The course "Advanced Neural Network Techniques" delves into advanced neural network methodologies, offering learners an in-depth understanding of cutting-edge techniques such as Recurrent Neural Networks (RNNs), Autoencoders, Generative Neural Networks, and Deep Reinforcement Learning. Through hands-on projects and practical applications, learners will master the mathematical foundations and deployment strategies behind these models.

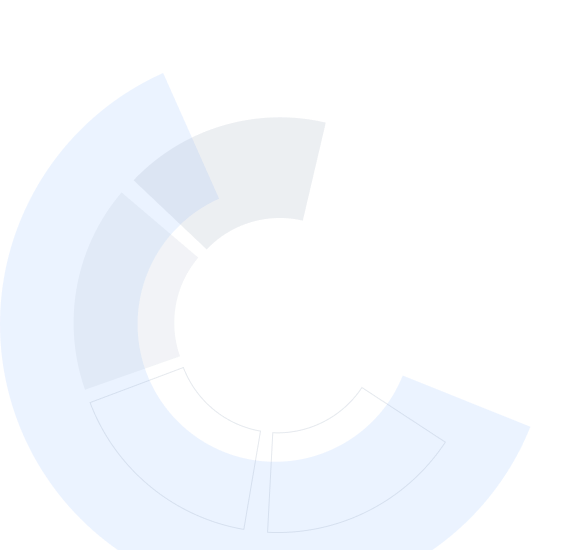
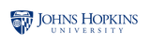
Advanced Neural Network Techniques
This course is part of Foundations of Neural Networks Specialization

Instructor: Zerotti Woods
Sponsored by Louisiana Workforce Commission
Recommended experience
What you'll learn
Analyze and implement Recurrent Neural Networks (RNNs) to process sequence data and solve tasks like time series prediction and language modeling.
Explore autoencoders for data compression, feature extraction, and anomaly detection, along with their applications in diverse fields.
