This course for practicing and aspiring data scientists and statisticians. It is the fourth of a four-course sequence introducing the fundamentals of Bayesian statistics. It builds on the course Bayesian Statistics: From Concept to Data Analysis, Techniques and Models, and Mixture models.

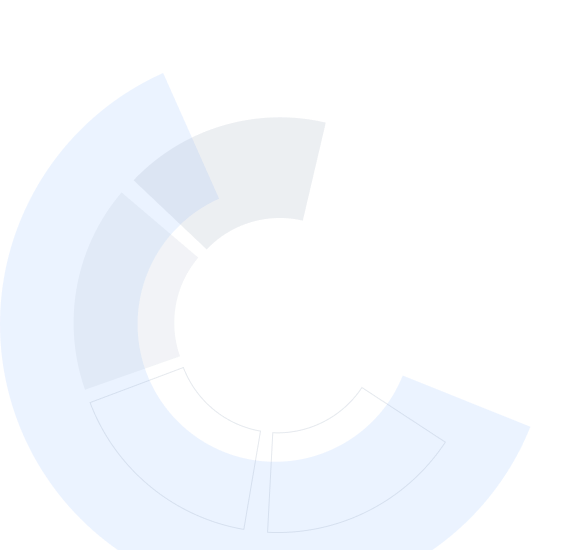
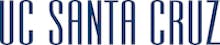
Bayesian Statistics: Time Series Analysis
This course is part of Bayesian Statistics Specialization

Instructor: Raquel Prado
Sponsored by Coursera Learning Team
4,809 already enrolled
(15 reviews)
Recommended experience
What you'll learn
Build models that describe temporal dependencies.
Use R for analysis and forecasting of times series.
Explain stationary time series processes.
Skills you'll gain
Details to know

Add to your LinkedIn profile
10 assignments
See how employees at top companies are mastering in-demand skills
