As Artificial Intelligence (AI) becomes integrated into high-risk domains like healthcare, finance, and criminal justice, it is critical that those responsible for building these systems think outside the black box and develop systems that are not only accurate, but also transparent and trustworthy. This course is a comprehensive, hands-on guide to Explainable Machine Learning (XAI), empowering you to develop AI solutions that are aligned with responsible AI principles.

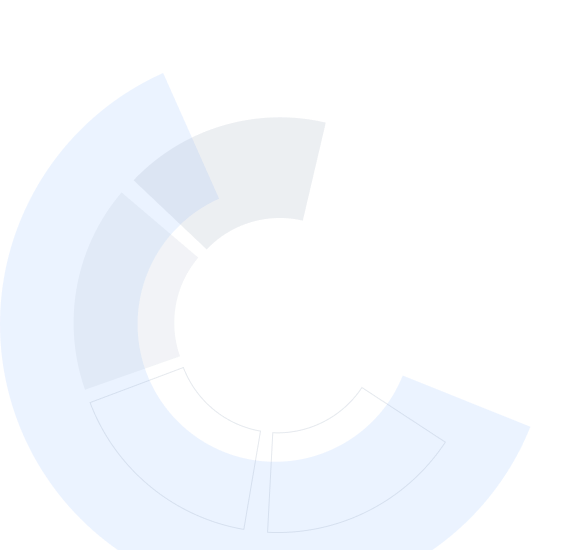
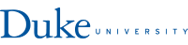
Explainable Machine Learning (XAI)
This course is part of Explainable AI (XAI) Specialization

Instructor: Brinnae Bent, PhD
Sponsored by PTT Global Chemical
Recommended experience
What you'll learn
Explain and implement model-agnostic explainability methods.
Visualize and explain neural network models using SOTA techniques.
Describe emerging approaches to explainability in large language models (LLMs) and generative computer vision.
