In this course, we’ll learn about more advanced machine learning methods that are used to tackle problems in the supply chain. We’ll start with an overview of the different ML paradigms (regression/classification) and where the latest models fit into these breakdowns. Then, we’ll dive deeper into some of the specific techniques and use cases such as using neural networks to predict product demand and random forests to classify products. An important part to using these models is understanding their assumptions and required preprocessing steps. We’ll end with a project incorporating advanced techniques with an image classification problem to find faulty products coming out of a machine.

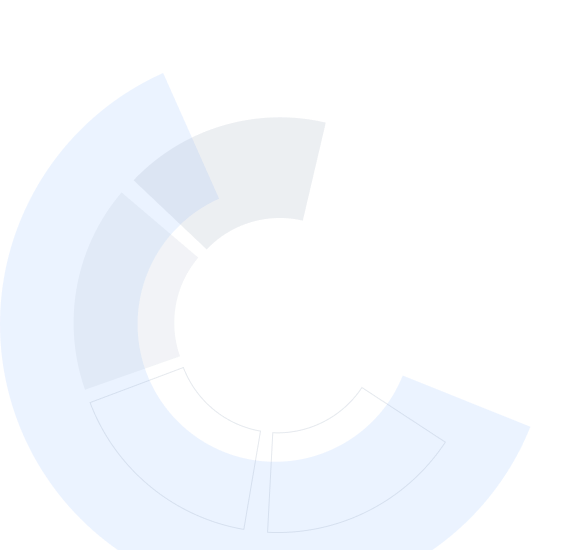
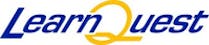
Advanced AI Techniques for the Supply Chain
This course is part of Machine Learning for Supply Chains Specialization


Instructors: Rajvir Dua
Sponsored by Louisiana Workforce Commission
1,891 already enrolled
(13 reviews)
