Probabilistic graphical models (PGMs) are a rich framework for encoding probability distributions over complex domains: joint (multivariate) distributions over large numbers of random variables that interact with each other. These representations sit at the intersection of statistics and computer science, relying on concepts from probability theory, graph algorithms, machine learning, and more. They are the basis for the state-of-the-art methods in a wide variety of applications, such as medical diagnosis, image understanding, speech recognition, natural language processing, and many, many more. They are also a foundational tool in formulating many machine learning problems.

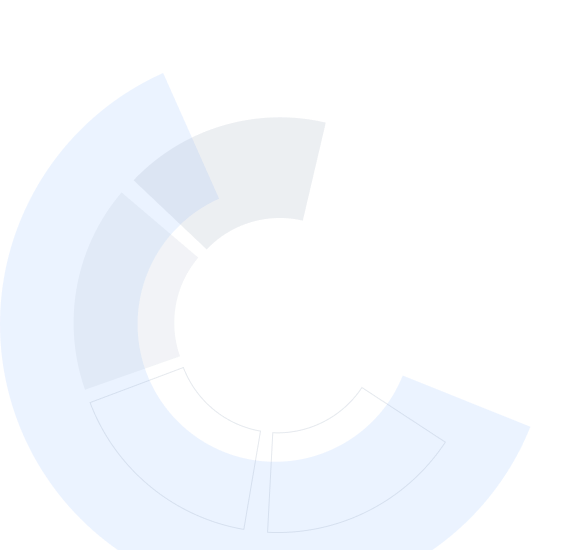
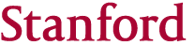
Probabilistic Graphical Models 2: Inference
This course is part of Probabilistic Graphical Models Specialization

Instructor: Daphne Koller
Sponsored by Louisiana Workforce Commission
26,108 already enrolled
(485 reviews)
Details to know

Add to your LinkedIn profile
8 assignments
See how employees at top companies are mastering in-demand skills
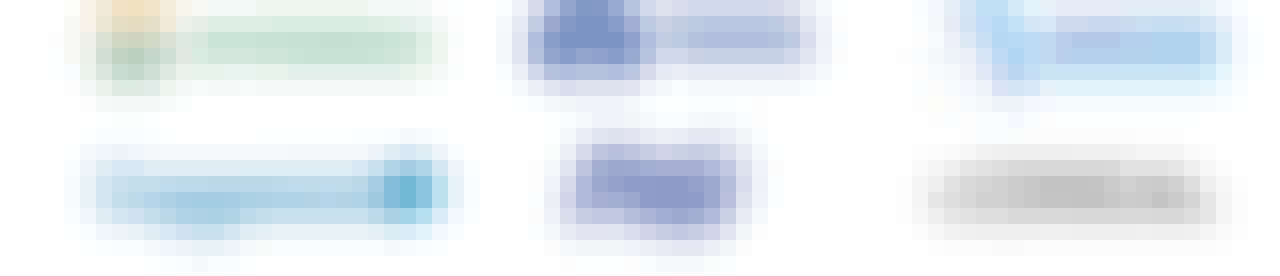
Build your subject-matter expertise
- Learn new concepts from industry experts
- Gain a foundational understanding of a subject or tool
