In the course "Training AI with Humans", you'll delve into the intersection of machine learning and human collaboration, exploring how to enhance AI performance through effective data annotation and crowdsourcing. You’ll gain a comprehensive understanding of machine learning principles and performance metrics while developing practical skills in using platforms like Amazon Mechanical Turk (AMT) for crowdsourced tasks. This unique approach combines theoretical knowledge with hands-on experience, allowing you to implement Inter-Annotator Agreement (IAA) techniques to ensure high-quality annotated data.

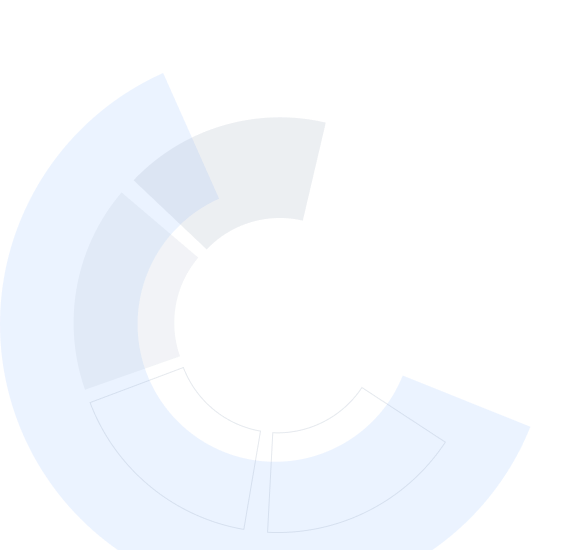
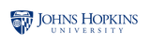
Training AI with Humans
This course is part of Social Computing Specialization

Instructor: Ian McCulloh
Sponsored by Barbados NTI
Recommended experience
What you'll learn
Learn to construct and evaluate various machine learning classifiers and performance metrics.
Master the calculation and implications of Inter-Annotator Agreement (IAA) for data consistency.
Understand how to design and implement effective crowdsourcing tasks using Amazon Mechanical Turk.
Analyze crowdsourced data to enhance machine learning models and understand ethical considerations in AI.
Details to know

Add to your LinkedIn profile
15 assignments
September 2024
See how employees at top companies are mastering in-demand skills
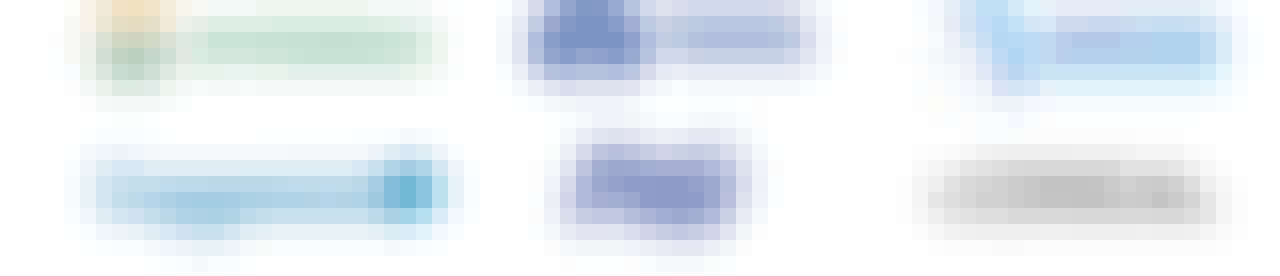
Build your subject-matter expertise
- Learn new concepts from industry experts
- Gain a foundational understanding of a subject or tool