Recommended experience
Skills you'll gain
Details to know

Add to your LinkedIn profile
4 assignments
See how employees at top companies are mastering in-demand skills
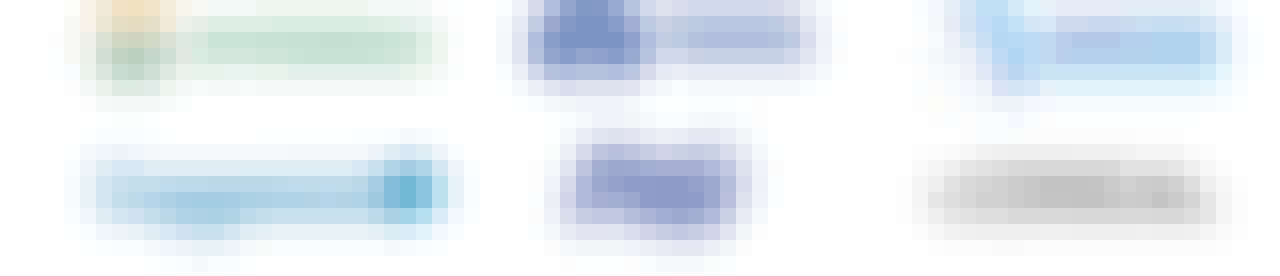
Build your subject-matter expertise
- Learn new concepts from industry experts
- Gain a foundational understanding of a subject or tool
- Develop job-relevant skills with hands-on projects
- Earn a shareable career certificate
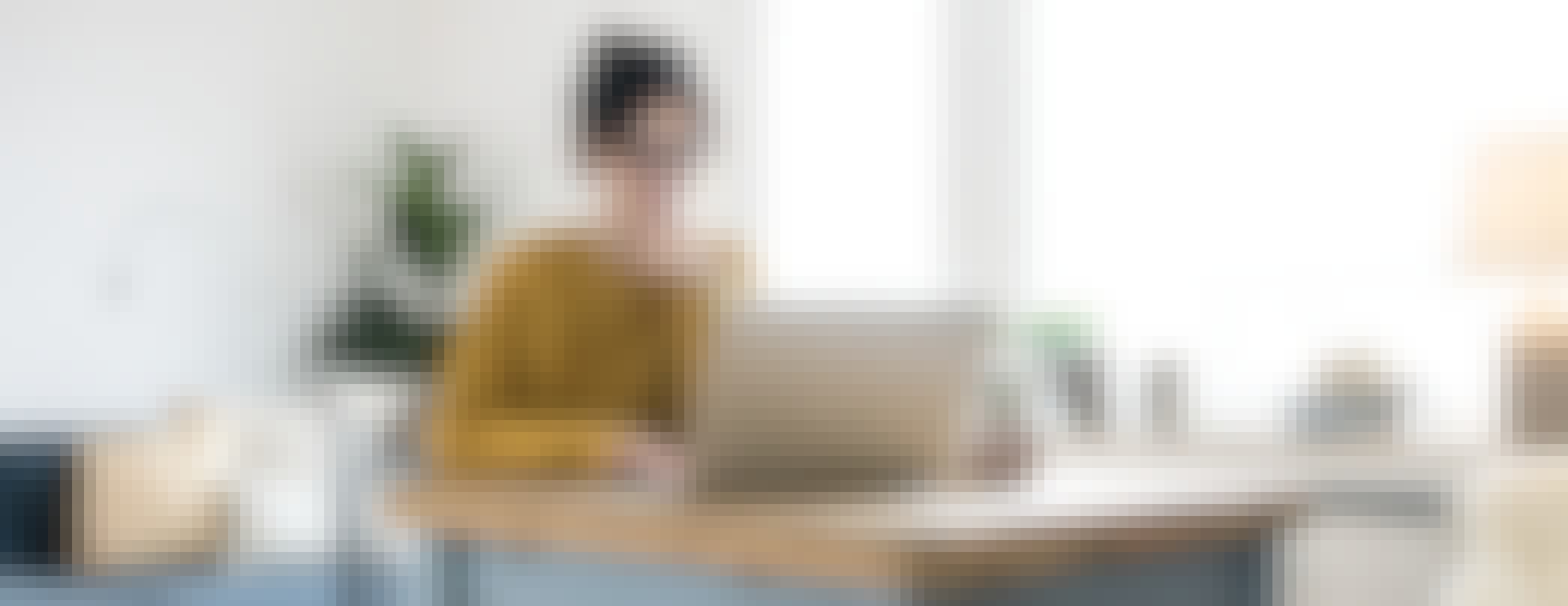
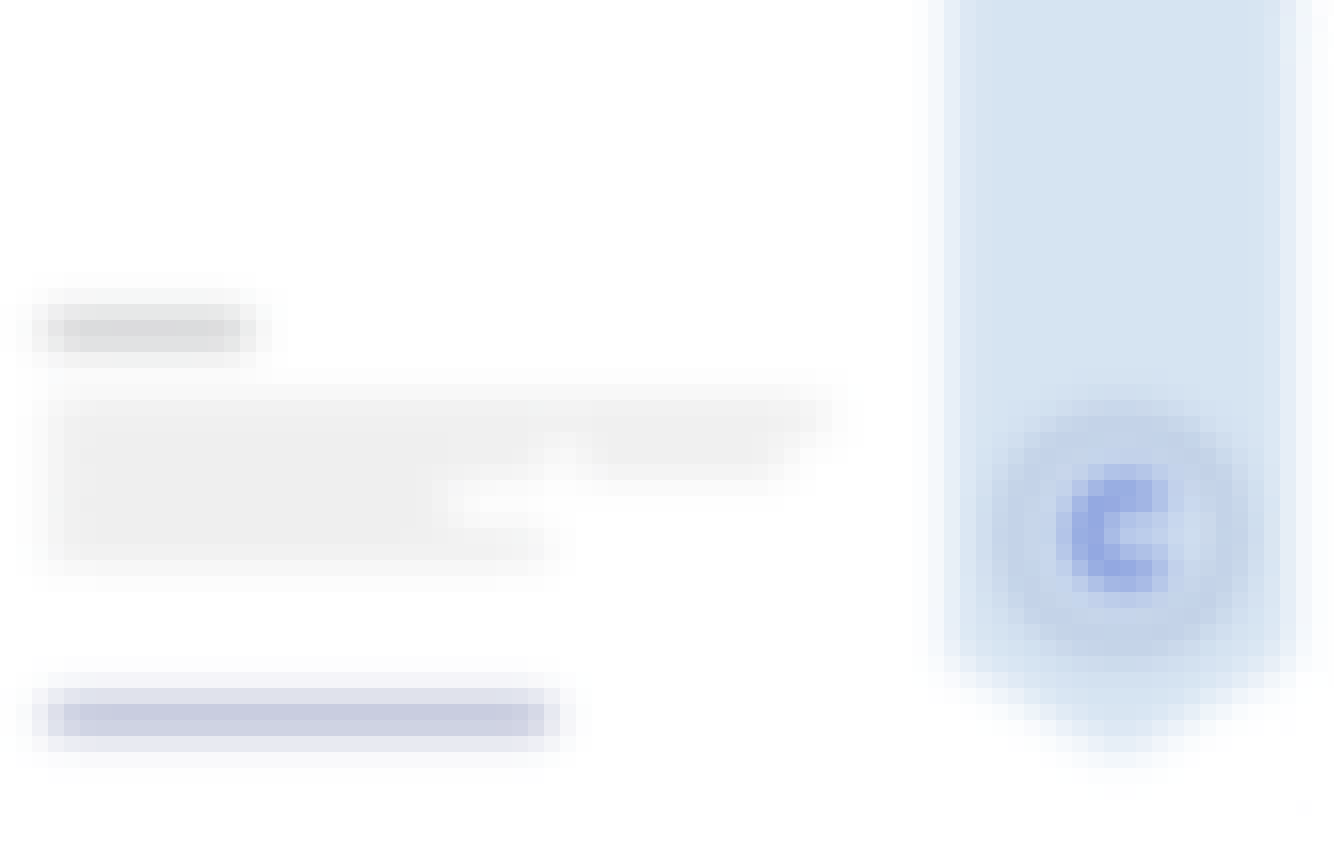
Earn a career certificate
Add this credential to your LinkedIn profile, resume, or CV
Share it on social media and in your performance review
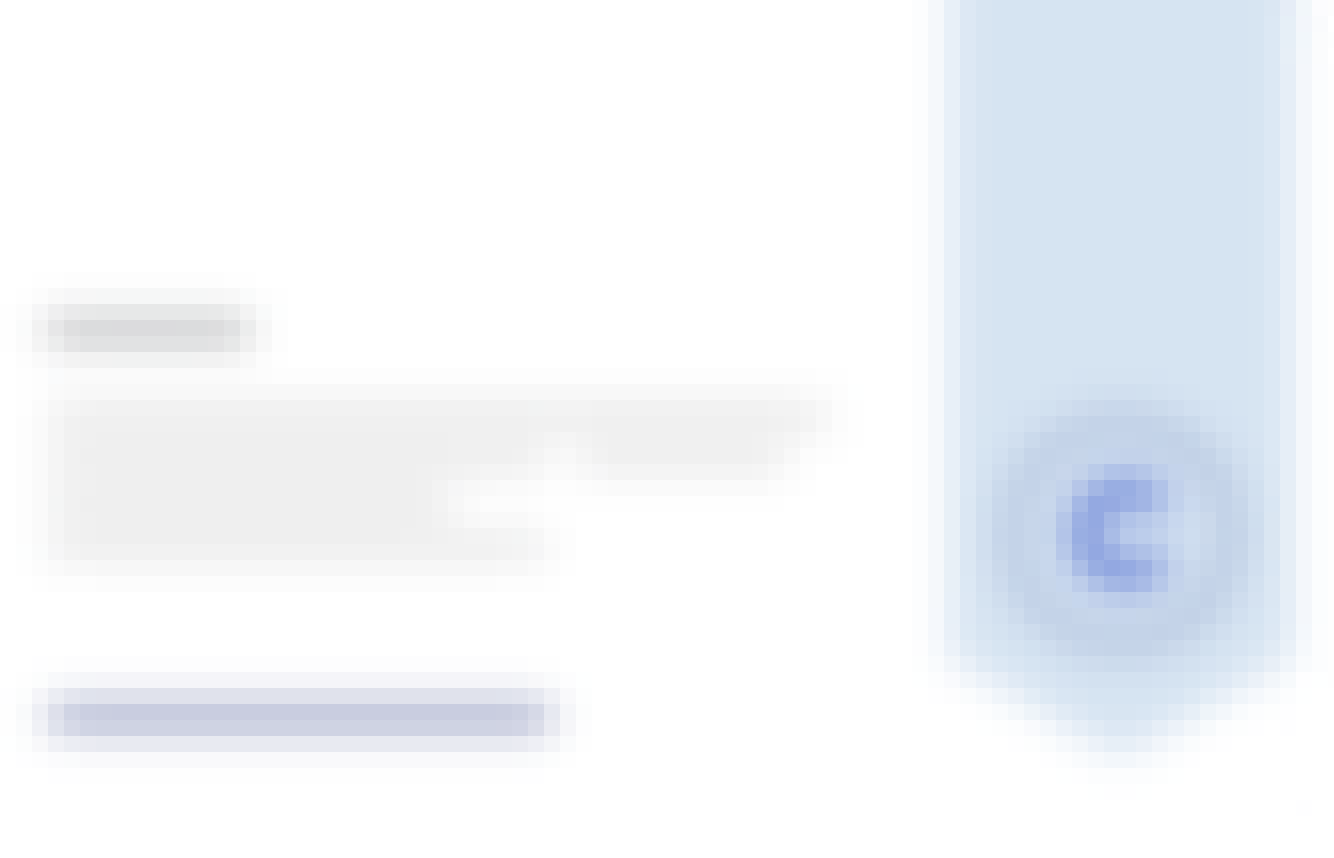
There are 4 modules in this course
In this module, we'll learn about the use cases of machine learning in the supply chain. We'll start with the big picture applications before diving deeper into specific algorithms, including neural networks. Throughout the module, we'll explain not only the general artificial intelligence concepts and mathematics, but also how these algorithms can specifically be used for the supply chain.
