Python is one of the most widely used programming languages in machine learning (ML), and many ML job listings require it as a core skill. This course equips aspiring machine learning practitioners with essential Python skills that help them stand out to employers.

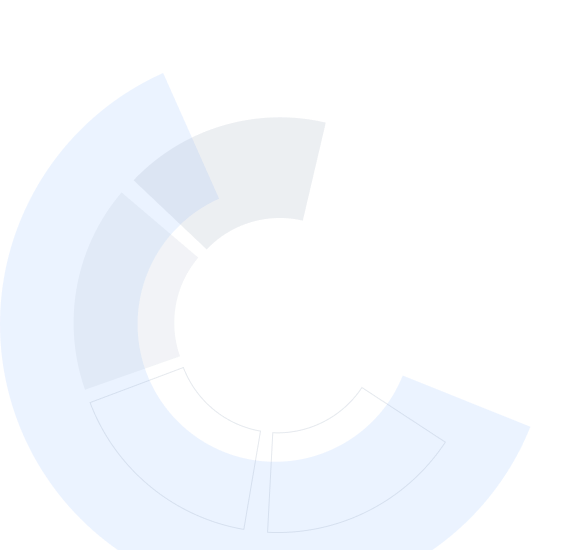
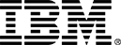
Machine Learning with Python
This course is part of multiple programs.


Instructors: Joseph Santarcangelo
Sponsored by Syrian Youth Assembly
501,832 already enrolled
(16,707 reviews)
Recommended experience
What you'll learn
Job-ready foundational machine learning skills in Python in just 6 weeks, including how to utilizeScikit-learn to build, test, and evaluate models.
