This course introduces you to one of the main types of Machine Learning: Unsupervised Learning. You will learn how to find insights from data sets that do not have a target or labeled variable. You will learn several clustering and dimension reduction algorithms for unsupervised learning as well as how to select the algorithm that best suits your data. The hands-on section of this course focuses on using best practices for unsupervised learning.

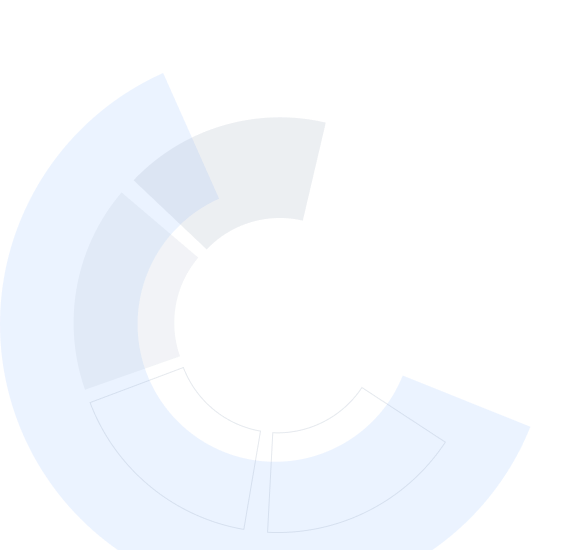
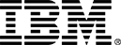
Unsupervised Machine Learning
This course is part of multiple programs.



Instructors: Mark J Grover
Sponsored by Coursera Learning Team
30,104 already enrolled
(275 reviews)
Skills you'll gain
Details to know

Add to your LinkedIn profile
14 assignments
See how employees at top companies are mastering in-demand skills
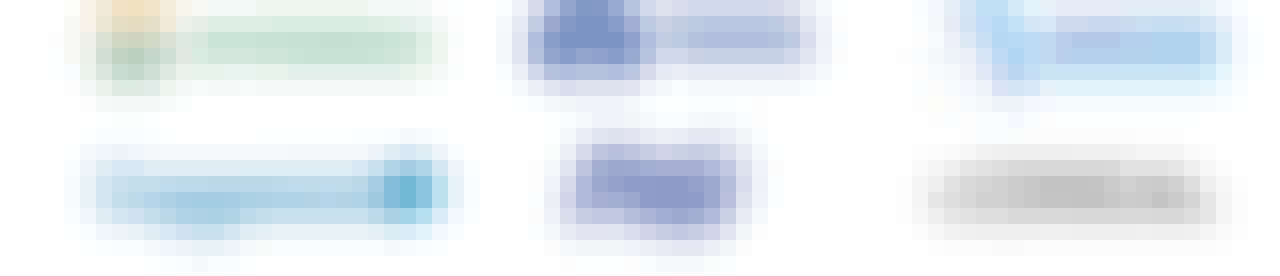
Build your subject-matter expertise
- Learn new concepts from industry experts
- Gain a foundational understanding of a subject or tool
