This intermediate-level course introduces the mathematical foundations to derive Principal Component Analysis (PCA), a fundamental dimensionality reduction technique. We'll cover some basic statistics of data sets, such as mean values and variances, we'll compute distances and angles between vectors using inner products and derive orthogonal projections of data onto lower-dimensional subspaces. Using all these tools, we'll then derive PCA as a method that minimizes the average squared reconstruction error between data points and their reconstruction.

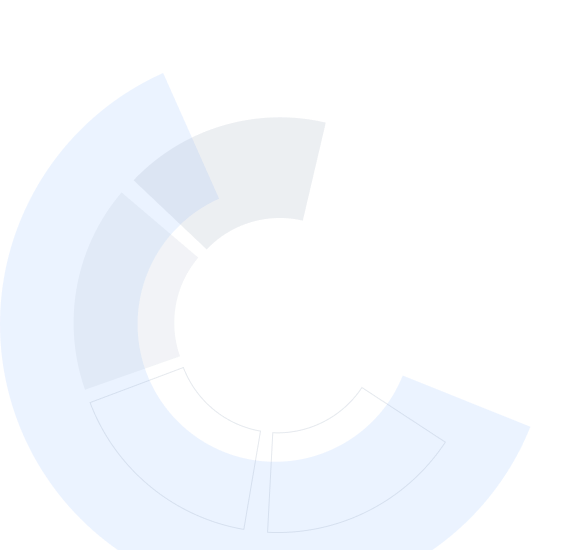
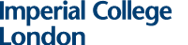
Mathematics for Machine Learning: PCA
This course is part of Mathematics for Machine Learning Specialization

Instructor: Marc Peter Deisenroth
Sponsored by Barbados NTI
92,951 already enrolled
(3,106 reviews)
What you'll learn
Implement mathematical concepts using real-world data
Derive PCA from a projection perspective
Understand how orthogonal projections work
Master PCA
Skills you'll gain
- Computer Science
- Unsupervised Learning
- NumPy
- Python Programming
- Data Science
- Statistical Modeling
- Exploratory Data Analysis
- General Mathematics
- Machine Learning Methods
- Mathematical Modeling
- Machine Learning
- Dimensionality Reduction
- Analytics
- Probability & Statistics
- Applied Mathematics
- Mathematics and Mathematical Modeling
- Statistics
- Linear Algebra
- Data Analysis
- Statistical Analysis
Details to know

Add to your LinkedIn profile
11 assignments
See how employees at top companies are mastering in-demand skills
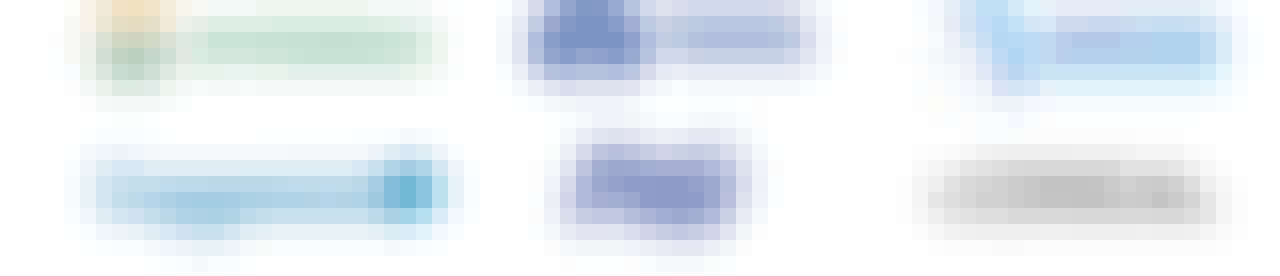
Build your subject-matter expertise
- Learn new concepts from industry experts