- Develop job-relevant skills with hands-on projects
- Earn a shareable career certificate
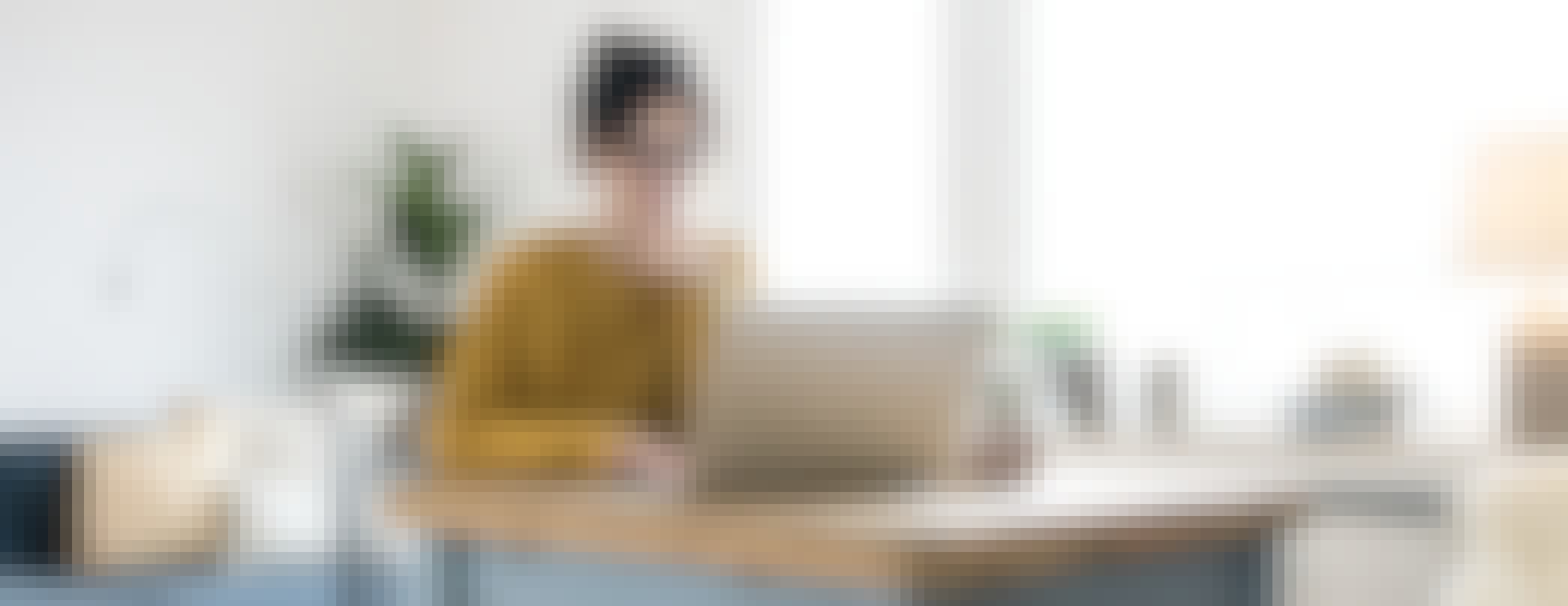
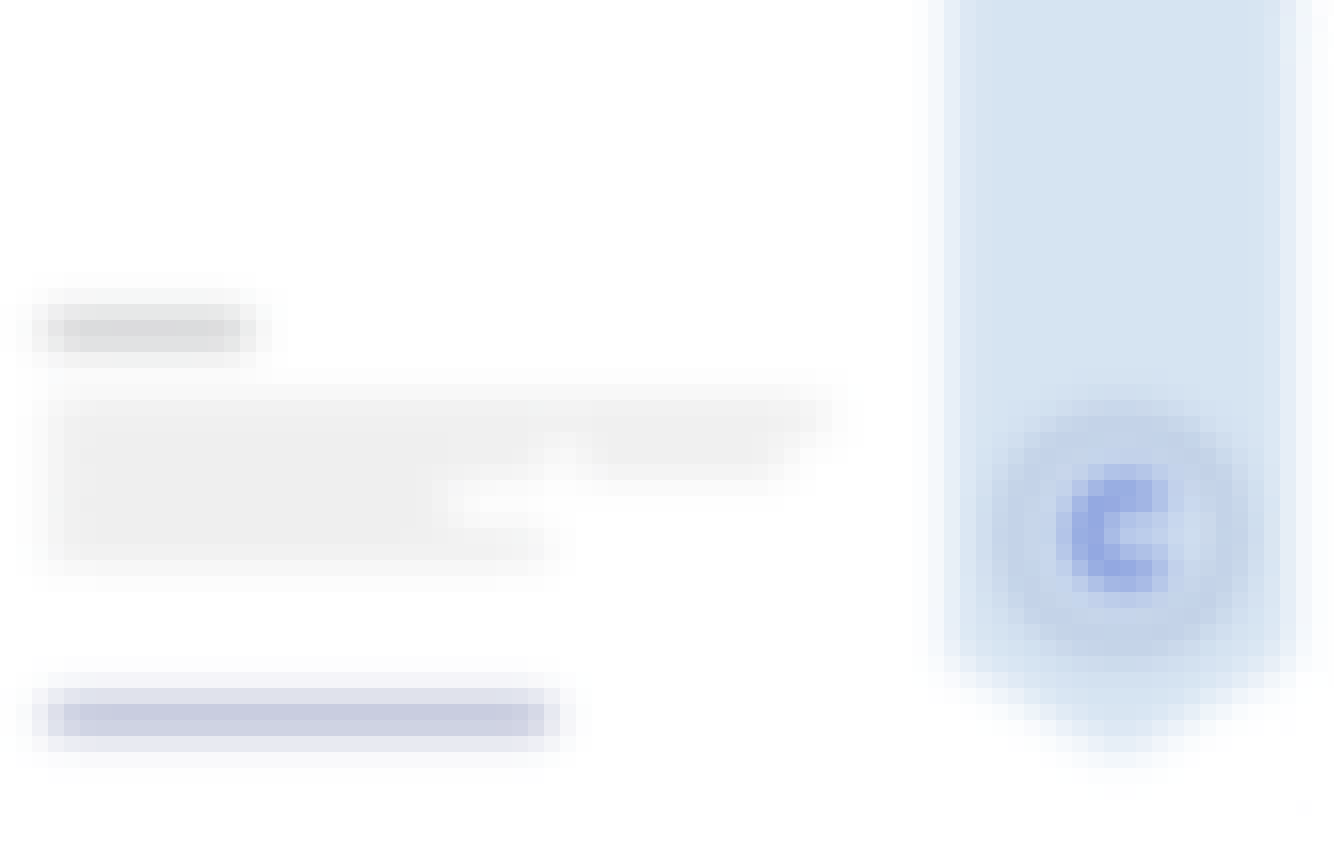
Earn a career certificate
Add this credential to your LinkedIn profile, resume, or CV
Share it on social media and in your performance review
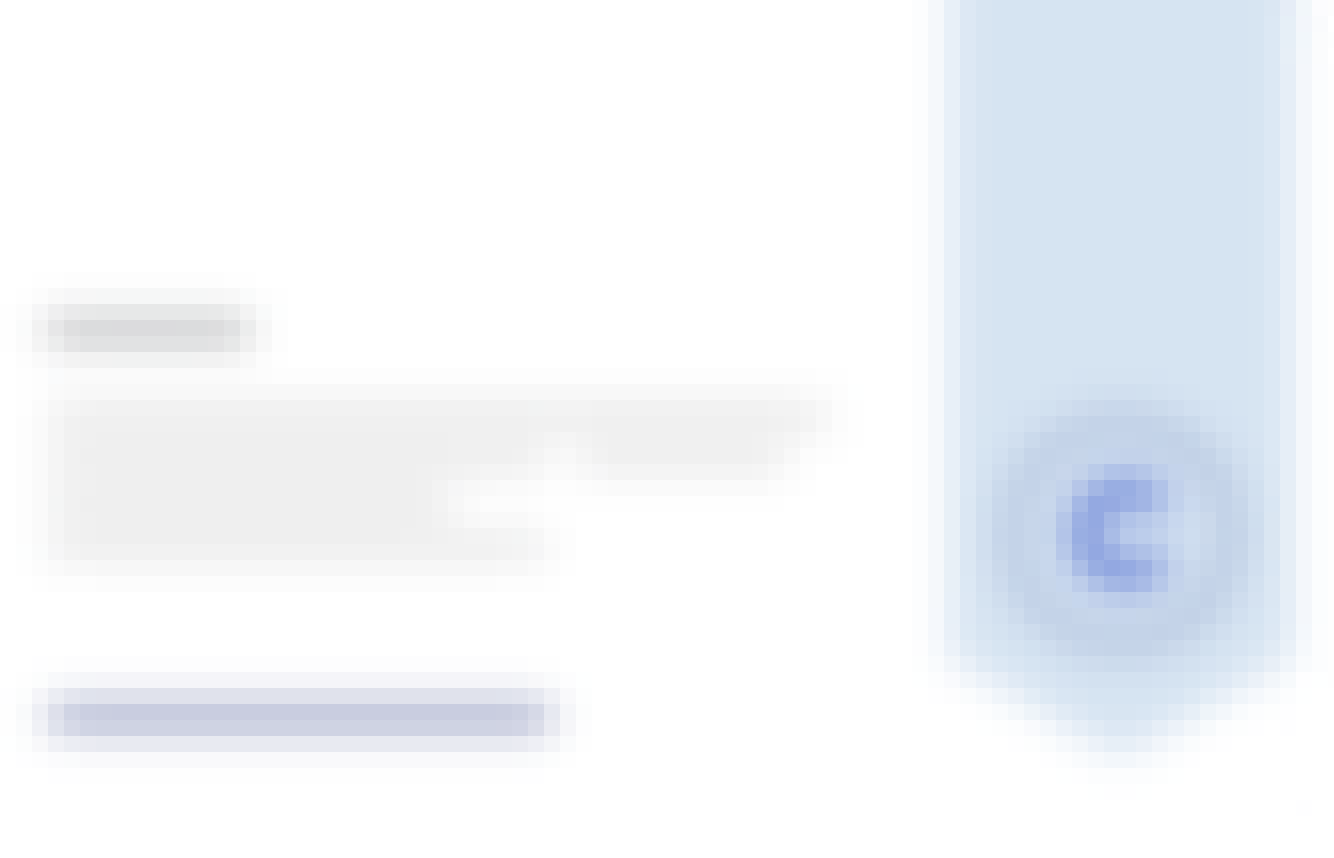
There are 6 modules in this course
This course explores the intersection of machine learning (ML) and human input through various methodologies and tools. Spanning five modules, you will gain a comprehensive understanding of machine learning techniques, the role of human annotation in ML performance, and the principles and practices of crowdsourcing. The course covers key aspects of designing and implementing crowdsourced studies, calculating inter-annotator agreements, and leveraging crowdsourcing to enhance ML performance. Practical skills will be developed through hands-on activities using platforms like Amazon Mechanical Turk (AMT) and analyzing the data collected from such platforms.
What's included
1 reading1 plugin
In this module, you will be introduced to the fundamentals of machine learning (ML). You will learn the definition and principles of ML, and gain practical skills in calculating and comparing ML performance metrics. You will get a chance to understand how to construct ML classifiers and analyze their effectiveness across different algorithms. This module prepares you to apply ML techniques effectively in various domains, enhancing your ability to solve complex problems using data-driven approaches.