- Develop job-relevant skills with hands-on projects
- Earn a shareable career certificate
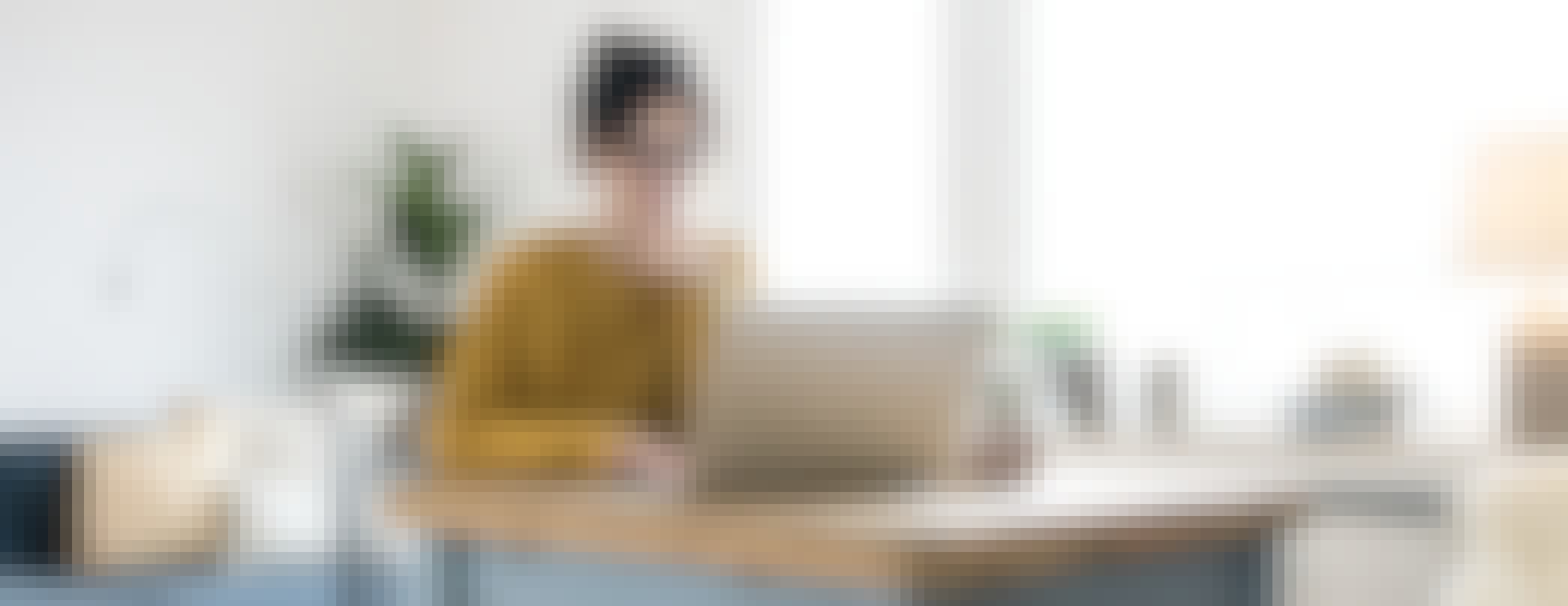
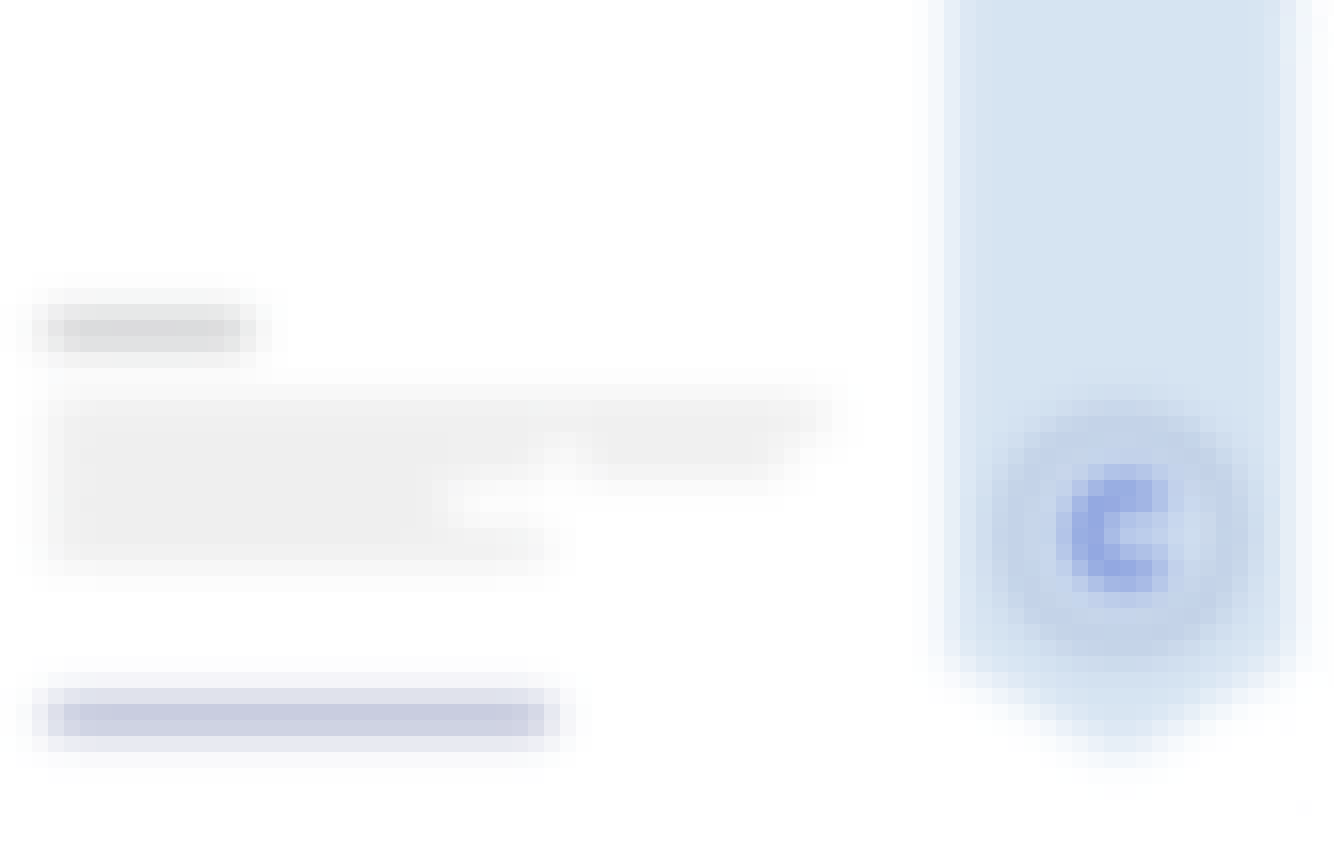
Earn a career certificate
Add this credential to your LinkedIn profile, resume, or CV
Share it on social media and in your performance review
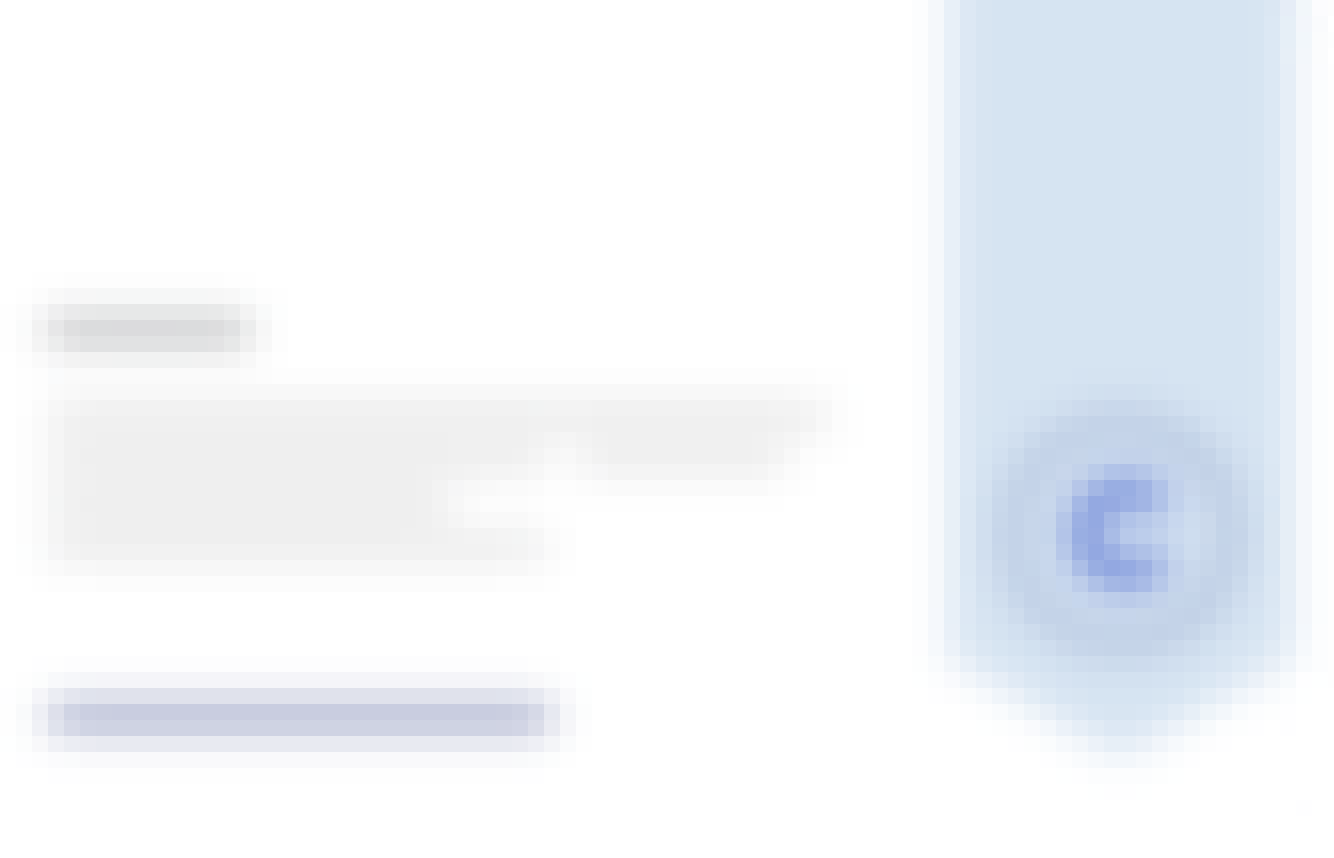
There are 7 modules in this course
This module introduces Unsupervised Learning and its applications. One of the most common uses of Unsupervised Learning is clustering observations using k-means. In this module, you become familiar with the theory behind this algorithm, and put it in practice in a demonstration.
What's included
11 videos2 readings3 assignments3 app items
What's included
6 videos1 reading2 assignments2 app items
In this module, you become familiar with some of the computational hurdles around clustering algorithms, and how different clustering implementations try to overcome them. After a brief recapitulation of common clustering algorithms, you will learn how to compare them and select the clustering technique that best suits your data.
