In this course, we will expand our exploration of statistical inference techniques by focusing on the science and art of fitting statistical models to data. We will build on the concepts presented in the Statistical Inference course (Course 2) to emphasize the importance of connecting research questions to our data analysis methods. We will also focus on various modeling objectives, including making inference about relationships between variables and generating predictions for future observations.

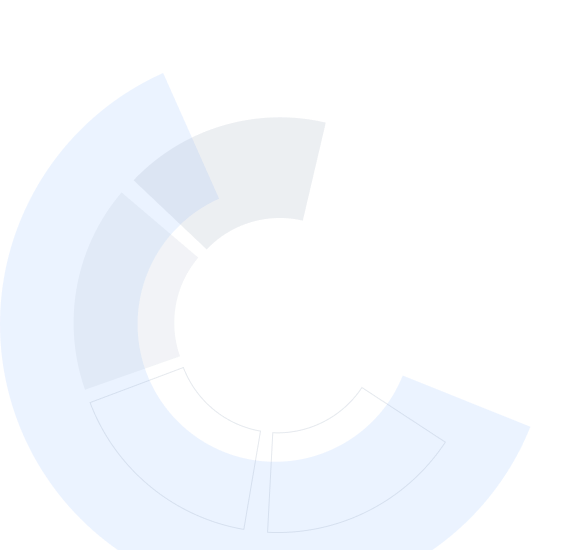
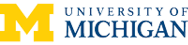
Fitting Statistical Models to Data with Python
This course is part of Statistics with Python Specialization



Instructors: Brenda Gunderson
Sponsored by FutureX
34,920 already enrolled
(692 reviews)
Recommended experience
What you'll learn
Deepen your understanding of statistical inference techniques by mastering the art of fitting statistical models to data.
Connect research questions with data analysis methods, emphasizing objectives, relationships between variables, and making predictions.
Explore various statistical modeling techniques like linear regression, logistic regression, and Bayesian inference using real data sets.
Work through hands-on case studies in Python with libraries like Statsmodels, Pandas, and Seaborn in the Jupyter Notebook environment.
Skills you'll gain
- Statistical Machine Learning
- Statistical Software
- Statistical Programming
