In this course, we dive into the components and best practices of building high-performing ML systems in production environments. We cover some of the most common considerations behind building these systems, e.g. static training, dynamic training, static inference, dynamic inference, distributed TensorFlow, and TPUs. This course is devoted to exploring the characteristics that make for a good ML system beyond its ability to make good predictions.

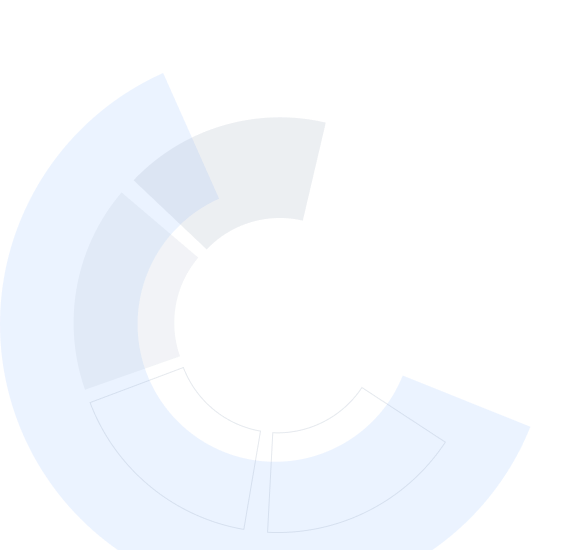
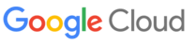
Production Machine Learning Systems
This course is part of multiple programs.

Instructor: Google Cloud Training
Sponsored by Syrian Youth Assembly
34,955 already enrolled
(996 reviews)
What you'll learn
Compare static versus dynamic training and inference
Manage model dependencies
Set up distributed training for fault tolerance, replication, and more
Export models for portability
Details to know

Add to your LinkedIn profile
4 assignments
See how employees at top companies are mastering in-demand skills
