This course will help us to evaluate and compare the models we have developed in previous courses. So far we have developed techniques for regression and classification, but how low should the error of a classifier be (for example) before we decide that the classifier is "good enough"? Or how do we decide which of two regression algorithms is better?

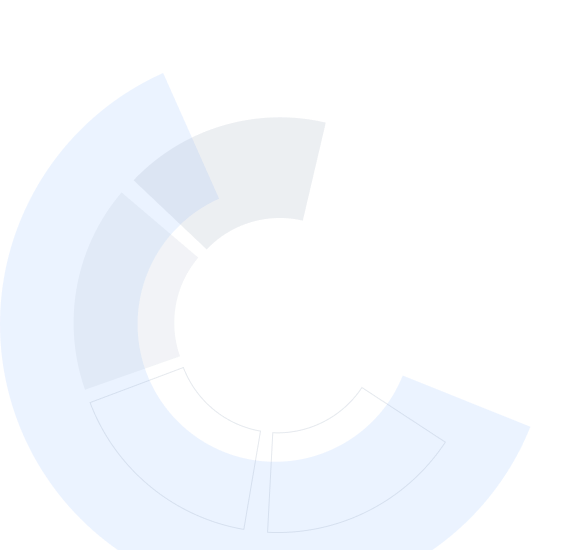

Meaningful Predictive Modeling
This course is part of Python Data Products for Predictive Analytics Specialization


Instructors: Julian McAuley
Sponsored by RIA PAYMENT INSTITUTION
6,320 already enrolled
(48 reviews)
What you'll learn
Understand the definitions of simple error measures (e.g. MSE, accuracy, precision/recall).
Evaluate the performance of regressors / classifiers using the above measures.
Understand the difference between training/testing performance, and generalizability.
Understand techniques to avoid overfitting and achieve good generalization performance.
Skills you'll gain
Details to know