How to apply data preparation techniques and manage bias-variance tradeoffs to optimize model performance.
How to implement core machine learning algorithms, including linear regression, decision trees, and SVM, for classification and regression tasks.
How to evaluate model performance using metrics, cross-validation, and hyperparameter tuning to ensure accuracy and reliability.
Skills you'll gain
Details to know

Add to your LinkedIn profile
15 assignments
See how employees at top companies are mastering in-demand skills
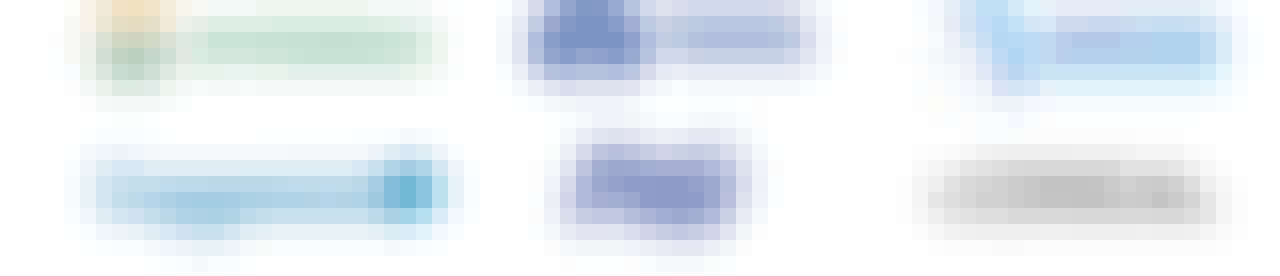
Build your subject-matter expertise
- Learn new concepts from industry experts
- Gain a foundational understanding of a subject or tool
- Develop job-relevant skills with hands-on projects
- Earn a shareable career certificate from IBM
