Unlock the full potential of PyTorch with this comprehensive course designed for advanced users. Starting with Recommender Systems, you’ll explore how to build and evaluate these models, incorporating user and item information to enhance recommendations. Moving on to Autoencoders, the course guides you through their fundamentals and practical implementation, providing a solid foundation for dimensionality reduction and data compression tasks.

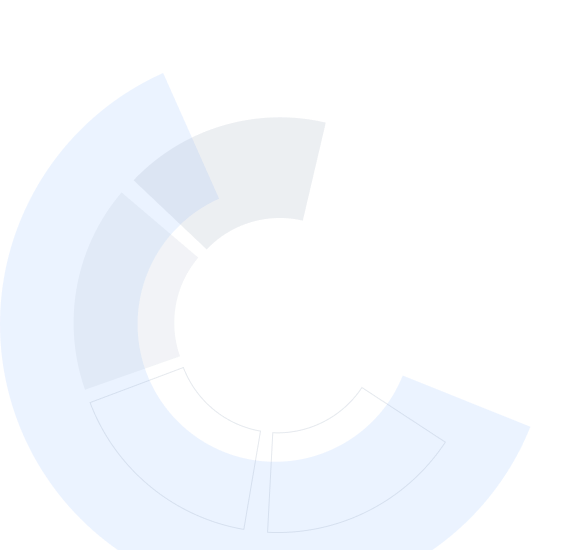
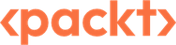
Advanced PyTorch Techniques and Applications
This course is part of PyTorch Ultimate 2024 - From Basics to Cutting-Edge Specialization

Instructor: Packt - Course Instructors
Sponsored by Pontificia Universidad Católica del Perú
Recommended experience
Recommended experience
What you'll learn
Create and assess ML models for specific datasets, evaluating performance with proper metrics.
Design autoencoders for dimensionality reduction and build GANs for data simulation, analyzing quality.
Develop Graph Neural Networks for graph data and implement Transformers, including Vision Transformers.
Enhance models with semi-supervised learning using limited data, and deploy them with Flask on Google Cloud.
Details to know

Add to your LinkedIn profile
5 assignments
September 2024
See how employees at top companies are mastering in-demand skills
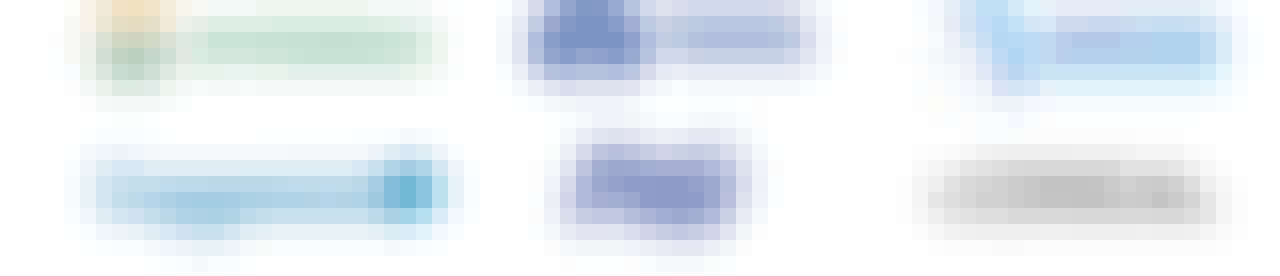
Build your subject-matter expertise
- Learn new concepts from industry experts
- Gain a foundational understanding of a subject or tool