- Develop job-relevant skills with hands-on projects
- Earn a shareable career certificate
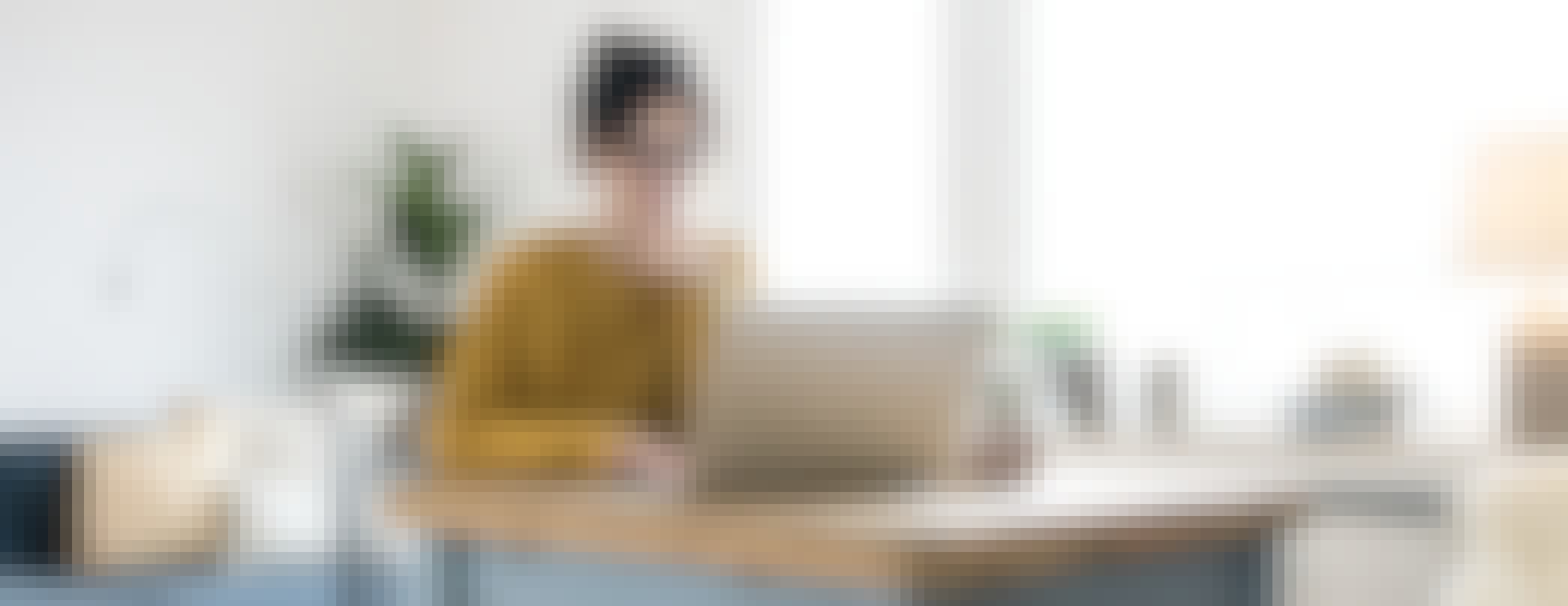
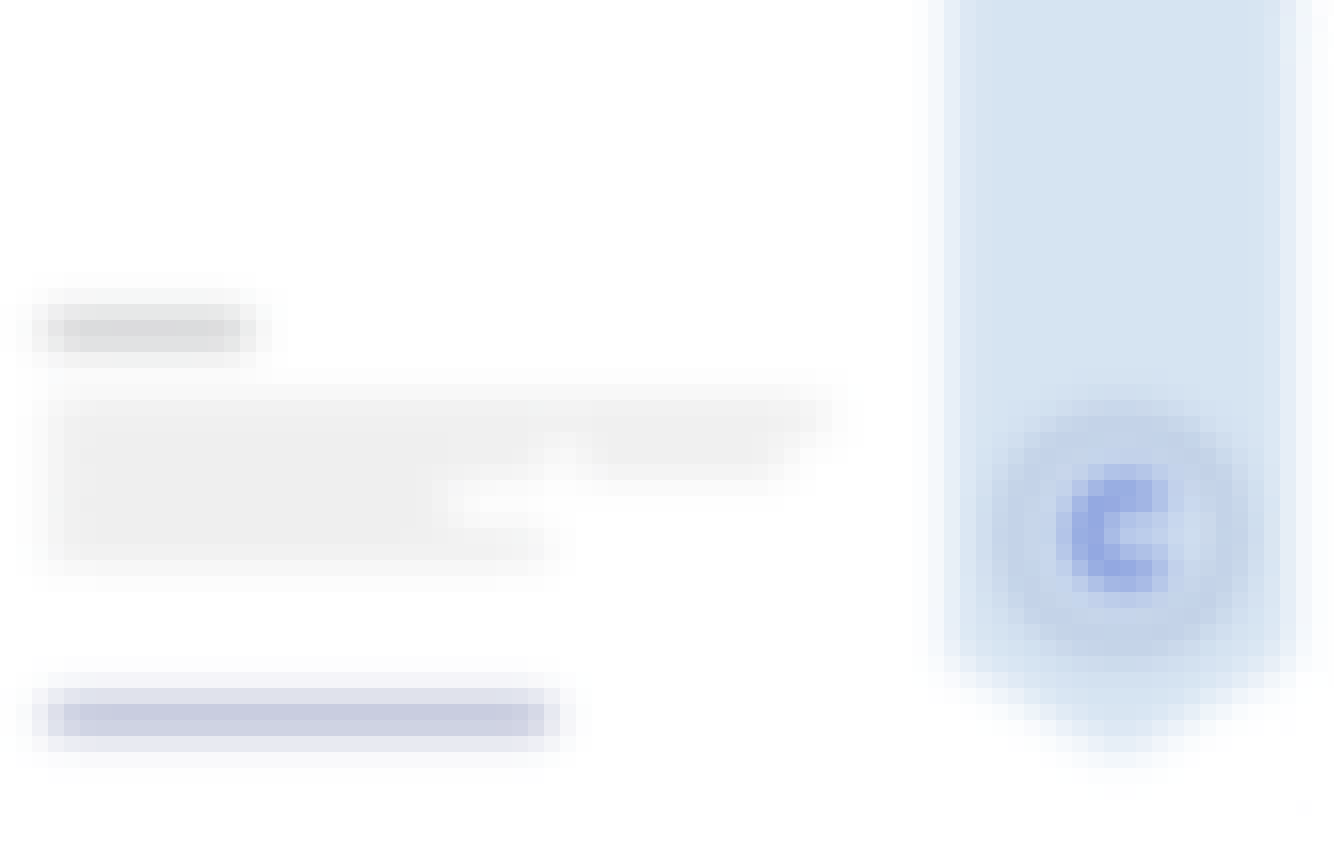
Earn a career certificate
Add this credential to your LinkedIn profile, resume, or CV
Share it on social media and in your performance review
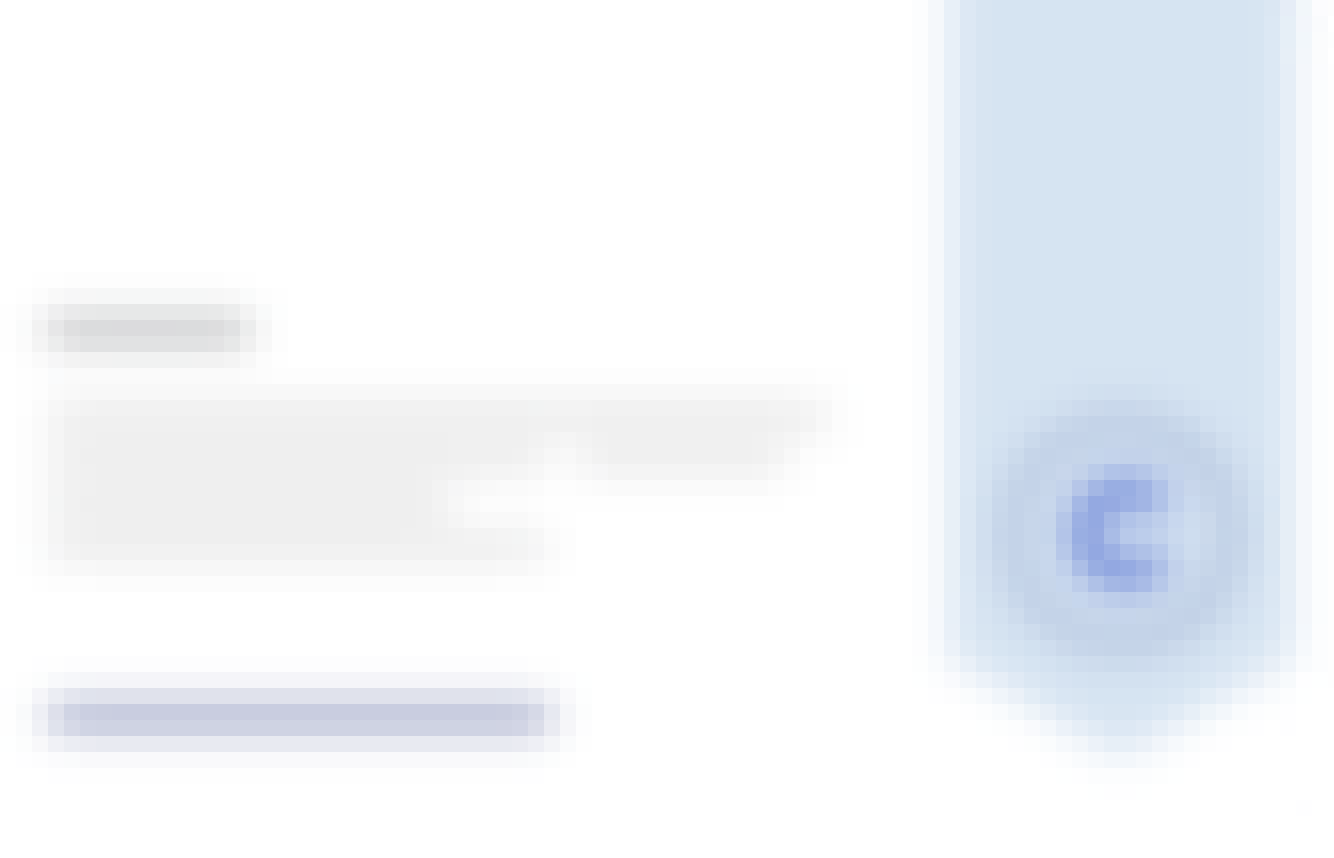
There are 7 modules in this course
This module provides a high-level overview of the main types of inference tasks typically encountered in graphical models: conditional probability queries, and finding the most likely assignment (MAP inference).
What's included
2 videos
This module presents the simplest algorithm for exact inference in graphical models: variable elimination. We describe the algorithm, and analyze its complexity in terms of properties of the graph structure.
What's included
4 videos1 assignment
This module describes an alternative view of exact inference in graphical models: that of message passing between clusters each of which encodes a factor over a subset of variables. This framework provides a basis for a variety of exact and approximate inference algorithms. We focus here on the basic framework and on its instantiation in the exact case of clique tree propagation. An optional lesson describes the loopy belief propagation (LBP) algorithm and its properties.