Add to your LinkedIn profile
10 assignments
See how employees at top companies are mastering in-demand skills
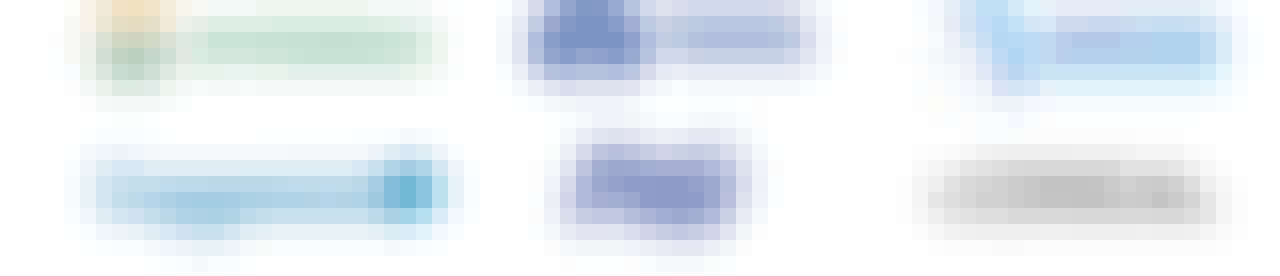
Build your subject-matter expertise
- Learn new concepts from industry experts
- Gain a foundational understanding of a subject or tool
- Develop job-relevant skills with hands-on projects
- Earn a shareable career certificate
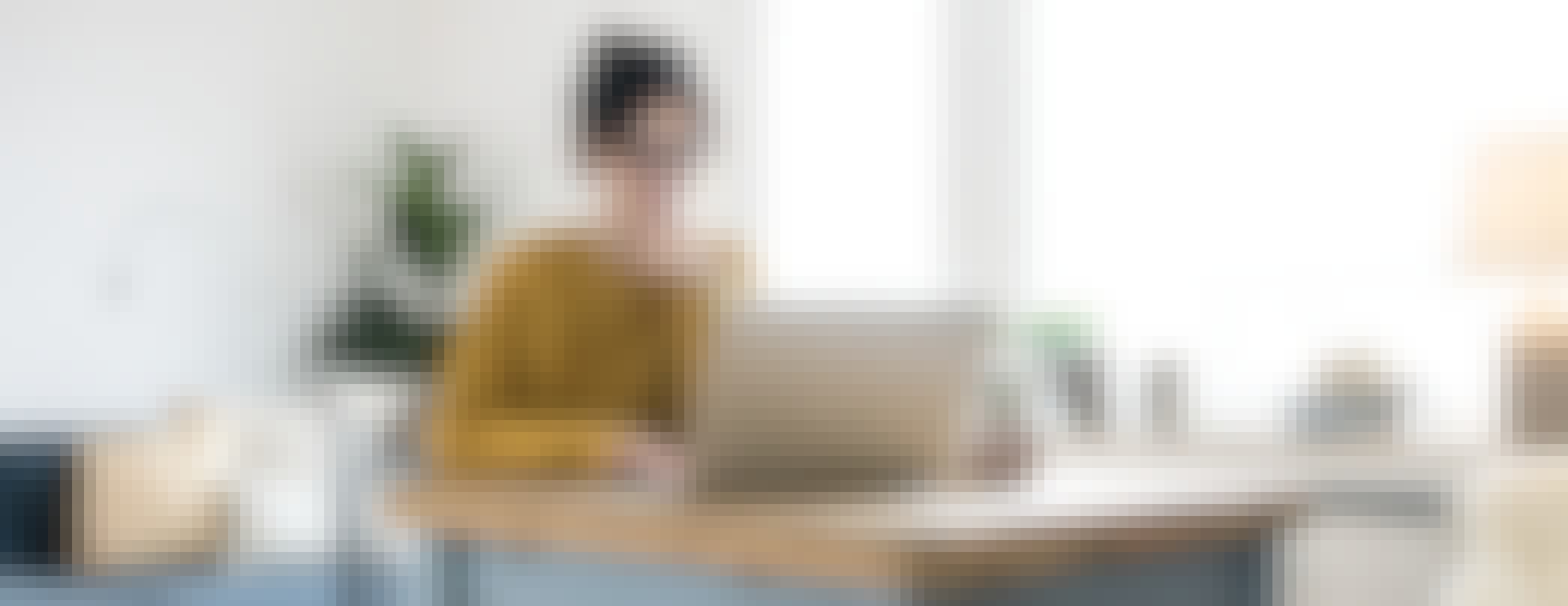
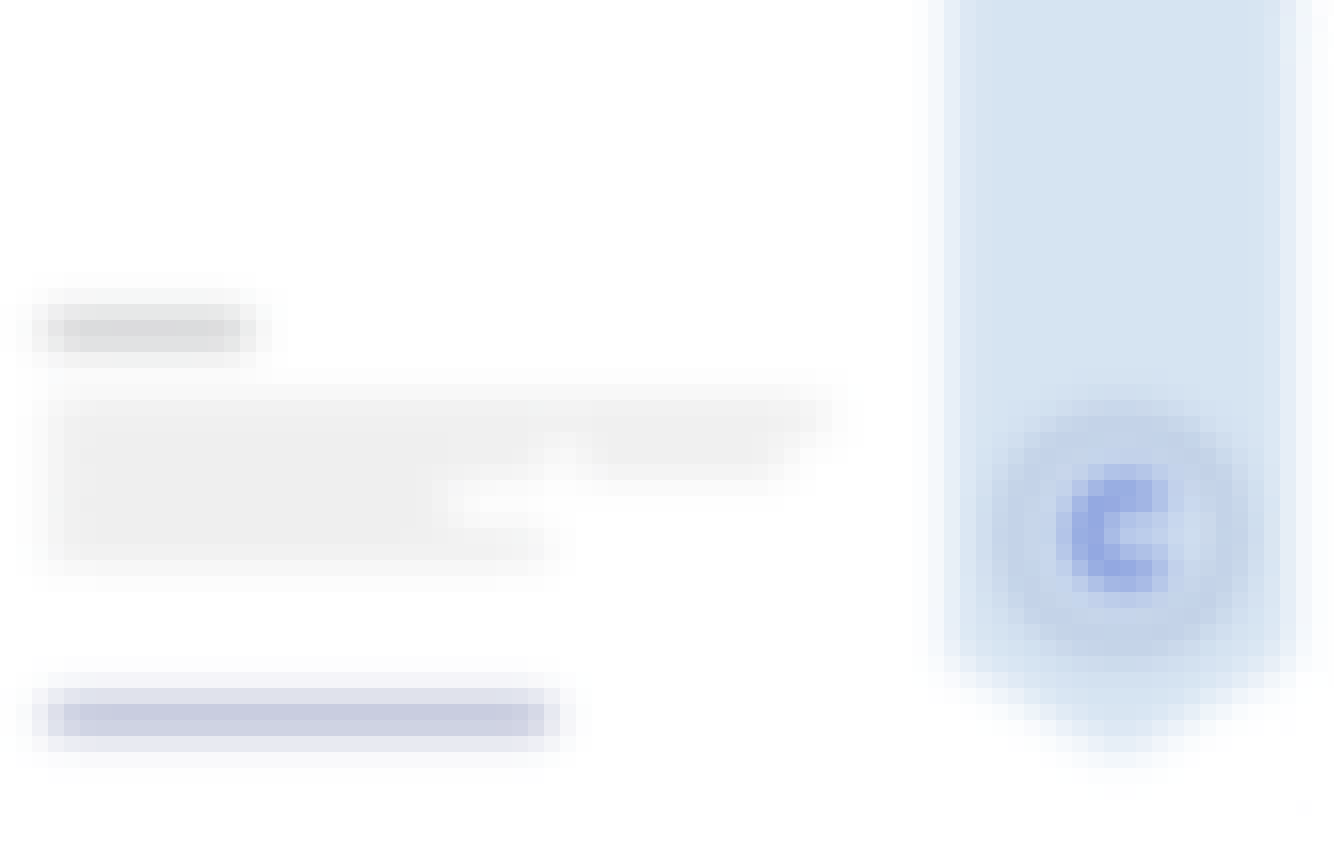
Earn a career certificate
Add this credential to your LinkedIn profile, resume, or CV
Share it on social media and in your performance review
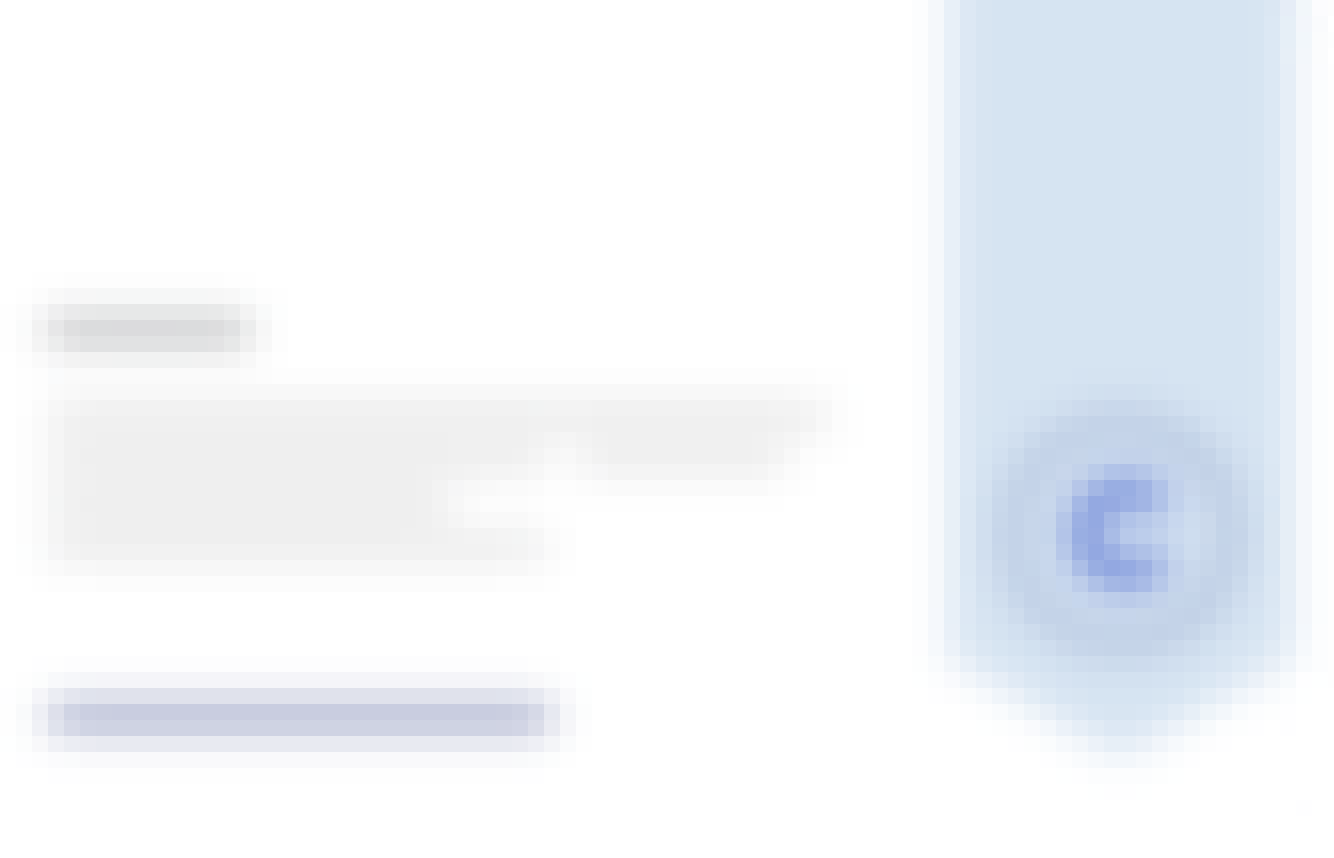
There are 4 modules in this course
For this first week, we will go over the syllabus, download all course materials, and get your system up and running for the course. We will also introduce the basics of diagnostics for the results of supervised learning.
What's included
6 videos4 readings3 assignments2 discussion prompts
