In this course on Linear Algebra we look at what linear algebra is and how it relates to vectors and matrices. Then we look through what vectors and matrices are and how to work with them, including the knotty problem of eigenvalues and eigenvectors, and how to use these to solve problems. Finally we look at how to use these to do fun things with datasets - like how to rotate images of faces and how to extract eigenvectors to look at how the Pagerank algorithm works.

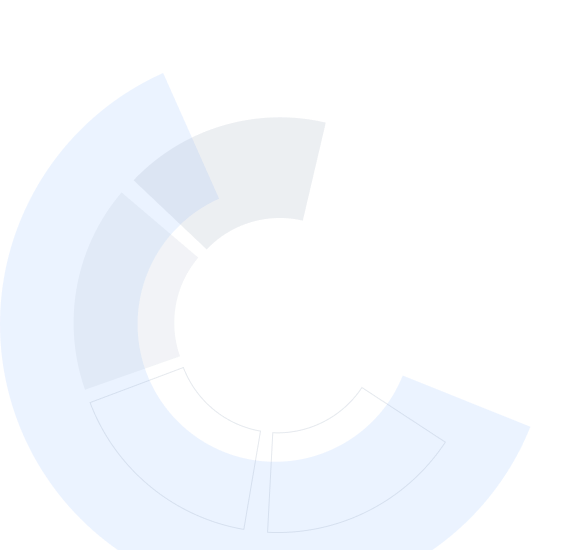
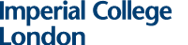
Mathematics for Machine Learning: Linear Algebra
This course is part of Mathematics for Machine Learning Specialization