Details to know

Add to your LinkedIn profile
4 assignments
September 2024
See how employees at top companies are mastering in-demand skills
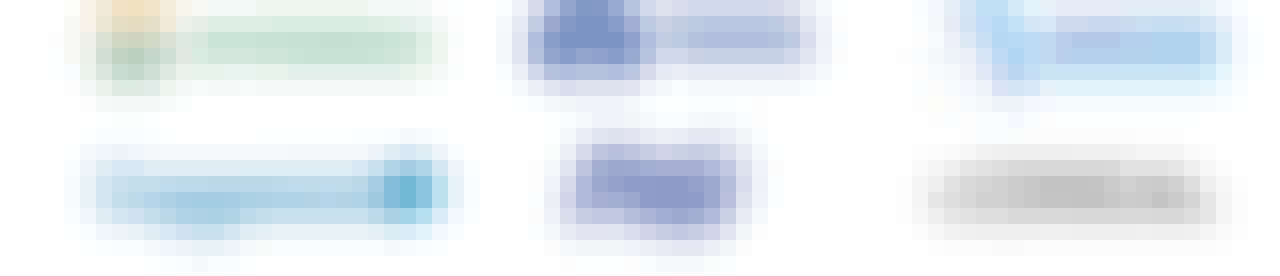
Build your subject-matter expertise
- Learn new concepts from industry experts
- Gain a foundational understanding of a subject or tool
- Develop job-relevant skills with hands-on projects
- Earn a shareable career certificate
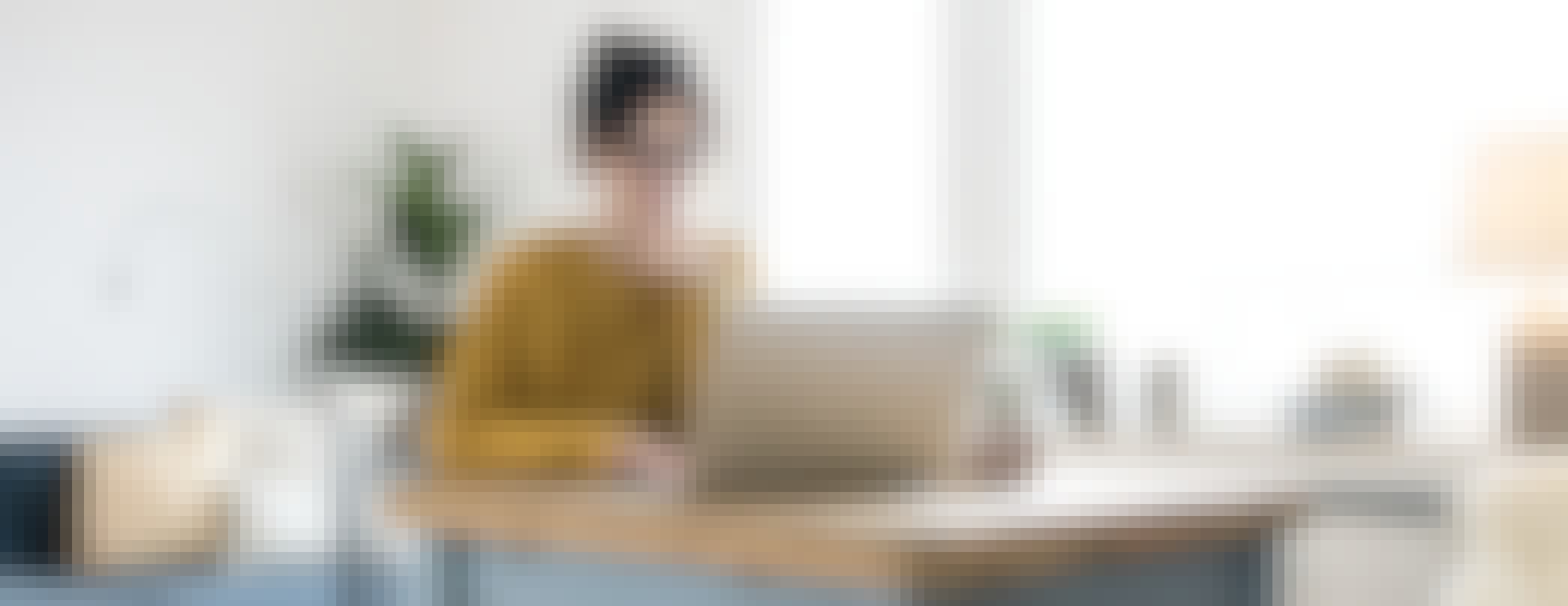
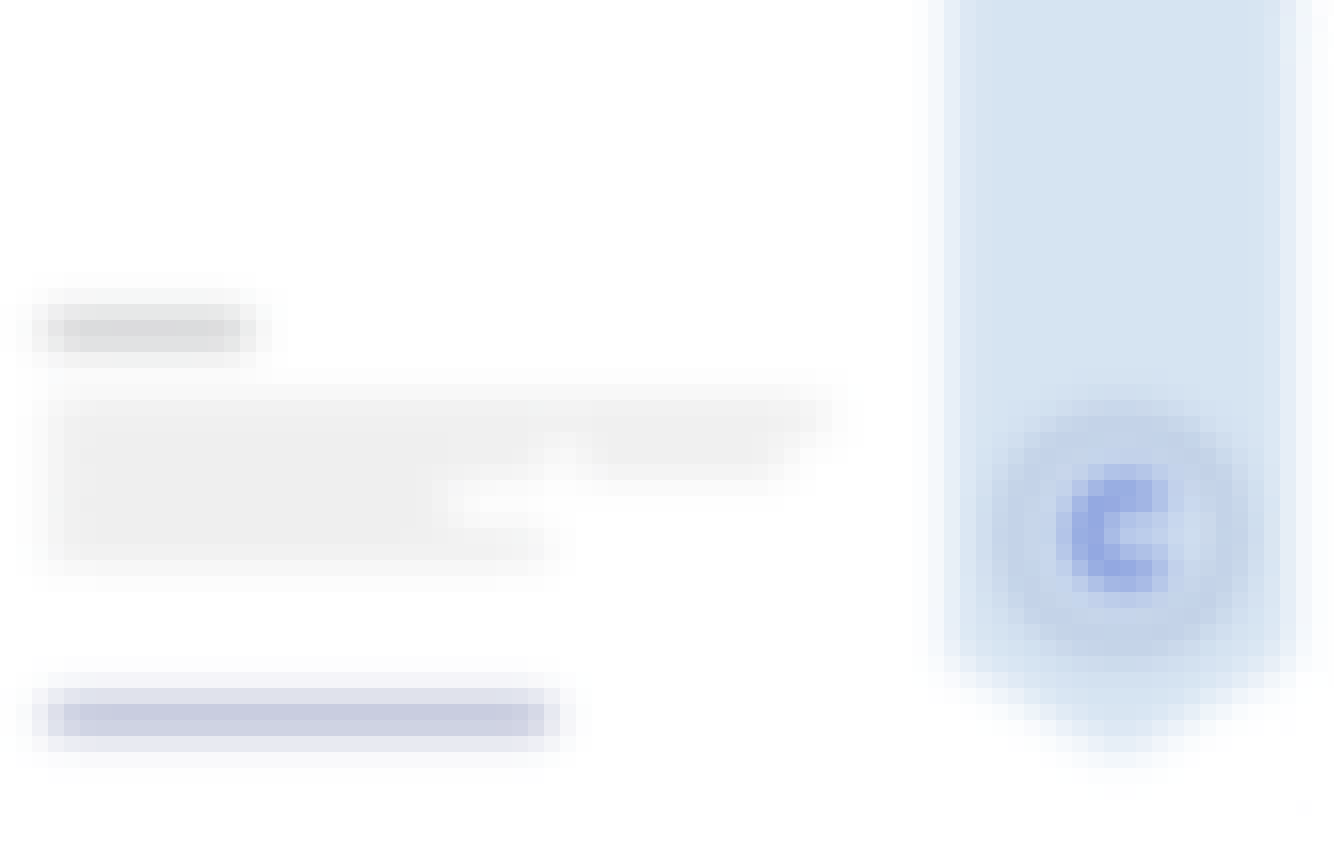
Earn a career certificate
Add this credential to your LinkedIn profile, resume, or CV
Share it on social media and in your performance review
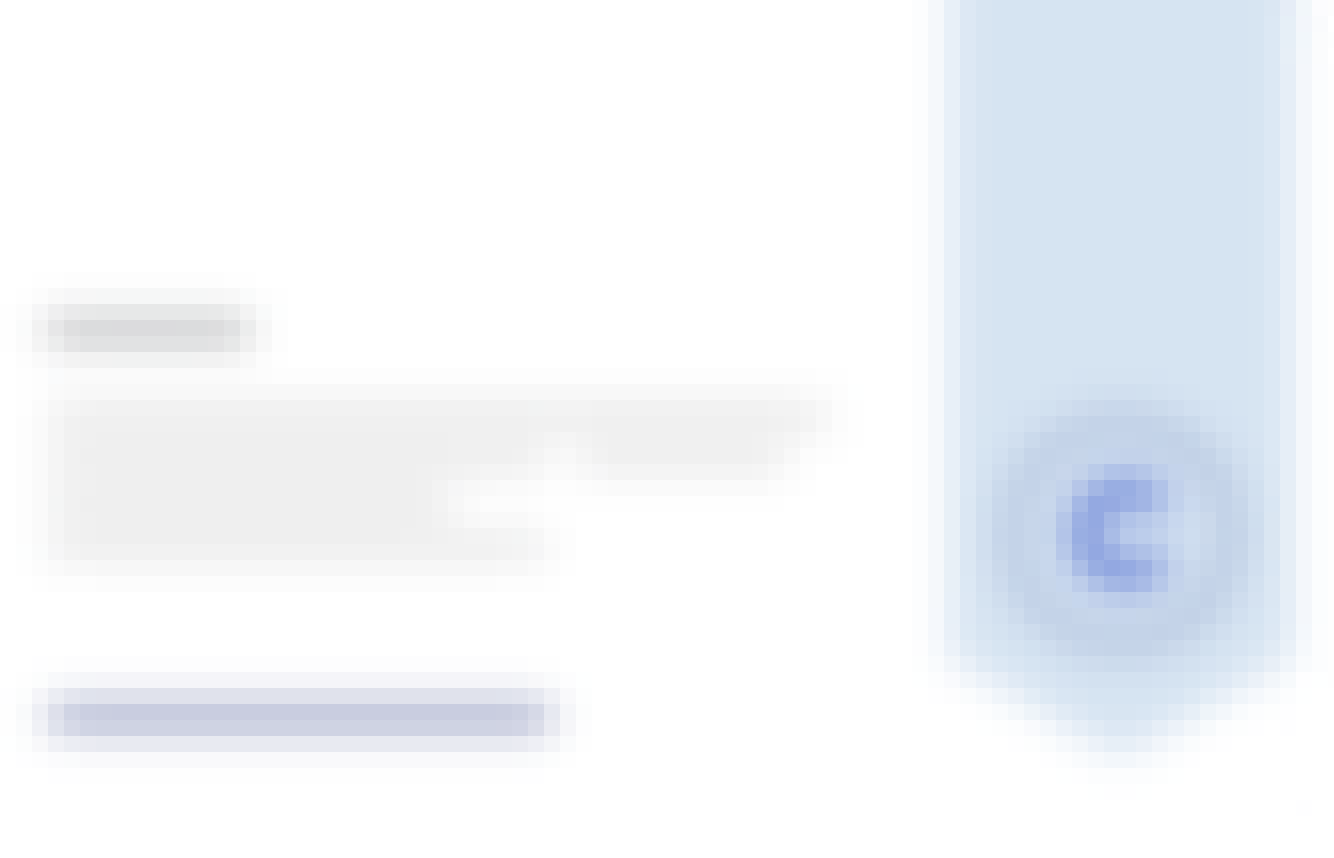
There are 3 modules in this course
In this module, you will be introduced to the concept of model-agnostic explainability and will explore techniques and approaches for local and global explanations. You will learn how to explain and implement local explainability