Statistical inference is the process of drawing conclusions about populations or scientific truths from data. There are many modes of performing inference including statistical modeling, data oriented strategies and explicit use of designs and randomization in analyses. Furthermore, there are broad theories (frequentists, Bayesian, likelihood, design based, …) and numerous complexities (missing data, observed and unobserved confounding, biases) for performing inference. A practitioner can often be left in a debilitating maze of techniques, philosophies and nuance. This course presents the fundamentals of inference in a practical approach for getting things done. After taking this course, students will understand the broad directions of statistical inference and use this information for making informed choices in analyzing data.

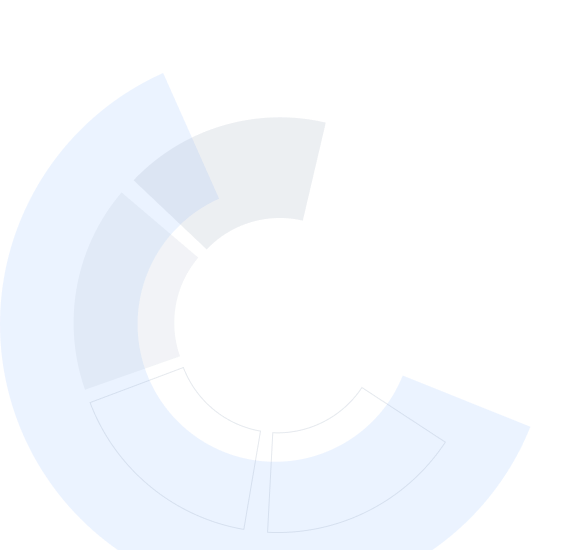
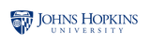
Statistical Inference
This course is part of multiple programs.



Instructors: Brian Caffo, PhD