- Gain a foundational understanding of a subject or tool
- Develop job-relevant skills with hands-on projects
- Earn a shareable career certificate
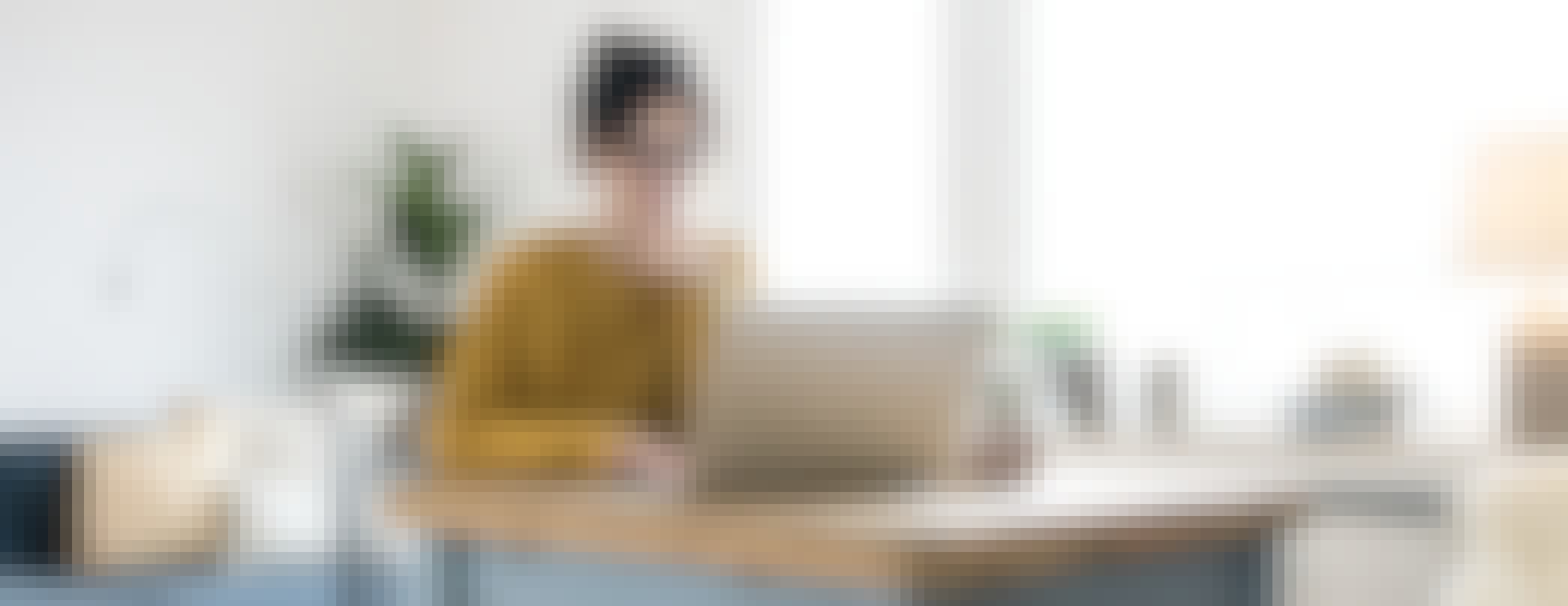
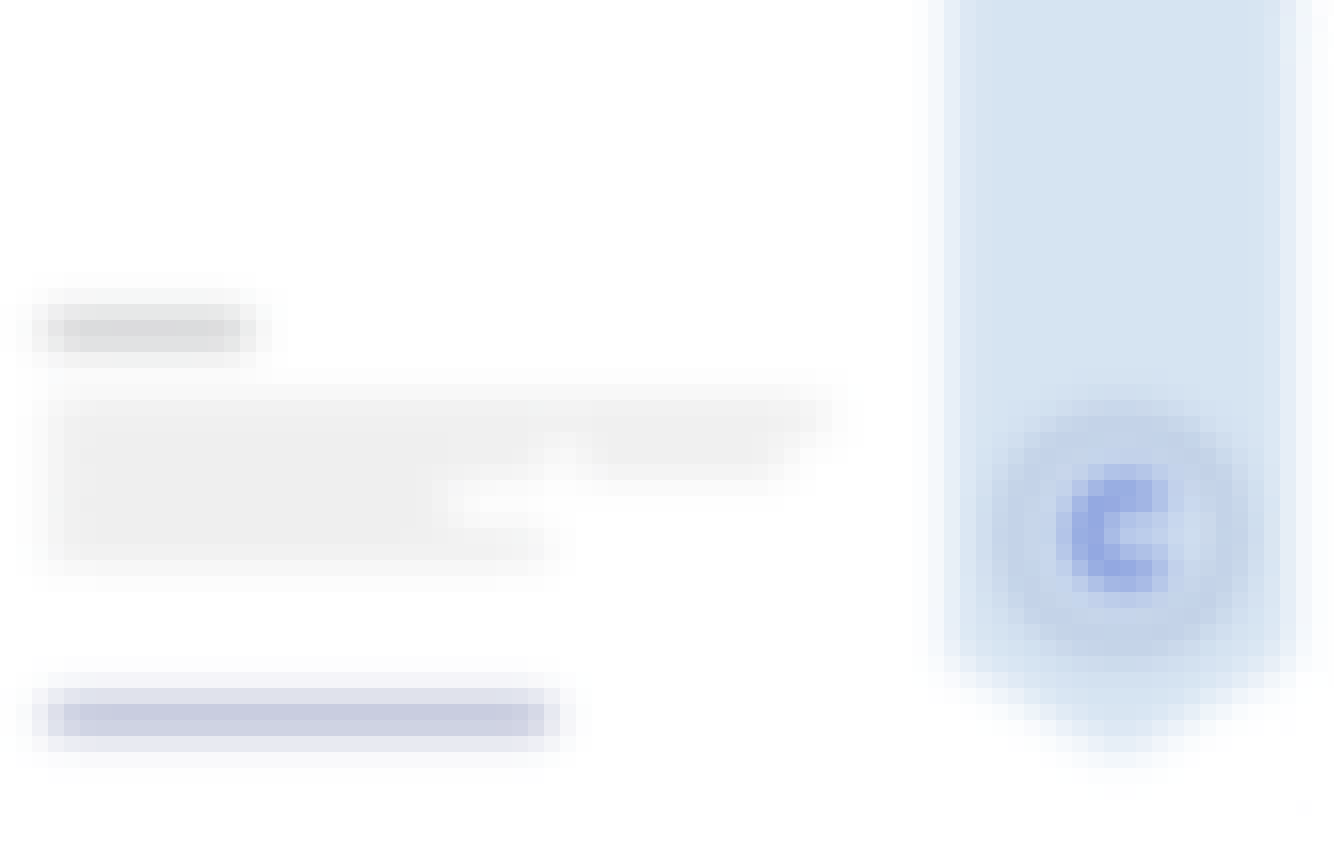
Earn a career certificate
Add this credential to your LinkedIn profile, resume, or CV
Share it on social media and in your performance review
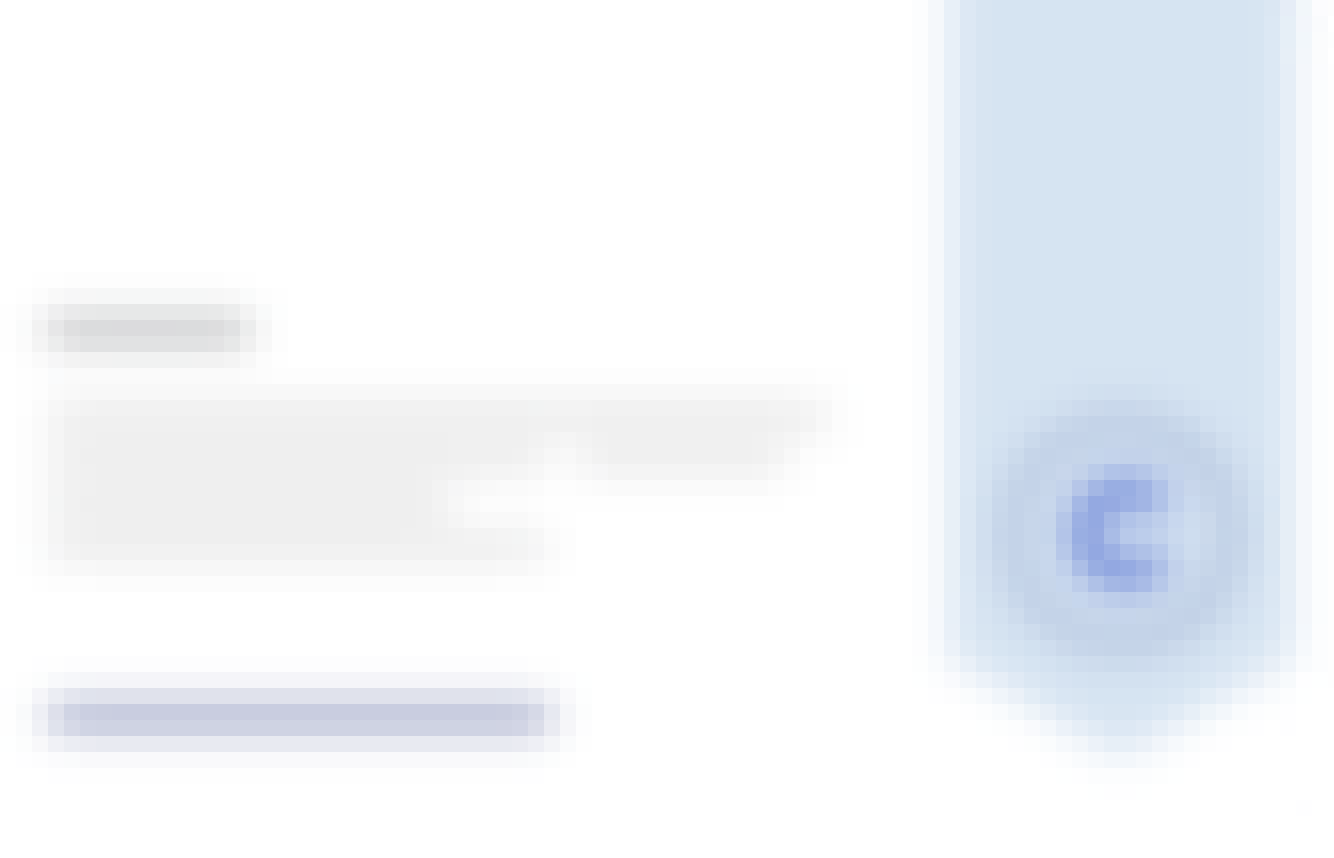
There are 4 modules in this course
Principal Component Analysis (PCA) is one of the most important dimensionality reduction algorithms in machine learning. In this course, we lay the mathematical foundations to derive and understand PCA from a geometric point of view. In this module, we learn how to summarize datasets (e.g., images) using basic statistics, such as the mean and the variance. We also look at properties of the mean and the variance when we shift or scale the original data set. We will provide mathematical intuition as well as the skills to derive the results. We will also implement our results in code (jupyter notebooks), which will allow us to practice our mathematical understand to compute averages of image data sets. Therefore, some python/numpy background will be necessary to get through this course. Note: If you have taken the other two courses of this specialization, this one will be harder (mostly because of the programming assignments). However, if you make it through the first week of this course, you will make it through the full course with high probability.
What's included
8 videos6 readings3 assignments1 programming assignment1 discussion prompt2 ungraded labs1 plugin