What's included
4 videos4 readings2 assignments1 discussion prompt1 ungraded lab
In this module, we'll cover the concepts relating to the ML paradigm. We'll start by learning how to pick a model, relying on considerations such as managing the bias-variance tradeoff. Next, we'll explore how machine learning models converge, including the use of stochastic gradient descent to minimize loss functions. Finally, we'll end with some practical considerations on coding advanced AI models with libraries for hyperparamter tuning.
What's included
3 videos4 readings1 assignment1 programming assignment3 ungraded labs
In this module, we'll expand beyond numbers and learn how to use machine learning on images and text. We'll start by talking about how to analyze text data and cover the primary methods behind natural language processing. Then, we'll learn how to analyze images by constructing convolutional neural networks complete with convolutions and pooling layers.
What's included
5 videos5 readings1 assignment1 discussion prompt2 ungraded labs
In this final project, we’ll apply what we learned in the last module to classify images of products based on whether there is a defect or not.
What's included
1 programming assignment1 ungraded lab
Offered by
Why people choose Coursera for their career




Recommended if you're interested in Data Science
Scrimba
Rutgers the State University of New Jersey
Korea Advanced Institute of Science and Technology(KAIST)
Emory University

Open new doors with Coursera Plus
Unlimited access to 10,000+ world-class courses, hands-on projects, and job-ready certificate programs - all included in your subscription
Advance your career with an online degree
Earn a degree from world-class universities - 100% online
Join over 3,400 global companies that choose Coursera for Business
Upskill your employees to excel in the digital economy