This course will introduce the learner to applied machine learning, focusing more on the techniques and methods than on the statistics behind these methods. The course will start with a discussion of how machine learning is different than descriptive statistics, and introduce the scikit learn toolkit through a tutorial. The issue of dimensionality of data will be discussed, and the task of clustering data, as well as evaluating those clusters, will be tackled. Supervised approaches for creating predictive models will be described, and learners will be able to apply the scikit learn predictive modelling methods while understanding process issues related to data generalizability (e.g. cross validation, overfitting). The course will end with a look at more advanced techniques, such as building ensembles, and practical limitations of predictive models. By the end of this course, students will be able to identify the difference between a supervised (classification) and unsupervised (clustering) technique, identify which technique they need to apply for a particular dataset and need, engineer features to meet that need, and write python code to carry out an analysis.

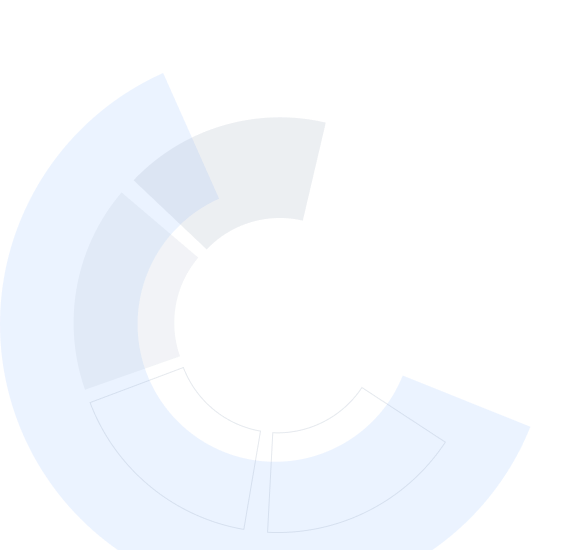
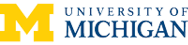
Applied Machine Learning in Python
This course is part of Applied Data Science with Python Specialization