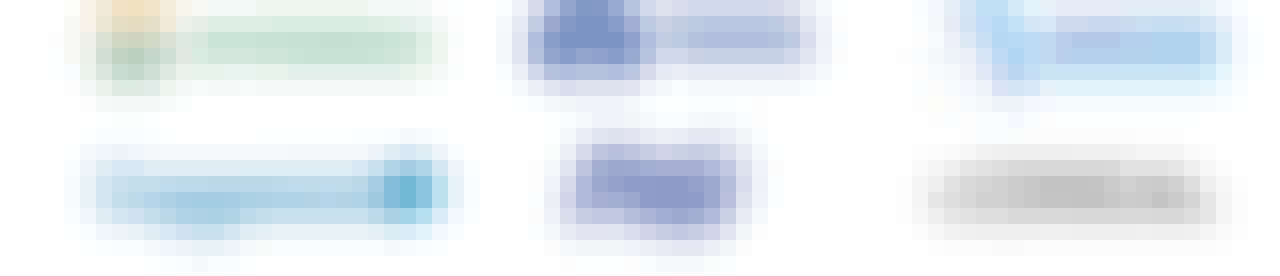
Build your subject-matter expertise
- Learn new concepts from industry experts
- Gain a foundational understanding of a subject or tool
- Develop job-relevant skills with hands-on projects
- Earn a shareable career certificate
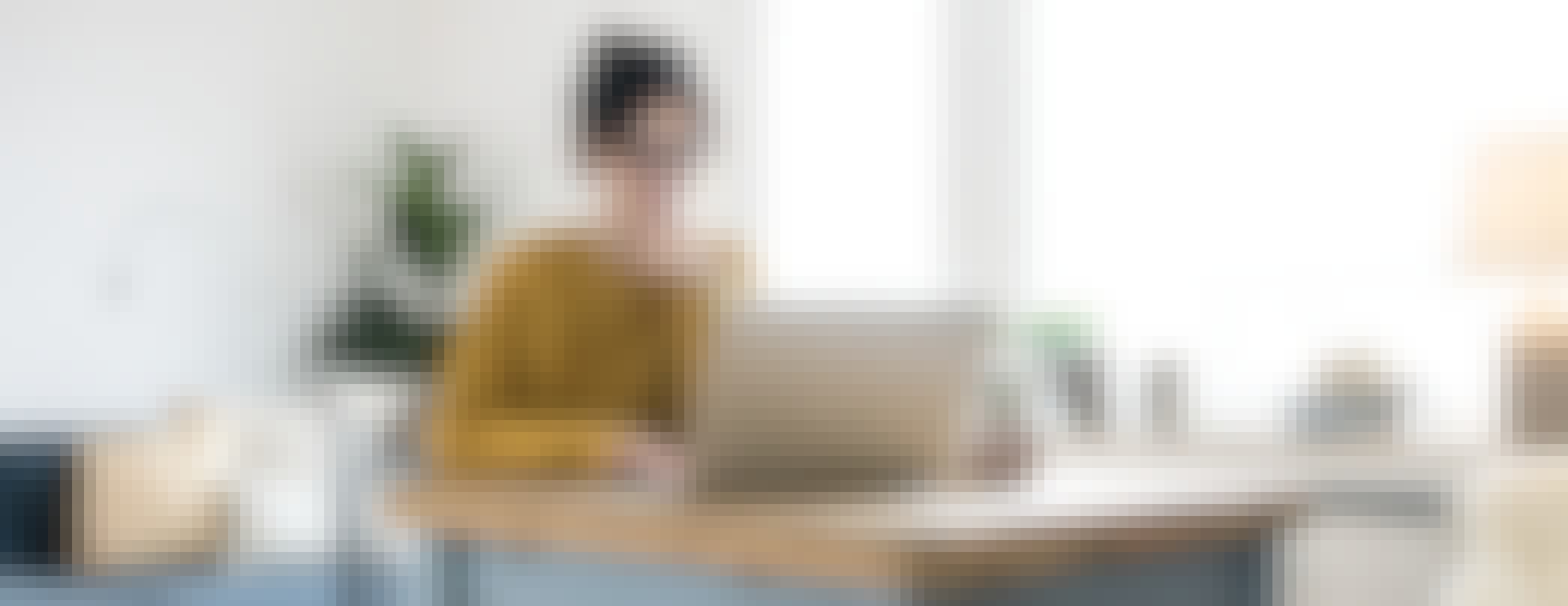
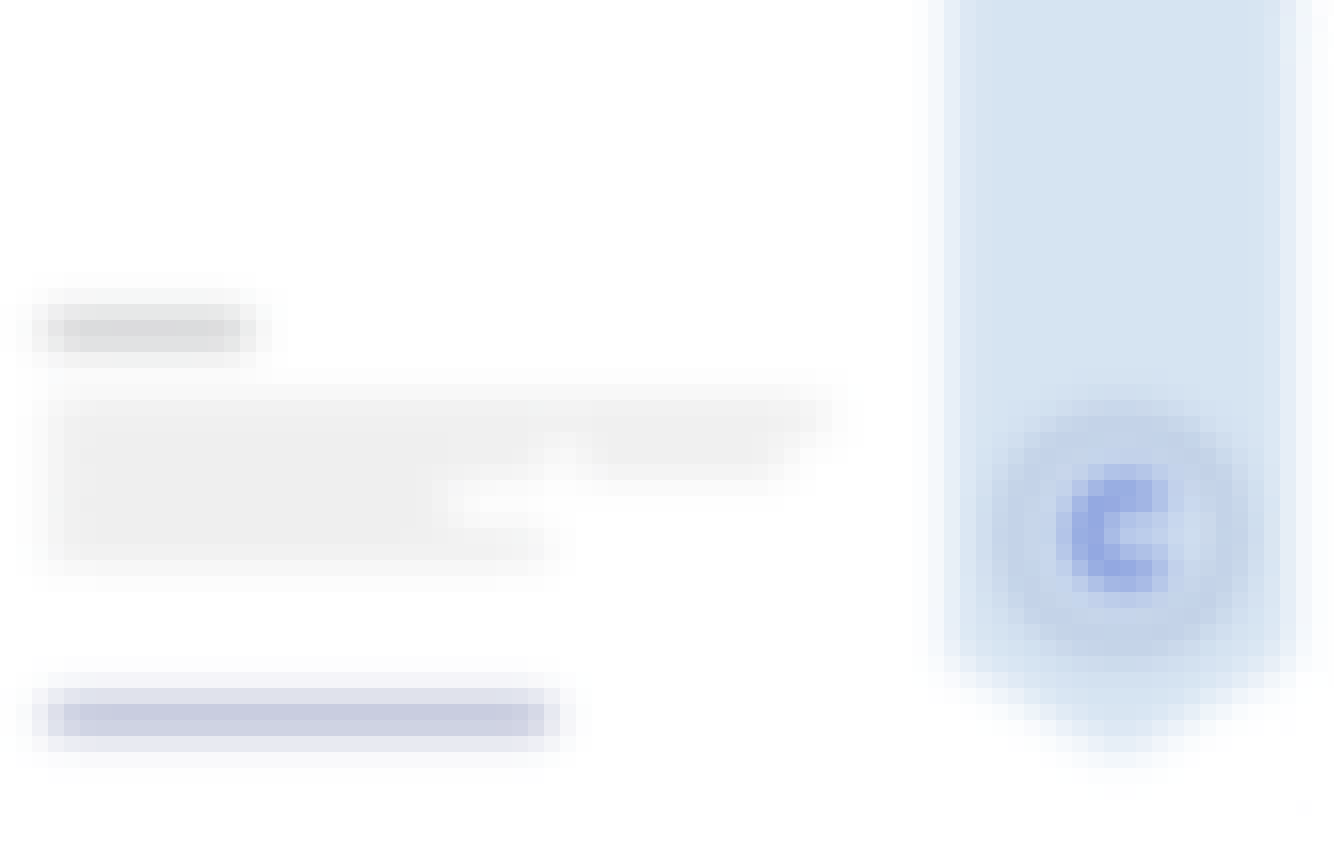
Earn a career certificate
Add this credential to your LinkedIn profile, resume, or CV
Share it on social media and in your performance review
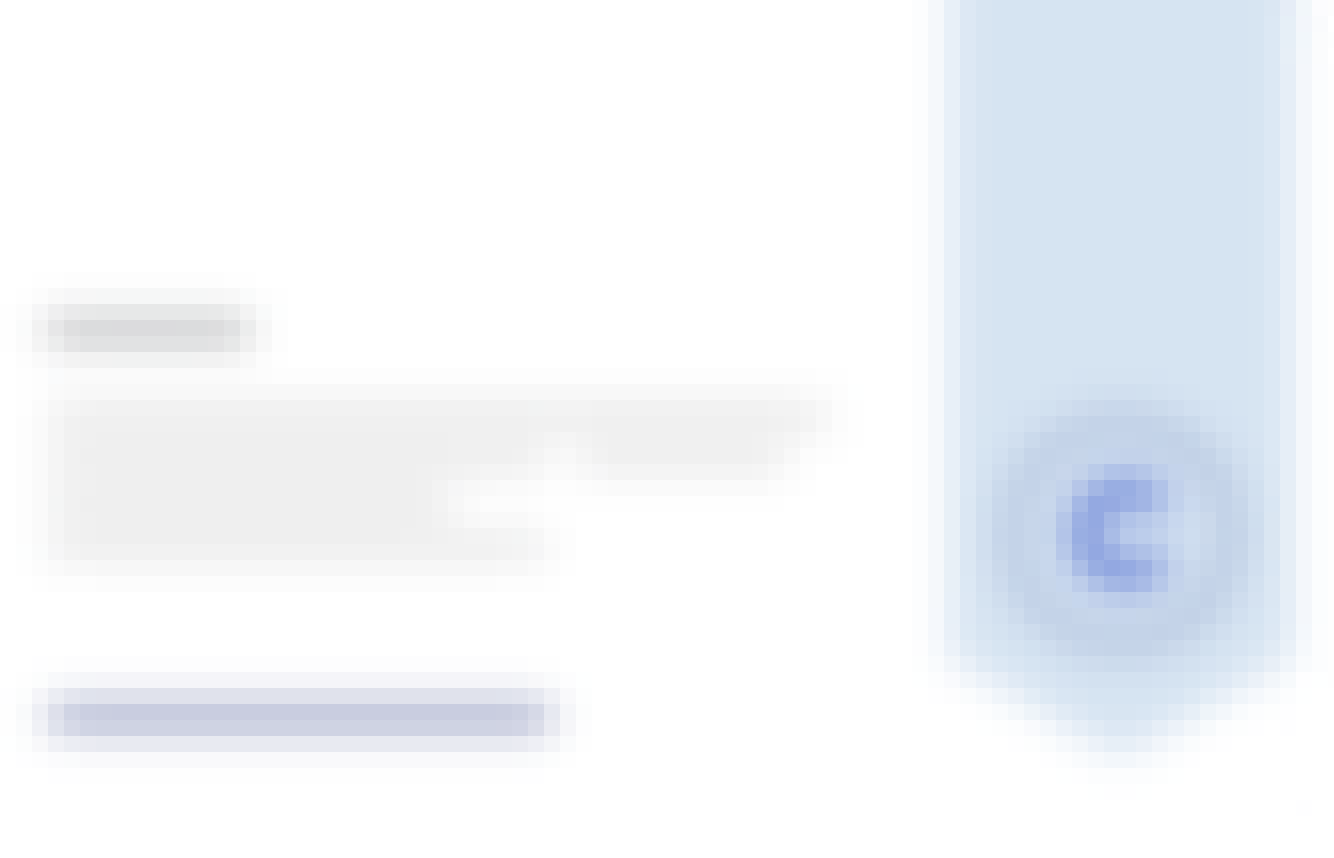
There are 5 modules in this course
This module defines stationary time series processes, the autocorrelation function and the autoregressive process of order one or AR(1). Parameter estimation via maximum likelihood and Bayesian inference in the AR(1) are also discussed.
What's included
9 videos12 readings4 assignments1 peer review