What's included
5 videos2 readings3 assignments1 ungraded lab
In this module, you will explore the significance of IAA in Machine Learning (ML) performance. You will learn to calculate IAA manually and implement Krippendorf’s Alpha using the software. You will gain insights into how IAA impacts the reliability of annotated data and its implications for ML model training. This module equips you with essential skills to ensure consistency and reliability in data annotation processes, crucial for effective ML applications.
What's included
3 videos2 readings3 assignments
In this module, you will be introduced to the concept and practical applications of crowdsourcing. You will get a chance to learn how crowdsourcing enhances problem-solving through collective efforts and explore real-world use cases. You will be able to establish your first Amazon Mechanical Turk (AMT) account and understand the platform's capabilities for executing crowdsourced tasks. You will get a chance to delve into crowdsourcing design principles to optimize task efficiency and reliability. This module prepares you to leverage crowdsourcing effectively for diverse applications, from data annotation to research experiments.
What's included
4 videos1 reading3 assignments1 ungraded lab
This module focuses on leveraging Amazon Mechanical Turk (AMT) for crowdsourcing studies. You will learn to design effective experiments using AMT, ensuring optimal task design and participant engagement. You will be able to collect data through AMT and perform initial analyses to derive meaningful insights from crowdsourced data. You will also understand the implications of AMT addiction and ethical considerations in platform-based research. This module equips you with practical skills to conduct reliable and insightful crowdsourcing studies using AMT.
What's included
2 videos3 readings3 assignments1 ungraded lab
This module explores the intersection of crowdsourcing and ML performance enhancement. You will be able to evaluate how Inter-Annotator Agreement (IAA) affects ML model reliability and accuracy. You will explore case studies such as COVID test kit distribution and organ transplant matching to understand real-world applications. You will learn to optimize ML performance through effective crowdsourcing design, ensuring data quality and reliability in machine learning applications.
What's included
4 videos3 readings3 assignments
Instructor

Offered by
Why people choose Coursera for their career




Recommended if you're interested in Computer Science
Coursera Instructor Network
University of Michigan
Johns Hopkins University
University of Michigan

Open new doors with Coursera Plus
Unlimited access to 10,000+ world-class courses, hands-on projects, and job-ready certificate programs - all included in your subscription
Advance your career with an online degree
Earn a degree from world-class universities - 100% online
Join over 3,400 global companies that choose Coursera for Business
Upskill your employees to excel in the digital economy