We have all heard the phrase “correlation does not equal causation.” What, then, does equal causation? This course aims to answer that question and more!

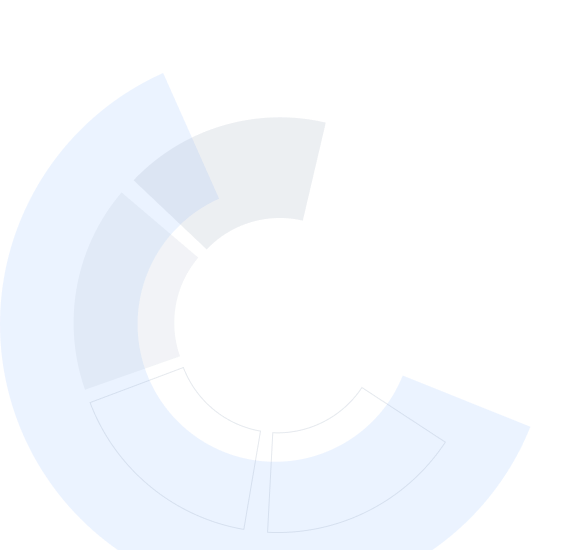
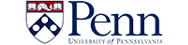
A Crash Course in Causality: Inferring Causal Effects from Observational Data

Instructor: Jason A. Roy, Ph.D.
Sponsored by EdgePoint Software
43,449 already enrolled
(560 reviews)
Skills you'll gain
- Graph Theory
- Analytics
- Data Science
- Business Analytics
- Statistical Methods
- Statistical Analysis
- Theoretical Computer Science
- Mathematics and Mathematical Modeling
- Statistical Machine Learning
- Mathematical Modeling
- Statistical Programming
- Regression Analysis
- R Programming
- Statistics
- Probability & Statistics
- Data Analysis
- Probability
- Applied Mathematics
- Statistical Modeling
- Statistical Inference
Details to know