Develop and evaluate generative models, such as GANs, understanding their mathematical foundations and deployment challenges.
Apply reinforcement learning techniques using Markov Chains and deep neural networks to tackle complex decision-making problems.
Details to know

Add to your LinkedIn profile
8 assignments
December 2024
See how employees at top companies are mastering in-demand skills
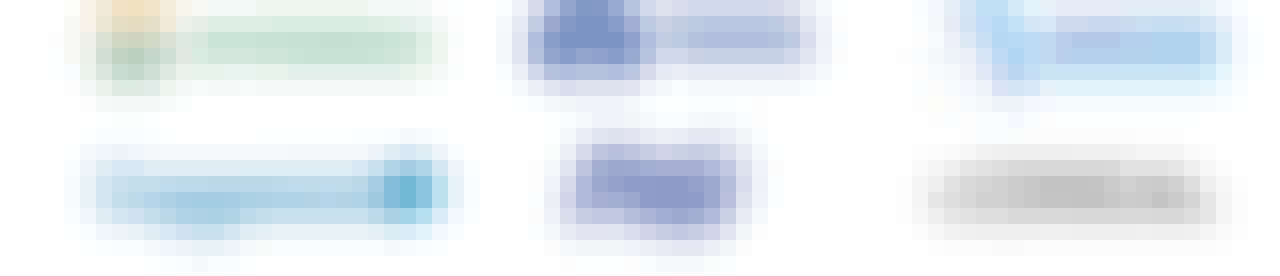
Build your subject-matter expertise
- Learn new concepts from industry experts
- Gain a foundational understanding of a subject or tool
- Develop job-relevant skills with hands-on projects
- Earn a shareable career certificate
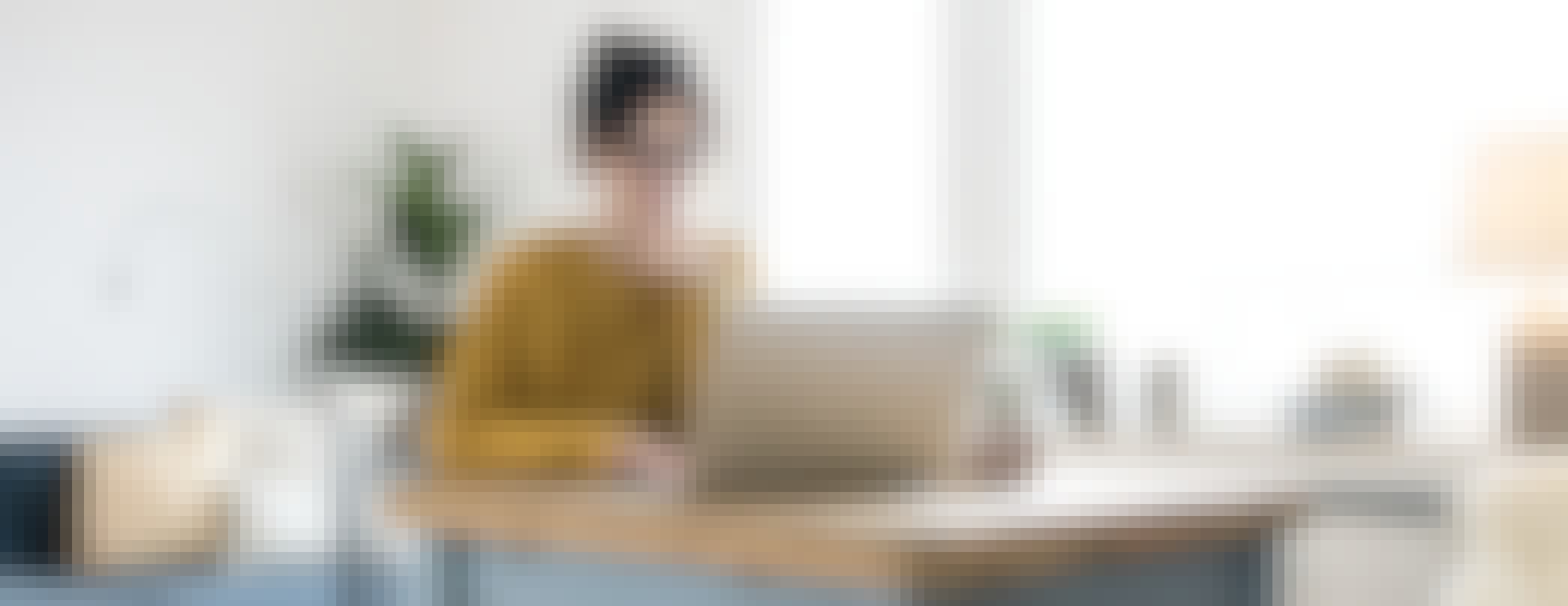
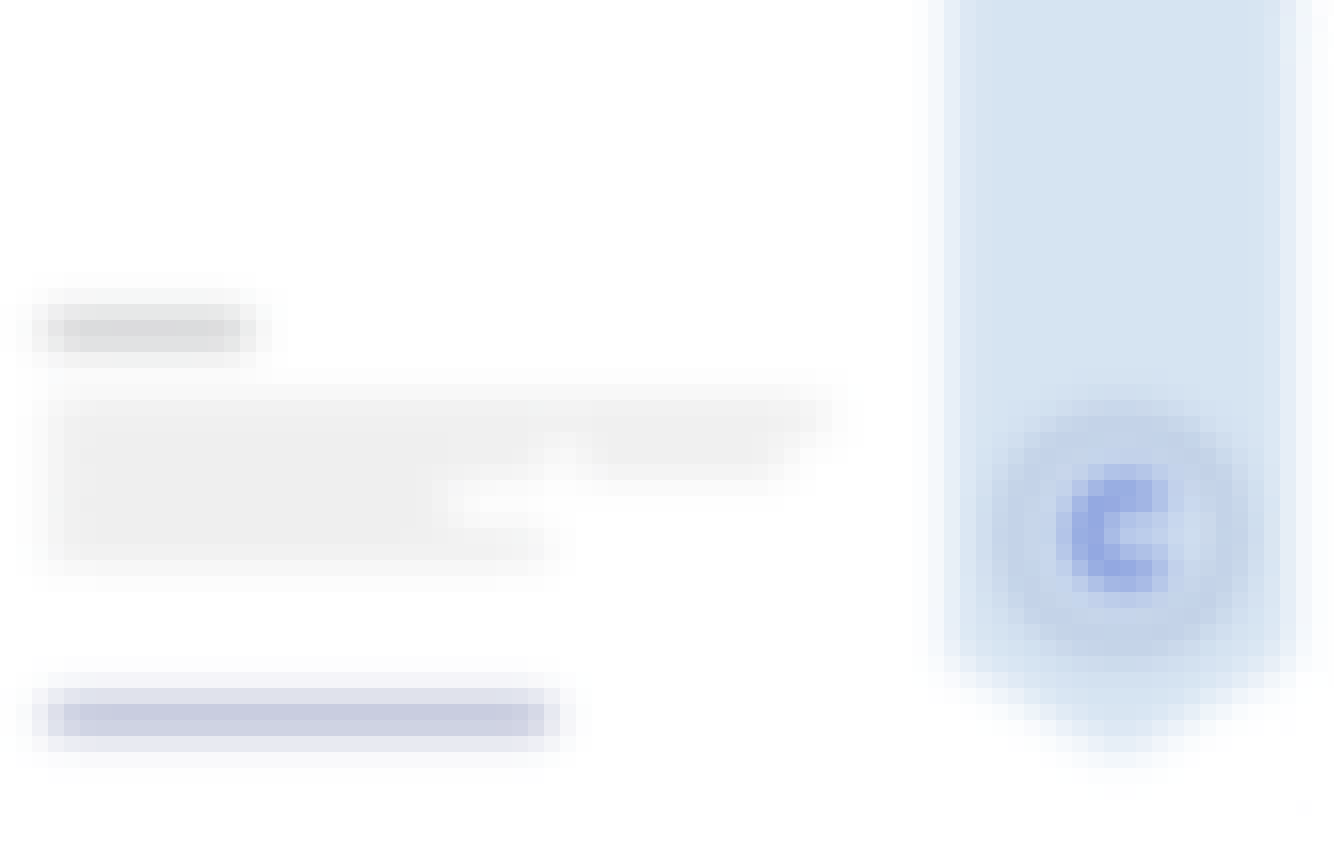
Earn a career certificate
Add this credential to your LinkedIn profile, resume, or CV
Share it on social media and in your performance review
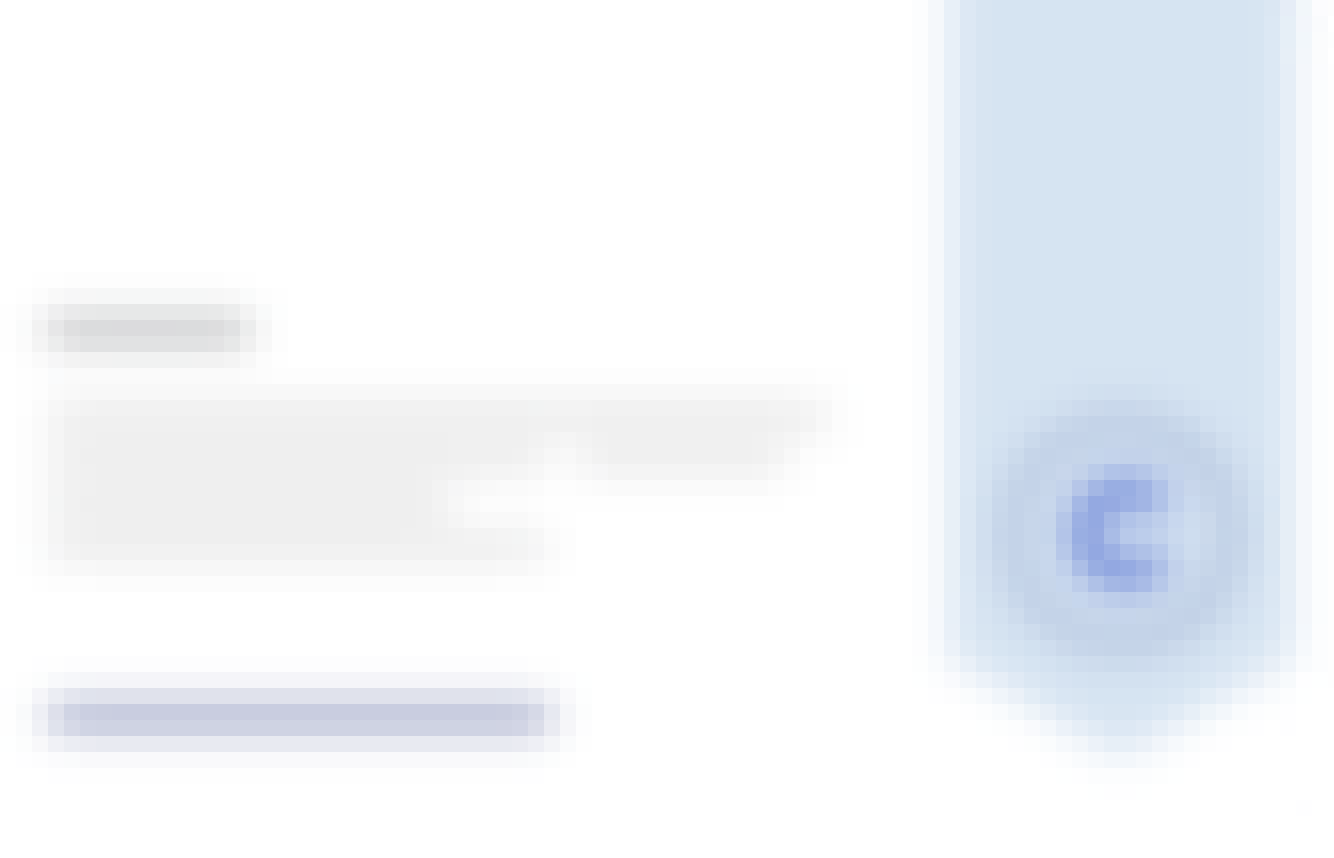
There are 5 modules in this course