This week, we will learn how to create a simple bag of words for analysis. We will also cover regularization and why it matters when building a model. Lastly, we will evaluate a model with regularization, focusing on classifiers.
What's included
4 videos4 assignments
This week, we will learn about validation and how to implement it in tandem with training and testing. We will also cover how to implement a regularization pipeline in Python and introduce a few guidelines for best practices.
What's included
4 videos3 assignments
In the final week of this course, you will continue building on the project from the first and second courses of Python Data Products for Predictive Analytics with simple predictive machine learning algorithms. Find a dataset, clean it, and perform basic analyses on the data. Evaluate your model, validate your analyses, and make sure you aren't overfitting the data.
What's included
2 readings1 peer review1 discussion prompt
Instructors

Offered by
Why people choose Coursera for their career




Learner reviews
48 reviews
- 5 stars
56.25%
- 4 stars
25%
- 3 stars
12.50%
- 2 stars
4.16%
- 1 star
2.08%
Showing 3 of 48
Reviewed on Nov 16, 2019
Excellent content, but presentation is a bit challenging at times.
Reviewed on Mar 31, 2021
The course provided a lot of insights into predictive modeling.
Recommended if you're interested in Data Science
University of Illinois Urbana-Champaign
University of Washington
Google Cloud
Alberta Machine Intelligence Institute

Open new doors with Coursera Plus
Unlimited access to 10,000+ world-class courses, hands-on projects, and job-ready certificate programs - all included in your subscription
Advance your career with an online degree
Earn a degree from world-class universities - 100% online
Join over 3,400 global companies that choose Coursera for Business
Upskill your employees to excel in the digital economy