Business professionals in non-technical roles have a unique opportunity to lead or influence machine learning projects. If you have questions about machine learning and want to understand how to use it, without the technical jargon, this course is for you. Learn how to translate business problems into machine learning use cases and vet them for feasibility and impact. Find out how you can discover unexpected use cases, recognize the phases of an ML project and considerations within each, and gain confidence to propose a custom ML use case to your team or leadership or translate the requirements to a technical team.

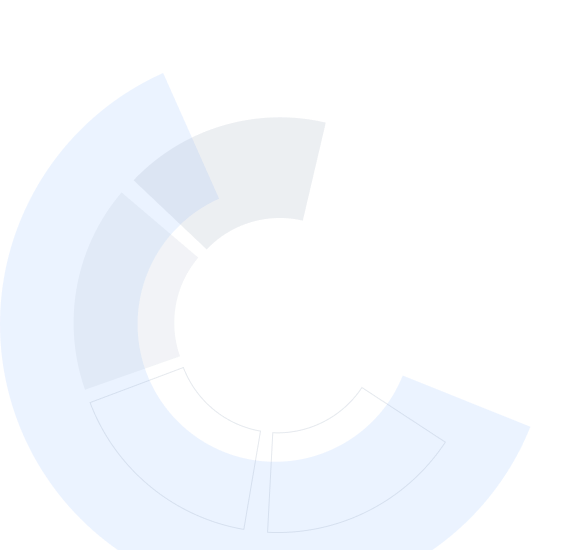
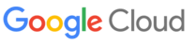
Managing Machine Learning Projects with Google Cloud