This course explores advanced concepts and methodologies in neural networks, focusing on Recurrent Neural Networks (RNNs) and Autoencoders. You will analyze the core elements of these architectures, evaluate their applications across various domains, and propose innovative research directions. The curriculum also covers Generative Neural Networks, including their mathematical foundations and deployment constraints. Additionally, learners will gain hands-on experience in Reinforcement Learning, utilizing Markov Chains and Deep Neural Networks to solve complex problems. By the end of the course, you will be equipped with the skills to drive advancements in the field of neural networks.
What's included
2 readings
This module will discuss Recurrent Neural Networks. Students will explore the reasons for RNNS along with different techniques.
What's included
1 video1 reading2 assignments1 ungraded lab
This module will discuss Auto Encoders. Learners will explore the reasons for autoencoders along with different techniques and applications.
What's included
1 video1 reading2 assignments
This module will discuss Generative Deep Learning Models. You will study two particular models and go through examples of where they have been successfully deployed.
What's included
1 video1 reading2 assignments
This module will introduce reinforcement learning. We will discuss Markov Chains, Q-learning, and Deep Q-learning.
What's included
4 videos1 reading2 assignments
Instructor

Offered by
Why people choose Coursera for their career




Recommended if you're interested in Computer Science
University of Illinois Urbana-Champaign
University of Alberta
DeepLearning.AI

Open new doors with Coursera Plus
Unlimited access to 10,000+ world-class courses, hands-on projects, and job-ready certificate programs - all included in your subscription
Advance your career with an online degree
Earn a degree from world-class universities - 100% online
Join over 3,400 global companies that choose Coursera for Business
Upskill your employees to excel in the digital economy