Add to your LinkedIn profile
16 assignments
See how employees at top companies are mastering in-demand skills
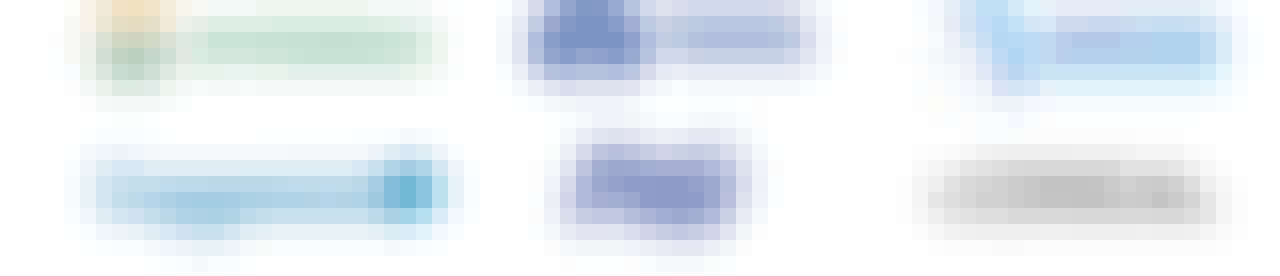
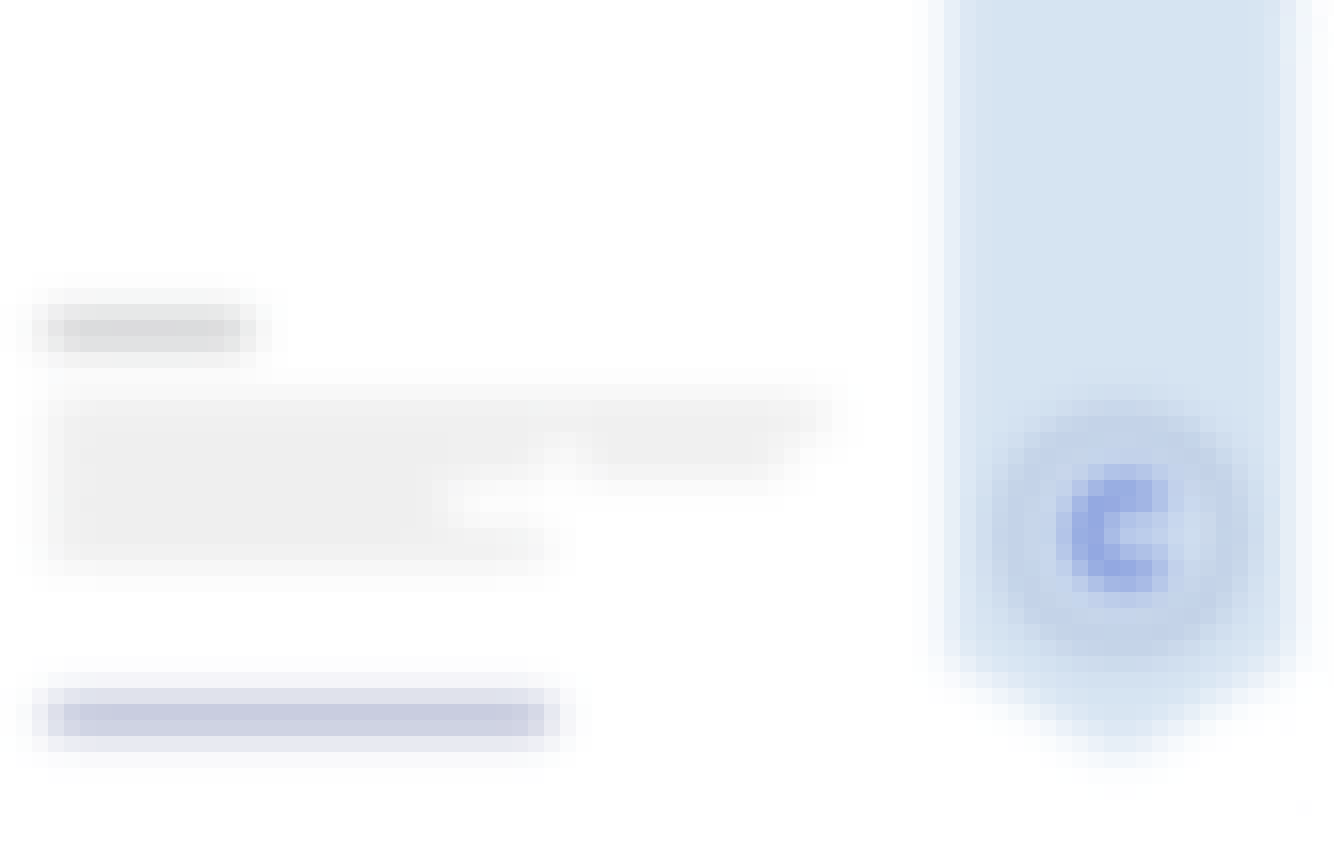
Earn a career certificate
Add this credential to your LinkedIn profile, resume, or CV
Share it on social media and in your performance review
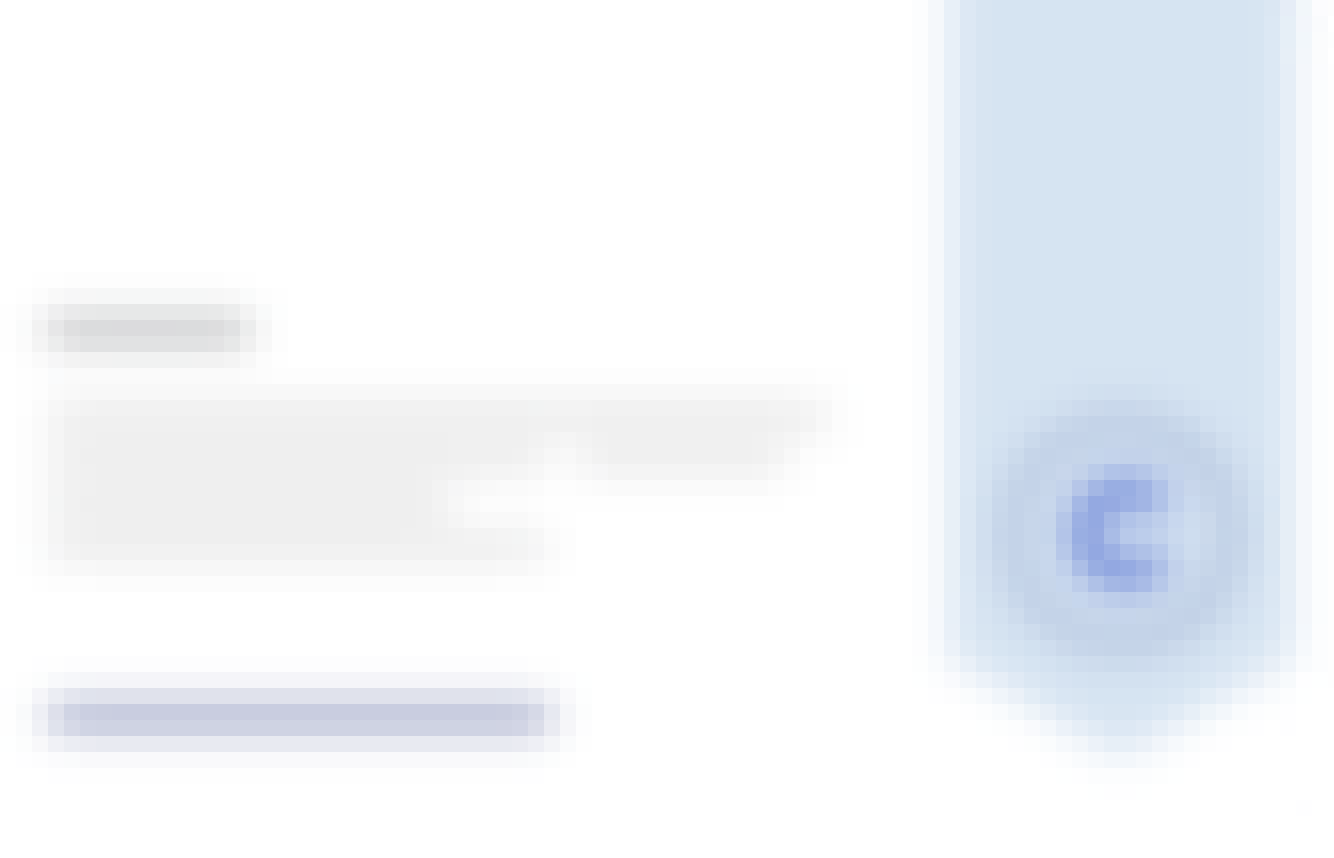
There are 5 modules in this course
This module focuses on defining causal effects using potential outcomes. A key distinction is made between setting/manipulating values and conditioning on variables. Key causal identifying assumptions are also introduced.
What's included
8 videos3 assignments
This module introduces directed acyclic graphs. By understanding various rules about these graphs, learners can identify whether a set of variables is sufficient to control for confounding.
What's included
8 videos2 assignments
An overview of matching methods for estimating causal effects is presented, including matching directly on confounders and matching on the propensity score. The ideas are illustrated with data analysis examples in R.
What's included
12 videos5 assignments
Inverse probability of treatment weighting, as a method to estimate causal effects, is introduced. The ideas are illustrated with an IPTW data analysis in R.
What's included
9 videos3 assignments
