This course introduces simple and multiple linear regression models. These models allow you to assess the relationship between variables in a data set and a continuous response variable. Is there a relationship between the physical attractiveness of a professor and their student evaluation scores? Can we predict the test score for a child based on certain characteristics of his or her mother? In this course, you will learn the fundamental theory behind linear regression and, through data examples, learn to fit, examine, and utilize regression models to examine relationships between multiple variables, using the free statistical software R and RStudio.

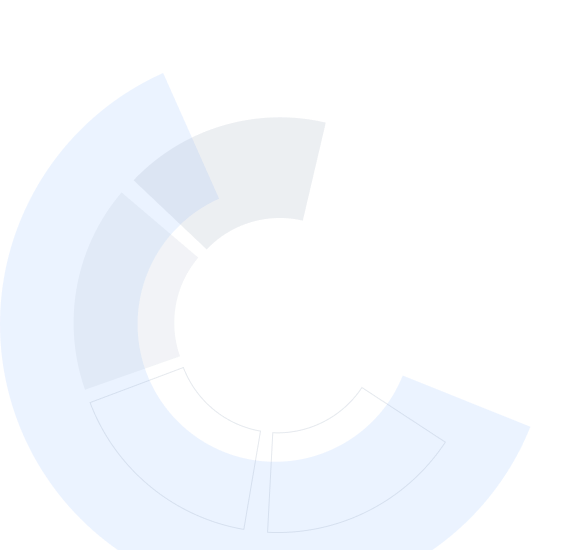
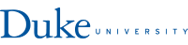
Linear Regression and Modeling
This course is part of Data Analysis with R Specialization
Instructor: Mine Çetinkaya-Rundel
Sponsored by Barbados NTI
98,731 already enrolled
(1,734 reviews)
Details to know

Add to your LinkedIn profile
8 assignments
See how employees at top companies are mastering in-demand skills
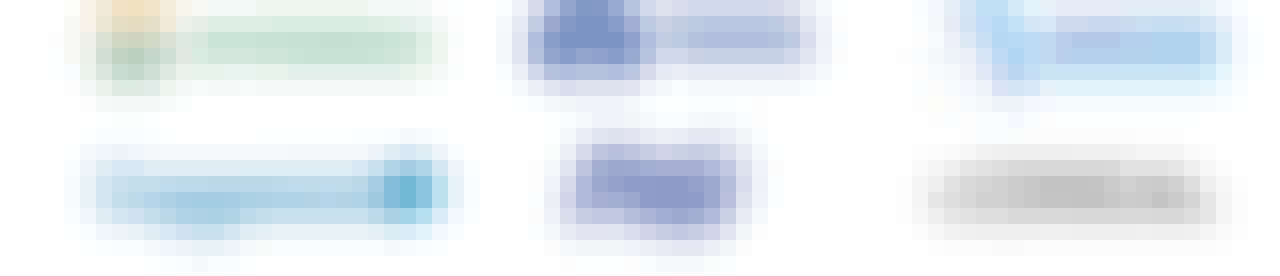