Instructors: David Dye
Sponsored by Coursera Learning Team
402,938 already enrolled
(12,232 reviews)
Skills you'll gain
Details to know

Add to your LinkedIn profile
15 assignments
See how employees at top companies are mastering in-demand skills
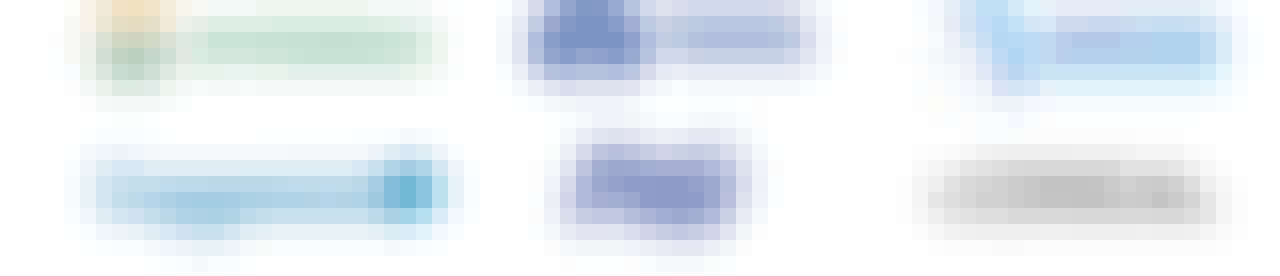
Build your subject-matter expertise
- Learn new concepts from industry experts
- Gain a foundational understanding of a subject or tool
- Develop job-relevant skills with hands-on projects
- Earn a shareable career certificate
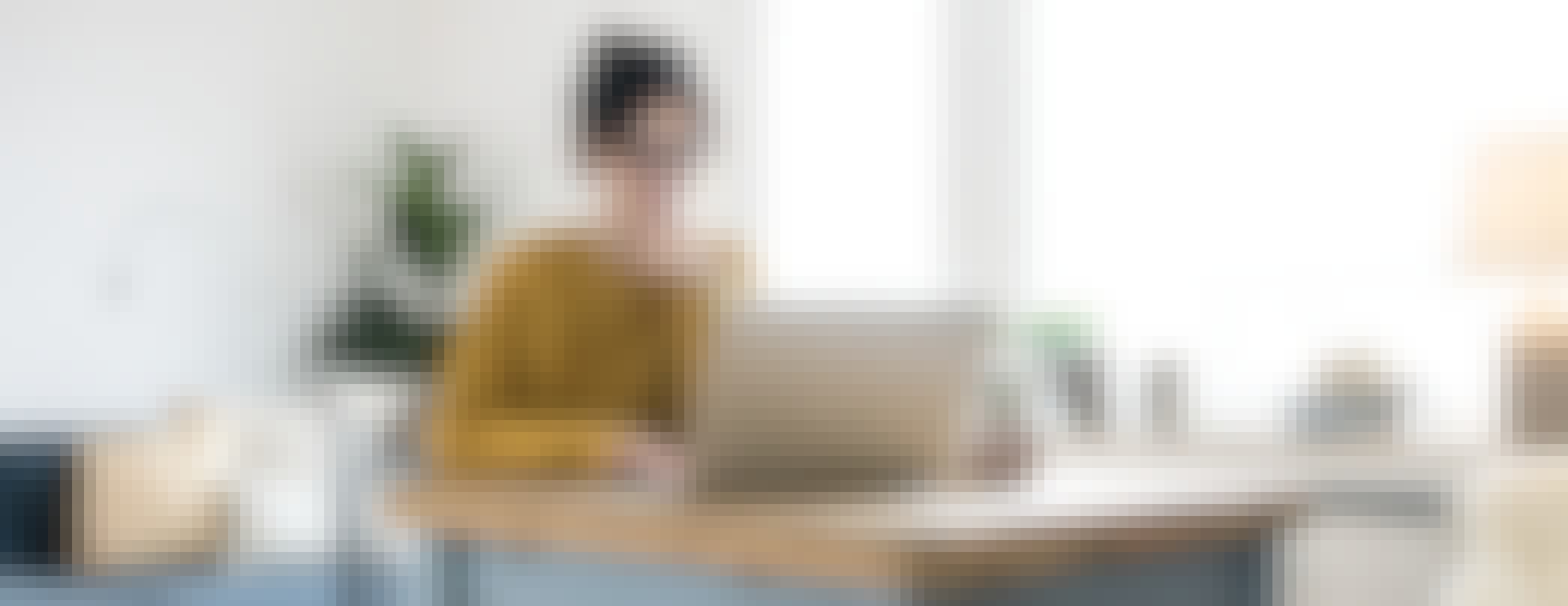
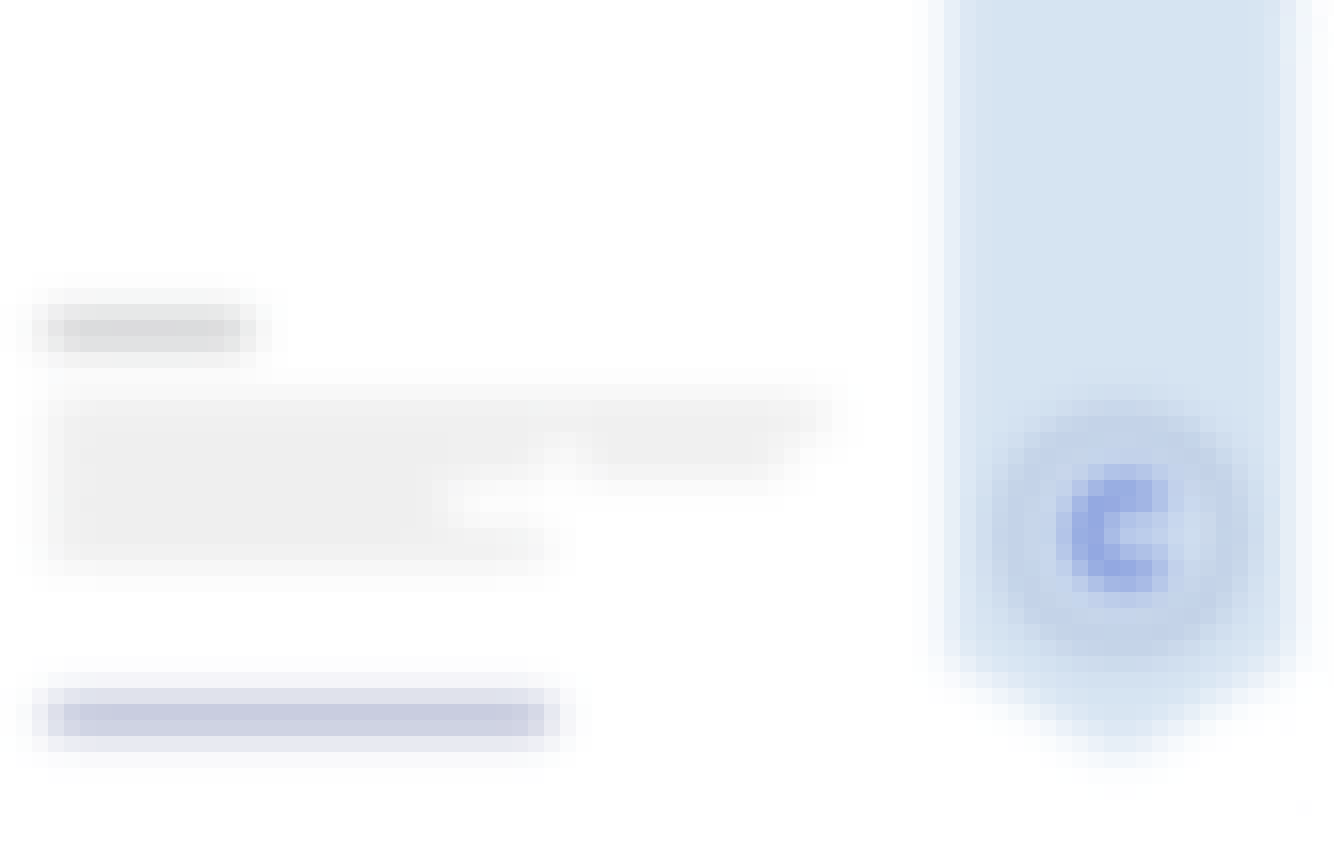
Earn a career certificate
Add this credential to your LinkedIn profile, resume, or CV