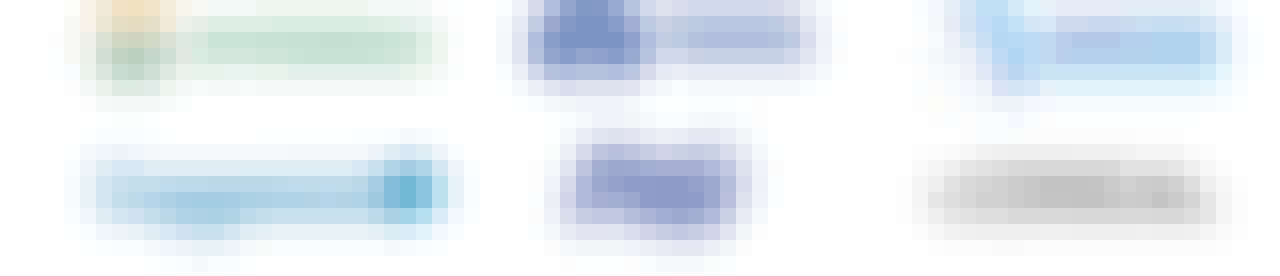
Build your subject-matter expertise
- Learn new concepts from industry experts
- Gain a foundational understanding of a subject or tool
- Develop job-relevant skills with hands-on projects
- Earn a shareable career certificate
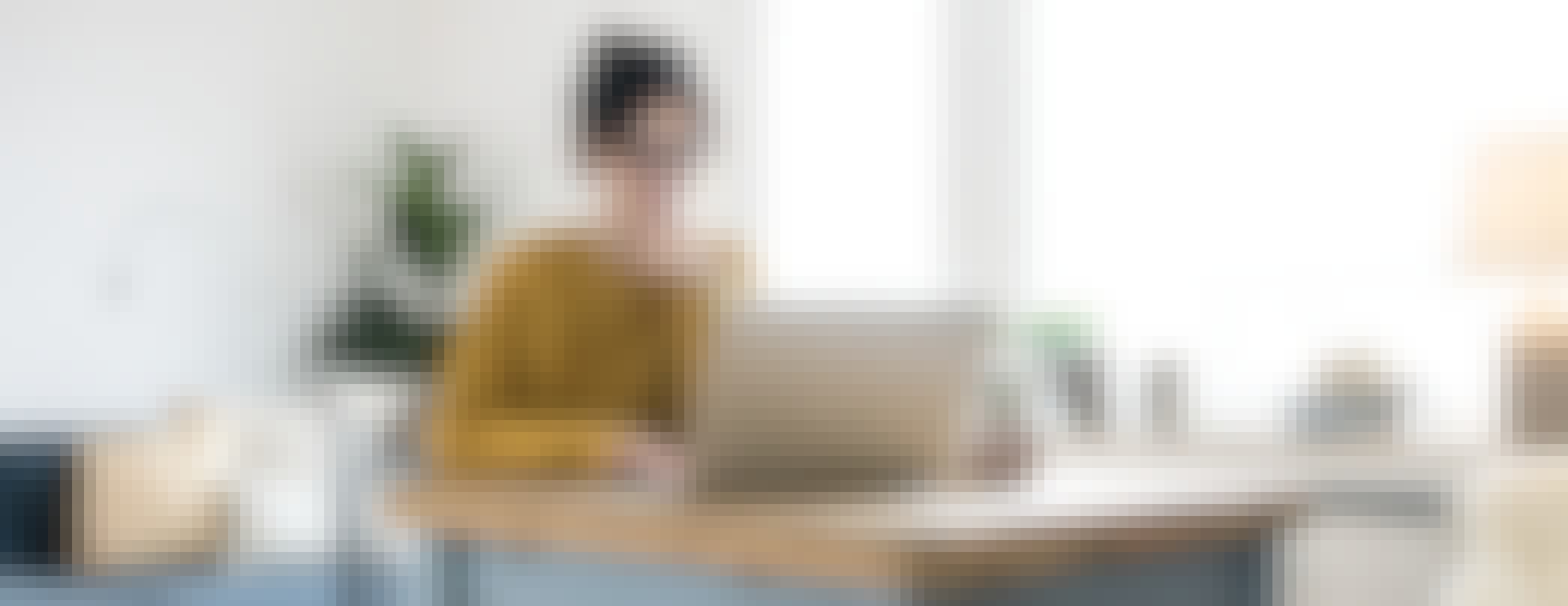
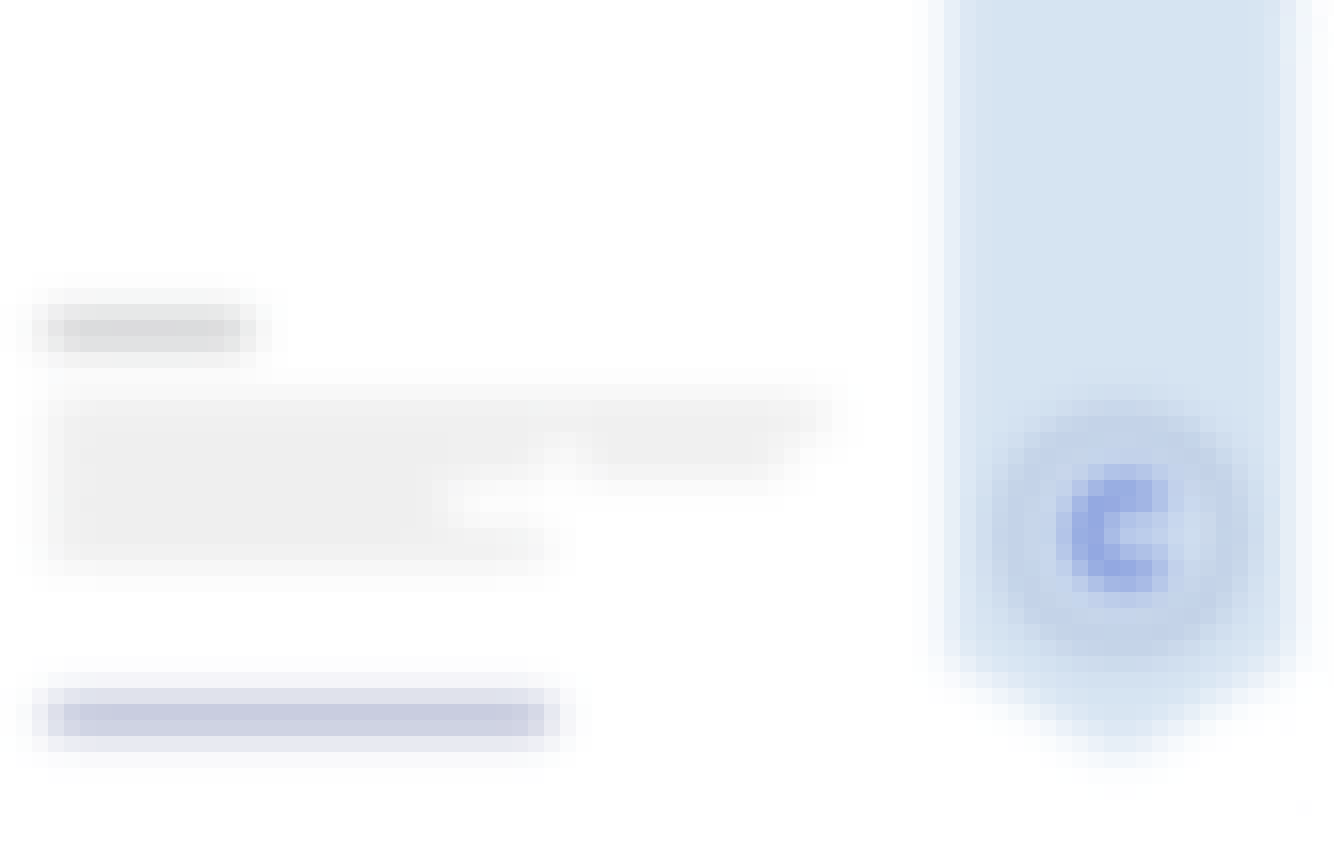
Earn a career certificate
Add this credential to your LinkedIn profile, resume, or CV
Share it on social media and in your performance review
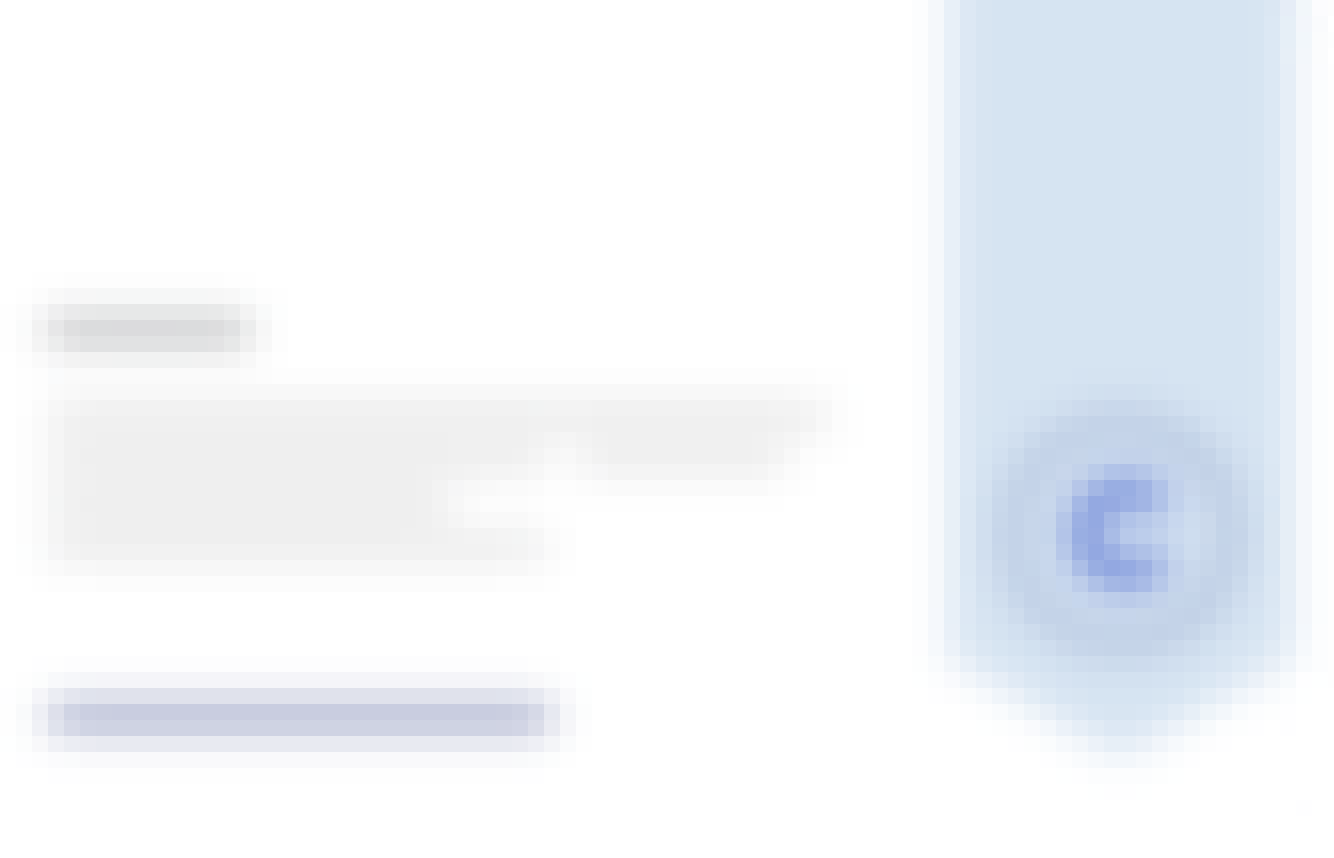
There are 6 modules in this course
This module previews the topics covered in the course and how to use Qwiklabs to complete each of your labs using Google Cloud.
What's included
2 videos2 readings
This module explores what else a production ML system needs to do and how to meet those needs. You review how to make important, high-level, design decisions around training and model serving need to make in order to get the right performance profile for your model.
What's included