Instructor: Kevyn Collins-Thompson
Sponsored by EdgePoint Software
313,316 already enrolled
(8,528 reviews)
What you'll learn
Describe how machine learning is different than descriptive statistics
Create and evaluate data clusters
Explain different approaches for creating predictive models
Build features that meet analysis needs
Skills you'll gain
Details to know

Add to your LinkedIn profile
4 assignments
See how employees at top companies are mastering in-demand skills
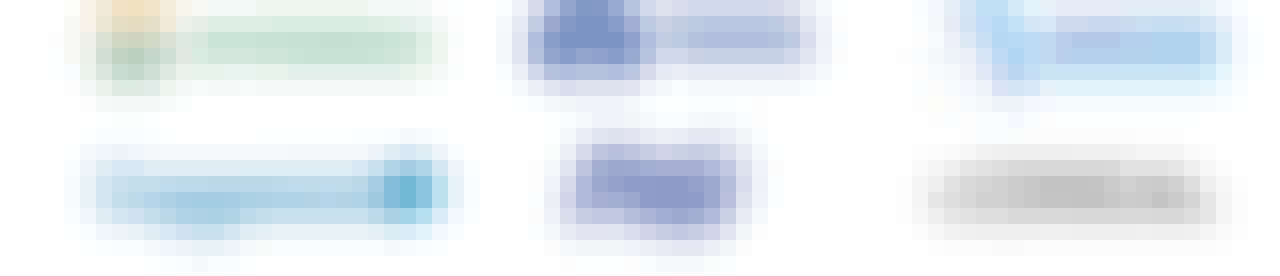
Build your subject-matter expertise
- Learn new concepts from industry experts
- Gain a foundational understanding of a subject or tool
- Develop job-relevant skills with hands-on projects
- Earn a shareable career certificate
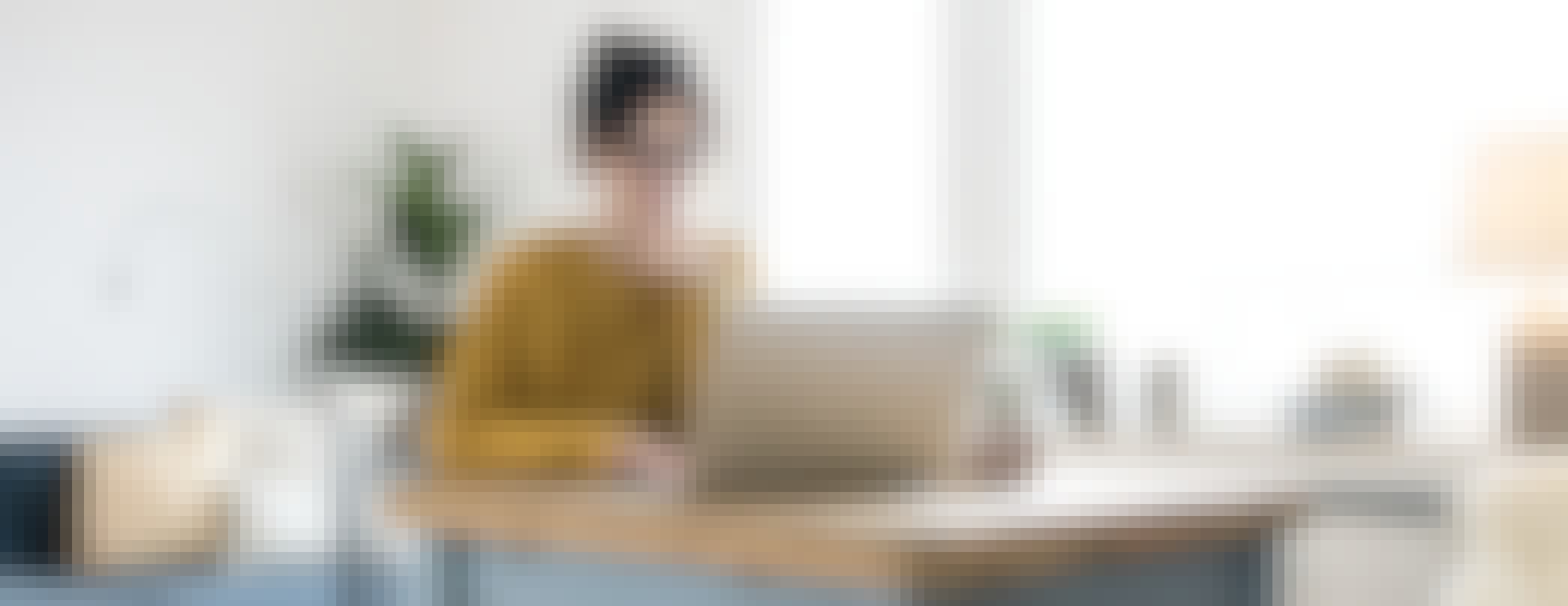
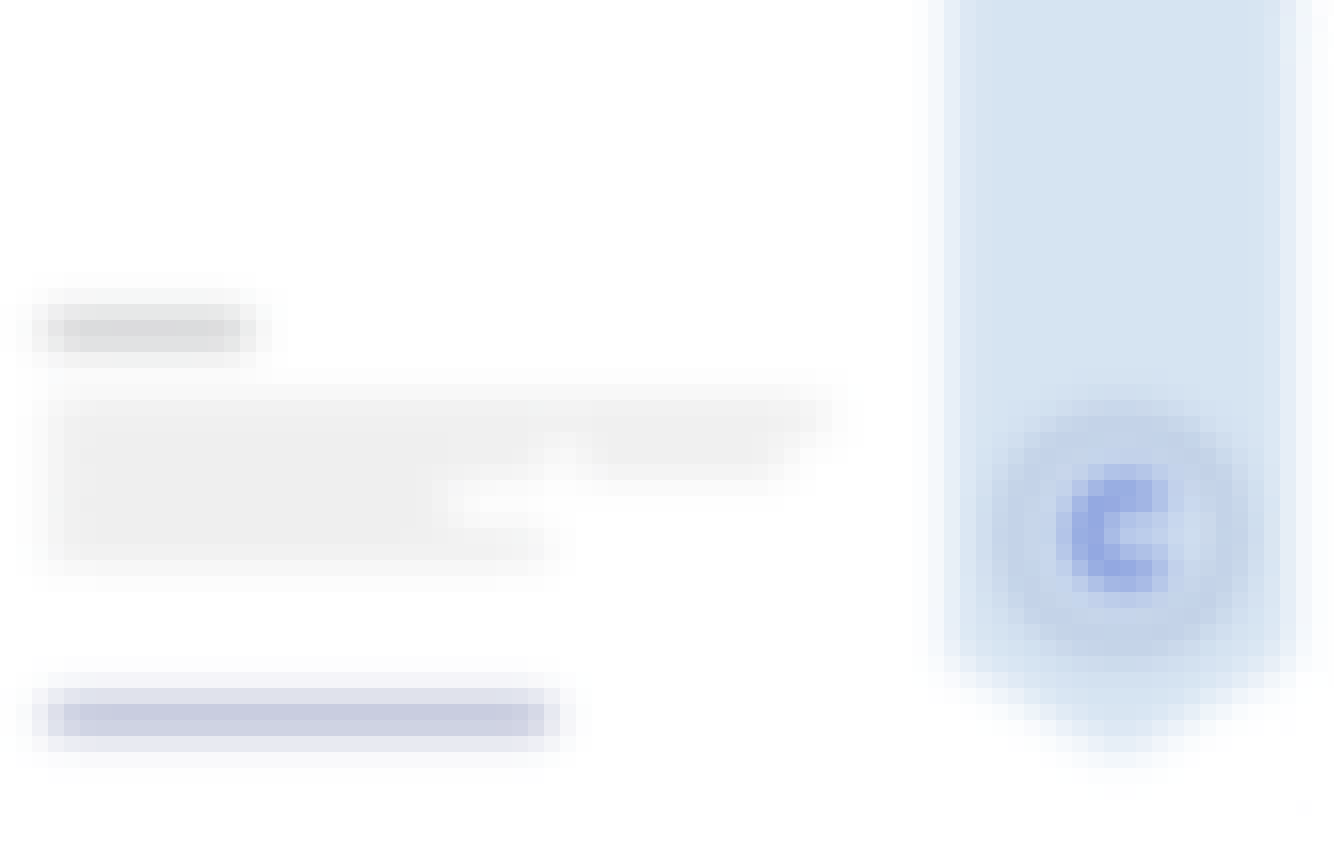
Earn a career certificate
Add this credential to your LinkedIn profile, resume, or CV
Share it on social media and in your performance review
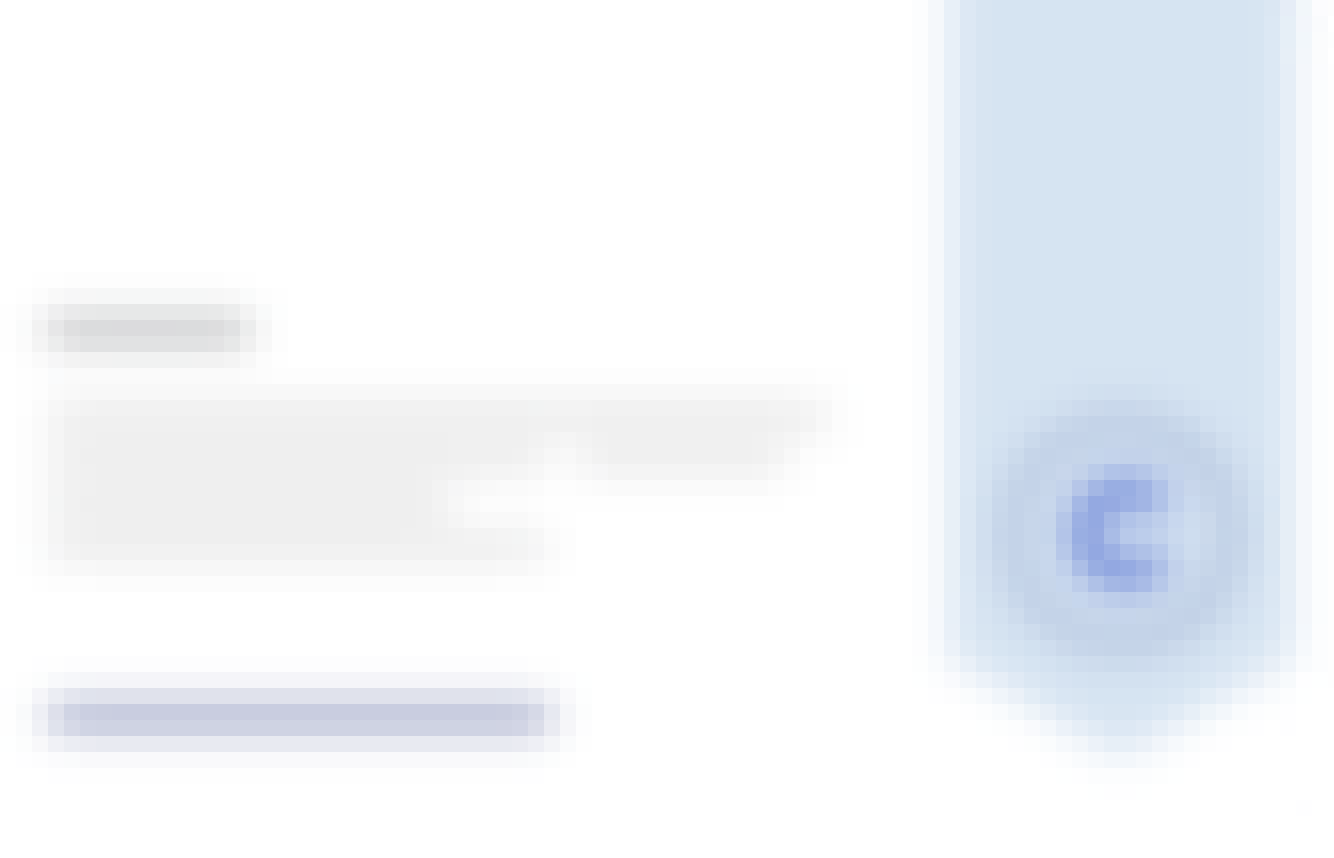
There are 4 modules in this course
This module introduces basic machine learning concepts, tasks, and workflow using an example classification problem based on the K-nearest neighbors method, and implemented using the scikit-learn library.
What's included
7 videos4 readings1 assignment1 programming assignment1 ungraded lab