What's included
11 videos1 reading3 assignments3 app items
This module introduces dimensionality reduction and Principal Component Analysis, which are powerful techniques for big data, imaging, and pre-processing data.
What's included
5 videos1 reading2 assignments4 app items
This module introduces dimensionality reduction techniques like Kernal Principal Component Analysis and multidimensional scaling. These methods are more powerful than Principal Component Analysis in many applications.
What's included
2 videos1 reading2 assignments3 app items
This module introduces matrix factorization, which is a powerful technique for big data, text mining, and pre-processing data.
What's included
3 videos1 reading2 assignments3 app items
Now, you have all the tools in your toolkit to highlight your Unsupervised Learning abilities in your final project.
What's included
1 peer review
Instructors



Offered by
Why people choose Coursera for their career




Learner reviews
275 reviews
- 5 stars
77.45%
- 4 stars
16.36%
- 3 stars
2.90%
- 2 stars
1.45%
- 1 star
1.81%
Showing 3 of 275
Reviewed on Dec 25, 2020
Great course for learning about Unsupervised Learning
Reviewed on Nov 6, 2020
Great course and very well structured. I'm really impressed with the instructor who give thorough walkthrough to the code.
Reviewed on Dec 9, 2024
Excellent use of labs to study material. Lectures were very informative and quizzes well designed.
Recommended if you're interested in Data Science
University of Colorado Boulder
University of Michigan

Open new doors with Coursera Plus
Unlimited access to 10,000+ world-class courses, hands-on projects, and job-ready certificate programs - all included in your subscription
Advance your career with an online degree
Earn a degree from world-class universities - 100% online
Join over 3,400 global companies that choose Coursera for Business
Upskill your employees to excel in the digital economy