This course is part of Digital Transformation Using AI/ML with Google Cloud Specialization

Instructor: Google Cloud Training
Sponsored by Louisiana Workforce Commission
122,609 already enrolled
(3,666 reviews)
What you'll learn
Explore common machine learning use cases implemented by businesses.
Assess the feasibility of your own ML use case and its ability to meaningfully impact your business.
Identify the requirements to build, train, and evaluate an ML model.
Define data characteristics and biases that affect the quality of ML models and recognize key considerations for managing ML projects.
Skills you'll gain
Details to know

Add to your LinkedIn profile
6 assignments
See how employees at top companies are mastering in-demand skills
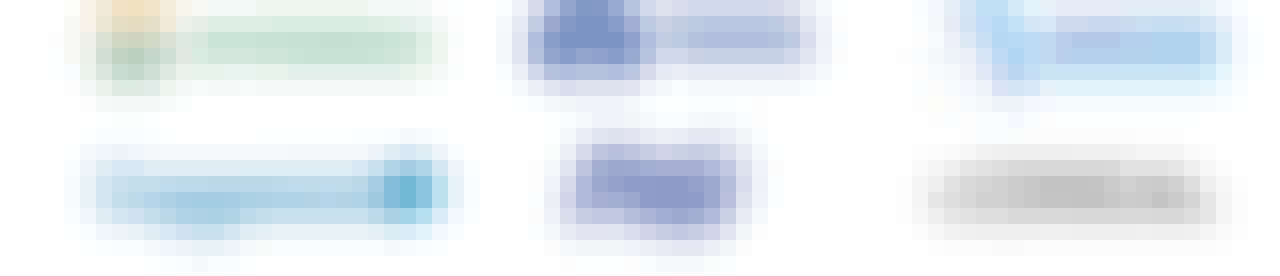
Build your subject-matter expertise
- Learn new concepts from industry experts
- Gain a foundational understanding of a subject or tool
- Develop job-relevant skills with hands-on projects
- Earn a shareable career certificate
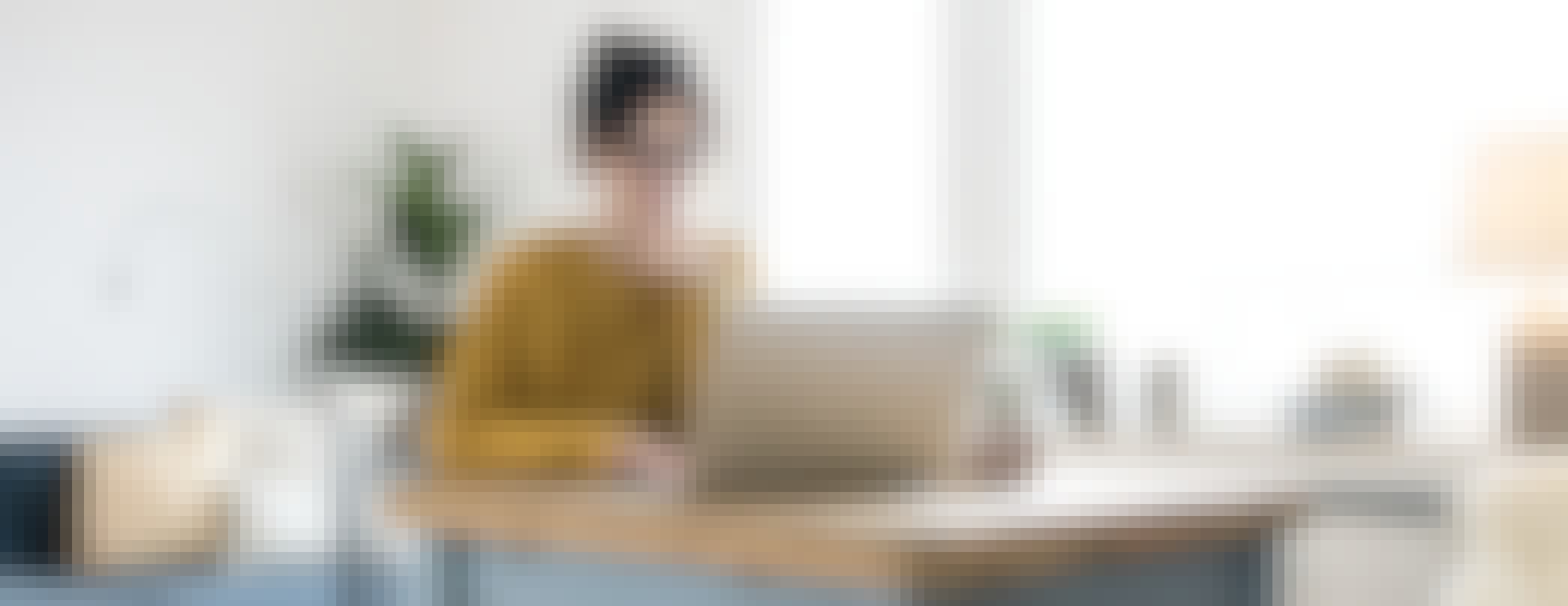
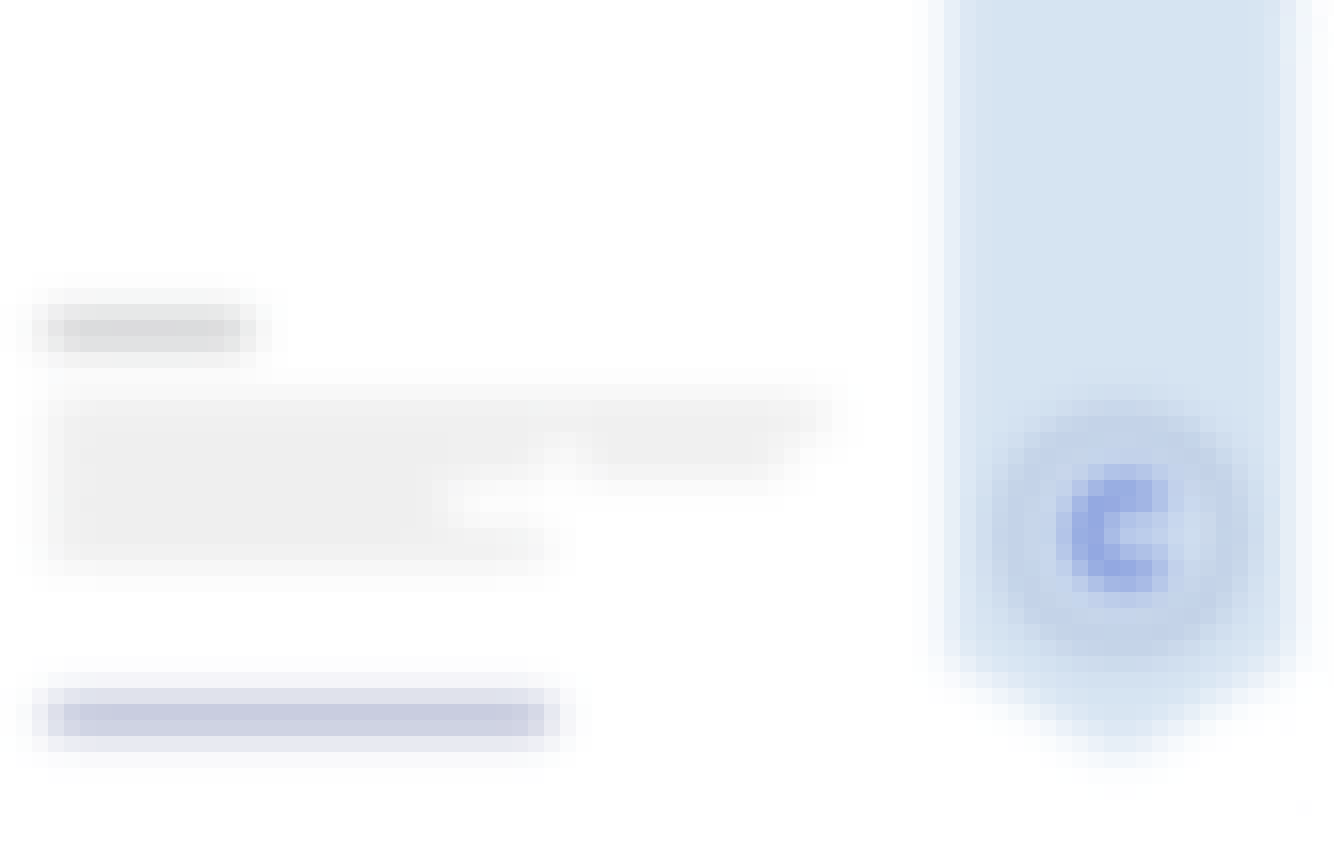
Earn a career certificate
Add this credential to your LinkedIn profile, resume, or CV
Share it on social media and in your performance review
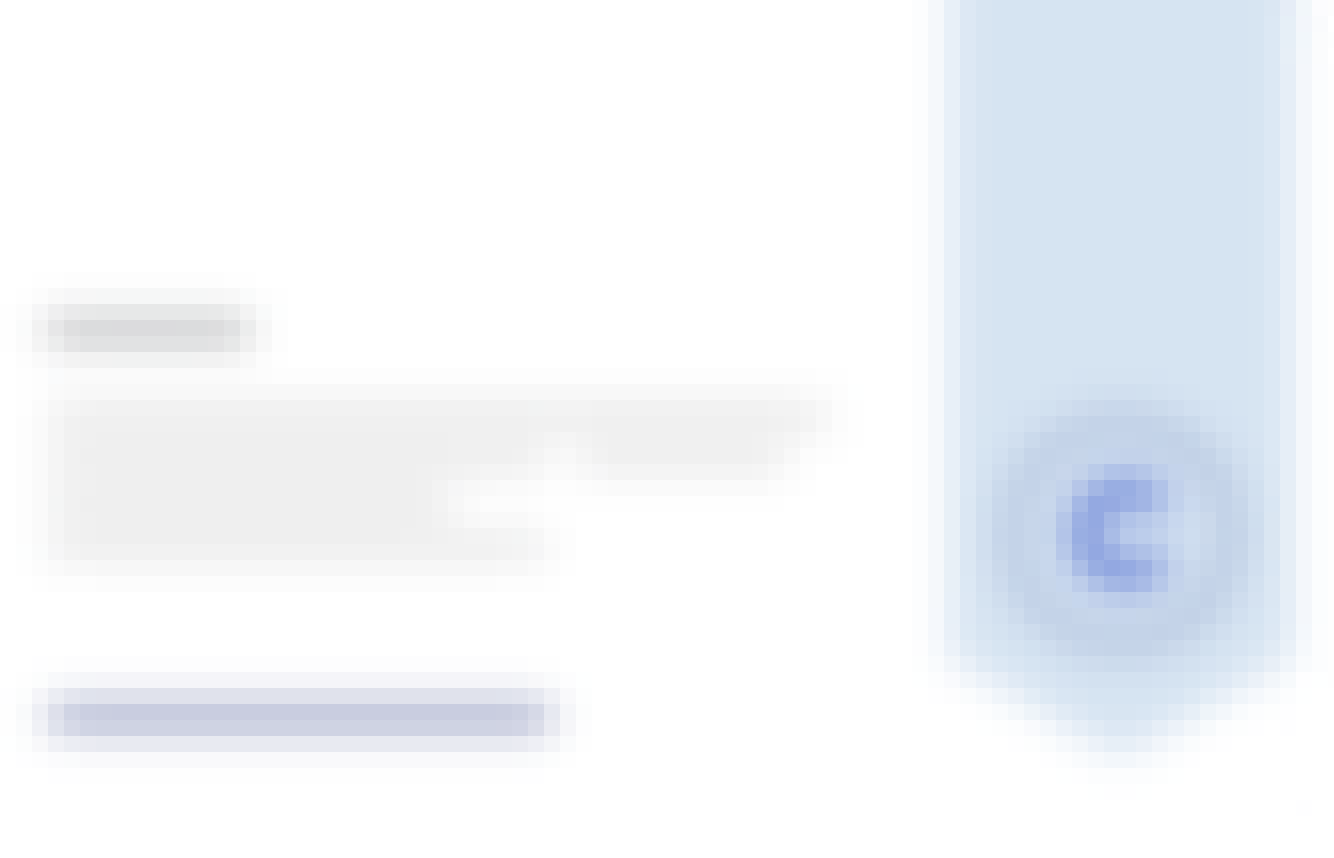
There are 8 modules in this course
Welcome to the course! In this module, you'll meet the instructor and learn about the course content and how to get started.