- Develop job-relevant skills with hands-on projects
- Earn a shareable career certificate
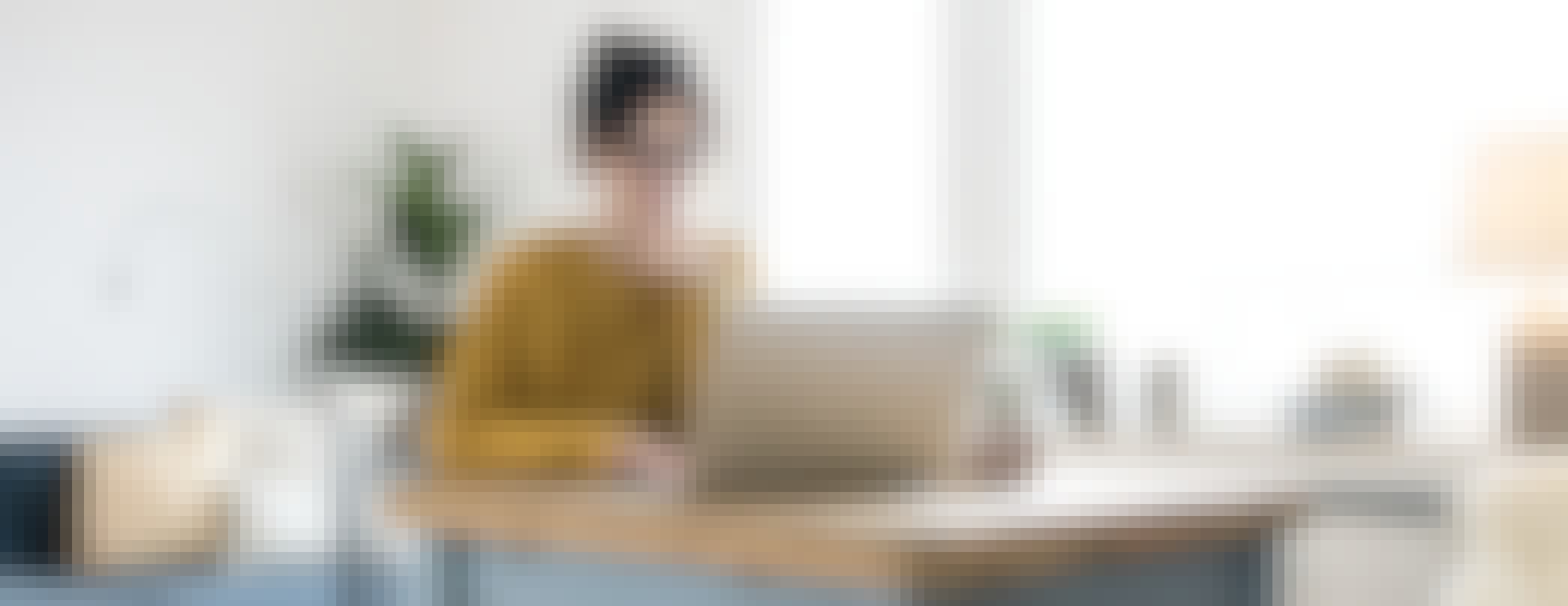
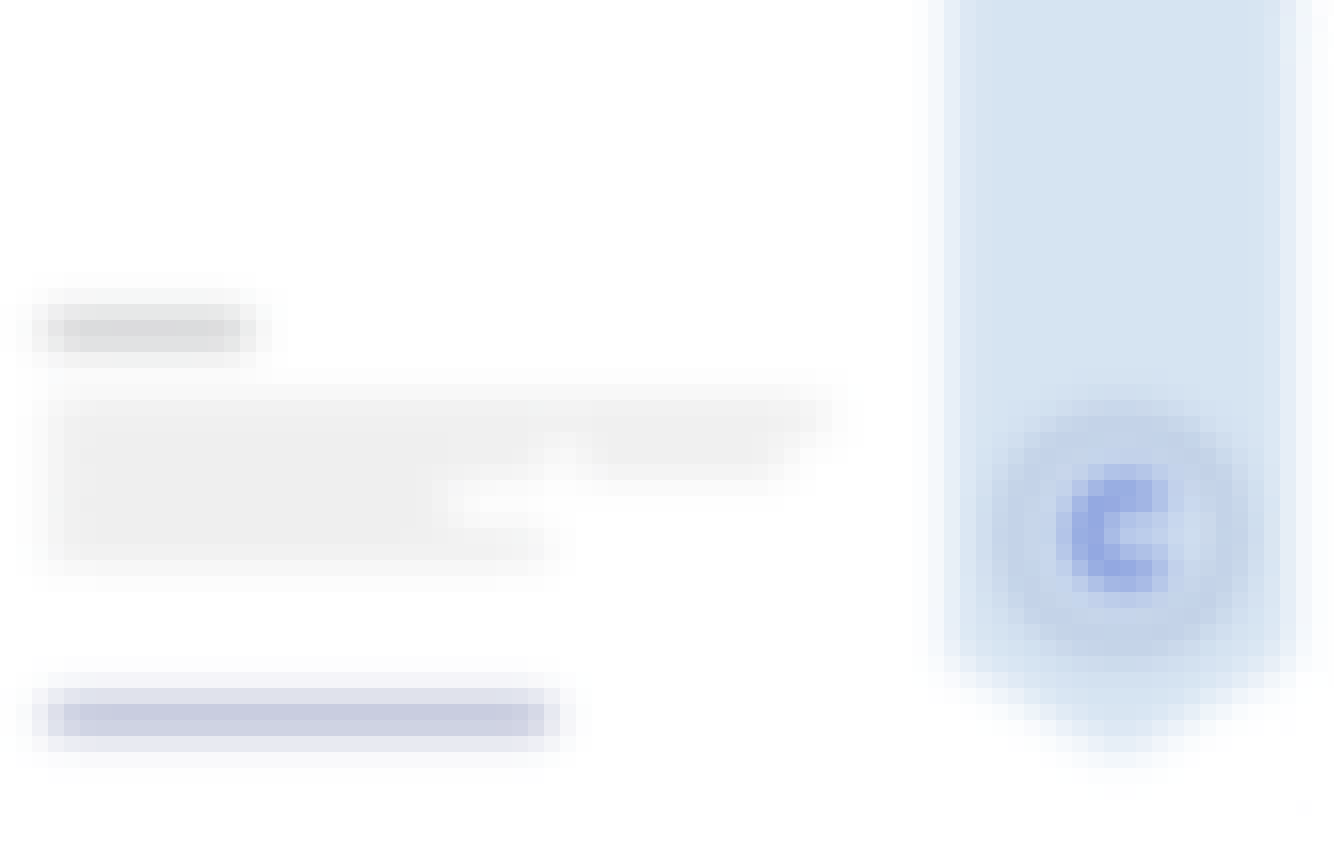
Earn a career certificate
Add this credential to your LinkedIn profile, resume, or CV
Share it on social media and in your performance review
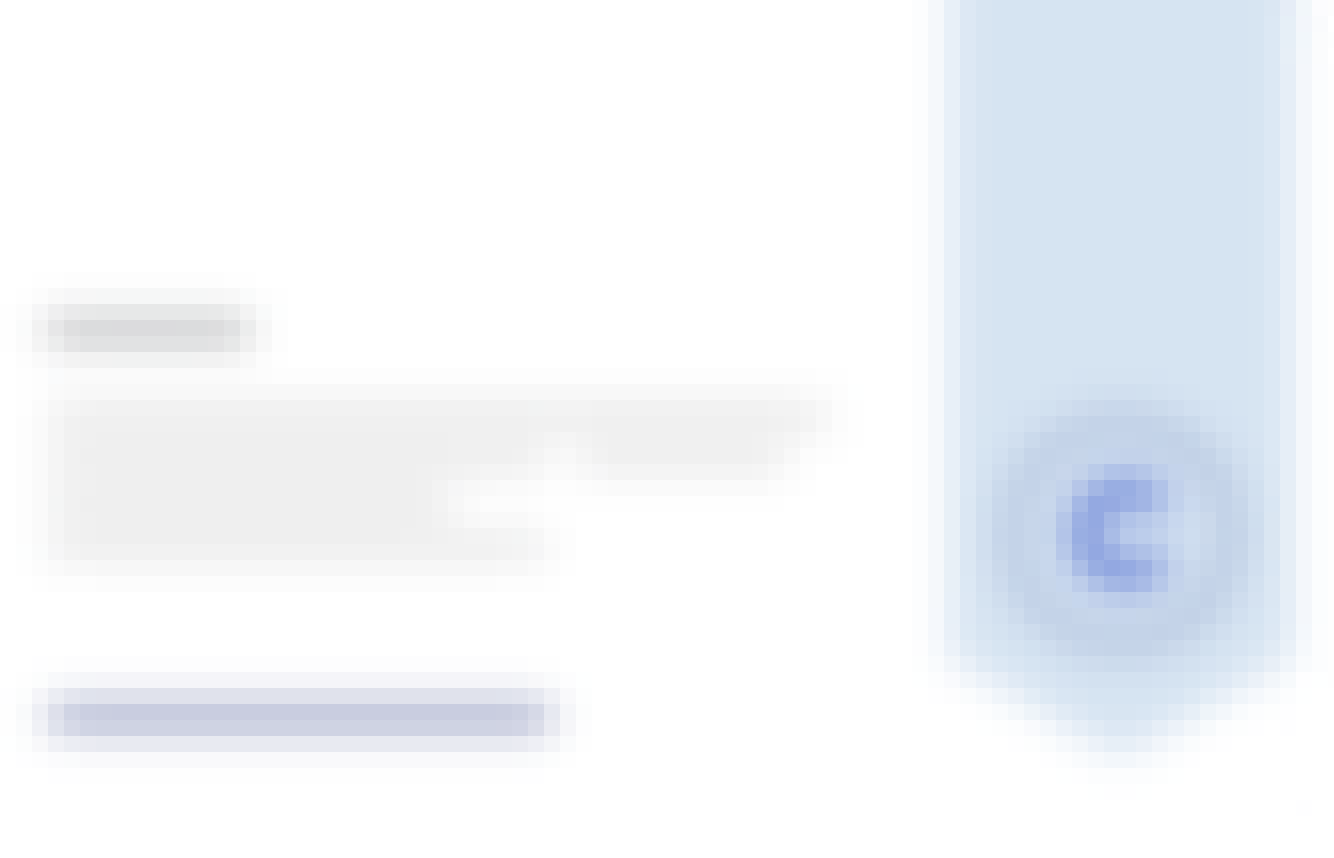
There are 12 modules in this course
In this module, we will explore the basics of recommender systems, starting from foundational concepts and progressing through hands-on coding exercises. You'll create datasets, develop and train models, and learn how to incorporate user and item information for improved recommendations. Finally, we will implement evaluation metrics to measure the system's performance.
What's included
5 videos2 readings
In this module, we will dive into autoencoders, covering both theoretical aspects and practical implementations. You will gain a solid understanding of how autoencoders work, their applications, and get hands-on experience coding these models.
What's included
3 videos
In this module, we will cover the essentials of generative adversarial networks, including an overview of their principles and coding implementations. You will learn to develop a GAN model and engage in exercises that challenge you to apply these techniques to specific tasks.
What's included
4 videos1 assignment
In this module, we will explore graph neural networks, starting with the basics and moving through coding implementations. You'll learn how to prepare data, train models, and evaluate their performance, all within the context of GNNs.