Access provided by EdgePoint Software
184,201 already enrolled
(4,444 reviews)
What you'll learn
Understand the process of drawing conclusions about populations or scientific truths from data
Describe variability, distributions, limits, and confidence intervals
Use p-values, confidence intervals, and permutation tests
Make informed data analysis decisions
Skills you'll gain
Details to know

Add to your LinkedIn profile
4 assignments
See how employees at top companies are mastering in-demand skills
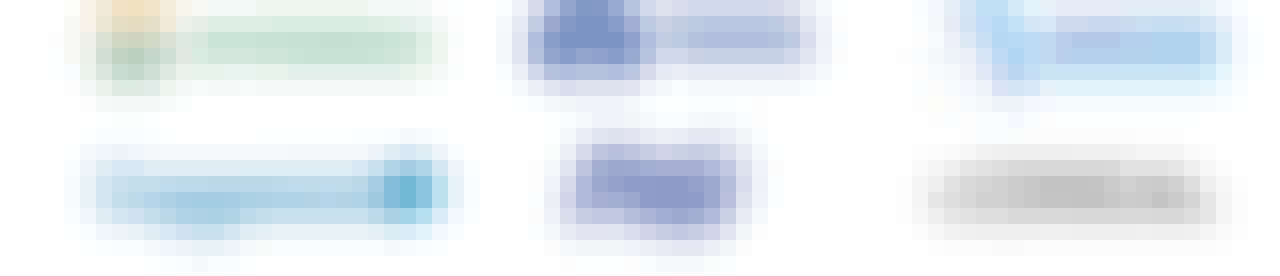
Build your subject-matter expertise
- Learn new concepts from industry experts
- Gain a foundational understanding of a subject or tool
- Develop job-relevant skills with hands-on projects
- Earn a shareable career certificate
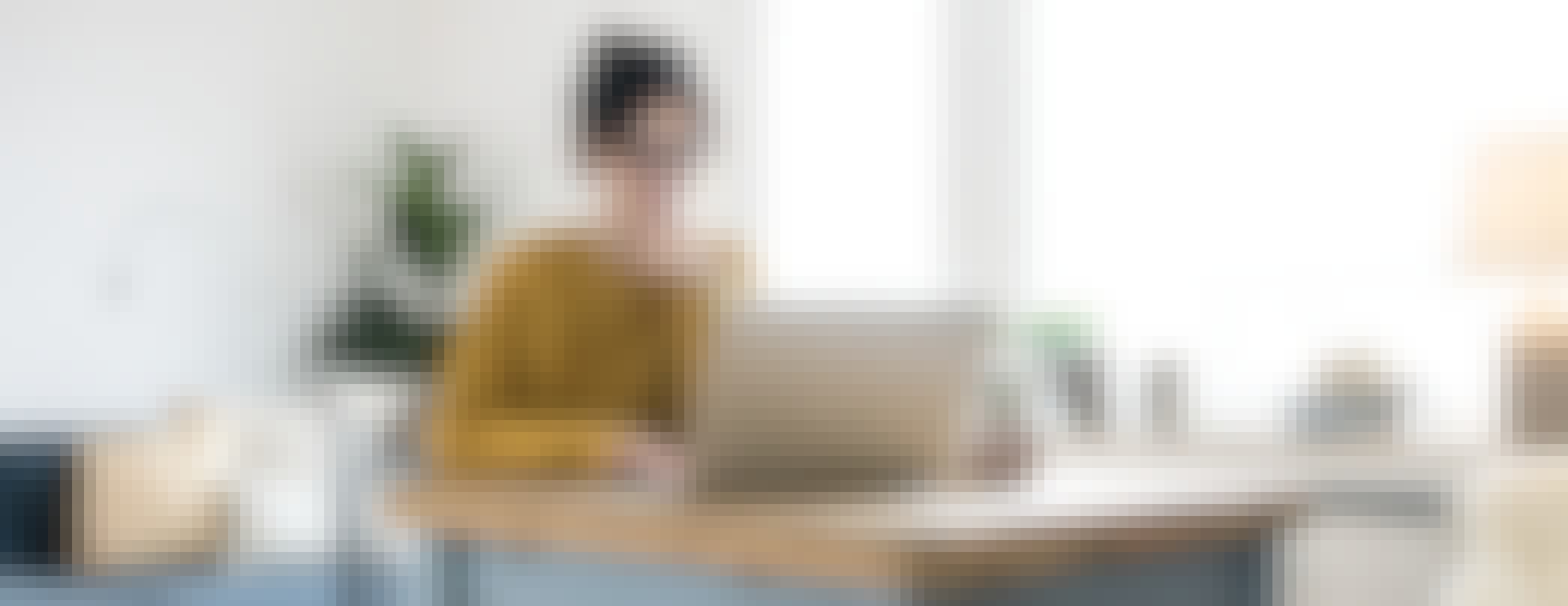
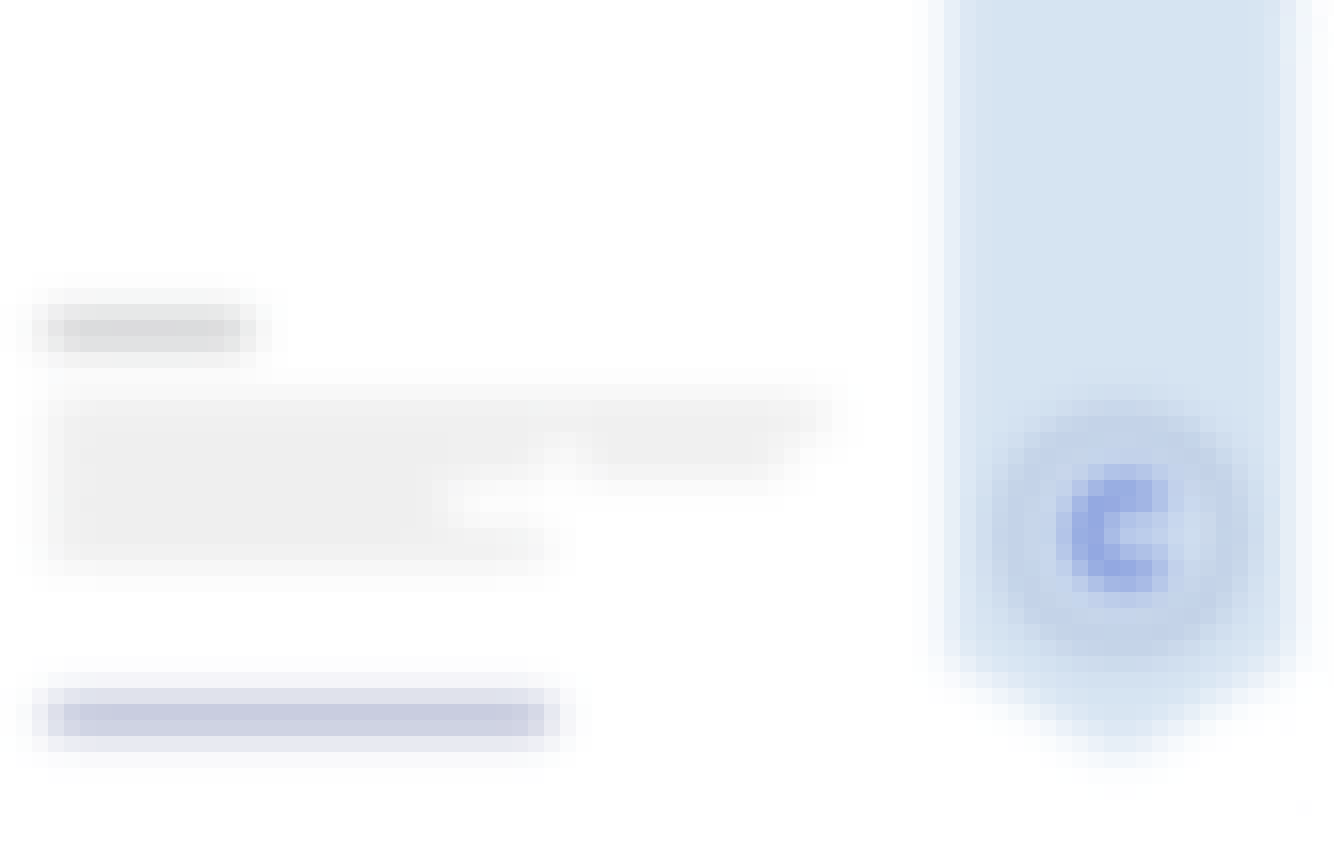
Earn a career certificate
Add this credential to your LinkedIn profile, resume, or CV
Share it on social media and in your performance review
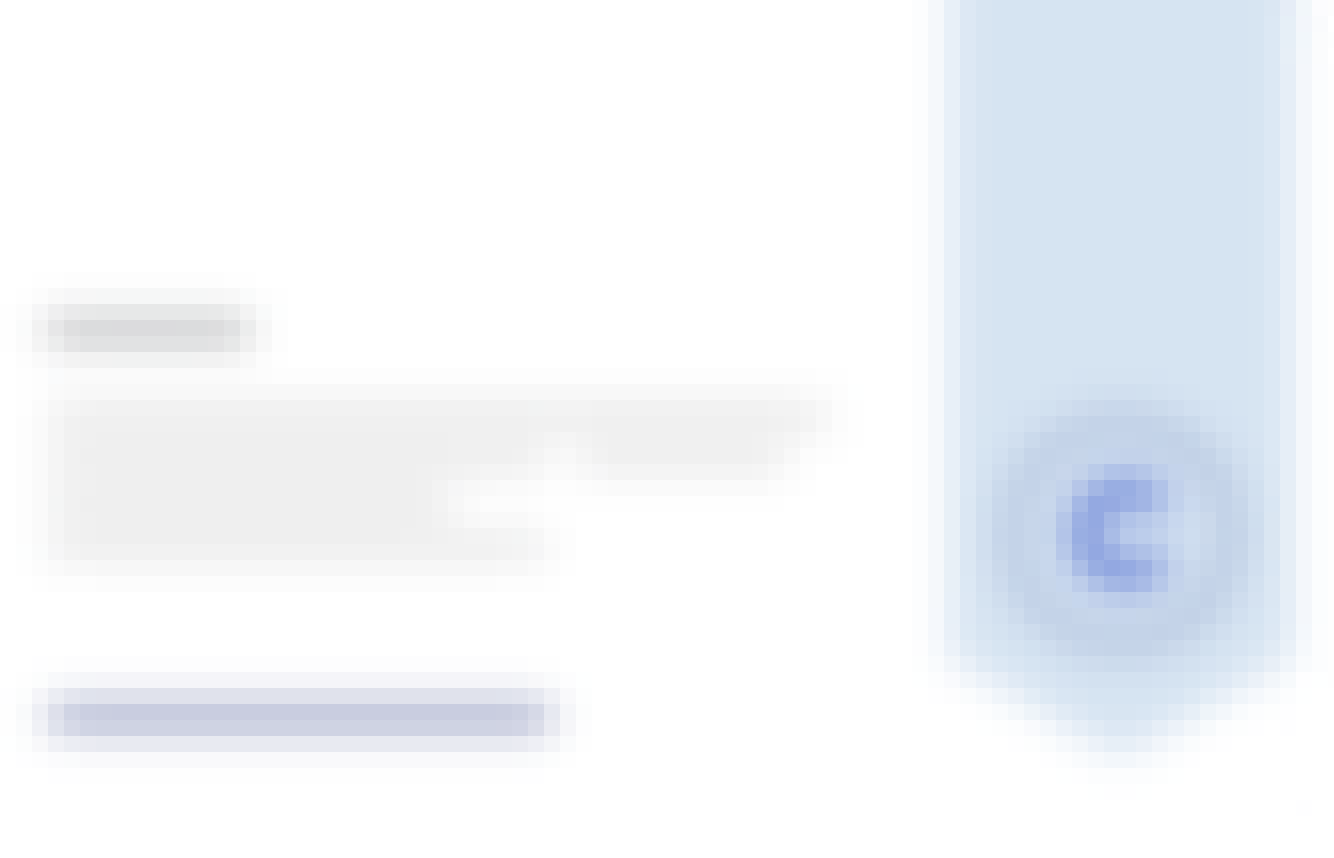