Share it on social media and in your performance review
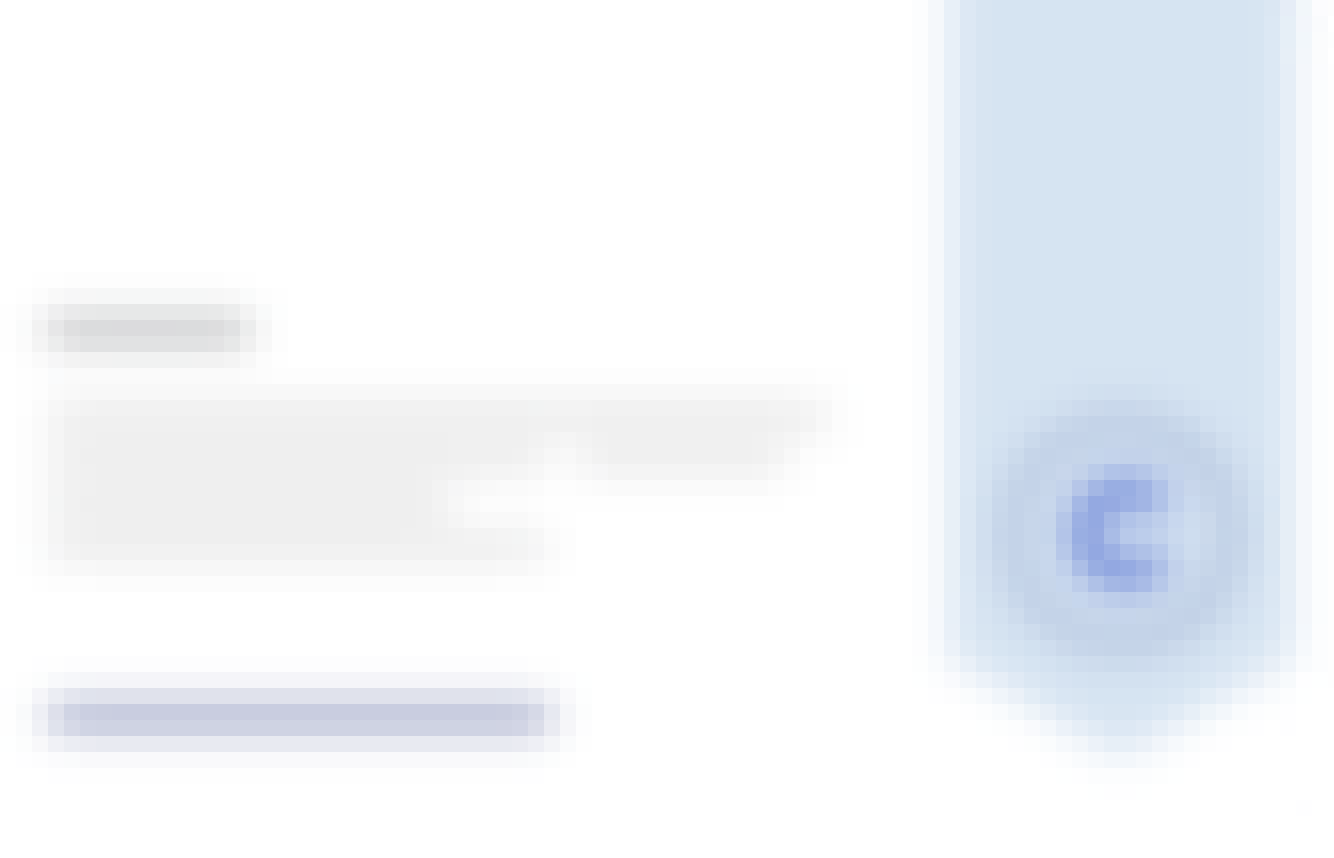
There are 5 modules in this course
In this first module we look at how linear algebra is relevant to machine learning and data science. Then we'll wind up the module with an initial introduction to vectors. Throughout, we're focussing on developing your mathematical intuition, not of crunching through algebra or doing long pen-and-paper examples. For many of these operations, there are callable functions in Python that can do the adding up - the point is to appreciate what they do and how they work so that, when things go wrong or there are special cases, you can understand why and what to do.