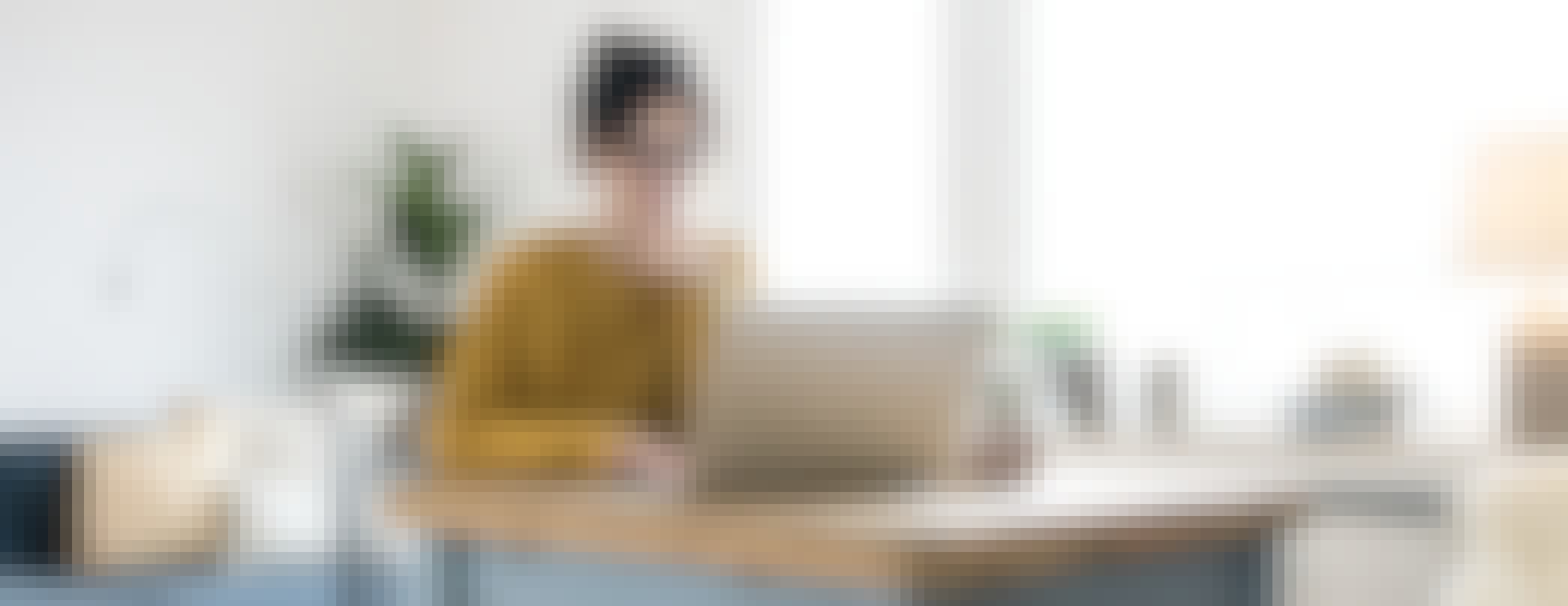
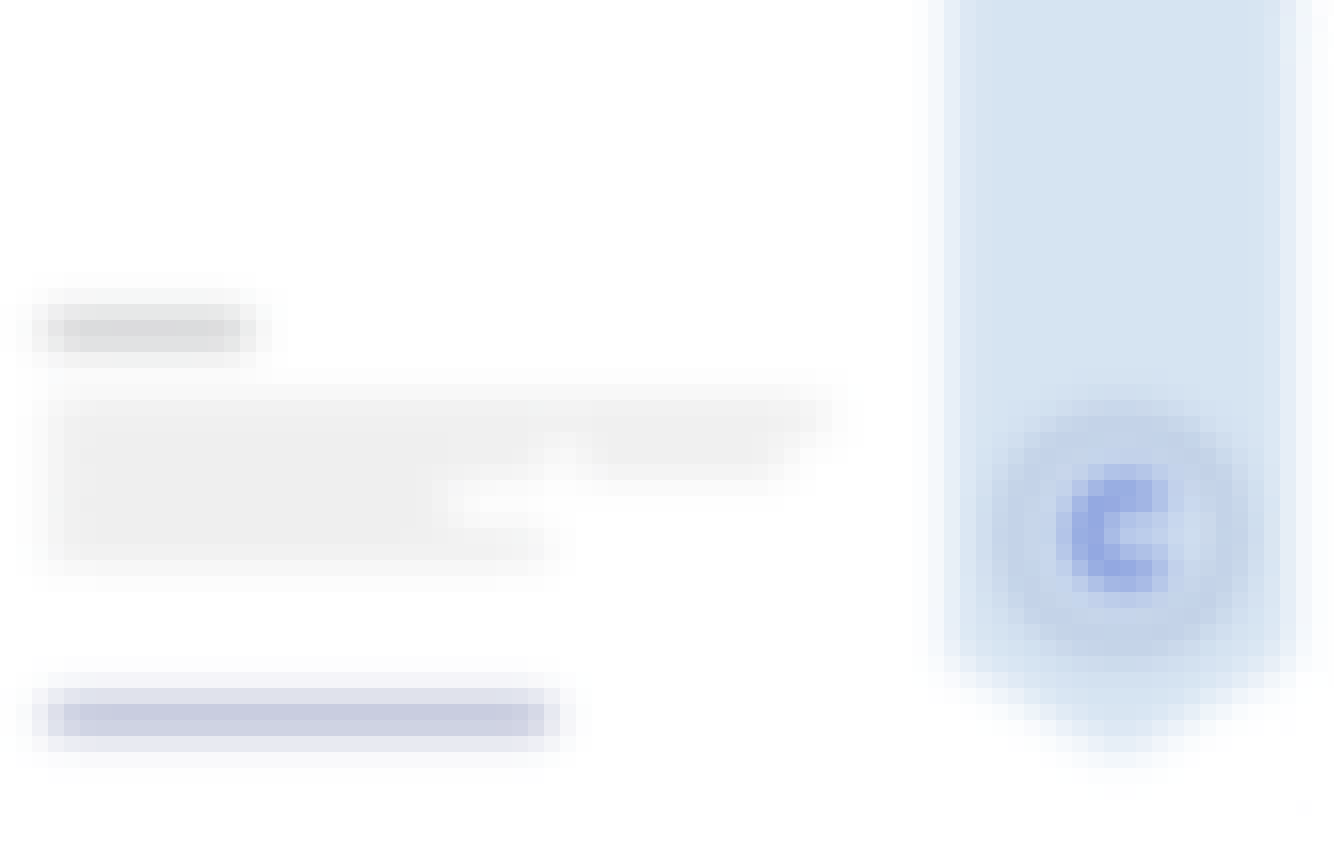
Earn a career certificate
Add this credential to your LinkedIn profile, resume, or CV
Share it on social media and in your performance review
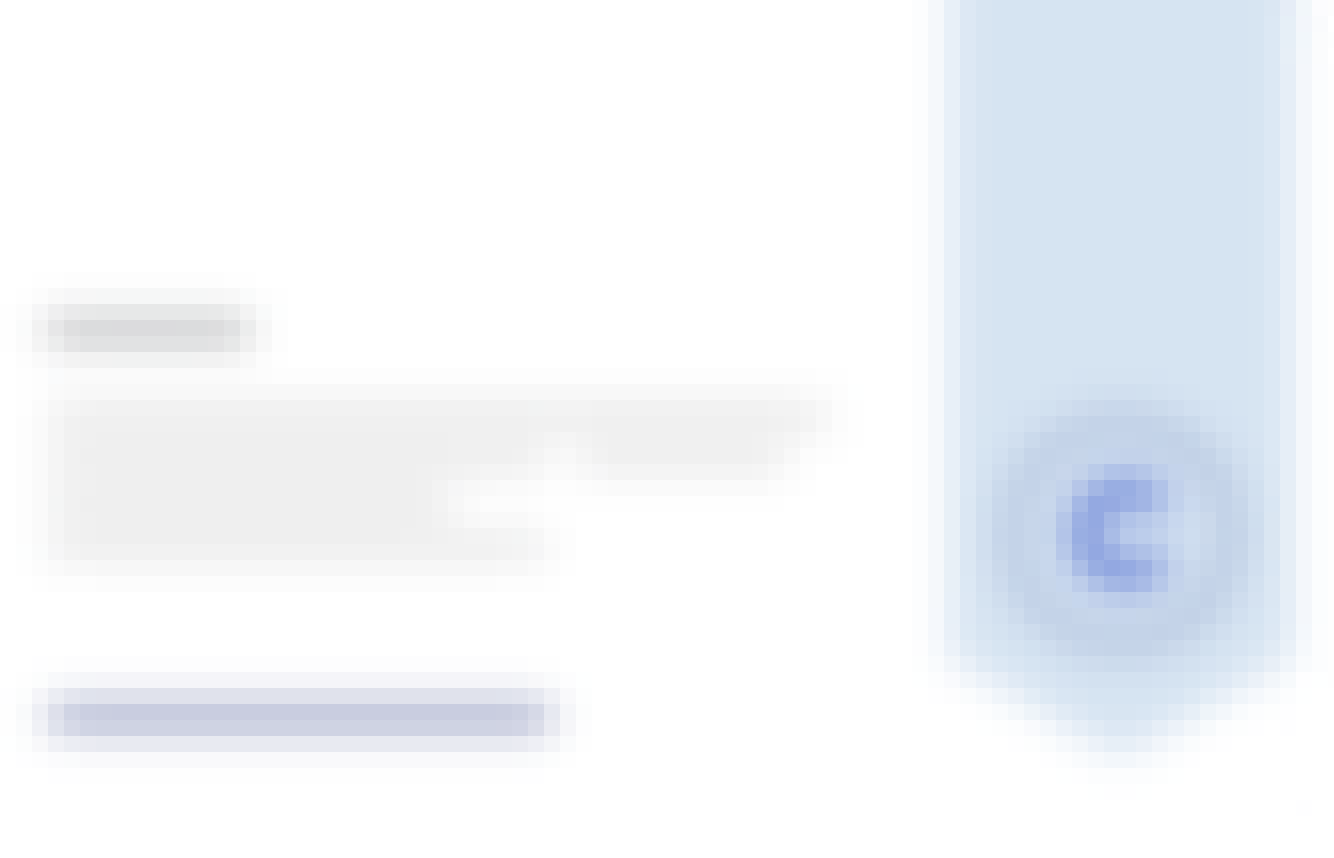
There are 6 modules in this course
This module provides you with knowledge of foundational machine learning concepts to delve deeper into applied machine learning modeling. You will learn that machine learning modeling is an iterative process with various lifecycle stages. You will also learn about the daily activities in the life of a machine learning engineer. Here, you will be introduced to various open-source tools for machine learning, including the popular Python package scikit-learn.