There are 4 modules in this course
This week, we'll focus on the fundamentals including probability, random variables, expectations and more.
What's included
10 videos11 readings1 assignment5 programming assignments
We're going to tackle variability, distributions, limits, and confidence intervals.
What's included
10 videos4 readings1 assignment3 programming assignments
We will be taking a look at intervals, testing, and pvalues in this lesson.
What's included
11 videos5 readings1 assignment3 programming assignments
We will begin looking into power, bootstrapping, and permutation tests.
What's included
9 videos4 readings1 assignment3 programming assignments1 peer review
Instructors

Offered by
Why people choose Coursera for their career




Learner reviews
4,444 reviews
- 5 stars
57.67%
- 4 stars
22.99%
- 3 stars
9.99%
- 2 stars
4.56%
- 1 star
4.77%
Showing 3 of 4444
Reviewed on Jul 8, 2021
Statistics was not, to put it mildly, my favorite subject in college. This class, however, managed to actually get me involved in the subject as it is tought with applicability in mind. Thank you.
Reviewed on May 22, 2017
Excellent course. After completion, I really feel like I have a great grasp of basic inferential statistics and this course introduced ideas that I had not even considered before.
Reviewed on May 10, 2020
This course explores many key statistical concepts, however you are expected to extend your learning beyond the course in order to fill in any foundational gaps in statistics.
Explore more from Data Science
Duke University
Eindhoven University of Technology
Duke University
Duke University

Open new doors with Coursera Plus
Unlimited access to 10,000+ world-class courses, hands-on projects, and job-ready certificate programs - all included in your subscription
Advance your career with an online degree
Earn a degree from world-class universities - 100% online
Join over 3,400 global companies that choose Coursera for Business
Upskill your employees to excel in the digital economy