What's included
1 video2 readings
This module begins by defining machine learning at a high level and then helps you gain a thorough understanding of its value for business by reviewing several real-world examples. It then introduces machine learning projects and provides practice using a tool to assess the feasibility of several ML problems.
What's included
4 videos1 reading1 assignment
This module begins by defining machine learning at a high level and then helps you gain a thorough understanding of its value for business by reviewing several real-world examples. It then introduces machine learning projects and provides practice using a tool to assess the feasibility of several ML problems.
What's included
9 videos1 reading1 assignment
After you have assessed the feasibility of your supervised ML problem, you're ready to move to the next phase of an ML project. This module explores the various considerations and requirements for building a complete dataset in preparation for training, evaluating, and deploying an ML model. It also includes two demos—Vision API and AutoML Vision—as relevant tools that you can easily access yourself or in partnership with a data scientist. You'll also have the opportunity to try out AutoML Vision with the first hands-on lab.
What's included
6 videos1 assignment1 app item
Data in the world is inherently biased, and that bias can be amplified through ML solutions. In this module, you'll learn about some of the most common biases and how they can disproportionately affect or harm an individual or groups of individuals. You'll also be given guidelines for uncovering possible biases at each phase of an ML project and strategies for achieving ML fairness as much as possible.
What's included
6 videos1 assignment1 app item
This module explores 5 general themes for discovering ML use cases within day-to-day business, followed by concrete customer examples. You'll learn about creative applications of ML, such as improving the resolution of images or generating music.
What's included
6 videos1 reading1 assignment1 app item
When you thoroughly understand the fundamentals of machine learning and considerations within in each phase of the project, you're ready to learn about the best practices for managing an ML project. This module describes 5 key considerations for successfully managing an ML project end-to-end: identifying the business value, developing a data strategy, establishing data governance, building successful ML teams, and enabling a culture of innovation. You'll also have an opportunity to gain further exposure to one of Google Cloud's tools by completing a final hands-on lab: Evaluate an ML Model with BigQuery ML.
What's included
7 videos1 assignment1 app item
This module provides a summary of the key points covered in each of the modules in the course.
What's included
1 video
Instructor

Offered by
Why people choose Coursera for their career




Learner reviews
3,666 reviews
- 5 stars
70.37%
- 4 stars
23.59%
- 3 stars
4.06%
- 2 stars
0.70%
- 1 star
1.25%
Showing 3 of 3666
Reviewed on Jun 18, 2020
A precise combination of theory and lab oriented study which throws light on why, how ML can be beneficial encompassing ethical questions and a change in the culture needed to enforce ML.
Reviewed on Aug 8, 2020
This course was informative and I learned a lot about Machine Learning and the Google Cloud Platform. The platform was easy to follow even though I had some bumps when accessing QwilLabs.
Reviewed on Sep 7, 2020
Excelente curso para adentrarse en el mundo de Machine Learning desde la perspectiva de la empresa que posee el conocimiento más amplio del tema. No necesitarás saber NADA de código.
Recommended if you're interested in Data Science
Google Cloud
Google Cloud

Open new doors with Coursera Plus
Unlimited access to 10,000+ world-class courses, hands-on projects, and job-ready certificate programs - all included in your subscription
Advance your career with an online degree
Earn a degree from world-class universities - 100% online
Join over 3,400 global companies that choose Coursera for Business
Upskill your employees to excel in the digital economy