techniques LIME, SHAP, and ICE plots, global explainable techniques including functional decomposition, PDP, and ALE plots, and example-based explanations in Python. You will apply these learnings through discussions, guided programming labs, and a quiz assessment.
What's included
19 videos6 readings1 assignment3 discussion prompts3 ungraded labs
In this module, you will be introduced to the concept of explainable deep learning and will explore techniques and approaches for explaining neural networks. You will learn how to explain and implement neural network visualization techniques, demonstrate knowledge of activation vectors in Python, and recognize and critique interpretable attention and saliency methods. You will apply these learnings through discussions, guided programming labs and case studies, and a quiz assessment.
What's included
8 videos5 readings2 assignments1 discussion prompt2 ungraded labs
In this module, you will be introduced to the concept of explainable generative AI. You will learn how to explain emerging approaches to explainability in LLMs, generative computer vision, and multimodal models. You will apply these learnings through discussions, guided programming labs, and a quiz assessment.
What's included
7 videos3 readings1 assignment2 discussion prompts2 ungraded labs
Instructor

Offered by
Why people choose Coursera for their career




Recommended if you're interested in Data Science
Fred Hutchinson Cancer Center
Johns Hopkins University
Amazon Web Services
University of Michigan

Open new doors with Coursera Plus
Unlimited access to 10,000+ world-class courses, hands-on projects, and job-ready certificate programs - all included in your subscription
Advance your career with an online degree
Earn a degree from world-class universities - 100% online
Join over 3,400 global companies that choose Coursera for Business
Upskill your employees to excel in the digital economy