What's included
9 videos2 assignments1 programming assignment
This module describes algorithms for finding the most likely assignment for a distribution encoded as a PGM (a task known as MAP inference). We describe message passing algorithms, which are very similar to the algorithms for computing conditional probabilities, except that we need to also consider how to decode the results to construct a single assignment. In an optional module, we describe a few other algorithms that are able to use very different techniques by exploiting the combinatorial optimization nature of the MAP task.
What's included
5 videos1 assignment
In this module, we discuss a class of algorithms that uses random sampling to provide approximate answers to conditional probability queries. Most commonly used among these is the class of Markov Chain Monte Carlo (MCMC) algorithms, which includes the simple Gibbs sampling algorithm, as well as a family of methods known as Metropolis-Hastings.
What's included
5 videos2 assignments1 programming assignment
In this brief lesson, we discuss some of the complexities of applying some of the exact or approximate inference algorithms that we learned earlier in this course to dynamic Bayesian networks.
What's included
1 video1 assignment
This module summarizes some of the topics that we covered in this course and discusses tradeoffs between different algorithms. It also includes the course final exam.
What's included
1 video1 assignment
Instructor

Offered by
Why people choose Coursera for their career




Learner reviews
485 reviews
- 5 stars
71.13%
- 4 stars
21.23%
- 3 stars
5.15%
- 2 stars
1.23%
- 1 star
1.23%
Showing 3 of 485
Reviewed on May 28, 2017
I learned pretty much from this course. It answered my quandaries from the representation course, and as well deepened my understanding of PGM.
Reviewed on Mar 9, 2018
Very interesting course. However, even after completing it with honors, I feel like I don't understand a lot.
Reviewed on Nov 7, 2017
Great introduction to inference. Requires some extra reading from the textbook.
Recommended if you're interested in Data Science
Johns Hopkins University
Google Cloud
Coursera Project Network
University of California, Santa Cruz

Open new doors with Coursera Plus
Unlimited access to 10,000+ world-class courses, hands-on projects, and job-ready certificate programs - all included in your subscription
Advance your career with an online degree
Earn a degree from world-class universities - 100% online
Join over 3,400 global companies that choose Coursera for Business
Upskill your employees to excel in the digital economy