This module extends the concepts learned in Week 1 about the AR(1) process to the general case of the AR(p). Maximum likelihood estimation and Bayesian posterior inference in the AR(p) are discussed.
What's included
9 videos8 readings2 assignments1 peer review
Normal Dynamic Linear Models (NDLMs) are defined and illustrated in this module using several examples. Model building based on the forecast function via the superposition principle is explained. Methods for Bayesian filtering, smoothing and forecasting for NDLMs in the case of known observational variances and known system covariance matrices are discussed and illustrated.
What's included
10 videos7 readings2 assignments1 peer review
What's included
7 videos4 readings2 assignments1 peer review
In this final project you will use normal dynamic linear models to analyze a time series dataset downloaded from Google trend.
What's included
1 peer review
Instructor

Offered by
Why people choose Coursera for their career




Learner reviews
15 reviews
- 5 stars
73.33%
- 4 stars
0%
- 3 stars
20%
- 2 stars
0%
- 1 star
6.66%
Showing 3 of 15
Reviewed on Feb 5, 2024
It was a nice course, but it would be better if there were more supplementary materials for the proof and theoretical discussion.
Recommended if you're interested in Data Science
Illinois Tech
IIMA - IIM Ahmedabad
University of Colorado Boulder
Johns Hopkins University

Open new doors with Coursera Plus
Unlimited access to 10,000+ world-class courses, hands-on projects, and job-ready certificate programs - all included in your subscription
Advance your career with an online degree
Earn a degree from world-class universities - 100% online
Join over 3,400 global companies that choose Coursera for Business
Upskill your employees to excel in the digital economy