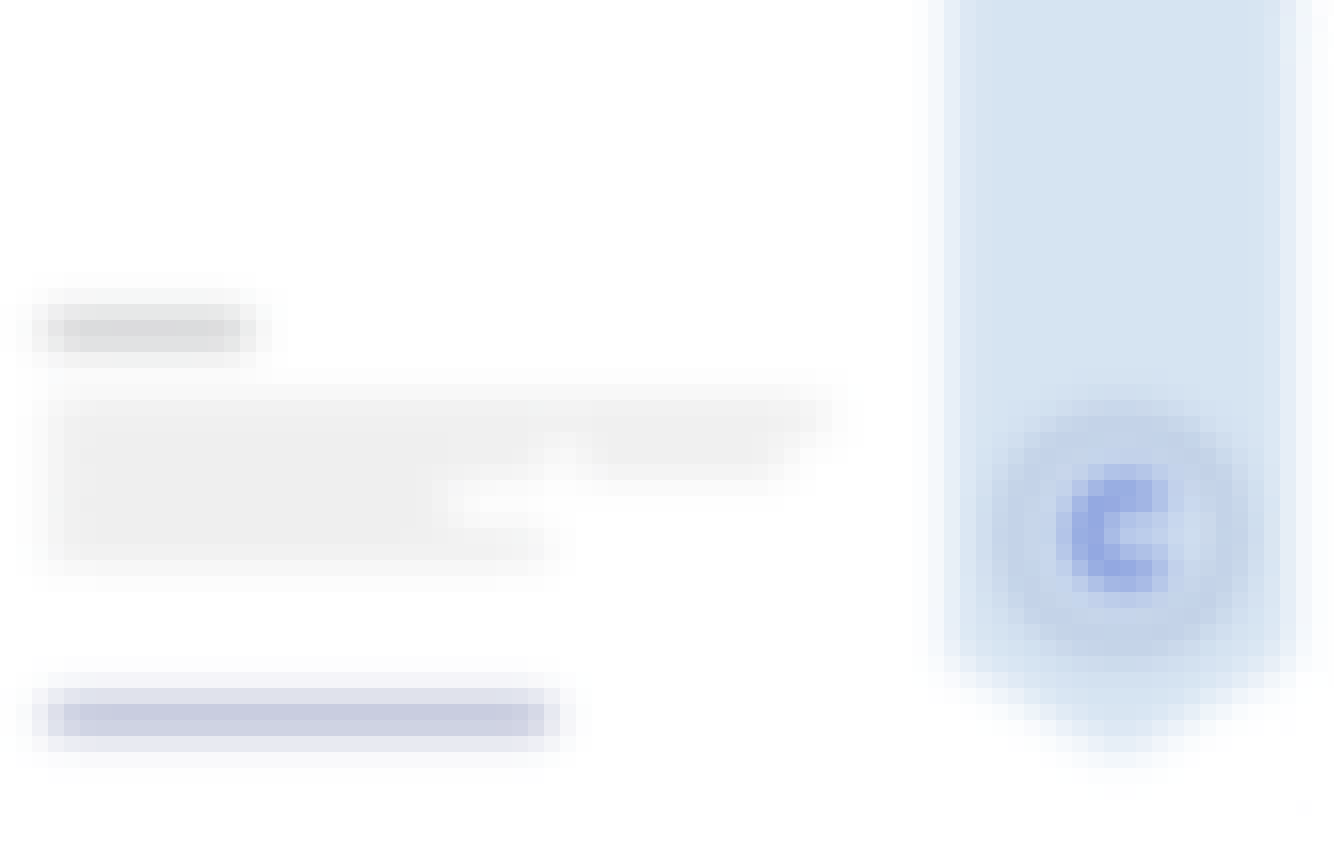
Earn a career certificate
Add this credential to your LinkedIn profile, resume, or CV
Share it on social media and in your performance review
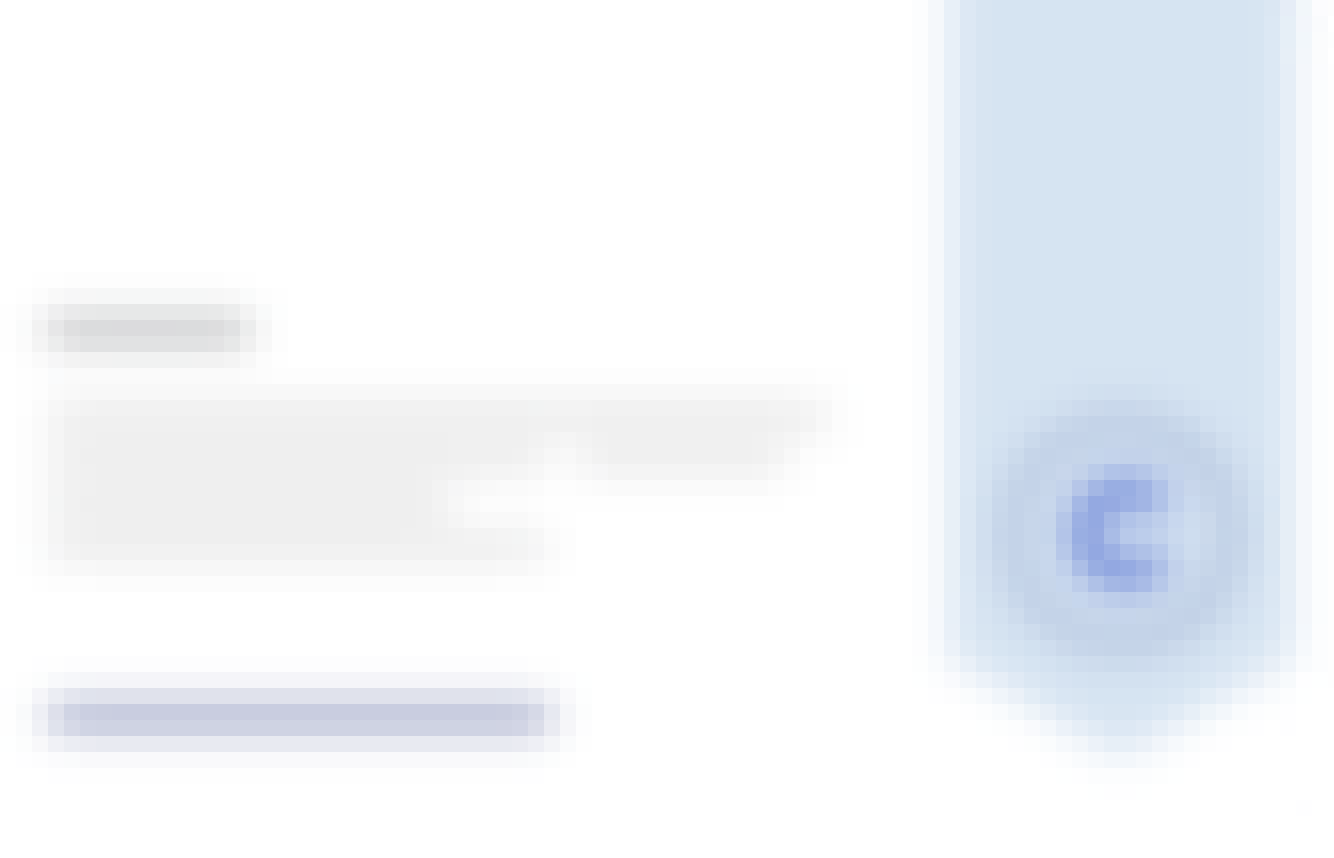
There are 4 modules in this course
In this module, we review the basics of probability and Bayes’ theorem. In Lesson 1, we introduce the different paradigms or definitions of probability and discuss why probability provides a coherent framework for dealing with uncertainty. In Lesson 2, we review the rules of conditional probability and introduce Bayes’ theorem. Lesson 3 reviews common probability distributions for discrete and continuous random variables.
What's included
8 videos4 readings5 assignments1 discussion prompt
This module introduces concepts of statistical inference from both frequentist and Bayesian perspectives. Lesson 4 takes the frequentist view, demonstrating maximum likelihood estimation and confidence intervals for binomial data. Lesson 5 introduces the fundamentals of Bayesian inference. Beginning with a binomial likelihood and prior probabilities for simple hypotheses, you will learn how to use Bayes’ theorem to update the prior with data to obtain posterior probabilities. This framework is extended with the continuous version of Bayes theorem to estimate continuous model parameters, and calculate posterior probabilities and credible intervals.
What's included
11 videos5 readings4 assignments1 discussion prompt
In this module, you will learn methods for selecting prior distributions and building models for discrete data. Lesson 6 introduces prior selection and predictive distributions as a means of evaluating priors. Lesson 7 demonstrates Bayesian analysis of Bernoulli data and introduces the computationally convenient concept of conjugate priors. Lesson 8 builds a conjugate model for Poisson data and discusses strategies for selection of prior hyperparameters.