10 videos1 reading1 assignment1 app item
In this module, you learn how to recognize the ways that our model is dependent on our data, make cost-conscious engineering decisions, know when to roll back our models to earlier versions, debug the causes of observed model behavior and implement a pipeline that is immune to one type of dependency.
What's included
14 videos1 reading1 assignment3 app items
In this module, you identify performance considerations for machine learning models. Machine learning models are not all identical. For some models, you focus on improving I/O performance, and on others, you focus on squeezing out more computational speed.
What's included
14 videos1 reading1 assignment2 app items
Understand the tools and systems available and when to leverage hybrid machine learning models.
What's included
7 videos1 reading1 assignment1 app item
This module reviews what you learned in this course.
What's included
1 video2 readings
Instructor

Offered by
Why people choose Coursera for their career




Learner reviews
996 reviews
- 5 stars
70.84%
- 4 stars
21.34%
- 3 stars
5.61%
- 2 stars
1.50%
- 1 star
0.70%
Showing 3 of 996
Reviewed on Sep 22, 2020
Unlike pure technical courses, this one specially packs you with knowledge that you may find yourself face to. The course is really well designed and the content is crystal clear, just Awesome !
Reviewed on Nov 3, 2018
This course reveals some practical techniques in Production Machine Learning Systems. I like the real world examples introduced in this course.
Reviewed on May 8, 2020
I got lots of new skills and I think it's a great course for ML
Recommended if you're interested in Data Science
Stanford University

Open new doors with Coursera Plus
Unlimited access to 10,000+ world-class courses, hands-on projects, and job-ready certificate programs - all included in your subscription
Advance your career with an online degree
Earn a degree from world-class universities - 100% online
Join over 3,400 global companies that choose Coursera for Business
Upskill your employees to excel in the digital economy