Build your subject-matter expertise
- Learn new concepts from industry experts
- Gain a foundational understanding of a subject or tool
- Develop job-relevant skills with hands-on projects
- Earn a shareable career certificate
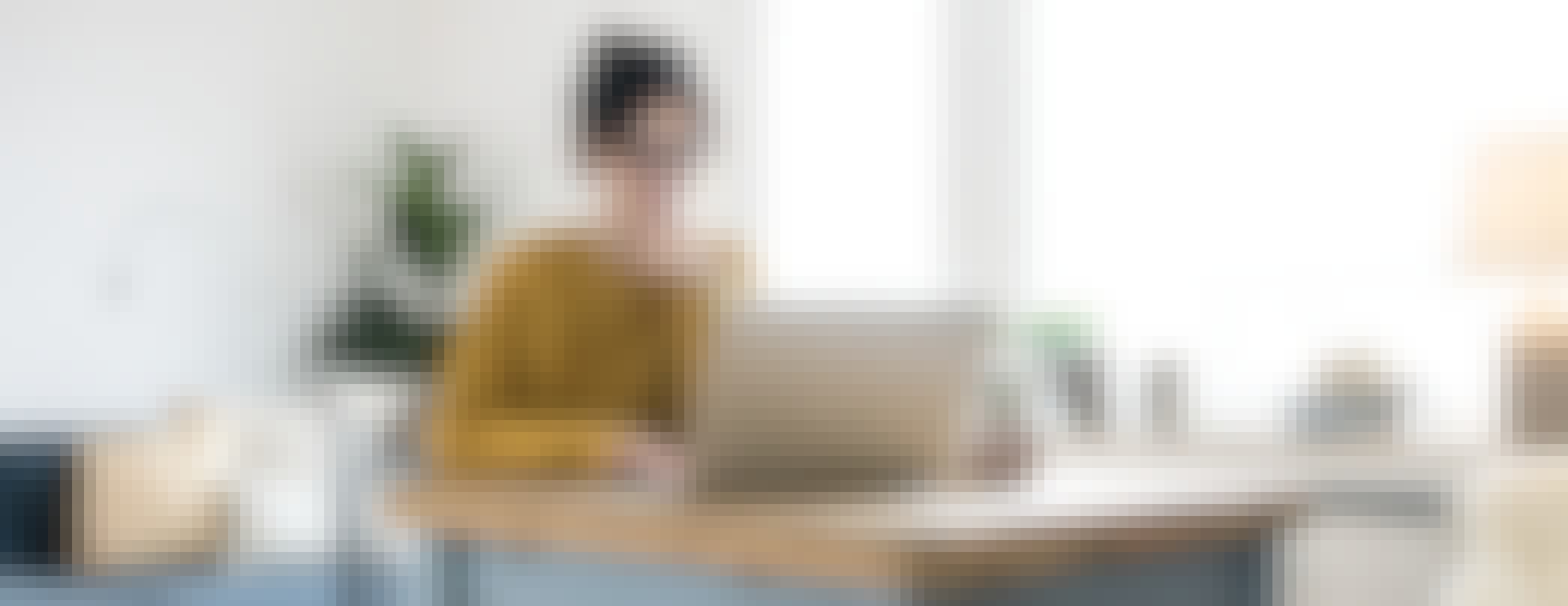
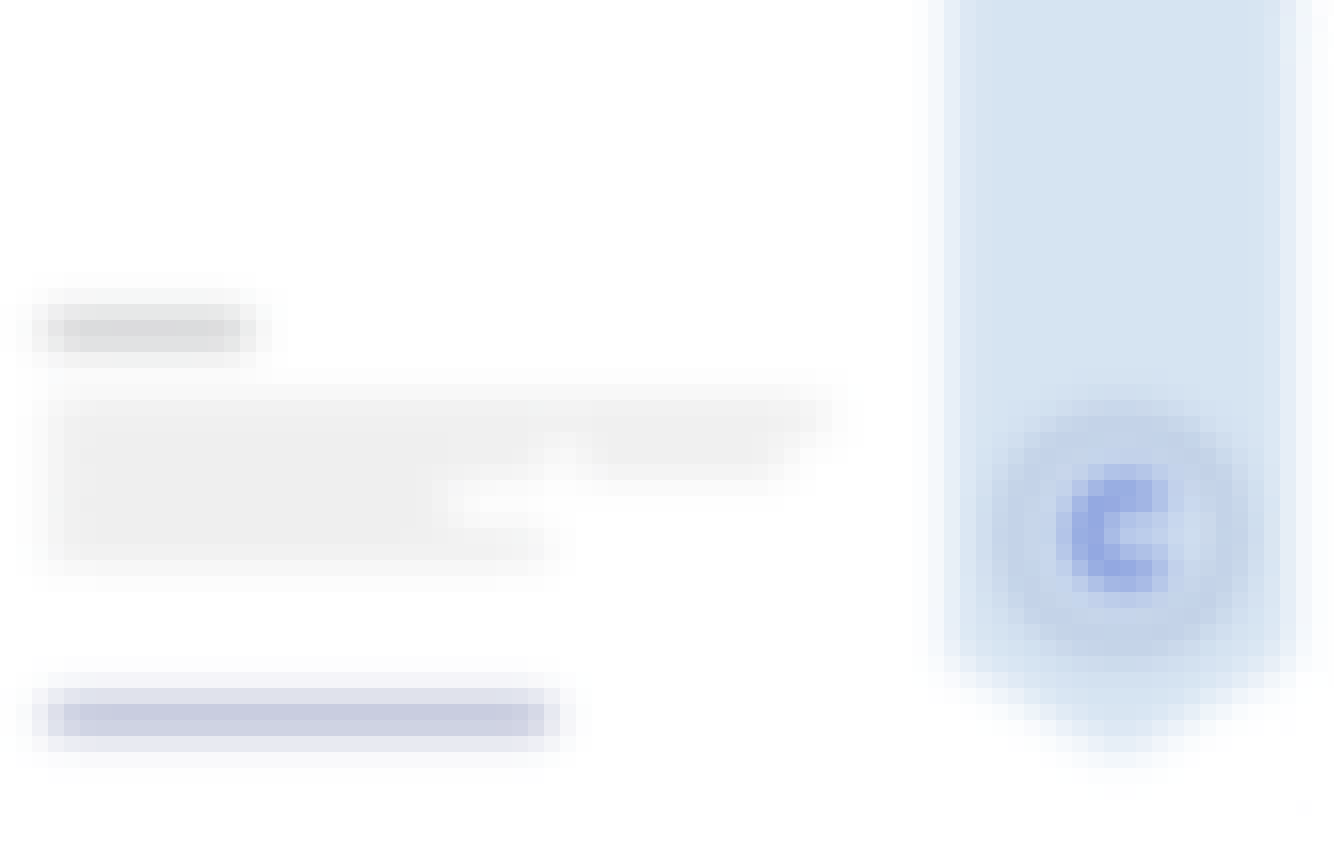
Earn a career certificate
Add this credential to your LinkedIn profile, resume, or CV
Share it on social media and in your performance review
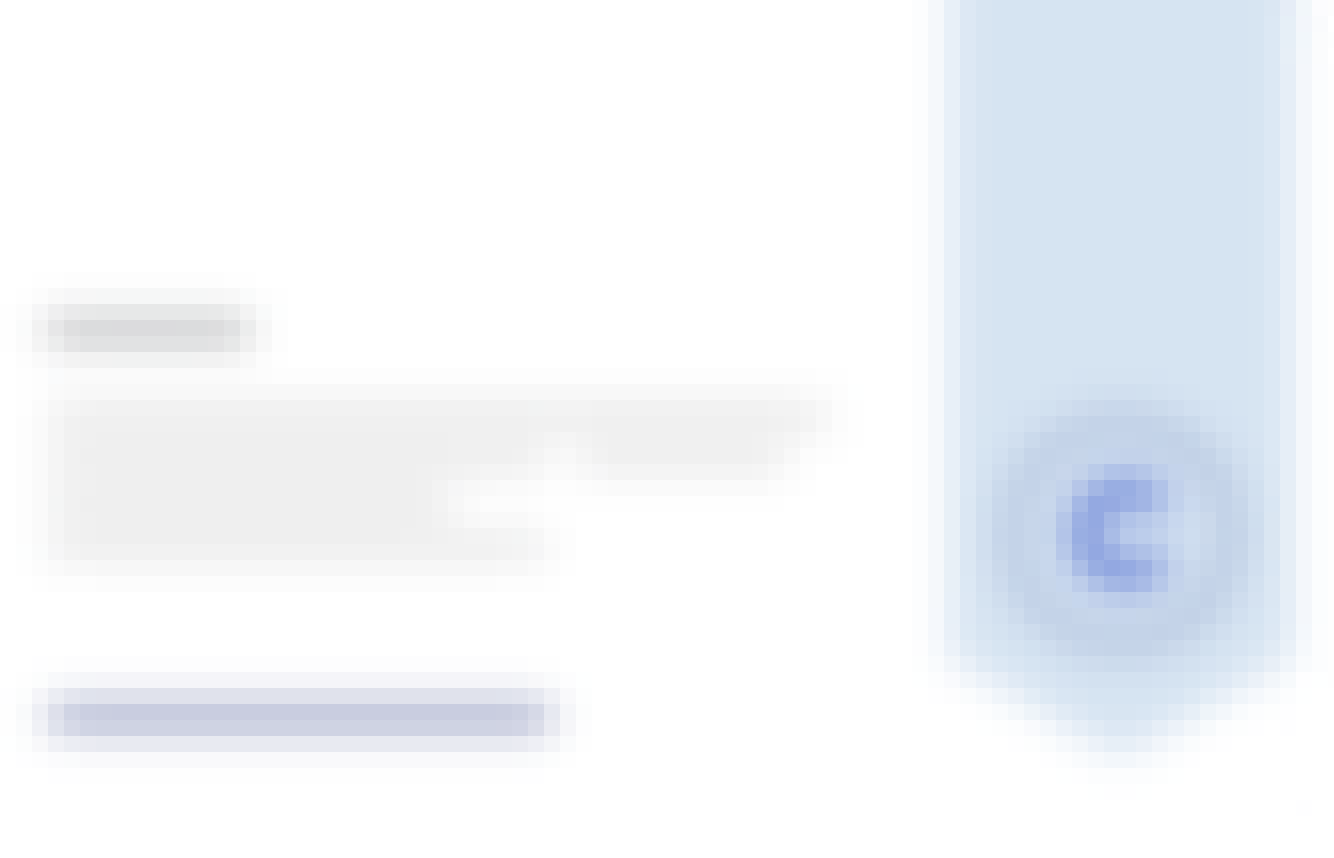
There are 4 modules in this course
This short module introduces basics about Coursera specializations and courses in general, this specialization: Statistics with R, and this course: Linear Regression and Modeling. Please take several minutes to browse them through. Thanks for joining us in this course!
What's included
1 video2 readings
In this week we’ll introduce linear regression. Many of you may be familiar with regression from reading the news, where graphs with straight lines are overlaid on scatterplots. Linear models can be used for prediction or to evaluate whether there is a linear relationship between two numerical variables.