- Bayesian Statistics
- Statistics
- Statistical Analysis
- Python Programming
- Mathematics and Mathematical Modeling
- Exploratory Data Analysis
- Data Analysis Software
- Mathematical Modeling
- Statistical Inference
- Regression Analysis
- Business Analytics
- Probability & Statistics
- Analytics
- Statistical Modeling
- Statistical Methods
- Data Analysis
- Data Science
Details to know

Add to your LinkedIn profile
7 assignments
See how employees at top companies are mastering in-demand skills
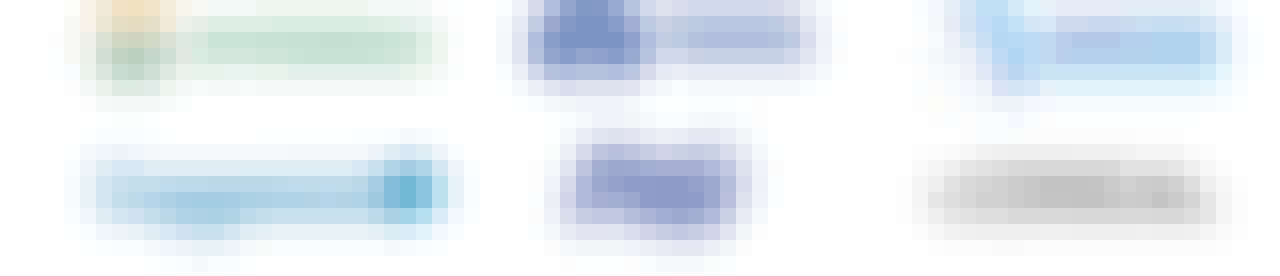
Build your subject-matter expertise
- Learn new concepts from industry experts
- Gain a foundational understanding of a subject or tool
- Develop job-relevant skills with hands-on projects
- Earn a shareable career certificate
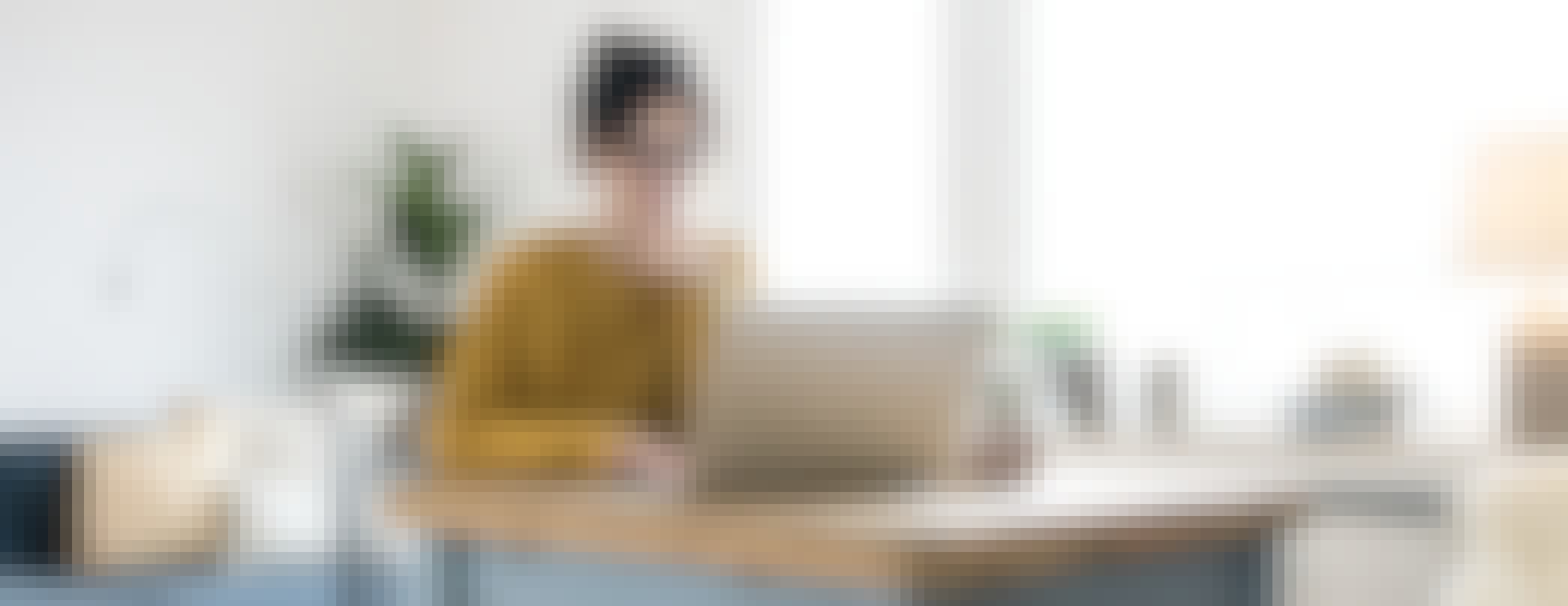
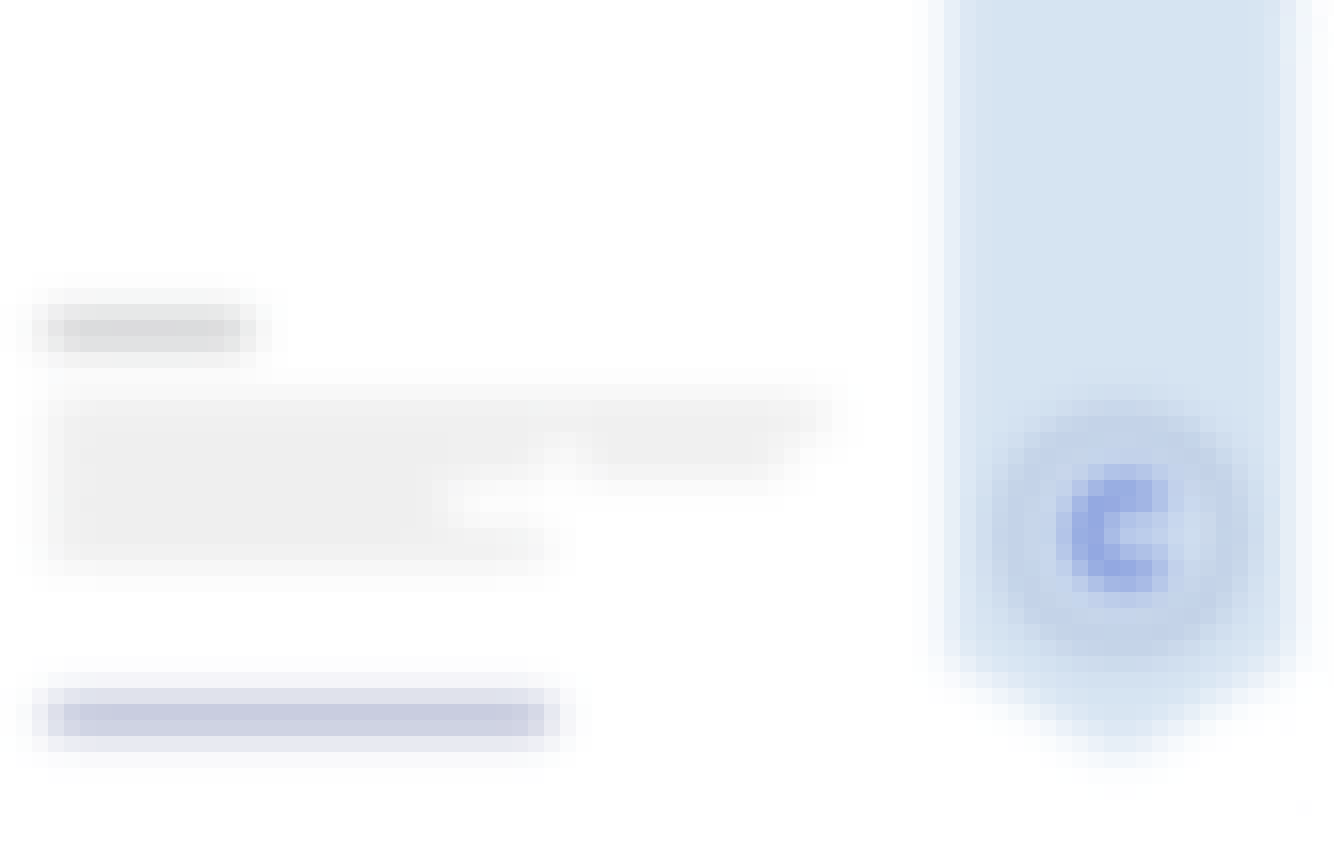
Earn a career certificate
Add this credential to your LinkedIn profile, resume, or CV
Share it on social media and in your performance review
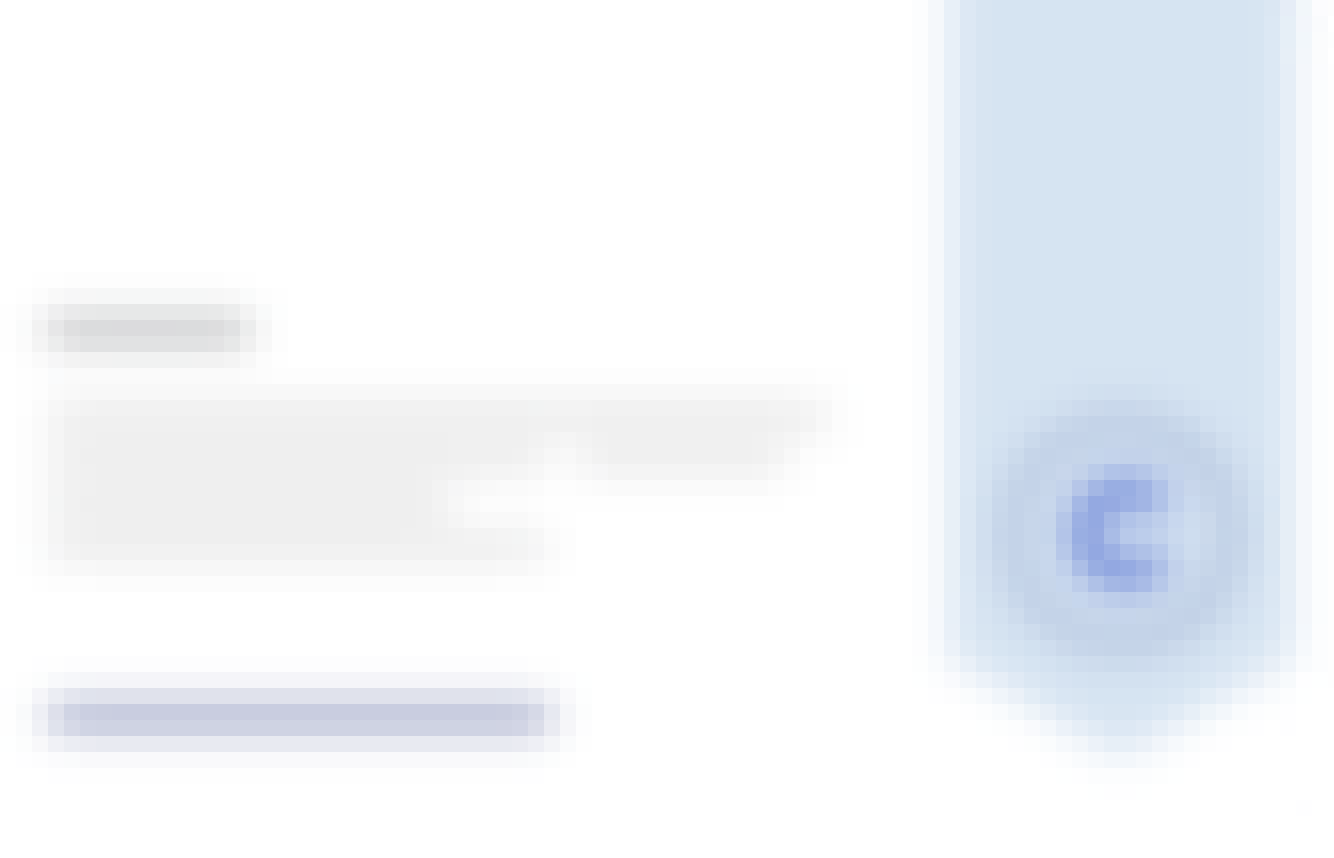
There are 4 modules in this course
We begin this third course of the Statistics with Python specialization with an overview of what is meant by “fitting statistical models to data.” In this first week, we will introduce key model fitting concepts, including the distinction between dependent and independent variables, how to account for study designs when fitting models, assessing the quality of model fit, exploring how different types of variables are handled in statistical modeling, and clearly defining the objectives of fitting models.