What's included
5 videos
In this module, we will delve into Transformers, beginning with foundational concepts and then focusing on their application to vision tasks. You'll gain hands-on experience in implementing and training a Vision Transformer on a custom dataset.
What's included
3 videos
In this module, we will introduce you to PyTorch Lightning, a powerful framework for PyTorch model development. You'll learn the basics, implement models, and explore techniques such as early stopping to optimize your training processes.
What's included
4 videos1 assignment
In this module, we will cover semi-supervised learning, beginning with foundational concepts and progressing through practical implementations. You will learn about supervised reference models, set up datasets, and develop models that effectively utilize both labeled and unlabeled data.
What's included
4 videos
In this module, we will explore the vast field of Natural Language Processing, from fundamental concepts to hands-on coding implementations. You'll learn to work with word embeddings, sentiment analysis, pre-trained models, and advanced topics like zero-shot classification and vector databases.
What's included
20 videos
In this module, we will cover a range of miscellaneous topics in machine learning, including architectures like ResNet and Inception, and concepts such as Extreme Learning Machines. Each topic will include both theoretical understanding and practical coding exercises.
What's included
6 videos1 assignment
In this module, we will focus on model debugging techniques, specifically using hooks. You'll learn the theoretical aspects and get hands-on experience implementing hooks to troubleshoot and optimize your models.
What's included
2 videos
In this module, we will explore the essentials of model deployment, covering both on-premise and cloud-based strategies. You'll learn to deploy models using Flask, consume data from APIs, and utilize Google Cloud for deploying model weights and REST APIs.
What's included
6 videos1 assignment
In this module, we will conclude the course by summarizing key concepts and techniques covered throughout. Additionally, we will provide resources and recommendations for further learning to help you continue your journey in advanced PyTorch techniques and applications.
What's included
1 video1 reading1 assignment
Instructor

Offered by
Why people choose Coursera for their career




Recommended if you're interested in Computer Science
École Polytechnique Fédérale de Lausanne
Johns Hopkins University
MathWorks
MathWorks

Open new doors with Coursera Plus
Unlimited access to 10,000+ world-class courses, hands-on projects, and job-ready certificate programs - all included in your subscription
Advance your career with an online degree
Earn a degree from world-class universities - 100% online
Join over 3,400 global companies that choose Coursera for Business
Upskill your employees to excel in the digital economy