What's included
8 videos3 readings2 assignments
Welcome to week 2! In this week, we will look at outliers, inference in linear regression and variability partitioning. Please use this week to strengthen your understanding on linear regression. Don't forget to post your questions, concerns and suggestions in the discussion forum!
What's included
3 videos5 readings3 assignments
In this week, we’ll explore multiple regression, which allows us to model numerical response variables using multiple predictors (numerical and categorical). We will also cover inference for multiple linear regression, model selection, and model diagnostics. There is also a final project included in this week. You will use the data set provided to complete and report on a data analysis question. Please read the project instructions to complete this self-assessment.
What's included
7 videos6 readings3 assignments
Instructor
Offered by
Why people choose Coursera for their career




Learner reviews
1,734 reviews
- 5 stars
80.50%
- 4 stars
15.85%
- 3 stars
2.88%
- 2 stars
0.23%
- 1 star
0.51%
Showing 3 of 1734
Reviewed on Sep 14, 2017
fantastic course on linear regression, concepts are well explained followed by quiz and practical exercises.
Reviewed on Dec 11, 2018
Files for this course were broken and I faced a lot of trouble to find good one. This course may be made more comprehensive and not assuming that reader have also understanding.
Reviewed on Dec 13, 2020
I learn a lot. It added more lessons beyond my graduate school. Especially that the course is based on R, this course is very helpful for my journey towards using R.
Recommended if you're interested in Data Science
University of Michigan
University of California San Diego
CertNexus
University of Pennsylvania

Open new doors with Coursera Plus
Unlimited access to 10,000+ world-class courses, hands-on projects, and job-ready certificate programs - all included in your subscription
Advance your career with an online degree
Earn a degree from world-class universities - 100% online
Join over 3,400 global companies that choose Coursera for Business
Upskill your employees to excel in the digital economy