What's included
8 videos6 readings1 assignment2 ungraded labs
In this second week, we’ll introduce you to the basics of two types of regression: linear regression and logistic regression. You’ll get the chance to think about how to fit models, how to assess how well those models fit, and to consider how to interpret those models in the context of the data. You’ll also learn how to implement those models within Python.
What's included
5 videos4 readings3 assignments3 ungraded labs
In the third week of this course, we will be building upon the modeling concepts discussed in Week 2. Multilevel and marginal models will be our main topic of discussion, as these models enable researchers to account for dependencies in variables of interest introduced by study designs. We’ll be covering why and when we fit these alternative models, likelihood ratio tests, as well as fixed effects and their interpretations.
What's included
7 videos3 readings2 assignments4 ungraded labs
In this final week, we introduce special topics that extend the curriculum from previous weeks and courses further. We will cover a broad range of topics such as various types of dependent variables, exploring sampling methods and whether or not to use survey weights when fitting models, and in-depth case studies utilizing Bayesian techniques to derive insights from data. You’ll also have the opportunity to apply Bayesian techniques in Python.
What's included
6 videos4 readings1 assignment1 discussion prompt1 ungraded lab
Instructors

Offered by
Why people choose Coursera for their career




Learner reviews
692 reviews
- 5 stars
65.89%
- 4 stars
19.94%
- 3 stars
7.94%
- 2 stars
3.46%
- 1 star
2.74%
Showing 3 of 692
Reviewed on Oct 20, 2020
Overall, the course was a great refresher of statistical theory and application with some great Python exercises. However, some of the Python coding instruction itself could have been more detailed.
Reviewed on Jun 19, 2020
The course was wonderful however, sometimes I felt that a little bit more details could be provided when python code was being explained for week 2.
Reviewed on Jun 4, 2020
The course is great, the only improvement I would make is to be a little more didactic in the last two units because it is a more complicated subject.
Recommended if you're interested in Data Science
University of Colorado Boulder
Johns Hopkins University

Open new doors with Coursera Plus
Unlimited access to 10,000+ world-class courses, hands-on projects, and job-ready certificate programs - all included in your subscription
Advance your career with an online degree
Earn a degree from world-class universities - 100% online
Join over 3,400 global companies that choose Coursera for Business
Upskill your employees to excel in the digital economy