This module delves into a wider variety of supervised learning methods for both classification and regression, learning about the connection between model complexity and generalization performance, the importance of proper feature scaling, and how to control model complexity by applying techniques like regularization to avoid overfitting. In addition to k-nearest neighbors, this week covers linear regression (least-squares, ridge, lasso, and polynomial regression), logistic regression, support vector machines, the use of cross-validation for model evaluation, and decision trees.
What's included
13 videos2 readings1 assignment1 programming assignment2 ungraded labs
This module covers evaluation and model selection methods that you can use to help understand and optimize the performance of your machine learning models.
What's included
8 videos2 readings1 assignment1 programming assignment1 ungraded lab
This module covers more advanced supervised learning methods that include ensembles of trees (random forests, gradient boosted trees), and neural networks (with an optional summary on deep learning). You will also learn about the critical problem of data leakage in machine learning and how to detect and avoid it.
What's included
10 videos13 readings1 assignment1 programming assignment2 ungraded labs
Instructor

Offered by
Why people choose Coursera for their career




Learner reviews
8,528 reviews
- 5 stars
71.68%
- 4 stars
20.95%
- 3 stars
4.88%
- 2 stars
1.18%
- 1 star
1.28%
Showing 3 of 8528
Reviewed on Oct 13, 2017
Very well structured course, and very interesting too! Has made me want to pursue a career in machine learning. I originally just wanted to learn to program, without true goal, now I have one thanks!!
Reviewed on Aug 19, 2018
Concise and clear presentation of the material with the majority of time focused around using TDD to learn and practice concepts through developing solutions to open ended coding challenges.
Reviewed on Jun 9, 2020
The course was really interesting to go through. All the related assignments whether be Quizzes or the Hands-On really test the knowledge. Kudos to the mentor for teaching us in in such a lucid way.
Recommended if you're interested in Data Science
Arizona State University
University of Michigan

Open new doors with Coursera Plus
Unlimited access to 10,000+ world-class courses, hands-on projects, and job-ready certificate programs - all included in your subscription
Advance your career with an online degree
Earn a degree from world-class universities - 100% online
Join over 3,400 global companies that choose Coursera for Business
Upskill your employees to excel in the digital economy