This module focuses on causal effect estimation using instrumental variables in both randomized trials with non-compliance and in observational studies. The ideas are illustrated with an instrumental variables analysis in R.
What's included
9 videos3 assignments
Instructor

Offered by
Why people choose Coursera for their career




Learner reviews
560 reviews
- 5 stars
76.96%
- 4 stars
19.28%
- 3 stars
1.96%
- 2 stars
0.71%
- 1 star
1.07%
Showing 3 of 560
Reviewed on Jul 14, 2018
Excellent course. Could use a small restructuring, as I had to go through the material more than once, but otherwise, very good material and presentation.
Reviewed on Nov 13, 2024
This is a great course to me! This course really helps me have a better understanding of what constitutes causal effects. I really appreciate him for this course!
Reviewed on Nov 29, 2017
The material is great. Just wished the professor was more active in the discussion forum. Have not showed up in the forum for weeks. At least there should be a TA or something.
Recommended if you're interested in Data Science
University of Illinois Urbana-Champaign
American Psychological Association
Johns Hopkins University

Open new doors with Coursera Plus
Unlimited access to 10,000+ world-class courses, hands-on projects, and job-ready certificate programs - all included in your subscription
Advance your career with an online degree
Earn a degree from world-class universities - 100% online
Join over 3,400 global companies that choose Coursera for Business
Upskill your employees to excel in the digital economy