What's included
9 videos2 readings4 assignments1 discussion prompt
This module covers conjugate and objective Bayesian analysis for continuous data. Lesson 9 presents the conjugate model for exponentially distributed data. Lesson 10 discusses models for normally distributed data, which play a central role in statistics. In Lesson 11, we return to prior selection and discuss ‘objective’ or ‘non-informative’ priors. Lesson 12 presents Bayesian linear regression with non-informative priors, which yield results comparable to those of classical regression.
What's included
9 videos5 readings5 assignments1 discussion prompt
Instructor

Offered by
Why people choose Coursera for their career




Learner reviews
3,179 reviews
- 5 stars
67.30%
- 4 stars
25.14%
- 3 stars
5.18%
- 2 stars
1.28%
- 1 star
1.06%
Showing 3 of 3179
Reviewed on May 24, 2020
This was an invaluable learning experience. I was delighted to go through this learning.
Reviewed on Sep 24, 2019
Very clear and informative. Would like a more extensive and combined reference material (PDF, so less need to lookup e.g. definitions of effective sample size for various distributions).
Reviewed on Feb 18, 2020
the notes for the lectures are missing.
Recommended if you're interested in Data Science
Coursera Project Network
IIMA - IIM Ahmedabad
Johns Hopkins University
University of Colorado Boulder

Open new doors with Coursera Plus
Unlimited access to 10,000+ world-class courses, hands-on projects, and job-ready certificate programs - all included in your subscription
Advance your career with an online degree
Earn a degree from world-class universities - 100% online
Join over 3,400 global companies that choose Coursera for Business
Upskill your employees to excel in the digital economy