What's included
8 videos2 readings2 assignments1 plugin
In this module, you will explore two foundational statistical modeling methods, linear regression and logistic regression, which are considered classical machine learning models. Linear regression, often applied in real-world problem-solving, models a linear relationship between independent variables and a dependent variable. Logistic regression, an extension of linear regression, functions as a classifier and can handle nonlinear relationships through input transformation. By implementing these models, you'll gain insight into their limitations and better understand the advancements offered by modern machine learning models.
What's included
6 videos1 reading3 assignments3 app items1 plugin
In this module, you’ll learn about implementing modern supervised machine learning models. You will start by understanding how binary classification works and discover how to construct a multiclass classifier from binary classification components. You’ll learn what decision trees are, how they learn, and how to build them. Decision trees, which are used to solve classification problems, have a natural extension called regression trees, which can handle regression problems. You’ll learn about other supervised learning models, such as KNN and SVM. You’ll learn what bias and variance are in model fitting and the tradeoff between bias and variance inherent to all learning models in various degrees. You’ll learn strategies for mitigating this tradeoff and work with models that do a very good job accomplishing that goal.
What's included
6 videos1 reading3 assignments6 app items1 plugin
In this module, you'll explore unsupervised learning, a machine-learning approach that doesn't require labeled data. Instead of using correct answers, these algorithms identify patterns in data based on similarity. These patterns form clusters in an N-dimensional feature space, where data points that are close together can be considered clusters. Clusters may have a hierarchical structure, similar to natural systems such as galaxies or biological taxonomies. You'll learn about clustering algorithms and how unsupervised learning can reduce features for other modeling tasks, using Python to implement various clustering and dimensionality reduction techniques.
What's included
5 videos1 reading3 assignments4 app items1 plugin
In this module, you will learn how to evaluate the performance of supervised machine learning models using various metrics, depending on whether you are building classification or regression models. You will explore hyperparameter tuning techniques like cross-validation to prevent overfitting and ensure an unbiased model evaluation. Additionally, you will learn about regularization techniques for linear regression to mitigate overfitting caused by noise and outliers. Finally, you will gain hands-on experience in building, fine-tuning, and evaluating models using these techniques.
What's included
6 videos1 reading3 assignments5 app items1 plugin
This module focuses on applying and demonstrating the skills you have gained throughout the course by completing a comprehensive final assignment. In this assignment, you will analyze historical rainfall data to develop and optimize a classification model. You will perform feature engineering, evaluate the model's performance using different classifiers, and summarize your findings through visualizations. Once completed, your assignment will be graded automatically by an AI grading tool in the next section.
What's included
1 video3 readings1 assignment3 app items
Instructors


Offered by
Why people choose Coursera for their career




Learner reviews
16,707 reviews
- 5 stars
76.03%
- 4 stars
18.63%
- 3 stars
3.38%
- 2 stars
0.95%
- 1 star
0.98%
Showing 3 of 16707
Reviewed on Dec 31, 2019
could be split in two courses to be given enough focus. it was very condensed and needed more time and explanation in each section. The instructor was very good but more details would have been nice
Reviewed on Oct 8, 2020
I'm extremely excited with what I have learnt so far. As a newbie in Machine Learning, the exposure gained will serve as the much needed foundation to delve into its application to real life problems.
Reviewed on Jan 14, 2025
good course , some part is typical more statistical part shown, even i have good understanding of ML , so new learner will find little typical. rest tutor voice and language is understandable.
Recommended if you're interested in Data Science
Korea Advanced Institute of Science and Technology(KAIST)
DeepLearning.AI
O.P. Jindal Global University

Open new doors with Coursera Plus
Unlimited access to 10,000+ world-class courses, hands-on projects, and job-ready certificate programs - all included in your subscription
Advance your career with an online degree
Earn a degree from world-class universities - 100% online
Join over 3,400 global companies that choose Coursera for Business
Upskill your employees to excel in the digital economy