Data can be interpreted as vectors. Vectors allow us to talk about geometric concepts, such as lengths, distances and angles to characterize similarity between vectors. This will become important later in the course when we discuss PCA. In this module, we will introduce and practice the concept of an inner product. Inner products allow us to talk about geometric concepts in vector spaces. More specifically, we will start with the dot product (which we may still know from school) as a special case of an inner product, and then move toward a more general concept of an inner product, which play an integral part in some areas of machine learning, such as kernel machines (this includes support vector machines and Gaussian processes). We have a lot of exercises in this module to practice and understand the concept of inner products.
What's included
8 videos1 reading4 assignments1 programming assignment2 ungraded labs
In this module, we will look at orthogonal projections of vectors, which live in a high-dimensional vector space, onto lower-dimensional subspaces. This will play an important role in the next module when we derive PCA. We will start off with a geometric motivation of what an orthogonal projection is and work our way through the corresponding derivation. We will end up with a single equation that allows us to project any vector onto a lower-dimensional subspace. However, we will also understand how this equation came about. As in the other modules, we will have both pen-and-paper practice and a small programming example with a jupyter notebook.
What's included
6 videos1 reading2 assignments1 programming assignment1 ungraded lab
We can think of dimensionality reduction as a way of compressing data with some loss, similar to jpg or mp3. Principal Component Analysis (PCA) is one of the most fundamental dimensionality reduction techniques that are used in machine learning. In this module, we use the results from the first three modules of this course and derive PCA from a geometric point of view. Within this course, this module is the most challenging one, and we will go through an explicit derivation of PCA plus some coding exercises that will make us a proficient user of PCA.
What's included
10 videos5 readings2 assignments1 programming assignment2 ungraded labs1 plugin
Instructor

Offered by
Why people choose Coursera for their career




Learner reviews
3,106 reviews
- 5 stars
51.31%
- 4 stars
22.26%
- 3 stars
12.69%
- 2 stars
6.55%
- 1 star
7.16%
Showing 3 of 3106
Reviewed on Nov 5, 2018
Programming assignment for week 1 wastes to much time due to lack of instructions.
Reviewed on Dec 27, 2019
This course is well worth the time. I have a better understanding of one of the most foundational and biologically plausible machine learning algorithms used today! Love it.
Reviewed on Jul 19, 2022
Really clear and well explained. The concepts are treated in detail enough to be applied. Very happy to have invested my time in this course. I strongly recomend it.
Recommended if you're interested in Data Science
Imperial College London
Imperial College London
DeepLearning.AI
Coursera Project Network

Open new doors with Coursera Plus
Unlimited access to 10,000+ world-class courses, hands-on projects, and job-ready certificate programs - all included in your subscription
Advance your career with an online degree
Earn a degree from world-class universities - 100% online
Join over 3,400 global companies that choose Coursera for Business
Upskill your employees to excel in the digital economy