What's included
5 videos4 readings3 assignments1 discussion prompt1 plugin
In this module, we look at operations we can do with vectors - finding the modulus (size), angle between vectors (dot or inner product) and projections of one vector onto another. We can then examine how the entries describing a vector will depend on what vectors we use to define the axes - the basis. That will then let us determine whether a proposed set of basis vectors are what's called 'linearly independent.' This will complete our examination of vectors, allowing us to move on to matrices in module 3 and then start to solve linear algebra problems.
What's included
8 videos4 assignments
Now that we've looked at vectors, we can turn to matrices. First we look at how to use matrices as tools to solve linear algebra problems, and as objects that transform vectors. Then we look at how to solve systems of linear equations using matrices, which will then take us on to look at inverse matrices and determinants, and to think about what the determinant really is, intuitively speaking. Finally, we'll look at cases of special matrices that mean that the determinant is zero or where the matrix isn't invertible - cases where algorithms that need to invert a matrix will fail.
What's included
8 videos2 assignments1 programming assignment1 ungraded lab
In Module 4, we continue our discussion of matrices; first we think about how to code up matrix multiplication and matrix operations using the Einstein Summation Convention, which is a widely used notation in more advanced linear algebra courses. Then, we look at how matrices can transform a description of a vector from one basis (set of axes) to another. This will allow us to, for example, figure out how to apply a reflection to an image and manipulate images. We'll also look at how to construct a convenient basis vector set in order to do such transformations. Then, we'll write some code to do these transformations and apply this work computationally.
What's included
6 videos2 assignments2 programming assignments2 ungraded labs
Eigenvectors are particular vectors that are unrotated by a transformation matrix, and eigenvalues are the amount by which the eigenvectors are stretched. These special 'eigen-things' are very useful in linear algebra and will let us examine Google's famous PageRank algorithm for presenting web search results. Then we'll apply this in code, which will wrap up the course.
What's included
9 videos1 reading4 assignments1 programming assignment1 ungraded lab2 plugins
Instructors


Offered by
Why people choose Coursera for their career




Learner reviews
12,232 reviews
- 5 stars
74.55%
- 4 stars
19.64%
- 3 stars
3.47%
- 2 stars
1.17%
- 1 star
1.15%
Showing 3 of 12232
Reviewed on Dec 22, 2018
Professors teaches in so much friendly manner. This is beginner level course. Don't expect you will dive deep inside the Linear Algebra. But the foundation will become solid if you attend this course.
Reviewed on Jul 11, 2019
It's a nice course but instructors should go in more details. It's mostly high school mathematics. I was expecting undergraduate level Linear Algebra. Otherwise it was a good learning experience.
Reviewed on May 29, 2021
This is a great course to built foundation for Machine Learning. Both the lecturers are amazing and great use of technology in presenting the concepts. Great example linked to PageRank algorithm.
Recommended if you're interested in Data Science
University of Colorado Boulder
University of Minnesota
Duke University

Open new doors with Coursera Plus
Unlimited access to 10,000+ world-class courses, hands-on projects, and job-ready certificate programs - all included in your subscription
Advance your career with an online degree
Earn a degree from world-class universities - 100% online
Join over 3,400 global companies that choose Coursera for Business
Upskill your employees to excel in the digital economy